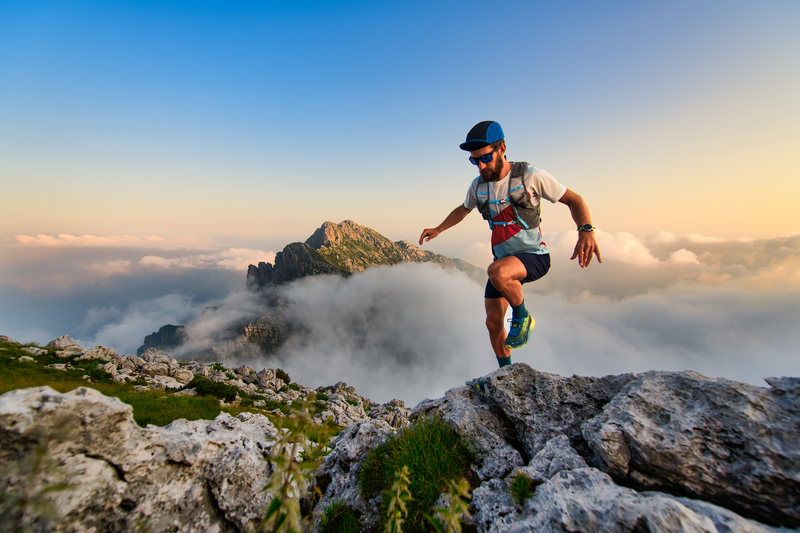
94% of researchers rate our articles as excellent or good
Learn more about the work of our research integrity team to safeguard the quality of each article we publish.
Find out more
ORIGINAL RESEARCH article
Front. Endocrinol. , 08 January 2024
Sec. Molecular and Structural Endocrinology
Volume 14 - 2023 | https://doi.org/10.3389/fendo.2023.1321576
This article is part of the Research Topic Pulmonary Fibrosis and Endocrine Factors View all 8 articles
Background: Previous observational studies have investigated the association between endocrine and metabolic factors and idiopathic pulmonary fibrosis (IPF), yet have produced inconsistent results. Therefore, it is imperative to employ the Mendelian randomization (MR) analysis method to conduct a more comprehensive investigation into the impact of endocrine and metabolic factors on IPF.
Methods: The instrumental variables (IVs) for 53 endocrine and metabolic factors were sourced from publicly accessible genome-wide association study (GWAS) databases, with GWAS summary statistics pertaining to IPF employed as the dependent variables. Causal inference analysis encompassed the utilization of three methods: inverse-variance weighted (IVW), weighted median (WM), and MR-Egger. Sensitivity analysis incorporated the implementation of MR-PRESSO and leave-one-out techniques to identify potential pleiotropy and outliers. The presence of horizontal pleiotropy and heterogeneity was evaluated through the MR-Egger intercept and Cochran’s Q statistic, respectively.
Results: The IVW method results reveal correlations between 11 traits and IPF. After correcting for multiple comparisons, seven traits remain statistically significant. These factors include: “Weight” (OR= 1.44; 95% CI: 1.16, 1.78; P=8.71×10-4), “Body mass index (BMI)” (OR= 1.35; 95% CI: 1.13, 1.62; P=1×10-3), “Whole body fat mass” (OR= 1.40; 95% CI: 1.14, 1.74; P=1.72×10-3), “Waist circumference (WC)” (OR= 1.54; 95% CI: 1.16, 2.05; P=3.08×10-3), “Trunk fat mass (TFM)” (OR=1.35; 95% CI: 1.10,1.65; P=3.45×10-3), “Body fat percentage (BFP)” (OR= 1.55; 95% CI: 1.15,2.08; P=3.86×10-3), “Apoliprotein B (ApoB)” (OR= 0.78; 95% CI: 0.65,0.93; P=5.47×10-3). Additionally, the sensitivity analysis results confirmed the reliability of the MR results.
Conclusion: The present study identified causal relationships between seven traits and IPF. Specifically, ApoB exhibited a negative impact on IPF, while the remaining six factors demonstrated a positive impact. These findings offer novel insights into the underlying etiopathological mechanisms associated with IPF.
Idiopathic pulmonary fibrosis (IPF) is a progressive lung disease featured by lung tissue scarring, with poor prognosis (1, 2). This condition is marked by the persistent development of scar tissue in the lungs, resulting in decreased lung flexibility, impaired oxygen exchange, and eventual respiratory failure and mortality (1, 3). Recent years have witnessed a global upsurge in the prevalence, hospitalization rate, and mortality associated with IPF, thus imposing a substantial burden on patients (4). However, due to the recognized unknown etiology, limited treatment options, and uncertain prognosis, it is imperative to conduct further investigations into the etiology and pathological mechanisms of IPF.
