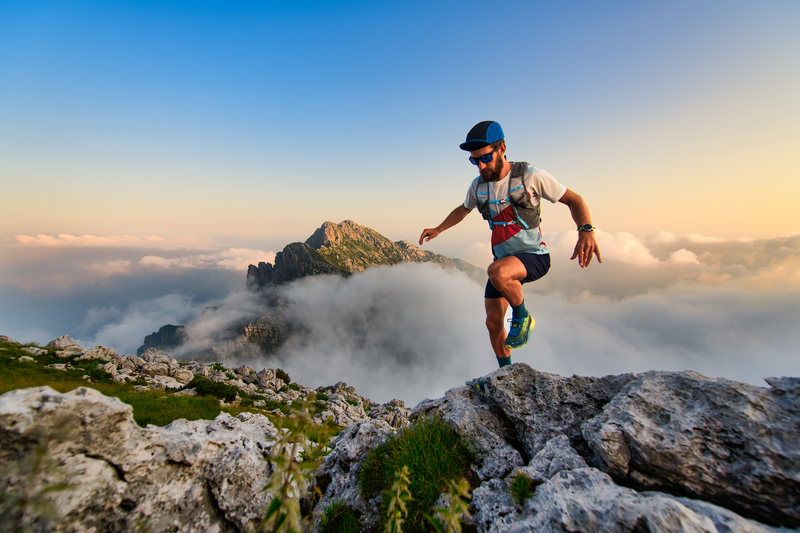
95% of researchers rate our articles as excellent or good
Learn more about the work of our research integrity team to safeguard the quality of each article we publish.
Find out more
ORIGINAL RESEARCH article
Front. Endocrinol. , 16 January 2024
Sec. Obesity
Volume 14 - 2023 | https://doi.org/10.3389/fendo.2023.1325454
This article is part of the Research Topic Obesity and Type 2 Diabetes Mellitus: Novel and Alternative Functional Bioactive Nutritional Interventions View all 12 articles
Objective: To examine the association between the weight-adjusted waist index (WWI) and the odds of type 2 diabetes mellitus(T2DM)among U.S. adults.
Methods: Data from the National Health and Nutrition Examination Survey (NHANES) spanning six years (2007–2018) were utilized, encompassing 31001 eligible participants. Weighted multivariate logistic regression models and smoothed fit curves were employed to assess the association between WWI and the odds of T2DM, as well as dose-response relationships in the overall population and the odds of T2DM in various subgroups.
Results: In the fully adjusted continuous model, each one-unit increase in WWI was associated with a 1.14-fold increase in the odds of T2DM within the entire study population (2.14 [1.98,2.31], P < 0.0001). In the fully adjusted categorical model, when using the lowest tertile of WWI (T1) as the reference group, the second tertile (T2) and the third tertile (T3) were associated with a 0.88-fold (1.88 [1.64,2.17], P < 0.0001) and a 2.63-fold (3.63 [3.11,4.23], P < 0.0001) increase in the odds of T2DM. These findings indicated a positive correlation between WWI values and the odds of T2DM, aligning with the results of the smoothed-fitted curves. In the analysis of subgroups, in addition to maintaining consistency with the overall population results, we found interactions between age and hypertension subgroups.
Conclusion: In conclusion, WWI was found to be positively associated with the odds of T2DM in U.S. adults.
Type 2 diabetes mellitus (T2DM) stands as a chronic metabolic disorder known for its hallmark features of insulin resistance and elevated blood glucose levels. Once diagnosed, it typically proves challenging to reverse, and it frequently leads to complications affecting the kidneys, retinal health, cardiovascular system, neural function, and liver. Regrettably, effective treatments for this condition have been lacking (1). Recent statistics indicate a concerning trend, with the prevalence of diabetes projected to surge to 578 million individuals by 2030 (2). Therefore, we must take effective measures to identify people at risk of developing diabetes and to give relevant preventive guidance and advice promptly, to achieve the goal of slowing down the onset and development of diabetes.
