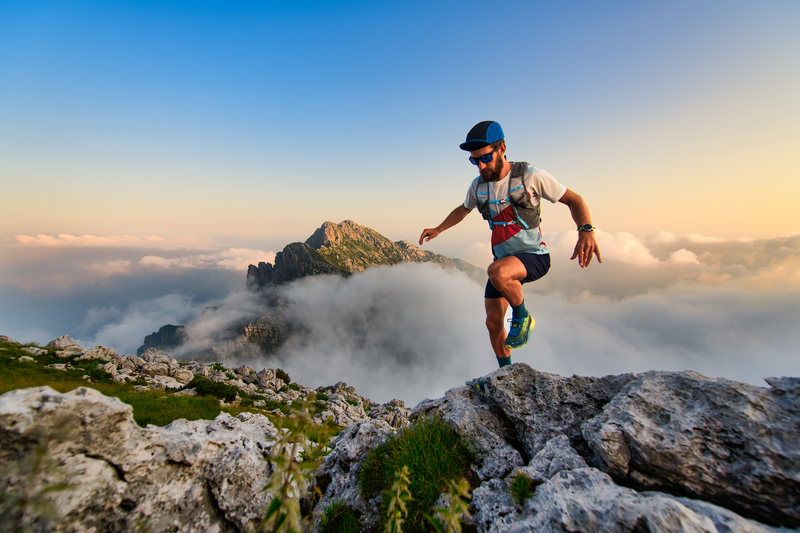
94% of researchers rate our articles as excellent or good
Learn more about the work of our research integrity team to safeguard the quality of each article we publish.
Find out more
ORIGINAL RESEARCH article
Front. Endocrinol. , 31 January 2024
Sec. Clinical Diabetes
Volume 14 - 2023 | https://doi.org/10.3389/fendo.2023.1337071
This article is part of the Research Topic Novel Insights into the Pathophysiology of Diabetes-related Complications: Implications for Improved Therapeutic Strategies, Volume II View all 38 articles
Background: The relationship between type 2 diabetes mellitus (T2DM) and gallstone disease (GSD) have been incompletely understood. We aimed to investigate their phenotypic and genetic associations and evaluate the biological mechanisms underlying these associations.
Methods: We first evaluated the phenotypic association between T2DM and GSD using data from the UK Biobank (n>450,000) using a prospective observational design. We then conducted genetic analyses using summary statistics from a meta-analysis of genome-wide association studies of T2DM, with and without adjusting for body mass index (BMI) (Ncase=74,124, Ncontrol=824,006; T2DMadjBMI: Ncase=50,409, Ncontrol=523,897) and GSD (Ncase=43,639, Ncontrol=506,798).
Results: A unidirectional phenotypic association was observed, where individuals with T2DM exhibited a higher GSD risk (hazard ratio (HR)=1.39, P<0.001), but not in the reverse direction (GSD→T2DM: HR=1.00, P=0.912). The positive T2DM-GSD genetic correlation (rg=0.35, P=7.71×10-23) remained even after adjusting for BMI (T2DMadjBMI: rg=0.22, P=4.48×10-10). Mendelian randomization analyses provided evidence of a unidirectional causal relationship (T2DM→GSD: odds ratio (OR)=1.08, P=4.6×10-8; GSD→T2DM: OR=1.02, P=0.48), even after adjusting for important metabolic confounders (OR=1.02, P=0.02). This association was further corroborated through a comprehensive functional analysis reflected by 23 pleiotropic single nucleotide polymorphisms, as well as multiple neural and motor-enriched tissues.
Conclusion: Through comprehensive observational and genetic analyses, our study clarified the causal relationship between T2DM and GSD, but not in the reverse direction. These findings might provide new insights into prevention and treatment strategies for T2DM and GSD.
Both type 2 diabetes mellitus (T2DM) and gallstone disease (GSD) are prevalent and expensive global public health issues (1, 2). The coexistence of these two conditions, known as multimorbidity, poses complex challenges for clinical management (3). For example, while laparoscopic cholecystectomy is the gold standard treatment for GSD (4), individuals with diabetes are usually high-risk candidates for any surgery. Therefore, it is imperative to comprehend the association between T2DM and GSD, as well as the underlying biological mechanisms, to ensure effective prevention and management of this multimorbidity.
The association between T2DM and GSD has been studied, but results have been inconsistent (5, 6). A Meta-analysis of prospective cohort studies found no association between diabetes and GSD risk (7), while two latest large-scale prospective cohorts reported a 31%-87% increased risk of GSD in individual with diabetes (5, 8). Conversely, three prospective cohort studies shown that GSD increased the risk of T2DM by 17%-42% (6, 9, 10). However, these observational studies are prone to bias, confounding, and reverse causality, making it difficult to establish a causal relationship. Mendelian randomization (MR) studies, which address confounding factors and reverse causation (11) have been used to evaluate the causal relationship between T2DM and GSD. Three MR studies consistently suggest that T2DM causally increases GSD risk (12–14), one study explored and refuted a reverse causal association (6). Despite the knowledge gained from exiting MR analyses advancing our understanding of causal relationships underlying T2DM and GSD, a few major gaps remain. Firstly, the only previous MR suggesting that GSD does not cause T2DM may be inaccurate due to its insufficient statistical power, using merely eight single nucleotide polymorphisms (SNPs) (15). Additionally, multivariable MR (MVMR) can be used to control for pleiotropic impact (16). However, previous MR studies either controlled for no potential confounders (6, 14) or only controlled for body mass index (BMI) (12, 13).