IPF is commonly believed to be influenced by the interplay of genetic and environmental factors, such as smoking, viral infections, occupational exposures to metal and wood chips, as well as agricultural activities (5). However, these risk factors fail to adequately account for the progressive nature of IPF. Numerous other factors may remain unidentified. An increasing number of studies have identified a higher prevalence of obesity, diabetes, cardiovascular disease in individuals with IPF (6–8). The occurrence of these three diseases is closely linked to abnormal endocrine and metabolic factors. However, previous studies on the effects of endocrine and metabolic factors on IPF have predominantly relied on observational research, yielding conflicting conclusions. The limitations of observational research, such as its inability to fully account for confounding factors, hinder its capacity to establish causal or reverse causality relationships. Regression models, which can only adjust for known confounders, are ineffective when confounders remain unidentified (9).
The Mendelian randomization (MR) method employs a valid instrumental variable (IV), including single nucleotide polymorphisms (SNPs), to simulate the random allocation of individual exposure and analyze the causal relationship between exposure and outcome (10). By randomly assigning the SNP allele during zygote formation prior to any exposure or outcome, it avoids associations with environmental confounders while minimizing the impact of reverse causality (9). Hence, the aim of this study is to employ MR to investigate the existence of a causal relationship between endocrine and metabolic factors and IPF.
The MR randomization method comprises three primary steps. The initial step involves evaluating whether the selected IV meets the three core assumptions. The second step encompasses conducting MR analysis to assess the causal effect between exposure and outcomes. Finally, the third step involves conducting sensitivity analysis to evaluate the reliability of the MR results.
To adhere to the fundamental principles of a two-sample MR design, data on exposure and outcome were collected from distinct European populations as described previously (11–13). The genome-wide association study (GWAS) datasets for 53 different exposures were extracted from sources such as the UK Biobank (UKBB) and the Genetic Investigation of ANthropometric Traits (GIANT), and can be accessed on the IEU OpenGWAS project website (https://gwas.mrcieu.ac.uk/). Furthermore, information on the IPF dataset was obtained from the study by Allen et al. (14). Supplementary Table 1 provides comprehensive details on the GWAS datasets utilized in this study.
The three fundamental assumptions are as follows: (1) IVs should be associated with the exposure; (2) IVs should not be associated with confounding factors; and (3) IVs should only be associated with the outcome through the exposure. To ensure adherence to these assumptions, specific implementation criteria were established. The inclusion criteria required a strong genetic association between the IVs and the exposure of interest, as indicated by a P-value < 5×10-8. Independent IVs with low levels of linkage disequilibrium (LD), characterized by an R2 value below 0.001, were identified using clumping methodology within a genomic window of 10 megabases. Following previous research findings, only IVs with minor allele frequencies (MAF) exceeding 0.01 were included in the analysis. Palindromic SNPs with ambiguous strand orientation were excluded from further analysis to ensure consistency in allele frequencies. F-statistics were calculated as measures of IV strength, with values greater than ten indicating minimal susceptibility to weak instrument bias (15).
The primary method employed in this study is the inverse variance-weighted (IVW) method. Following the approach outlined in (16), the regression line intercept was constrained to cross zero. To assess the possible presence of unbalanced horizontal pleiotropy, we employed the weighted median (WM) and MR-Egger methods. The WM method remains unbiased as long as no more than 50% of the weight comes from invalid instruments. Unlike the IVW method, MR-Egger does not assume that the regression line intersects at zero. A non-zero intercept in MR-Egger indicates the presence of unbalanced horizontal pleiotropy. The slope of the regression line provides an estimate of the effect as explained by (17).
To evaluate potential horizontal pleiotropy, we conducted an MR-Egger intercept test. In order to account for potential outliers, we incorporated pleiotropy-corrected data from MR-PRESSO. The Cochrane Q value was calculated to assess heterogeneity. To examine the influence of individual IVs on causal relationships and validate the reliability of the results, a leave-one-out sensitivity analysis was performed. In the MR analyses, causal effects were assessed using regression coefficients and odds ratios (ORs), along with their corresponding 95% confidence intervals (CIs), as the outcome variable was dichotomous. To address multiple comparisons, a false discovery rate (FDR) threshold of 5% was employed. All MR analyses were conducted using the TwoSampleMR package in R.
In this study, MR analysis was utilized to examine the associations between endocrine and metabolic factors and IPF. The IVs for the traits displayed F-statistics ranging from 28.62 to 3093.37. These F-statistics indicate strong instrument strength, as detailed in Supplementary Table 2.