Globally, the rates of obesity have reached epidemiological levels and are impacting an increasing population of individuals (3–5). Studies have demonstrated a strong association between obesity and the development of type 2 diabetes (6). Although Body Mass Index (BMI) is the most commonly used method to assess obesity, an important limitation of applying BMI is that it does not reflect the true body fat distribution (7). Waist circumference (WC) is a simple and reliable method for assessing abdominal obesity and has been used to measure total body fat (8), but fails to distinguish between subcutaneous and visceral fat (9). It has been shown that visceral fat produces more free fatty acids than subcutaneous fat, which increases insulin resistance and the risk of diabetes (10, 11). Quantifying visceral fat and muscle mass by applying Computer Tomography (CT) or Magnetic Resonance Imaging (MRI) lacks feasibility in clinical work. Therefore, Park Y et al. proposed a novel index of obesity called the “weight-adjusted waist circumference index (WWI)” (12). The WWI is calculated as waist circumference (WC) divided by the square root of body weight, which not only weakens the relationship with BMI but also combines the advantages of WC to provide a better reflection of body fat distribution and muscle mass, as well as reflecting the problem of central obesity, which is not related to body weight (13). Therefore, WWI has potential advantages as a predictor of obesity.
Previous studies have shown a positive association between WWI and the prevalence of type 2 diabetes (14, 15), but these studies have been conducted in Asian countries, and there is a lack of research on the association between WWI and the prevalence of type 2 diabetes in the US population. Therefore, we applied a large sample of data obtained from the NHANES database from 2007 to 2018 to further validate the potential relationship between WWI and the odds of T2DM.
NHANES, a nationally representative cross-sectional study, was purposefully designed to evaluate the health and nutritional status of an ambulatory population within the United States. The U.S. Centers for Disease Control and Prevention secured approval from the Research Ethics Review Board for this study, and all participants provided written informed consent, obviating the need for further ethical review. The present study adhered to the guidelines outlined in the Epidemiologic Statement for Enhanced Reporting of Observational Studies (16).
For the investigation, a comprehensive screening of the entire 2007-2018 NHANES database was carried out, encompassing a total of 59842 participants. To ensure the relevance and accuracy of the study, specific exclusion criteria were applied. Participants who were younger than 20 years old were excluded, resulting in the exclusion of 25072 participants. Additionally, participants with missing data on the waist circumference were excluded, leading to the exclusion of 3385 participants. Participants with missing data on weight were excluded, leading to the exclusion of 40 participants. Moreover, those with incomplete records regarding a diagnosis of T2DM were also excluded, accounting for an additional 344 participants. Consequently, the final dataset analyzed for the study consisted of 31001 subjects. Further details are illustrated in Figure 1.
Under the 2013 U.S. Diabetes Guidelines (17), T2DM was categorized as meeting any of the following criteria: 1) self-reported physician-diagnosed T2DM; 2)Currently taking hypoglycemic drugs or injecting insulin;3) random blood glucose level equal to or exceeding 11.1 mmol/L; 4) glycosylated hemoglobin (HbA1c) level equal to or exceeding 6.5%; 5) fasting blood glucose level equal to or exceeding 7.0 mmol/L; 6) 2-h blood glucose level (determined by oral glucose tolerance test (OGTT)) equal to or exceeding 11.1 mmol/L. In the analysis, the incidence of T2DM was regarded as the outcome of interest.
WWI (cm/√kg) was measured by calculating WC (cm) divided by the square root of the weight (kg). Anthropometric measurements were recorded by trained medical personnel and specialized recorders to ensure the exact accuracy of the data. Body weight was determined using a digital scale. Participants were asked to wear their examination clothing, and then stand barefoot on the digital scale with arms held close to their bodies and gaze fixed straight ahead, as outlined previously (18). WC was calculated using a tape measure, which was positioned at the intersection of the midaxillary line and the horizontal line just above the outermost upper point of the right kneecap (19). The WWI value was used as an exposure variable in this study.