Despite the increasing number of studies reporting evidence between T2DM and GSD, the biological mechanisms linking these two conditions remain unclear. Genome-wide cross-trait analysis presents an opportunity to comprehensively characterize the shared genetic architectures across traits, shedding light on the underlying biological mechanisms of complex diseases (17). Moreover, both T2DM and GSD are moderately heritable, with SNP-heritability estimates of 25% for GSD (18) and 25%-69% for T2DM (19). Large-scale genome-wide association studies (GWASs) have identified a number of disease-associated variants for GSD (20) (N=62) and T2DM (21) (N=386), of which, several risk loci, including PNPLA3, are shared by both conditions (22). However, to the best of our knowledge, no genome-wide cross-trait analysis has been performed to systematically investigate the underlying shared genetic architectures of T2DM and GSD.
Therefore, we conducted a comprehensive bidirectional analysis to investigate the phenotypic and genetic associations between T2DM and GSD. Furthermore, we sought to identify the shared genetic architecture between T2DM and GSD in order to elucidate the underlying biological mechanisms. The study design is outlined in Figure 1.
Figure 1 Overview of the study design, including the analysis methods in each phase. GSD, gallstone disease; GWAS, genome-wide association study; IVs, instrumental variables; PH, proportional hazards; T2DM, type 2 diabetes mellitus.
Our observational analyses used data from UK Biobank (UKB). UK Biobank (UKB) is a large prospective cohort study that enrolled over 500,000 participants aged 40-69 years from England, Wales, and Scotland between 2006 and 2010. The study was approved by the National Health Service North West Multi-Centre Research Ethics Committee and all participants provided written informed consent. The diagnoses of T2DM and GSD were based on the International Classification of Diseases, Tenth Revision (ICD-10) and Ninth Revision (ICD-9), as well as UK-Biobank-specific codes, which are available in Supplementary Table S1. We included only 472,050 white participants and excluded those with a history of events at baseline. Participants who self-reported a history of T2DM/GSD at study enrolment (self-reported non-cancer illness, Data-Field 20002), but did not have an ICD-10 or ICD-9 code for T2DM/GSD were also excluded. The participant selection flow chart is presented in Supplementary Figure S1.
We performed genetic analyses using meta-analysis of GWAS summary data. As instrumental variables (IVs), we selected independent genome-wide significant SNPs (P < 5×10-8). In the European ancestry GWAS of T2DM, which included 898,130 participants (Ncase=74,124, Ncontrol=824,006), we identified 344 T2DM-associated index SNPs (21). Additionally, we incorporated 144 index SNPs associated with T2DM adjusted for BMI (T2DMadjBMI) from a GWAS involving 574,306 participants (Ncase=50,409, Ncontrol=523,897). The genotype data were imputed using the Haplotype Reference Consortium (HRC) reference panel. SNPs with a low imputation quality (INFO <0.4) were excluded. Additionally, SNPs with a minor allele frequency (MAF) < 0.05 were further filtered out. For GSD, the GWAS involving 550,437 European participants (Ncase=43,639, Ncontrol=506,798) identified 62 GSD-associated index SNPs (20). Data were imputed by the HRC.r1-1 reference panel for UBKK and the population-specific SISu v3 imputation reference panel for FinnGen. Subsequently, data were filtered by INFO>0.30, MAF>0.001.
We also collected the largest GWAS data available for BMI (23), Waist-to-hip (WHR) (23), WHRadjBMI (23), fasting insulin (FI) (24), FIadjBMI (25), total cholesterol (TC) (26), triglycerides (TG) (26), high density lipoprotein (HDL) (26), low density lipoprotein (LDL) (26), lipoprotein A (LPA) (27), apolipoprotein A (ApoA) (27), apolipoprotein B (ApoB) (27), smoking (28), and alcohol intake (28) to conduct our MVMR. All these GWAS data were imputed using the reference panel (e.g., HRC) and filtered based on INFO>(0.3~0.5) and MAF>0.01 or 0.001. Supplementary Table S2 provide further details on these GWAS summary data.
To investigate the observational association between T2DM and GSD, we first assessed the proportional hazards (PH) assumption using stphtest in Stata. We then employed flexible parametric survival models (FPSMs) and reported the results as hazard ratios (HRs) with 95% confidence intervals (CIs). This approach allows us to account for time-dependent effects (29). We utilized restricted cubic splines (with four degrees of freedom) in implementation of FPSMs using stpm2 in Stata. The determination of the optimal number of knots and model was based on minimizing the Akaike’s information criterion and Bayesian information criterion (29). Covariates were selected based on existing literature and model selection.
The full model was ultimately implemented using potential confounders extracted from baseline questionnaires, including sex, age, assessment centre, the top 40 genetic principal components, BMI, Townsend deprivation index (TDI), education, smoking, alcohol intake, sleep duration, time spent watching television, physical activity (IPAQ), family history of T2DM, diastolic blood pressure (DBP), systolic blood pressure (SBP), TC, LDL and TG.
To ensure the reliability of our findings, we conducted two sensitivity analyses: 1) excluding participants who experienced events within the first two years after being diagnosed with exposure, to minimize potential reverse causation; and 2) using a flexible parametric competing risk regression model (30), with death as the competing event, to rectify overestimation of the probability for the event of interest occurring over time. All P values were two-sided, with statistical significance set at P<0.05. Survival analyses were performed using Stata version 13 (Stata Corp., College Station, TX).