The IVW approach in MR analysis revealed strong associations between genetically predicted traits and IPF. Specifically, the following factors showed significant links with IPF: “Weight” (OR= 1.44; 95% CI: 1.16, 1.78; P=8.71×10-4), “BMI” (OR= 1.35; 95% CI: 1.13, 1.62; P=1×10-3), “Whole body fat mass” (OR= 1.40; 95% CI: 1.14, 1.74; P=1.72×10-3), “Waist circumference (WC)” (OR= 1.54; 95% CI: 1.16, 2.05; P=3.08×10-3), “Trunk fat mass (TFM)” (OR=1.35; 95% CI: 1.10, 1.65; P=3.45×10-3), “Body fat percentage (BFP)” (OR= 1.55; 95% CI: 1.15, 2.08; P=3.86×10-3), “Apoliprotein B (ApoB)” (OR= 0.78; 95% CI: 0.65, 0.93; P=5.47×10-3). These findings, presented in Figures 1 and 2 as well as Supplementary Table 3, remained statistically significant even after adjusting for multiple comparisons. Figure 3 visually represents the causal relationships between the seven traits and IPF through a scatter plot.
Figure 1 The P-value distribution of associations between endocrine and metabolic factors and idiopathic pulmonary fibrosis in the Mendelian randomization analysis. The significance threshold adjusted by False Discovery Rate is illustrated by the red dashed line, while the suggestive significance threshold, established at P = 0.05, is depicted by the blue dash-dotted line.
Figure 2 Associations between genetically predicted 7 traits and idiopathic pulmonary fibrosis examined by three MR methods. MR, Mendelian randomization; IVW, inverse-variance weighted; WM, weighted median; OR, odds ratio; CI, confidence interval.
Figure 3 Scatter plot showing the causal effects of 7 traits on idiopathic pulmonary fibrosis. SNP, single nucleotide polymorphism.
The potential heterogeneity was tested (see Figure 4 and Supplementary Table 4). The examination of the intercept term using the MR-Egger method did not reveal significant evidence of horizontal pleiotropy (see Supplementary Table 5). The findings from the MR-PRESSO analysis are consistent with the results mentioned earlier. It is worth noting that although MR-PRESSO identified outlier IVs, the results were not significantly altered (see Supplementary Table 6). As shown in Supplementary Figure 1, the leave-one-out analysis demonstrates that none of the individual SNPs were solely responsible for the observed outcomes. This sensitivity analysis method supports the reliability of our MR results.
Figure 4 Funnel plot indicating the causal associations of 7 traits on idiopathic pulmonary fibrosis. SNP, single nucleotide polymorphism; IV, instrumental variable; SE, standard error.
In summary, our findings indicate a positive causal association between six traits (weight, BMI, whole body fat mass, WC, TFM, and BFP) and IPF. Additionally, a negative causal relationship between ApoB and IPF was also observed.
In this study, we conducted a two-sample Mendelian randomization analysis using summary statistics from the GWAS database. After conducting sensitivity analysis and correcting for multiple comparisons, we identified causal relationships between seven traits and IPF. Based on the various measurement methods employed, these seven traits will be discussed in the following three groups.
The present study identified a negative causal relationship between ApoB and IPF. Previous studies on ApoB and lung fibrosis are limited, with most focusing on fibrosis in other organs such as the heart, liver, and kidney.
Cardiac fibrosis primarily refers to fibrotic changes in the blood vessels of the heart, contributing to the widespread occurrence of coronary atherosclerosis. This condition predominantly arises due to lipid deposition within the arterial intima, leading to the development of arterial intima fibrosis, subsequent formation of atheromatous plaques, and consequent obstruction of the coronary artery lumen. Low-density lipoprotein (LDL) cholesterol has long been recognized as the important protein implicated in the development of atherosclerosis (18). Nonetheless, emerging evidence from a recent study indicates that ApoB surpasses LDL cholesterol as a more effective predictor of cardiovascular risk (19). A cohort study involving 4232 participants revealed an association between elevated ApoB levels and the progression of atherosclerotic lesions (20). Furthermore, case-control studies comprising 57973 individuals demonstrated an association between ApoB truncation and a decreased incidence of coronary heart disease (21). ApoB serves as the primary apolipoprotein for triglyceride-rich lipoproteins [including milk fat particles and very low-density lipoprotein (VLDL)], LDL, medium-density lipoprotein, total cholesterol, and triglycerides (22). Apolipoprotein B exists primarily in two forms: Apolipoprotein-B100 (ApoB-100) and Apolipoprotein-B48 (ApoB-48) (23). ApoB-100 is the main protein responsible for transporting cholesterol (including VLDL and LDL) to tissues. Prior research has established the significant involvement of ApoB, specifically ApoB-100, in the advancement of atherosclerosis through its facilitation of atherogenic lipoprotein assembly (23). Moreover, ApoB-100 has been employed as a prognostic marker for steatosis, whereby heightened levels exhibit a substantial correlation with high-grade hepatic steatosis (24). The p210 vaccine, derived from the ApoB-100-related 20 amino acid peptide antigen, exhibited renal protection and decreased inflammation and fibrosis in CD8+ T cell-mediated apoE mice (25).