Covariates, such as age, gender, race/ethnicity (Non-Hispanic white, Non-Hispanic black, Mexican-American, etc.), educational level (less than high school, high school or equivalent, college or above), marital status (married/living with partner, never married, widowed/divorced/separated), family poverty-to-income ratio (PIR), smoking status (never, now, former), alcohol user (never, former, mild, moderate, heavy), Physical activity(PA, Mets), Alanine aminotransferase (ALT, U/L), Aspartate aminotransferase (AST, U/L), creatinine(umol/L), uric acid(UA,umol/L), triglycerides (TG, mmol/L), high-density lipoprotein-cholesterol (HDL-C, mmol/L), total cholesterol (TC, mmol/L). Hypertension was defined as meeting any of the following criteria: 1) self-reported physician diagnosis of hypertension; 2) current use of antihypertensive medication; 3) an average of three measurements of systolic blood pressure (SBP) exceeding 140 mmHg and/or diastolic blood pressure (DBP) exceeding 90 mmHg. Hyperlipidemia can be diagnosed by meeting any of the following conditions: 1) Diagnosed as hypertriglyceridemia (TG>=150mg/dL or>=1.70mmol/L); 2) Diagnosis of hypercholesterolemia can be made by meeting any of the following conditions: 1. TC>=200mg/dL (>=5.18mmol/L) 2. Low-density lipoprotein-cholesterol(LDL-C)>=130mg/dL (>=3.37mmol/L) 3. Male HDL<40mg/dL (<1.04mmol/L), female HDL<50mg/dL (<1.30mmol/L); 3) Currently taking lipid-lowering medication. The diagnosis of coronary heart disease (CHD) was determined based on self-reported responses to the NHANES questionnaire. The calculation of physical activity is based on multiplying the number of days (in days/week) of specific activities per week by the duration of specific activities per day (in minutes/day) to calculate the resulting exercise metabolic equivalents (METs). Stroke diagnoses were derived from self-reported stroke diagnoses from the questionnaire. Some covariates had missing values, although the extent of missing data for each variable was less than 20%. These missing values were addressed through the utilization of the “mice” R package, employing multiple interpolations to impute the missing data for continuous variables.
The statistical analysis was conducted using R 4.3.1 software. The analysis incorporated the variables for weights, sdmvstra, and sdmvpsu. Continuous variables were expressed as mean ± standard deviation, while categorical variables were presented as numbers and percentages. To make the comparison between the two groups, weighted Student’s t-test, Mann-Whitney U test, and the Chi-square test were utilized.
To explore the relationship between WWI level and the odds of developing T2DM, the multivariate logistic regression model was employed. Odds ratios (ORs) and their corresponding 95% confidence intervals (CIs) were computed using the “survey” R package. Model 1 was unadjusted for any covariates. Model 2 was adjusted for age, gender, and race/ethnicity. Model 3 further included adjustments for educational level, marital status, PIR, smoking status, alcohol user, ALT, AST, creatinine, UA, TC, TG, HDL, hypertension, CHD, stroke, and Hyperlipidemia. The multivariate logistic regression model was divided into categorical and continuous models. In the categorical model, WWI values were categorized into thirds, and nonlinear trends were assessed by treating the median value of each trichotomy as a continuous variable.
In addition, subgroup analysis was conducted to indicate whether age, gender, race/ethnicity, hypertension, CHD, stroke, and Hyperlipidemia were associated with the odds of T2DM in different population subgroups. The level of statistical significance was set at P<0.05.
A total of 31001 participants were included, and Table 1 shows the demographic and clinical features of participants by tertiles of baseline WWI values. All variables were statistically significant among the three WWI subgroups. Compared with participants in the T1 group, participants in the T3 group were mostly female, older, non-Hispanic white, less educated, Divorced/widowed/separated, exhibited a higher prevalence of former smokers and previous drinkers, had lower PIR, creatinine, PA and HDL, had higher values of WC, Weight, ALT, AST, UA, TC, TG, and BMI. Besides, they had higher incidence rates of hypertension, CHD, Hyperlipidemia, T2DM, and stroke.
As indicated in Table 2, in the completely adjusted continuous model, with each 1-unit increase in WWI, the total odds of T2DM increased by 1.14-fold [2.14 [1.98,2.31], P<0.0001]. In the completely adjusted categorical model, compared to the lowest tertile of WWI (T1) serving as the reference group, T2 and T3 were associated with a 0.88-fold [1.88 [1.64,2.17], P<0.0001] and 2.63-fold [3.63 [3.11,4.23], P<0.0001] increase in the odds of developing T2DM, respectively. These findings underscore a significant positive correlation between WWI and the odds of T2DM. Furthermore, the results from the fitted curves indicated a non-linear trend (P non-linear=0.0017), as illustrated in Figure 2.
Figure 2 The association between WWI and the odds of T2DM. Age, sex, race/ethnicity, educational level, marital status, PIR, smoking status, alcohol user, Alt, Ast, UA, creatinine, TC, TG, HDL-C, physical activity,hypertension, stroke, coronary heart disease, and hyperlipidemia were adjusted.