Genetic correlation represents the average sharing of genetic effect between two traits that is not influenced by environmental confounders. We used linkage-disequilibrium score regression (LDSC) (31) to estimate the global genetic correlation (rg) between T2DM and GSD using GWAS summary data. We utilized pre-calculated HapMap3 LD scores computed from ~1.2 million common SNPs in European ancestry, commonly acknowledged as well-imputed. A Bonferroni-corrected P-value (0.025 = 0.05/2) was used to define statistical significance.
We conducted MR analyses to investigate the causal relationship between T2DM and GSD. Initially, we performed a bidirectional two-sample MR using the TwoSampleMR (https://mrcieu.github.io/TwoSampleMR/). The inverse-variance weighted (IVW) method was utilized as primary analysis (32). To ensure the robustness of our findings, we also performed sensitivity analyses by: 1) using MR-Egger regression (33); 2) using the weighted-median method (34); 3) removing palindromic IVs; and 4) removing pleiotropic IVs. Additionally, we assessed the relevance and exclusion restriction assumption by examining the R2 values and F-statistics (>10) (35) of our IVs and by detecting MR-Egger intercept. A Bonferroni-corrected P-value (0.025 = 0.05/2) was employed.
To further validate the causal relationship identified in the univariable MR analysis, we conducted MVMR and considered potential confounders such as adult BMI, WHR, FI, TC, TG, HDL, LDL, LPA, ApoA, ApoB, smoking, and alcohol intake based on a review of existing literature.
To identify genetic variants with pleiotropic effects, we performed a cross-trait meta-analysis using the Cross-Phenotype Association (CPASSOC) analysis approach (17) with GWAS summary data. The pairwise SHet was used to combine summary statistics as it can maintain statistical power when heterogeneity exists (17). We then obtained independent shared variants using the software PLINK (https://www.cog-genomics.org/plink/1.9/) with parameters (–clump-p1 5e-8 –clump-p2 1e-5 –clump-r2 0.2 –clump-kb 500). The significant index SNP was determined as the variant with Psingle-trait<1×10-5 (both traits) and PCPASSOC<5×10-8. We mapped the nearest gene of these shared SNPs identified by CPASSOC using Ensemble Variant Effect Predictor (https://grch37.ensembl.org/info/docs/tools/vep/index.html).
To investigate the causal SNP for these index SNPs identified from CPASSOC, we performed a fine-mapping (FM) analysis using Bayesian FM method (36) to estimate credible sets of SNPs that had a 99% likelihood of containing the causal SNPs. We estimated a posterior inclusion probability (PIP) for each index SNP using the steepest descent approximation.
We further conducted colocalization analysis to determine whether shared SNPs identified by CPASSOC were shared causal variant or distinct causal variants using a Bayesian method, Coloc (37). A locus was considered colocalized if the posterior probability for H4 (PPH4) exceeded 0.7.
We conducted a transcriptome-wide association study (TWAS) analysis using FUSION (38) to investigate specific tissue-gene pairs shared by T2DM and GSD. Firstly, we performed single-trait TWAS using the expression weights from 49 post-mortem Genotype-Tissue Expression project tissues. Subsequently, we combined the single-trait TWAS result and determined the shared gene-tissue pairs across T2DM and GSD. Bonferroni correction was used to identify expression-trait associations.
The baseline characteristics of UKB participants included in the observational analysis are presented in Supplementary Tables S3 and S4. In terms of the impact of T2DM on GSD risk, 455,405 participants were followed for 5,306,344 person-years (11.7 ± 2.7 years), during which 1,553 participants with T2DM and 14,425 T2DM-free participants developed GSD. The PH assumption test indicated that T2DM and certain covariates, such as BMI, education, and alcohol intake, exhibited a time-dependent effect on GSD risk. The FPSM analysis revealed that participants with T2DM had a higher risk of GSD (HR=1.71, 95%CI=1.58-1.84, P<0.001), which decreased over time when adjusting for sex, age, assessment center, and the top 40 genetic principal components. Additional adjustment for confounders had minimal impact on the hazard of GSD in the final model (HR=1.39, 95% CI=1.29-1.50, P<0.001). Furthermore, the association between T2DM and GSD remained consistent when considering death as a competing event (HR=1.23, 95% CI=1.14-1.32, P<0.001), or when excluding participants who developed GSD within the first two years after T2DM diagnosis (HR=1.17, 95% CI=1.08-1.28, P<0.001) (Table 1).
Regarding the impact of GSD on T2DM risk, 457,608 participants were followed for 5,348,344 person-years (11.7 ± 2.7 years). Among these participants, 1,601 participants with GSD and 22,952 GSD-free participants developed T2DM. The analysis showed participants with GSD had a higher risk of T2DM (HR=1.87, 95%CI=1.78-1.97, P<0.001) in model 1. However, the risk decreased significantly after further adjustment for potential confounders (HR=1.16, 95% CI=1.08-1.24, P<0.001) or when considering death as a competing event. Moreover, the association disappeared when participants who developed T2DM within the first two years after GSD diagnosis were excluded (HR=1.00, 95% CI=0.93-1.08, P=0.91) (Table 1).
After Bonferroni correction, a positive overall genetic correlation was observed between T2DM and GSD (rg=0.35, P=7.71×10-23). The positive overall correlation remained significant even after removing the effect of BMI on T2DM (rg=0.22, P=4.48×10-10) (Supplementary Table S5).