A previous study found that a decrease in ApoB in lung cancer leads to an increase in oxidative stress (26), and an increase in ApoB may presumably be related to a reduction in oxidative stress. IPF is a progressive, end-stage, age-related lung fibrosis disease, and its etiology is still unclear. Research has shown that mitochondrial ROS from epithelial cells, fibroblasts, and alveolar macrophages are involved in the development of IPF (27, 28). The endoplasmic reticulum (ER) is also a crucial contributor of ROS. Abnormal activation of ER stress response has been linked to the pathogenesis of IPF (29). Thus, an increase in ROS (which disrupts redox signaling and/or causes molecular damage) in mitochondria or ER contributes to the development of IPF (30). This study found that ApoB can decrease the risk of IPF, and it is hypothesized that the altered oxidative stress levels may play an important role in this process.
Weight, BMI, and WC are commonly used anthropometric measures in clinical research (31–35). IPF consistently correlates with nutritional status, and anthropometry serves as an initial step in its evaluation (36). In our study, we established a positive causal relationship between weight, BMI, WC, and IPF. It is important to note that this relationship was specifically observed during the onset stage of the disease. However, in later stages of IPF, higher BMI and weight gain have been found to potentially mitigate adverse outcomes associated with the condition (37–40). Nevertheless, our results find support from another MR study, which demonstrates that each standard deviation increase in BMI is associated with a 40-70% higher risk of IPF (41). Another MR study reveals positive associations between BMI and WC with IPF risk. However, after correction for multiple comparisons, WC loses significance while BMI remains significant (5).
Limited studies have been conducted to investigate the mechanism by which anthropometric measurements impact IPF. IPF primarily focuses on the sensitivity of type II alveolar epithelial cells (AECII) to ER stress response (42–44). Previous studies have shown that ER stress leads to a decrease in PINK1 expression in AECIIs (45). This decrease in PINK1 expression results in enlarged mitochondria, decreased cellular viability, and activation of pro-fibrotic responses (45). Additionally, mitochondrial substances can induce the actions of cytokines in the lungs that possess proliferative and pro-fibrotic characteristics (45). WC is a simple measurement method that effectively determines abdominal fat distribution (46). Previous studies have found a correlation between increased WC, indicative of abdominal obesity, and reduced mitochondrial metabolism in muscles (47). In addition, significantly increased numbers of malformed and functionally impaired mitochondria have been observed in AECIIs in the lungs of IPF patients (45). Interestingly, our study revealed a positive causal relationship between WC and IPF. Therefore, we hypothesize that decreased mitochondrial metabolism in AECIIs may interplay with the accumulation of abdominal obesity, leading to an increase in IPF.
This study uncovered a significant positive causal relationship between whole body fat mass, TFM, BFP, and IPF. Whole body fat mass, TFM, and BFP are widely recognized as reliable indicators of body composition. Assessing body composition ranks as the second most crucial factor in evaluating the physical nutritional status of IPF patients (36). However, there is a dearth of research on the link between body composition and IPF, with one study reporting that each standard deviation increase in BFP level raises the risk of IPF by approximately 40-70% (41), which aligns with our findings. The underlying mechanism by which whole body fat mass, TFM, and BFP contribute to IPF remains unknown and requires further investigation in future studies.
Our study possesses numerous strengths. Firstly, MR studies offer various advantages compared to observational studies. By utilizing genetic variation as an IV, this study effectively mitigates the influence of confounding factors and reverse causality. Secondly, we conducted a novel investigation into the causal relationship between multiple endocrine and metabolic factors and IPF. Additionally, we performed several sensitivity analyses to reinforce the reliability of our findings.
Our study is subject to several limitations. Firstly, our study was conducted exclusively on populations of European ancestry, which limits the generalizability of our findings to other populations. Therefore, caution should be exercised when extrapolating the results to populations of different ancestries. Secondly, our study can only establish causality based on adherence to linear associations, and we cannot explicitly confirm that the relationship between these endocrine and metabolic factors and IPF follows strict linear causality. For example, a cohort study conducted in the UK identified a J-shaped association between BMI and respiratory disease-related mortality (48). Lastly, while efforts were made to minimize selective bias, its complete elimination may not have been achieved. Additionally, due to the absence of certain individual baseline data in the GWAS, we were unable to stratify the analyses by covariates of interest, such as age and gender.