The subgroup analysis was performed to assess the association between WWI and the odds of T2DM in different populations. As shown in Table 3, the relationship between WWI and the odds of T2DM was not markedly affected by age, gender, race/ethnicity, hypertension, CHD, stroke, and Hyperlipidemia(P<0.05). Significant interaction (p for interaction < 0.0001) was identified within subgroups based on age(<60、>=60). Among individuals younger than 60 years, each 1-unit increase in WWI was associated with a 1.79-fold elevated odds of T2DM. In contrast, for those aged 60 years or older, each 1-unit increase in WWI was linked with a 0.84-fold increased odds of T2DM. We also found a certain degree of interaction (p for interaction=0.01) in the hypertension (yes/no) subgroup. In hypertensive populations, each 1-unit increasement in WWI was correlated with a 1.06-fold increased odds of T2DM, and in non-hypertensive populations, each 1-unit increasement in WWI was related to a 1.20-fold increased odds of T2DM.
In this cross-sectional study involving 31,001 nationally representative participants, we found a strong positive correlation between WWI values and the odds of type 2 diabetes, implying that individuals with a higher WWI are more likely to have type 2 diabetes. Moreover, in the subgroup analyses, we found an interaction between age and hypertension in both subgroups.
A prospective cohort study from Northeast China (n=9205) observed a significant positive correlation between WWI values and the occurrence of type 2 diabetes and suggested that WWI could be a simple and effective predictor for the diagnosis of type 2 diabetes mellitus in a rural population in China (14). Sun H et al. (15) conducted a secondary analysis of a retrospective cohort study in a Japanese population (n=15,464) and found that WWI is a new metabolic index that can be used to predict T2DM in a Japanese population. WWI was found to be a novel metabolic index that can be used to predict T2DM occurrence in the Japanese population. Our findings were generally consistent with the results of these two studies. In addition, subgroup analyses revealed that WWI was more strongly associated with T2DM in people younger than 60 years of age (p for interaction< 0.0001), which may be related to changes in body composition such as significant increases in adiposity and decreases in muscle mass in older adults due to aging (20, 21), and other studies have suggested that it may also be related to differences in adiposity distribution between the younger and the older population (22, 22). differently in younger and older populations (22, 23). Our study also found higher odds of T2DM in the nonhypertensive population than in the hypertensive population.
In addition to being associated with T2DM, WWI is significantly associated with a variety of cardiovascular diseases and poor prognosis. A prospective cohort study from China included 10,338 nonhypertensive subjects with a mean follow-up of 6 years and found that high WWI was significantly linked to an increased risk of hypertension (24).In a cross-sectional study that included 21,040 subjects, Fang H et al. found that high levels of WWI were significantly associated with an elevated risk of development of CVD, especially prominent in those under 50 years of age, suggesting that WWI may be an interventional indicator for reducing the risk of cardiovascular disease in the general adult population (25). In a prospective cohort study (n=26822) with a mean follow-up of 69 months, elevated levels of WWI were found to be independently correlated with an increased risk of cardiovascular mortality and all-cause mortality (26). Zhang D et al. also reported that WWI was associated with heart failure, suggesting that WWI may be a significant marker of heart failure predictability (27). Ye J et al. found in a cross-sectional study of 23,389 cases that larger WWI might be an independently predictive factor for stroke (28). In addition, Cai S et al. have reported that WWI is associated with left heart ventricular fertilization, suggesting that WWI may be an important predictor of cardiometabolic risk (29).
BMI and WC, the traditional measures of obesity, are strongly associated with the development of diabetes mellitus. J M Chan et al. conducted a cross-sectional study that included 51,529 men aged 40-75 years and found that those with a BMI greater than 35 kg/m2 had a substantially increased risk of type 2 diabetes mellitus compared to those with a BMI less than 23 kg/m2 (30). A cohort study from Colombia that included 6,580 subjects with a mean follow-up of 12 years resulted in the definition of waist circumference thresholds of 89 cm in men and 86 cm in women, which were used to identify an elevated risk of developing diabetes (31). A retrospective cohort study from Japan, which included 4754 subjects, found that both BMI and WC were positively associated with the risk of developing diabetes (32). The obesity paradox is still present despite the growing evidence that these traditional obesity markers are correlated with type 2 diabetes mellitus (33). The reason for the controversy may partly stem from the inability of traditional indices to differentiate between fat distribution and muscle mass (34, 35).WWI can accurately reveal centripetal obesity independent of body weight (13). Previous studies have shown that high WWI values are associated with unfavorable body composition outcomes such as high-fat content, low muscle mass, and low bone mass (36). Thus, WWI may serve as a more comprehensible and precise measurement of obesity, reflecting the correlation between obesity and type 2 diabetes mellitus. In recent studies, WWI has been identified as a robust predictor of multiple diseases, superior to BMI and WC (12, 18, 37). WWI is more consistent and reliable in predicting the occurrence of diseases in different races and populations, especially in cross-racial or multicenter studies (37). Regarding other novel indicators such as waist-to-hip ratio (WHR) and waist-to-height ratio (WHtR), which have been used in diabetes research, there is a lack of comparative studies on the comparison with WWI, which may be one of the directions for future studies evaluating obesity and diabetes.