The bidirectional two-sample MR analysis revealed a causal association between T2DM and GSD risk (Figure 2). Genetic liability to T2DM increased the risk of GSD (odds ratio (OR)=1.08, 95%CI=1.05-1.11, P=4.6 ×10-8, PMR-Egger intercept=0.29). However, no causal association was observed between genetic liability to GSD and T2DM risk (OR=1.02, 95%CI=0.96-1.08, P=0.48, PMR-Egger intercept= 0.74). Sensitivity analysis using the MR-Egger (T2DM→GSD: OR=1.05, 95%CI=0.99-1.12, P=0.08; GSD→T2DM: OR=1.01, 95%CI=0.92-1.10, P=0.86) and weighted median (T2DM→GSD: OR=1.05, 95%CI=1.02-1.08, P=0.002; GSD→T2DM: OR=1.00, 95%CI=0.97-1.03, P=0.9) estimator methods supported the unidirectional causal association. Removing palindromic (T2DM→GSD: OR =1.06, 95%CI=1.03-1.09, P=2.7×10-4; GSD→T2DM: OR=1.00, 95%CI=0.95-1.06, P=0.98) and pleiotropic (T2DM→GSD: OR=1.09, 95%CI=1.06-1.13, P=3.6×10-8; GSD→T2DM: OR=1.03, 95%CI=0.95-1.11, P=0.52) IVs also demonstrated similar causal effects. After removing the effect of BMI on T2DM, the causal associations remained almost unchanged (T2DMadjBMI→GSD: OR=1.05, 95%CI=1.01-1.09, P=0.014, PMR-Egger intercept=0.49; GSD→T2DMadjBMI: OR=1.03,95%CI=0.97-1.09, P=0.29, PMR-Egger intercept=0.92). Furthermore, even after adjusting for each potential confounder, T2DM still increased GSD risk (Supplementary Table S6). The final model of MVMR, which adjusting for BMI, WHRadjBMI and FIadjBMI, showed a slightly attenuated yet significant effect size compared to the univariable MR (OR=1.02, 95%CI=1.00-1.03, P=0.02) (Figure 2, Supplementary Table S7).
Figure 2 Univariable and multivariable mendelian randomization analysis between type 2 diabetes mellitus and gallstone disease. CI, confidence intervals; GSD, gallstone disease; MR, mendelian randomization; No. of, the number of; SNPs, single nucleotide polymorphisms; T2DM, type 2 diabetes mellitus; *, removing the effect of body mass index on type 2 diabetes mellitus.
To identify shared genetic architectures and to elucidate the underlying biological mechanisms, we performed cross-trait meta-analysis, fine-mapping analysis and colocalization analysis (Table 2 and Supplementary Tables S8-S15). Cross-trait meta-analysis identified 23 pleiotropic SNPs for T2DM and GSD. The most significant pleiotropic SNP was rs11075985 (PCPASSOC=3.35×10-83) located near FTO, followed by rs1800961 (PCPASSOC=5.69×10-58) and rs1260326 (PCPASSOC=1.00×10-46), located near HNF4A and GCKR, respectively.
Table 2 The cross-trait meta-analysis, fine-mapping analysis and colocalization analysis for type 2 diabetes mellitus and gallstone disease‡.
To investigate the causal SNP for these 23 pleiotropic SNPs, we estimated the 99% credible sets of causal SNPs. A total of 368 potential causal SNPs were obtained from the 23 pleiotropic SNPs. Note that the 99% credible set of rs1800961, rs1260326 and five other pleiotropic SNPs only included themselves. To further distinguish the shared causal SNPs from the distinct ones, we assessed statistical colocalization of these 23 pleiotropic SNPs. The results revealed that 56.2% (containing rs1800961, rs1260326 and 11 other pleiotropic SNPs) of shared loci colocalized at the same candidate causal SNPs. In summary, we found a good number of loci shared by T2DM and GSD, and in particular identified rs1800961 and rs1260326 as potential shared causal variant for T2DM and GSD.
After removing the effect of BMI on T2DM, we identified five additional pleiotropic SNPs, in addition to the ten pleiotropic SNPs also shared by T2DM and GSD. Notably, rs1800961 (PCPASSOC=1.44×10-59) and rs1260326 (PCPASSOC=6.81×10-40) were also identified as the most significant pleiotropic SNPs and shared causal SNPs for T2DM and GSD even after adjusting for BMI.
Regarding gene expressions and biological insights, we used TWAS to explore the tissue-gene pairs shared by both diseases (Table 3, Supplementary Table S16). A total of 31 tissue-gene pairs were observed for T2DM and GSD, including 16 genes (DMWD, GPN1, GTF3C2, IFT172, KRTCAP3, LINC01126, LINC01460, NRBP1, OASL, P2RX4, PPM1G, RBKS, SPPL3, SNX17, THADA, UNC119B), mainly expressed in multiple tissues from nervous and motor systems. After removing the effect of BMI on T2DM, 26 out of the 31 (83.9%) tissue-gene pairs and 13 out of 16 (81.3%) genes remained significant.
Table 3 Shared transcriptome-wide association study significant genes between type 2 diabetes mellitus and gallstone disease.
To our knowledge, this study represents the most comprehensive research on the bidirectional relationships between T2DM and GSD, combining observational and genetic analysis. Our study identified a unidirectional causality running from T2DM to the risk of GSD. Furthermore, we identified specific genetic variants, such as rs1800961 (HNF4A) and rs1260326 (GCKR), that contribute to the biological links between T2DM and GSD. These findings advance our understanding of the complicated relationship underlying T2DM and GSD, providing important implications for preventing and treating these common diseases.