This study found causal associations between seven traits and IPF. Specifically, ApoB had a negative impact on IPF, whereas the other six factors had positive effects. These findings provide novel insights into the underlying etiopathological mechanism of IPF. However, the specific mechanism by which these factors affect IPF remains unknown and warrants further investigation.
The original contributions presented in the study are included in the article/Supplementary Material. Further inquiries can be directed to the corresponding authors.
The GWAS included in this work were approved by their relevant review board, and informed consent were given by all participants.
YJ: Writing – original draft. RC: Writing – original draft. SX: Writing – original draft. YD: Writing – original draft. MZ: Writing – original draft. MB: Writing – review & editing. BH: Writing – review & editing. SL: Writing – review & editing.
The author(s) declare financial support was received for the research, authorship, and/or publication of this article. This study was supported by the BUCM Precision Cultivation Program (Grant No. JZPY-202205), the BUCM Research Development Fund (Grant No. 2021-ZXFZJJ-052), the Scientific Research Project of Hunan Provincial Department of Education (Grant No. 22C0676), and the Hunan Provincial Natural Science Foundation of China (Grant No. 2021JJ40643).
The authors declare that the research was conducted in the absence of any commercial or financial relationships that could be construed as a potential conflict of interest.
All claims expressed in this article are solely those of the authors and do not necessarily represent those of their affiliated organizations, or those of the publisher, the editors and the reviewers. Any product that may be evaluated in this article, or claim that may be made by its manufacturer, is not guaranteed or endorsed by the publisher.
The Supplementary Material for this article can be found online at: https://www.frontiersin.org/articles/10.3389/fendo.2023.1321576/full#supplementary-material
Supplementary Table 1 | Information of GWAS summary datasets used in MR analyses. GWAS, genome-wide association study; ID, Identification; IPF, Idiopathic Pulmonary Fibrosis; MR, Mendelian randomization; ukb, UK Biobank; HDL, High-density lipoprotein; IGF-1, Insulin-like growth factor 1; LDL, Low-density lipoprotein; SHBG, Sex hormone-binding globulin.
Supplementary Table 2 | The F-statistics of IVs. IVs, instrumental variables; SNP, single nucleotide polymorphism.
Supplementary Table 3 | MR analysis results. MR, Mendelian randomization; SNP, single nucleotide polymorphism; OR, Odds ratio; CI, confidence interval; IVW, inverse-variance weighted; WM, Weighted median.
Supplementary Table 4 | Heterogeneity test results.
Supplementary Table 5 | The pleiotropy test results. SE, standard error.
Supplementary Table 6 | MR-PRESSO analyses between exposures and outcomes with outlier. MR, Mendelian randomization; SD, standard deviation.
IPF, Idiopathic pulmonary fibrosis; MR, Mendelian randomization; IVs, instrumental variables; GWAS, genome-wide association study; IVW, inverse-variance weighted; WM, weighted median; BMI, Body mass index; WC, Waist circumference; TFM, Trunk fat mass; BFP, Body fat percentage; ApoB, Apoliprotein B; SNP, single nucleotide polymorphism; UKBB, UK Biobank; LD, linkage disequilibrium; MAF, minor allele frequency; OR, odds ratio; CI, confidence interval; FDR, false discovery rate; VLDL, very low-density lipoprotein; LDL, low-density lipoprotein; ApoB-100, Apolipoprotein-B100; ApoB-48, Apolipoprotein-B48; TGF-β1, transforming growth factor-β1; AECII, type II alveolar epithelial cells; ER, endoplasmic reticulum; RCTs, randomized controlled trials.