Several underlying mechanisms could account for this positivity in the relationship between WWI and T2DM. Firstly, the increase in WWI may reflect adipose tissue dysfunction, especially the accumulation of visceral adipose tissue, and the large accumulation of visceral fat leads to the release of large quantities of pro-inflammatory factors, including leptin and aldosterone, which lead to insulin resistance and inflammatory responses occurring that can lead to diabetes mellitus (38). Second, adipocytes in centripetally obese individuals are characterized by a hyperlipolytic state that is resistant to the antilipolytic effects of insulin, and the resulting flow of nonesterified fatty acids to the liver not only impairs hepatic metabolism but also leads to an increase in hepatic gluconeogenesis (39). Finally, adipose tissue in obese individuals releases more reactive oxygen species (ROS), and excessive ROS not only reduces the biological availability of nitric oxide (NO) but also hyperoxides tend to react with NO to generate deleterious hydrogen peroxide, which ultimately leads to the development of endothelial cell dysfunction (40, 41). Previous studies have shown that the development of diabetes mellitus is closely related to endothelial dysfunction (42–44).
The advantage of this study is that it is based on NHANES data which were gathered using a graded multi-stage probability sampling strategy, thus making the study more trustworthy and representational. However, our study has several limitations. First, since the current study is a cross-sectional study, causal conclusions cannot be drawn from our results. Second, although we adjusted for various confounders in our model, we could not exclude the effects of other unmeasured potential confounders, such as dietary intake and environmental exposures. Finally, the results may not be extrapolated to other countries because of differences between countries.
We report that WWI is strongly associated with the odds of T2DM in U.S. adults and found higher odds of T2DM among young adults and non-hypertensive populations. Future studies should focus on the role of different obesity indices in the development of diabetes.
The raw data supporting the conclusions of this article will be made available by the authors, without undue reservation.
The studies involving humans were approved by National Health and Nutrition Examination Survey center in the United States. The studies were conducted in accordance with the local legislation and institutional requirements. The participants provided their written informed consent to participate in this study.
DZ: Writing – original draft. SZ: Methodology, Conceptualization, Formal analysis, Validation, Visualization, Writing – review & editing. DL: Data curation, Funding acquisition, Supervision, Writing – review & editing. FL: Supervision, Project administration, Validation, Writing – review & editing. ZR: Methodology, Writing – review & editing. XD: Data curation, Funding acquisition, Writing – review & editing. WC: Funding acquisition, Supervision, Writing – review & editing.
The author(s) declare financial support was received for the research, authorship, and/or publication of this article. This study was supported by the Shandong Natural Science Foundation Youth Program (ZR2020QH339) and Natural Science Foundation of Shandong Province (No.ZR2021LZY040).
Thanks to Zhang Jing (Second Department of Infectious Disease, Shanghai Fifth People’s Hospital, Fudan University) for his work on the NHANES database. His outstanding work, the nhanesR package, and the webpage make it easier for us to explore the NHANES database.
The authors declare that the research was conducted in the absence of any commercial or financial relationships that could be construed as a potential conflict of interest.
All claims expressed in this article are solely those of the authors and do not necessarily represent those of their affiliated organizations, or those of the publisher, the editors and the reviewers. Any product that may be evaluated in this article, or claim that may be made by its manufacturer, is not guaranteed or endorsed by the publisher.