Expanding upon prior research, our study takes a comprehensive approach to investigate the association between T2DM and GSD risk through both observational and MR studies. Our findings, obtained through survival and MR analyses, consistently demonstrate a causal relationship between T2DM and GSD risk. These results align with the updated meta-analysis of prospective cohort studies (RR=1.49, 95%CI=1.09-2.03, P=0.012, Supplementary Figure 2), which pooled data from previous meta-analysis (7) and two recent large-scale studies (5, 8). While in line with the findings of three existing MR studies (12–14), our MR expands previous results in two critical aspects. First, we minimize the influence of reverse causality by applying a bidirectional design. Second, we limit the impact of confounders by adjusting for potential confounding factors using the MVMR. Consequently, we assert that a causal relationship exists between T2DM and GSD risk.
Regarding the association between GSD and T2DM risk, previous studies have reported conflicting results. Some prospective cohort studies (6, 9, 10) have shown a positive association, while one MR study fund no association (6). Our study used both survival and MR analyses and found no causal association between GSD and T2DM risk. The discrepancy between our study and previous cohort studies may be attributed to two aspects. Firstly, two of these previous cohort studies (9, 10) relied on self-reported data, which may have led to misclassification or underestimation of cases. Secondly, observational studies are prone to reverse causality, which the previous cohort study did not account for (6). However, after considering a 2-year lag time, our study found no association between GSD and T2DM. This implies that previous significant findings may have been distorted by reverse causality. MR studies are commonly employed to address reverse causality (11). Our MR analysis replicates the null findings of the previous MR study (6), yet with a much larger sample size (43,639 vs. 1,110 T2DM cases) and more IVs (62 vs. 8). Additionally, our study considered the significant contribution of BMI to T2DM development, which was not accounted for in the previous MR study.
Through cross-trait and colocalization analyses, we identified shared biological mechanisms. Specifically, the key SNPs associated with both conditions were rs1800961 mapped HNF4A and rs1260326 mapped GCKR. HNF4A regulates liver-specific gene expression involved in lipid transport, glucose, and bile metabolism (39). It is also essential for insulin secretion in pancreatic beta cells (40). Additionally, HNF4A has been linked to elevated levels of gamma-glutamyl transferase (GGT), a sensitive marker of cholestasis (41). The effects of HNF4A on insulin action and GGT contribute to the development of both T2DM and GSD. On the other hand, GCKR regulates glucose conversion to glucose-6-phosphate in the liver and pancreas (42, 43). It is associated with various metabolites involved in carbohydrate and lipid metabolism (42). The minor allele of GCKR has been associated with hepatospecific glucokinase activation, reduced plasma insulin levels, and protection against T2DM (42). Additionally, GCKR enhances hepatic cholesterol availability, leading to elevated bile cholesterol concentration and GSD development (43).
Our findings deliver important clinical and public health implications. First, our found that T2DM causally contributes to the development of GSD, but GSD does not increase T2DM risk. This suggests that preventing and treating GSD may not significantly reduce T2DM risk. However, our findings highlight the important of focusing on prevention and treatment of T2DM. Second, while new drugs for T2DM have been developed, further research is needed to explore novel treatments (44). It is noteworthy that certain medications commonly prescribed for T2DM have been associated with an increased risk of GSD in large meta-analyses of randomized controlled trials (45). Furthermore, our genetic analyses reveal shared genetic architectures between T2DM and GSD, which can enhance our understanding of biological mechanisms and potentially identify therapeutic targets for T2DM. For example, our findings suggest that HNF4A and GCKR may be promising targets for T2DM therapies (42), but their effects on GSD and other comorbidities should be considered.
Several limitations should be considered when interpreting the results and conclusions of this study. Firstly, fasting insulin may confound the association between T2DM and GSD risk, which we were unable to adjust for in our multivariable survival analysis due to a lack of information from prospective cohort. However, after adjusting for FI in our MVMR, we confirmed the causal relationship between T2DM and GSD risk. Additionally, dietary behaviors such as egg, vegetable, and fruit intake may act as other confounders for the association between T2DM and GSD. Unfortunately, due to a larger proportion (>50%) of missing data in the prospective cohort, we were unable to adjust for these factors in our multivariable survival analysis. Furthermore, it is worth noting that half of the genetic variants associated with dietary behaviors are a consequence of increased BMI (46). Considering the potential collinearity between BMI and dietary behaviors, we chose not to include the latter in the MVMR analysis. Secondly, our findings were restricted to European population to control for population stratification. However, this may limit the generalizability of our results. Additionally, despite over 90% of gallstones in Europe are cholesterol gallstones, there are also pigment gallstones with different etiologies, which were not specifically investigated in our study.
Our study demonstrates an association between T2DM and GSD risk at both the phenotypic and genetic levels using large-scale prospective cohort and GWAS data. This association is independent of BMI, WHR, and FI, suggesting an intrinsic and causal relationship. However, no causal association was found between GSD and T2DM risk. Furthermore, the shared genetic architecture between GSD and T2DM enhance our understanding of the underlying biological mechanisms. These findings might offer valuable insights for the identification of potential therapeutic targets for T2DM and novel perspectives on preventing GSD, ultimately contributing to a decrease in multimorbidity incidence.
Publicly available datasets were analyzed in this study. This data can be found here: The UK Biobank analysis was conducted with the application 50538. GWAS summary statistics for type 2 diabetes mellitus are publicly available through the DIAGRAM consortium (http://diagram-consortium.org/downloads.html). GWAS summary statistics for gallstone disease were applied by the corresponding authors.
Ethical approval was not required for the study involving humans in accordance with the local legislation and institutional requirements. Written informed consent to participate in this study was not required from the participants or the participants’ legal guardians/next of kin in accordance with the national legislation and the institutional requirements.