1. Richeldi L, Collard HR, Jones MG. Idiopathic pulmonary fibrosis. Lancet (2017) 389(10082):1941–52. doi: 10.1016/S0140-6736(17)30866-8
2. Wang M, Xie Z, Xu J, Feng Z. TWEAK/Fn14 axis in respiratory diseases. Clin Chim Acta (2020) 509:139–48. doi: 10.1016/j.cca.2020.06.007
3. Spagnolo P, Kropski JA, Jones MG, Lee JS, Rossi G, Karampitsakos T, et al. Idiopathic pulmonary fibrosis: Disease mechanisms and drug development. Pharmacol Ther (2021) 222:107798. doi: 10.1016/j.pharmthera.2020.107798
4. Huang D, Gong L, Wu Z, Shi Y, Liang Z. Genetic association of circulating adipokines with risk of idiopathic pulmonary fibrosis: A two-sample Mendelian randomization study. Lung (2023) 201(4):355–62. doi: 10.1007/s00408-023-00640-8
5. Ma Y, Feng C, Tang H, Deng P, Li Y, Wang J, et al. Management of BMI is a potential new approach for the prevention of idiopathic pulmonary fibrosis. Front Genet (2022) 13:821029. doi: 10.3389/fgene.2022.821029
6. Glassberg MK, Nathan SD, Lin CY, Morgenthien EA, Stauffer JL, Chou W, et al. Cardiovascular risks, bleeding risks, and clinical events from 3 phase III trials of pirfenidone in patients with idiopathic pulmonary fibrosis. Adv Ther (2019) 36(10):2910–26. doi: 10.1007/s12325-019-01052-y
7. Lee AS, Mira-Avendano I, Ryu JH, Daniels C. The burden of idiopathic pulmonary fibrosis: an unmet public health need. Respir Med (2014) 108(7):955–67. doi: 10.1016/j.rmed.2014.03.015
8. Spagnolo P, Kreuter M, Maher TM, Wuyts W, Bonella F, Corte TJ, et al. Metformin does not affect clinically relevant outcomes in patients with idiopathic pulmonary fibrosis. Respiration (2018) 96(4):314–22. doi: 10.1159/000489668
9. Sekula P, Del Greco MF, Pattaro C, Köttgen A. Mendelian randomization as an approach to assess causality using observational data. J Am Soc Nephrol (2016) 27(11):3253–65. doi: 10.1681/ASN.2016010098
10. Burgess S, Butterworth A, Malarstig A, Thompson SG. Use of Mendelian randomisation to assess potential benefit of clinical intervention. Bmj (2012) 345:e7325. doi: 10.1136/bmj.e7325
11. Chen R, Xu S, Ding Y, Li L, Huang C, Bao M, et al. Dissecting causal associations of type 2 diabetes with 111 types of ocular conditions: a Mendelian randomization study. Front Endocrinol (2023) 14. doi: 10.3389/fendo.2023.1307468
12. Jiang Y, Chen R, Xu S, Ding Y, Zhang M, Bao M, et al. Assessing causal associations of hyperparathyroidism with blood counts and biochemical indicators: a Mendelian randomization study. Front Endocrinol (Lausanne) (2023) 14. doi: 10.3389/fendo.2023.1295040
13. Fu Q, Chen R, Ding Y, Xu S, Huang C, He B, et al. Sodium intake and the risk of various types of cardiovascular diseases: A Mendelian randomization study. Front Nutr (2023) 10. doi: 10.3389/fnut.2023.1250509
14. Allen RJ, Stockwell A, Oldham JM, Guillen-Guio B, Schwartz DA, Maher TM, et al. Genome-wide association study across five cohorts identifies five novel loci associated with idiopathic pulmonary fibrosis. Thorax (2022) 77(8):829–33. doi: 10.1136/thoraxjnl-2021-218577
15. Burgess S, Thompson SG. Avoiding bias from weak instruments in Mendelian randomization studies. Int J Epidemiol (2011) 40(3):755–64. doi: 10.1093/ije/dyr036
16. Hemani G, Bowden J, Davey Smith G. Evaluating the potential role of pleiotropy in Mendelian randomization studies. Hum Mol Genet (2018) 27(R2):R195–r208. doi: 10.1093/hmg/ddy163
17. Bowden J, Davey Smith G, Burgess S. Mendelian randomization with invalid instruments: effect estimation and bias detection through Egger regression. Int J Epidemiol (2015) 44(2):512–25. doi: 10.1093/ije/dyv080
18. Bao MH, Li JM, Zhou QL, Li GY, Zeng J, Zhao J, et al. Effects of miR−590 on oxLDL−induced endothelial cell apoptosis: Roles of p53 and NF−κB. Mol Med Rep (2016) 13(1):867–73. doi: 10.3892/mmr.2015.4606
19. Zhong X, Jiao H, Zhao D, Teng J. Association between serum apolipoprotein B and atrial fibrillation: a case-control study. Sci Rep (2022) 12(1):9597. doi: 10.1038/s41598-022-13773-2