1. Demir S, Nawroth PP, Herzig S, Ekim Üstünel B. Emerging targets in type 2 diabetes and diabetic complications. Adv Sci (Weinh) (2021) 8:e2100275. doi: 10.1002/advs.202100275
2. Saeedi P, Petersohn I, Salpea P, Malanda B, Karuranga S, Unwin N, et al. Global and regional diabetes prevalence estimates for 2019 and projections for 2030 and 2045: Results from the International Diabetes Federation Diabetes Atlas, 9(th) edition. Diabetes Res Clin Pract (2019) 157:107843. doi: 10.1016/j.diabres.2019.107843
3. NCD Risk Factor Collaboration (NCD-RisC). Trends in adult body-mass index in 200 countries from 1975 to 2014: a pooled analysis of 1698 population-based measurement studies with 19·2 million participants. Lancet (2016) 387:1377–96. doi: 10.1016/S0140-6736(16)30054-X
4. NCD Risk Factor Collaboration (NCD-RisC). Worldwide trends in body-mass index, underweight, overweight, and obesity from 1975 to 2016: a pooled analysis of 2416 population-based measurement studies in 128·9 million children, adolescents, and adults. Lancet (2017) 390:2627–42. doi: 10.1016/S0140-6736(17)32129-3
5. Yanovski JA. Obesity: Trends in underweight and obesity - scale of the problem. Nat Rev Endocrinol (2018) 14:5–6. doi: 10.1038/nrendo.2017.157
6. Maggio CA, Pi-Sunyer FX. Obesity and type 2 diabetes. Endocrinol Metab Clin North Am (2003) 32:805–22. doi: 10.1016/s0889-8529(03)00071-9
7. Lavie CJ, De Schutter A, Patel D, Artham SM, Milani RV. Body composition and coronary heart disease mortality–an obesity or a lean paradox? Mayo Clin Proc (2011) 86:857–64. doi: 10.4065/mcp.2011.0092
8. Ford ES, Maynard LM, Li C. Trends in mean waist circumference and abdominal obesity among US adults, 1999-2012. JAMA (2014) 312:1151–3. doi: 10.1001/jama.2014.8362
10. Fox CS, Massaro JM, Hoffmann U, Pou KM, Maurovich-Horvat P, Liu C-Y, et al. Abdominal visceral and subcutaneous adipose tissue compartments: association with metabolic risk factors in the Framingham Heart Study. Circulation (2007) 116:39–48. doi: 10.1161/CIRCULATIONAHA.106.675355
11. Boden G, Shulman GI. Free fatty acids in obesity and type 2 diabetes: defining their role in the development of insulin resistance and beta-cell dysfunction. Eur J Clin Invest (2002) 32 Suppl 3:14–23. doi: 10.1046/j.1365-2362.32.s3.3.x
12. Park Y, Kim NH, Kwon TY, Kim SG. A novel adiposity index as an integrated predictor of cardiometabolic disease morbidity and mortality. Sci Rep (2018) 8:16753. doi: 10.1038/s41598-018-35073-4
13. Kim NH, Park Y, Kim NH, Kim SG. Weight-adjusted waist index reflects fat and muscle mass in the opposite direction in older adults. Age Ageing (2021) 50:780–6. doi: 10.1093/ageing/afaa208
14. Yu S, Wang B, Guo X, Li G, Yang H, Sun Y. Weight-adjusted-waist index predicts newly diagnosed diabetes in chinese rural adults. J Clin Med (2023) 12. doi: 10.3390/jcm12041620
15. Sun H, Li Y, Shi J, Li K, Zhao Y, Shang L, Tang B. Weight-adjusted waist index is not superior to conventional anthropometric indices for predicting type 2 diabetes: a secondary analysis of a retrospective cohort study. Fam Pract (2023) 40:782–8. doi: 10.1093/fampra/cmad047
16. von Elm E, Altman DG, Egger M, Pocock SJ, Gøtzsche PC, Vandenbroucke JP. The Strengthening the Reporting of Observational Studies in Epidemiology (STROBE) statement: guidelines for reporting observational studies. Lancet (2007) 370:1453–7. doi: 10.1016/S0140-6736(07)61602-X
17. American Diabetes Association. Standards of medical care in diabetes--2013. Diabetes Care (2013) 36(Suppl 1):S11–66. doi: 10.2337/dc13-S011
18. Xie F, Xiao Y, Li X, Wu Y. Association between the weight-adjusted-waist index and abdominal aortic calcification in United States adults: Results from the national health and nutrition examination survey 2013-2014. Front Cardiovasc Med (2022) 9:948194. doi: 10.3389/fcvm.2022.948194