PY: Conceptualization, Data curation, Formal Analysis, Methodology, Visualization, Writing – original draft, Writing – review & editing. LZ (2nd author): Conceptualization, Data curation, Formal Analysis, Methodology, Visualization, Writing – original draft, Writing – review & editing. CY: Conceptualization, Data curation, Formal Analysis, Methodology, Visualization, Writing – original draft, Writing – review & editing. WZ: Data curation, Writing – review & editing. YW: Data curation, Writing – review & editing. MZ: Data curation, Writing – review & editing. HC: Data curation, Writing – review & editing. MT: Data curation, Writing – review & editing. LC: Data curation, Writing – review & editing. XW: Data curation, Writing – review & editing. XZ: Data curation, Writing – review & editing. YZ: Data curation, Writing – review & editing. JX: Data curation, Writing – review & editing. YL: Data curation, Writing – review & editing. CX: Data curation, Writing – review & editing. YFY: Methodology, Writing – review & editing. LZ (17th author): Methodology, Writing – review & editing. YQY: Methodology, Writing – review & editing. JL: Methodology, Writing – review & editing. ZL: Methodology, Writing – review & editing. CXY: Methodology, Writing – review & editing. XJ: Conceptualization, Methodology, Supervision, Writing – original draft, Writing – review & editing. BZ: Conceptualization, Funding acquisition, Methodology, Supervision, Writing – review & editing.
The author(s) declare financial support was received for the research, authorship, and/or publication of this article. This work was supported by the National Key R&D Program of China (2022YFC3600600, 2022YFC3600604), the National Natural Science Foundation of China (U22A20359, 81874283, 81673255), the Recruitment Program for Young Professionals of China, the Promotion Plan for Basic Medical Sciences and the Development Plan for Cutting-Edge Disciplines, Sichuan University, and other Projects from West China School of Public Health and West China Fourth Hospital, Sichuan University. The funder had no role in study design, data collection, analysis, interpretation, writing of the report, or the decision for submission.
We are grateful to all the investigators who shared genome-wide summary statistics.
The authors declare that the research was conducted in the absence of any commercial or financial relationships that could be construed as a potential conflict of interest.
All claims expressed in this article are solely those of the authors and do not necessarily represent those of their affiliated organizations, or those of the publisher, the editors and the reviewers. Any product that may be evaluated in this article, or claim that may be made by its manufacturer, is not guaranteed or endorsed by the publisher.
The Supplementary Material for this article can be found online at: https://www.frontiersin.org/articles/10.3389/fendo.2023.1337071/full#supplementary-material
1. Tomic D, Morton JI, Chen L, Salim A, Gregg EW, Pavkov ME, et al. Lifetime risk, life expectancy, and years of life lost to type 2 diabetes in 23 high-income jurisdictions: a multinational, population-based study. Lancet Diabetes Endocrinol (2022) 10:795–803. doi: 10.1016/s2213-8587(22)00252-2
2. Kharazmi E, Scherer D, Boekstegers F, Liang Q, Sundquist K, Sundquist J, et al. Gallstones, cholecystectomy, and kidney cancer: observational and Mendelian randomization results based on large cohorts. Gastroenterology (2023) 165:218–27.e8. doi: 10.1053/j.gastro.2023.03.227
3. Skou ST, Mair FS, Fortin M, Guthrie B, Nunes BP, Miranda JJ, et al. Multimorbidity. Nat Rev Dis Primers (2022) 8:48. doi: 10.1038/s41572-022-00376-4
4. Jensen SAS, Fonnes S, Gram-Hanssen A, Andresen K, Rosenberg J. Long-term mortality and intestinal obstruction after laparoscopic cholecystectomy: A systematic review and meta-analysis. Int J Surg (2022) 105:106841. doi: 10.1016/j.ijsu.2022.106841
5. Unalp-Arida A, Ruhl CE. Increasing gallstone disease prevalence and associations with gallbladder and biliary tract mortality in the US. Hepatology (2023) 77:1882–95. doi: 10.1097/hep.0000000000000264
6. Wang F, Wang J, Li Y, Yuan J, Yao P, Wei S, et al. Gallstone disease and type 2 diabetes risk: A Mendelian randomization study. Hepatology (2019) 70:610–20. doi: 10.1002/hep.30403
7. Aune D, Vatten LJ. Diabetes mellitus and the risk of gallbladder disease: A systematic review and meta-analysis of prospective studies. J Diabetes Complications (2016) 30:368–73. doi: 10.1016/j.jdiacomp.2015.11.012
8. Colvin HS, Kimura T, Iso H, Ikehara S, Sawada N, Tsugane S. Risk factors for gallstones and cholecystectomy: A large-scale population-based prospective cohort study in Japan. Dig Dis (2022) 40:385–93. doi: 10.1159/000517270
9. Lv J, Yu C, Guo Y, Bian Z, Yang L, Chen Y, et al. Gallstone disease and the risk of type 2 diabetes. Sci Rep (2017) 7:15853. doi: 10.1038/s41598-017-14801-2