20. Gigante B, Leander K, Vikstrom M, Frumento P, Carlsson AC, Bottai M, et al. Elevated ApoB serum levels strongly predict early cardiovascular events. Heart (2012) 98(16):1242–5. doi: 10.1136/heartjnl-2012-301852
21. Welty FK. Hypobetalipoproteinemia and abetalipoproteinemia: liver disease and cardiovascular disease. Curr Opin Lipidol (2020) 31(2):49–55. doi: 10.1097/MOL.0000000000000663
22. Nass KJ, van den Berg EH, Faber KN, Schreuder T, Blokzijl H, Dullaart RPF. High prevalence of apolipoprotein B dyslipoproteinemias in non-alcoholic fatty liver disease: The lifelines cohort study. Metabolism (2017) 72:37–46. doi: 10.1016/j.metabol.2017.04.004
23. Olofsson SO, Borèn J. Apolipoprotein B: a clinically important apolipoprotein which assembles atherogenic lipoproteins and promotes the development of atherosclerosis. J Intern Med (2005) 258(5):395–410. doi: 10.1111/j.1365-2796.2005.01556.x
24. Lin MS, Guo SE, Lin HS, Hsu JT, Lin YS, Lin TH, et al. Impact of apolipoprotein B on hepatosteatosis in a population infected with hepatitis C virus: A cross-sectional observational study. Obes Facts (2016) 9(2):101–11. doi: 10.1159/000443692
25. Chyu KY, Zhao X, Dimayuga PC, Zhou J, Li X, Yano J, et al. CD8+ T cells mediate the athero-protective effect of immunization with an ApoB-100 peptide. PloS One (2012) 7(2):e30780. doi: 10.1371/journal.pone.0030780
26. Zabłocka-Słowińska K, Płaczkowska S, Skórska K, Prescha A, Pawełczyk K, Porębska I, et al. Oxidative stress in lung cancer patients is associated with altered serum markers of lipid metabolism. PloS One (2019) 14(4):e0215246. doi: 10.1371/journal.pone.0215246
27. He C, Murthy S, McCormick ML, Spitz DR, Ryan AJ, Carter AB. Mitochondrial Cu,Zn-superoxide dismutase mediates pulmonary fibrosis by augmenting H2O2 generation. J Biol Chem (2011) 286(17):15597–607. doi: 10.1074/jbc.M110.187377
28. Zank DC, Bueno M, Mora AL, Rojas M. Idiopathic pulmonary fibrosis: aging, mitochondrial dysfunction, and cellular bioenergetics. Front Med (Lausanne) (2018) 5:10. doi: 10.3389/fmed.2018.00010
29. Tanjore H, Blackwell TS, Lawson WE. Emerging evidence for endoplasmic reticulum stress in the pathogenesis of idiopathic pulmonary fibrosis. Am J Physiol Lung Cell Mol Physiol (2012) 302(8), L721–9. doi: 10.1152/ajplung.00410.2011
30. Otoupalova E, Smith S, Cheng G, Thannickal VJ. Oxidative stress in pulmonary fibrosis. Compr Physiol (2020) 10(2):509–47. doi: 10.1002/cphy.c190017
31. Xiao D, Guo Y, Li X, Yin JY, Zheng W, Qiu XW, et al. The Impacts of SLC22A1 rs594709 and SLC47A1 rs2289669 Polymorphisms on Metformin Therapeutic Efficacy in Chinese Type 2 Diabetes Patients. Int J Endocrinol (2016) 2016:4350712. doi: 10.1155/2016/4350712
32. Chen Y, Chen L, Zhou Q. Genetic association between eNOS gene polymorphisms and risk of carotid atherosclerosis: A meta-analysis. Herz (2021) 46(Suppl 2):253–64. doi: 10.1007/s00059-020-04995-z
33. Xu Z, Zhang P, Chen Y, Jiang J, Zhou Z, Zhu H. Comparing SARC-CalF with SARC-F for screening sarcopenia in adults with type 2 diabetes mellitus. Front Nutr (2022) 9:803924. doi: 10.3389/fnut.2022.803924
34. Chai XN, Zhou BQ, Ning N, Pan T, Xu F, He SH, et al. Effects of lifestyle intervention on adults with metabolic associated fatty liver disease: A systematic review and meta-analysis. Front Endocrinol (Lausanne) (2023) 14:1081096. doi: 10.3389/fendo.2023.1081096
35. Amin F, Fatima SS, Islam N, Gilani AH. Prevalence of obesity and overweight, its clinical markers and associated factors in a high risk South-Asian population. BMC Obes (2015) 2:16. doi: 10.1186/s40608-015-0044-6
36. Faverio P, Fumagalli A, Conti S, Madotto F, Bini F, Harari S, et al. Nutritional assessment in idiopathic pulmonary fibrosis: a prospective multicentre study. ERJ Open Res (2022) 8(1):00443-2021. doi: 10.1183/23120541.00443-2021
37. Nakatsuka Y, Handa T, Kokosi M, Tanizawa K, Puglisi S, Jacob J, et al. The clinical significance of body weight loss in idiopathic pulmonary fibrosis patients. Respiration (2018) 96(4):338–47. doi: 10.1159/000490355