19. Tao J, Zhang Y, Tan C, Tan W. Associations between weight-adjusted waist index and fractures: a population-based study. J Orthop Surg Res (2023) 18:290. doi: 10.1186/s13018-023-03776-8
20. Enzi G, Gasparo M, Biondetti PR, Fiore D, Semisa M, Zurlo F. Subcutaneous and visceral fat distribution according to sex, age, and overweight, evaluated by computed tomography. Am J Clin Nutr (1986) 44:739–46. doi: 10.1093/ajcn/44.6.739
21. Roubenoff R. Sarcopenic obesity: the confluence of two epidemics. Obes Res (2004) 12:887–8. doi: 10.1038/oby.2004.107
22. Szulc P, Duboeuf F, Chapurlat R. Age-related changes in fat mass and distribution in men-the cross-sectional STRAMBO study. J Clin Densitom (2017) 20:472–9. doi: 10.1016/j.jocd.2016.08.003
23. Oda E, Kawai R. Age- and gender-related differences in correlations between abdominal obesity and obesity-related metabolic risk factors in Japanese. Intern Med (2009) 48:497–502. doi: 10.2169/internalmedicine.48.1765
24. Li Q, Qie R, Qin P, Zhang D, Guo C, Zhou Q, et al. Association of weight-adjusted-waist index with incident hypertension: The Rural Chinese Cohort Study. Nutr Metab Cardiovasc Dis (2020) 30:1732–41. doi: 10.1016/j.numecd.2020.05.033
25. Fang H, Xie F, Li K, Li M, Wu Y. Association between weight-adjusted-waist index and risk of cardiovascular diseases in United States adults: a cross-sectional study. BMC Cardiovasc Disord (2023) 23:435. doi: 10.1186/s12872-023-03452-z
26. Han Y, Shi J, Gao P, Zhang L, Niu X, Fu N. The weight-adjusted-waist index predicts all-cause and cardiovascular mortality in general US adults. Clinics (Sao Paulo) (2023) 78:100248. doi: 10.1016/j.clinsp.2023.100248
27. Zhang D, Shi W, Ding Z, Park J, Wu S, Zhang J. Association between weight-adjusted-waist index and heart failure: Results from National Health and Nutrition Examination Survey 1999-2018. Front Cardiovasc Med (2022) 9:1069146. doi: 10.3389/fcvm.2022.1069146
28. Ye J, Hu Y, Chen X, Yin Z, Yuan X, Huang L, et al. Association between the weight-adjusted waist index and stroke: a cross-sectional study. BMC Public Health (2023) 23:1689. doi: 10.1186/s12889-023-16621-8
29. Cai S, Zhu T, Ding Y, Cheng B, Zhang A, Bao Q, et al. The relationship between the weight-adjusted-waist index and left ventricular hypertrophy in Chinese hypertension adults. Hypertens Res (2023) 46:253–60. doi: 10.1038/s41440-022-01075-z
30. Chan JM, Rimm EB, Colditz GA, Stampfer MJ, Willett WC. Obesity, fat distribution, and weight gain as risk factors for clinical diabetes in men. Diabetes Care (1994) 17:961–9. doi: 10.2337/diacare.17.9.961
31. Lopez-Lopez JP, Gonzalez AM, Lanza P, Martinez-Bello D, Gomez-Arbelaez D, Otero J, et al. Waist circumference cut-off points to identify major cardiovascular events and incident diabetes in Latin America: findings from the prospective Urban rural epidemiology study Colombia. Front Cardiovasc Med (2023) 10:1204885. doi: 10.3389/fcvm.2023.1204885
32. Abe M, Fujii H, Funakoshi S, Satoh A, Kawazoe M, Maeda T, et al. Comparison of body mass index and waist circumference in the prediction of diabetes: A retrospective longitudinal study. Diabetes Ther (2021) 12:2663–76. doi: 10.1007/s13300-021-01138-3
33. Han SJ, Boyko EJ. The evidence for an obesity paradox in type 2 diabetes mellitus. Diabetes Metab J (2018) 42:179–87. doi: 10.4093/dmj.2018.0055
34. Antonopoulos AS, Oikonomou EK, Antoniades C, Tousoulis D. From the BMI paradox to the obesity paradox: the obesity-mortality association in coronary heart disease. Obes Rev (2016) 17:989–1000. doi: 10.1111/obr.12440