10. Weikert C, Weikert S, Schulze MB, Pischon T, Fritsche A, Bergmann MM, et al. Presence of gallstones or kidney stones and risk of type 2 diabetes. Am J Epidemiol (2010) 171:447–54. doi: 10.1093/aje/kwp411
11. Burgess S, Thompson DJ, Rees JMB, Day FR, Perry JR, Ong KK. Dissecting causal pathways using Mendelian randomization with summarized genetic data: application to age at menarche and risk of breast cancer. Genetics (2017) 207:481–7. doi: 10.1534/genetics.117.300191
12. Yuan S, Gill D, Giovannucci EL, Larsson SC. Obesity, type 2 diabetes, lifestyle factors, and risk of gallstone disease: A Mendelian randomization investigation. Clin Gastroenterol Hepatol (2022) 20:e529–e37. doi: 10.1016/j.cgh.2020.12.034
13. Chen J, Yuan S, Fu T, Ruan X, Qiao J, Wang X, et al. Gastrointestinal consequences of type 2 diabetes mellitus and impaired glycemic homeostasis: A Mendelian randomization study. Diabetes Care (2023) 46:828–35. doi: 10.2337/dc22-1385
14. Chen L, Yang H, Li H, He C, Yang L, Lv G. Insights into modifiable risk factors of cholelithiasis: A Mendelian randomization study. Hepatology (2022) 75:785–96. doi: 10.1002/hep.32183
15. Danford CJ, Ezaz G. Letter to the editor: hypotheses and assumptions in Mendelian randomization: proceed with caution. Hepatology (2019) 70:449–50. doi: 10.1002/hep.30599
16. Sanderson E, Davey Smith G, Windmeijer F, Bowden J. An examination of multivariable Mendelian randomization in the single-sample and two-sample summary data settings. Int J Epidemiol (2019) 48:713–27. doi: 10.1093/ije/dyy262
17. Zhu X, Feng T, Tayo BO, Liang J, Young JH, Franceschini N, et al. Meta-analysis of correlated traits via summary statistics from GWASs with an application in hypertension. Am J Hum Genet (2015) 96:21–36. doi: 10.1016/j.ajhg.2014.11.011
18. Katsika D, Grjibovski A, Einarsson C, Lammert F, Lichtenstein P, Marschall HU. Genetic and environmental influences on symptomatic gallstone disease: a Swedish study of 43,141 twin pairs. Hepatology (2005) 41:1138–43. doi: 10.1002/hep.20654
19. Almgren P, Lehtovirta M, Isomaa B, Sarelin L, Taskinen MR, Lyssenko V, et al. Heritability and familiality of type 2 diabetes and related quantitative traits in the Botnia Study. Diabetologia (2011) 54:2811–9. doi: 10.1007/s00125-011-2267-5
20. Fairfield CJ, Drake TM, Pius R, Bretherick AD, Campbell A, Clark DW, et al. Genome-wide analysis identifies gallstone-susceptibility loci including genes regulating gastrointestinal motility. Hepatology (2021) 75:1081–94. doi: 10.1002/hep.32199
21. Mahajan A, Taliun D, Thurner M, Robertson NR, Torres JM, Rayner NW, et al. Fine-mapping type 2 diabetes loci to single-variant resolution using high-density imputation and islet-specific epigenome maps. Nat Genet (2018) 50:1505–13. doi: 10.1038/s41588-018-0241-6
22. Sakaue S, Kanai M, Tanigawa Y, Karjalainen J, Kurki M, Koshiba S, et al. A cross-population atlas of genetic associations for 220 human phenotypes. Nat Genet (2021) 53:1415–24. doi: 10.1038/s41588-021-00931-x
23. Pulit SL, Stoneman C, Morris AP, Wood AR, Glastonbury CA, Tyrrell J, et al. Meta-analysis of genome-wide association studies for body fat distribution in 694 649 individuals of European ancestry. Hum Mol Genet (2019) 28:166–74. doi: 10.1093/hmg/ddy327
24. Lagou V, Mägi R, Hottenga J-J, Grallert H, Perry JRB, Bouatia-Naji N, et al. Sex-dimorphic genetic effects and novel loci for fasting glucose and insulin variability. Nat Commun (2021) 12:24. doi: 10.1038/s41467-020-19366-9
25. Chen J, Spracklen CN, Marenne G, Varshney A, Corbin LJ, Luan J, et al. The trans-ancestral genomic architecture of glycemic traits. Nat Genet (2021) 53:840–60. doi: 10.1038/s41588-021-00852-9
26. Graham SE, Clarke SL, Wu KH, Kanoni S, Zajac GJM, Ramdas S, et al. The power of genetic diversity in genome-wide association studies of lipids. Nature (2021) 600:675–9. doi: 10.1038/s41586-021-04064-3
27. Sinnott-Armstrong N, Tanigawa Y, Amar D, Mars N, Benner C, Aguirre M, et al. Genetics of 35 blood and urine biomarkers in the UK Biobank. Nat Genet (2021) 53:185–94. doi: 10.1038/s41588-020-00757-z
28. Liu M, Jiang Y, Wedow R, Li Y, Brazel DM, Chen F, et al. Association studies of up to 1.2 million individuals yield new insights into the genetic etiology of tobacco and alcohol use. Nat Genet (2019) 51:237–44. doi: 10.1038/s41588-018-0307-5
30. Mozumder SI, Rutherford MJ, Lambert PC. A flexible parametric competing risks model using a direct likelihood approach for the cause-specific cumulative incidence function. Stata J (2017) 17:462–89. doi: 10.1177/1536867X1701700212
31. Bulik-Sullivan B, Finucane HK, Anttila V, Gusev A, Day FR, Loh PR, et al. An atlas of genetic correlations across human diseases and traits. Nat Genet (2015) 47:1236–41. doi: 10.1038/ng.3406