38. Pugashetti J, Graham J, Boctor N, Mendez C, Foster E, Juarez M, et al. Weight loss as a predictor of mortality in patients with interstitial lung disease. Eur Respir J (2018) 52(3):1801289. doi: 10.1183/13993003.01289-2018
39. Kulkarni T, Yuan K, Tran-Nguyen TK, Kim YI, de Andrade JA, Luckhardt T, et al. Decrements of body mass index are associated with poor outcomes of idiopathic pulmonary fibrosis patients. PloS One (2019) 14(10):e0221905. doi: 10.1371/journal.pone.0221905
40. Jouneau S, Crestani B, Thibault R, Lederlin M, Vernhet L, Valenzuela C, et al. Analysis of body mass index, weight loss and progression of idiopathic pulmonary fibrosis. Respir Res (2020) 21(1):312. doi: 10.1186/s12931-020-01528-4
41. Wu W, Li C, Zhu X, Liu X, Li P, Wan R, et al. Genetic association of telomere length, obesity and tobacoo smoking with idiopathic pulmonary fibrosis risk. BMC Public Health (2023) 23(1):868. doi: 10.1186/s12889-023-15733-5
42. Korfei M, Ruppert C, Mahavadi P, Henneke I, Markart P, Koch M, et al. Epithelial endoplasmic reticulum stress and apoptosis in sporadic idiopathic pulmonary fibrosis. Am J Respir Crit Care Med (2008) 178(8):838–46. doi: 10.1164/rccm.200802-313OC
43. Lawson WE, Cheng DS, Degryse AL, Tanjore H, Polosukhin VV, Xu XC, et al. Endoplasmic reticulum stress enhances fibrotic remodeling in the lungs. Proc Natl Acad Sci U.S.A. (2011) 108(26):10562–7. doi: 10.1073/pnas.1107559108
44. Noble PW, Barkauskas CE, Jiang D. Pulmonary fibrosis: patterns and perpetrators. J Clin Invest (2012) 122(8):2756–62. doi: 10.1172/JCI60323
45. Bueno M, Lai YC, Romero Y, Brands J, St Croix CM, Kamga C, et al. PINK1 deficiency impairs mitochondrial homeostasis and promotes lung fibrosis. J Clin Invest (2015) 125(2):521–38. doi: 10.1172/JCI74942
46. Huxley R, Mendis S, Zheleznyakov E, Reddy S, Chan J. Body mass index, waist circumference and waist:hip ratio as predictors of cardiovascular risk–a review of the literature. Eur J Clin Nutr (2010) 64(1):16–22. doi: 10.1038/ejcn.2009.68
47. Bellissimo MP, Fleischer CC, Reiter DA, Goss AM, Zhou L, Smith MR, et al. Sex differences in the relationships between body composition, fat distribution, and mitochondrial energy metabolism: a pilot study. Nutr Metab (Lond) (2022) 19(1):37. doi: 10.1186/s12986-022-00670-8
Keywords: endocrine factors, metabolic factors, idiopathic pulmonary fibrosis, Mendelian randomization, UK Biobank
Citation: Jiang Y, Chen R, Xu S, Ding Y, Zhang M, Bao M, He B and Li S (2024) Endocrine and metabolic factors and the risk of idiopathic pulmonary fibrosis: a Mendelian randomization study. Front. Endocrinol. 14:1321576. doi: 10.3389/fendo.2023.1321576
Received: 14 October 2023; Accepted: 15 December 2023;
Published: 08 January 2024.
Edited by:
Pengxiu Cao, Hebei Normal University, ChinaReviewed by:
Ke Tan, Hebei Normal University, ChinaCopyright © 2024 Jiang, Chen, Xu, Ding, Zhang, Bao, He and Li. This is an open-access article distributed under the terms of the Creative Commons Attribution License (CC BY). The use, distribution or reproduction in other forums is permitted, provided the original author(s) and the copyright owner(s) are credited and that the original publication in this journal is cited, in accordance with accepted academic practice. No use, distribution or reproduction is permitted which does not comply with these terms.
*Correspondence: Meihua Bao, bWhiYW83OEAxNjMuY29t; Binsheng He, aGJzY3NtdUAxNjMuY29t; Sen Li, c2VubGlAY29ubmVjdC5oa3UuaGs=
†These authors have contributed equally to this work and share first authorship
Disclaimer: All claims expressed in this article are solely those of the authors and do not necessarily represent those of their affiliated organizations, or those of the publisher, the editors and the reviewers. Any product that may be evaluated in this article or claim that may be made by its manufacturer is not guaranteed or endorsed by the publisher.
Research integrity at Frontiers
Learn more about the work of our research integrity team to safeguard the quality of each article we publish.