35. Romero-Corral A, Somers VK, Sierra-Johnson J, Thomas RJ, Collazo-Clavell ML, Korinek J, et al. Accuracy of body mass index in diagnosing obesity in the adult general population. Int J Obes (Lond) (2008) 32:959–66. doi: 10.1038/ijo.2008.11
36. Kim KJ, Son S, Kim KJ, Kim SG, Kim NH. Weight-adjusted waist as an integrated index for fat, muscle and bone health in adults. J Cachexia Sarcopenia Muscle (2023) 14:2196–203. doi: 10.1002/jcsm.13302
37. Kim JY, Choi J, Vella CA, Criqui MH, Allison MA, Kim NH. Associations between weight-adjusted waist index and abdominal fat and muscle mass: multi-ethnic study of atherosclerosis. Diabetes Metab J (2022) 46:747–55. doi: 10.4093/dmj.2021.0294
38. Huby A-C, Antonova G, Groenendyk J, Gomez-Sanchez CE, Bollag WB, Filosa JA, et al. Adipocyte-derived hormone leptin is a direct regulator of aldosterone secretion, which promotes endothelial dysfunction and cardiac fibrosis. Circulation (2015) 132:2134–45. doi: 10.1161/CIRCULATIONAHA.115.018226
39. Després J-P, Lemieux I. Abdominal obesity and metabolic syndrome. Nature (2006) 444:881–7. doi: 10.1038/nature05488
40. Heitzer T, Schlinzig T, Krohn K, Meinertz T, Münzel T. Endothelial dysfunction, oxidative stress, and risk of cardiovascular events in patients with coronary artery disease. Circulation (2001) 104:2673–8. doi: 10.1161/hc4601.099485
41. Furukawa S, Fujita T, Shimabukuro M, Iwaki M, Yamada Y, Nakajima Y, et al. Increased oxidative stress in obesity and its impact on metabolic syndrome. J Clin Invest (2004) 114:1752–61. doi: 10.1172/JCI21625
42. Shah MS, Brownlee M. Molecular and cellular mechanisms of cardiovascular disorders in diabetes. Circ Res (2016) 118:1808–29. doi: 10.1161/CIRCRESAHA.116.306923
43. Yuan T, Yang T, Chen H, Fu D, Hu Y, Wang J, et al. New insights into oxidative stress and inflammation during diabetes mellitus-accelerated atherosclerosis. Redox Biol (2019) 20:247–60. doi: 10.1016/j.redox.2018.09.025
Keywords: weight-adjusted waist index, type 2 diabetes mellitus, NHANES, United States adults, cross-sectional study
Citation: Zheng D, Zhao S, Luo D, Lu F, Ruan Z, Dong X and Chen W (2024) Association between the weight-adjusted waist index and the odds of type 2 diabetes mellitus in United States adults: a cross-sectional study. Front. Endocrinol. 14:1325454. doi: 10.3389/fendo.2023.1325454
Received: 21 October 2023; Accepted: 19 December 2023;
Published: 16 January 2024.
Edited by:
Renato Branco, Purdue University Indianapolis, United StatesReviewed by:
Amani Alrasheedi, King Abdulaziz University, Saudi ArabiaCopyright © 2024 Zheng, Zhao, Luo, Lu, Ruan, Dong and Chen. This is an open-access article distributed under the terms of the Creative Commons Attribution License (CC BY). The use, distribution or reproduction in other forums is permitted, provided the original author(s) and the copyright owner(s) are credited and that the original publication in this journal is cited, in accordance with accepted academic practice. No use, distribution or reproduction is permitted which does not comply with these terms.
*Correspondence: Wenjing Chen, d2VuamluZzE5ODQxMkAxNjMuY29t ; Xiaokang Dong, bG92ZWR4a2NhdGh5QDE2My5jb20=
†These authors have contributed equally to this work and share first authorship
Disclaimer: All claims expressed in this article are solely those of the authors and do not necessarily represent those of their affiliated organizations, or those of the publisher, the editors and the reviewers. Any product that may be evaluated in this article or claim that may be made by its manufacturer is not guaranteed or endorsed by the publisher.
Research integrity at Frontiers
Learn more about the work of our research integrity team to safeguard the quality of each article we publish.