32. Burgess S, Scott RA, Timpson NJ, Davey Smith G, Thompson SG, Consortium E-I. Using published data in Mendelian randomization: a blueprint for efficient identification of causal risk factors. Eur J Epidemiol (2015) 30:543–52. doi: 10.1007/s10654-015-0011-z
33. Bowden J, Davey Smith G, Burgess S. Mendelian randomization with invalid instruments: effect estimation and bias detection through Egger regression. Int J Epidemiol (2015) 44:512–25. doi: 10.1093/ije/dyv080
34. Bowden J, Davey Smith G, Haycock PC, Burgess S. Consistent estimation in Mendelian randomization with some invalid instruments using a weighted median estimator. Genet Epidemiol (2016) 40:304–14. doi: 10.1002/gepi.21965
35. Pierce BL, Ahsan H, Vanderweele TJ. Power and instrument strength requirements for Mendelian randomization studies using multiple genetic variants. Int J Epidemiol (2011) 40:740–52. doi: 10.1093/ije/dyq151
36. Huang H, Fang M, Jostins L, Umicevic Mirkov M, Boucher G, Anderson CA, et al. Fine-mapping inflammatory bowel disease loci to single-variant resolution. Nature (2017) 547:173–8. doi: 10.1038/nature22969
37. Giambartolomei C, Vukcevic D, SChadt EE, Franke L, Hingorani AD, Wallace C, et al. Bayesian test for colocalisation between pairs of genetic association studies using summary statistics. PloS Genet (2014) 10:e1004383. doi: 10.1371/journal.pgen.1004383
38. Gusev A, Ko A, Shi H, Bhatia G, Chung W, Penninx BW, et al. Integrative approaches for large-scale transcriptome-wide association studies. Nat Genet (2016) 48:245–52. doi: 10.1038/ng.3506
39. Hwang-Verslues WW, Sladek FM. HNF4α–role in drug metabolism and potential drug target? Curr Opin Pharmacol (2010) 10:698–705. doi: 10.1016/j.coph.2010.08.010
40. Gupta RK, Vatamaniuk MZ, Lee CS, Flaschen RC, Fulmer JT, Matschinsky FM, et al. The MODY1 gene HNF-4alpha regulates selected genes involved in insulin secretion. J Clin Invest (2005) 115:1006–15. doi: 10.1172/jci22365
41. Gellert-Kristensen H, Dalila N, Fallgaard Nielsen S, Grønne Nordestgaard B, Tybjaerg-Hansen A, Stender S. Identification and replication of six loci associated with gallstone disease. Hepatology (2019) 70:597–609. doi: 10.1002/hep.30313
42. Brouwers M, Jacobs C, Bast A, Stehouwer CDA, Schaper NC. Modulation of glucokinase regulatory protein: A double-edged sword? Trends Mol Med (2015) 21:583–94. doi: 10.1016/j.molmed.2015.08.004
43. Ford BE, Chachra SS, Rodgers K, Moonira T, Al-Oanzi ZH, Anstee QM, et al. The GCKR-P446L gene variant predisposes to raised blood cholesterol and lower blood glucose in the P446L mouse-a model for GCKR rs1260326. Mol Metab (2023) 72:101722. doi: 10.1016/j.molmet.2023.101722
44. Nauck MA, Wefers J, Meier JJ. Treatment of type 2 diabetes: challenges, hopes, and anticipated successes. Lancet Diabetes Endocrinol (2021) 9:525–44. doi: 10.1016/S2213-8587(21)00113-3
45. He L, Wang J, Ping F, Yang N, Huang J, Li W, et al. Dipeptidyl peptidase-4 inhibitors and gallbladder or biliary disease in type 2 diabetes: systematic review and pairwise and network meta-analysis of randomised controlled trials. BMJ (2022) 377:e068882. doi: 10.1136/bmj-2021-068882
Keywords: type 2 diabetes mellitus, gallstone disease, Mendelian randomization, bidirectional, causal relationship, genetic association, cohort
Citation: Yan P, Zhang L, Yang C, Zhang W, Wang Y, Zhang M, Cui H, Tang M, Chen L, Wu X, Zhao X, Zou Y, Xiao J, Liu Y, Xiao C, Yang Y, Zhang L, Yao Y, Li J, Liu Z, Yang C, Jiang X and Zhang B (2024) Observational and genetic analyses clarify the relationship between type 2 diabetes mellitus and gallstone disease. Front. Endocrinol. 14:1337071. doi: 10.3389/fendo.2023.1337071
Received: 12 November 2023; Accepted: 26 December 2023;
Published: 31 January 2024.
Edited by:
Xuebin Fu, Ann & Robert H. Lurie Children’s Hospital of Chicago, United StatesCopyright © 2024 Yan, Zhang, Yang, Zhang, Wang, Zhang, Cui, Tang, Chen, Wu, Zhao, Zou, Xiao, Liu, Xiao, Yang, Zhang, Yao, Li, Liu, Yang, Jiang and Zhang. This is an open-access article distributed under the terms of the Creative Commons Attribution License (CC BY). The use, distribution or reproduction in other forums is permitted, provided the original author(s) and the copyright owner(s) are credited and that the original publication in this journal is cited, in accordance with accepted academic practice. No use, distribution or reproduction is permitted which does not comply with these terms.
*Correspondence: Xia Jiang, eGlhamlhbmdAc2N1LmVkdS5jbg==; Ben Zhang, d2NzcGh3Y2ZoQHZpcC4xNjMuY29t
Disclaimer: All claims expressed in this article are solely those of the authors and do not necessarily represent those of their affiliated organizations, or those of the publisher, the editors and the reviewers. Any product that may be evaluated in this article or claim that may be made by its manufacturer is not guaranteed or endorsed by the publisher.
Research integrity at Frontiers
Learn more about the work of our research integrity team to safeguard the quality of each article we publish.