- 1Department of General Practice, The Affiliated Hospital of Xuzhou Medical University, Xuzhou, Jiangsu, China
- 2Institute of Cardiovascular Disease Research, Xuzhou Medical University, Xuzhou, Jiangsu, China
- 3Department of Cardiology, The Affiliated Hospital of Xuzhou Medical University, Xuzhou, Jiangsu, China
- 4Department of Stomatology, The Affiliated Hospital of Xuzhou Medical University, Xuzhou, Jiangsu, China
- 5Department of Cardiology, Suining County Branch Hospital for the Affiliated Hospital of Xuzhou Medical University, Xuzhou, Jiangsu, China
Background: The triglyceride glucose-body mass index (TyG-BMI) is a surrogate indicator of insulin resistance. However, the association of TyG-BMI with heart failure (HF) in individuals with diabetes mellitus or prediabetes mellitus is unknown.
Methods: This study included 7,472 participants aged 20–80 years old with prediabetes or diabetes from the National Health and Nutrition Examination Survey (2007–2018). The TyG-BMI was calculated as Ln [triglyceride (mg/dL) × fasting blood glucose (mg/dL)/2] × BMI, and individuals were categorized into tertiles based on TyG-BMI levels. The relationship of TyG-BMI with HF was analyzed using multiple logistic regression models. Subgroup analyses were stratified by gender, age, hypertension, and diabetes mellitus status.
Results: This cross-sectional study had 7,472 participants (weighted n = 111,808,357), including 329 HF participants. Participants with a high TyG-BMI were prone to HF. The highest tertile group with a fully adjusted model was more likely to have HF compared to the lowest tertile group (odds ratio [OR], 2.645; 95% CI, 1.529–4.576). Restricted cubic spline analysis showed a significant dose-response relationship between TyG-BMI and HF (P < 0.001). In subgroup analyses, similar results were seen in terms of age (≥50 years old), gender, hypertension, and diabetes mellitus status.
Conclusion: A high TyG-BMI is significantly associated with HF risk in participants with diabetes mellitus or prediabetes mellitus.
Introduction
Heart failure (HF) is a systemic disease caused by multiple factors (1). The main features are damage to the structure and function of the heart and alterations in neurohormonal regulation (2). The mortality rate of hospitalized patients with HF is approximately 4% (3, 4), and the mortality rate of these patients within one month of discharge is 10% (5). One in five individuals older than 40 years will develop HF (6). This creates health hazards and adds pressure to the economy. Thus, early identification and intervention in individuals at high risk of developing HF are essential.
Many studies have shown that the incidence of HF is very high in the diabetes mellitus population (7), and diabetics with HF have a greater risk of death (8). Diabetic women have five times the risk of developing HF as non-diabetic women (9, 10). Prediabetes mellitus is the intermediate stage between normal blood glucose and diabetes mellitus, and it is characterized by impaired glucose metabolism (11). In a related study, prediabetes mellitus also increased the risk of HF, and prediabetic patients were more likely to develop ejection-preserved HF and have worse clinical prognosis (12). A high risk of HF in patients with diabetes mellitus or prediabetes mellitus may be related to insulin resistance (IR), but no study has examined the mechanism of action (13, 14).
The hyperinsulinemic euglycemia clamp technique is the gold standard for measuring IR (15). Because of economic cost and ethical issues, the application of the technique in clinical practice is difficult (15). Recently, the triglyceride glucose (TyG) index was found to be similarly sensitive and specific in predicting IR (16, 17), with triglyceride glucose-body mass index (TyG-BMI) predicting IR better than the TyG index. Therefore, our study explores the association of TyG-BMI with HF in patients with diabetes mellitus or prediabetes mellitus.
Materials and methods
Participants and study design
The participants were selected from the National Health and Nutrition Examination Survey (NHANES) from 2007–2018. The National Center for Health Statistics, the heart of the Centers for Disease Control and Prevention, comprises NHANES, which is a study investigating the nutritional and health status of adults and children in the United States. A total of 12,745 individuals with fasting blood glucose (FBG) and hemoglobin A1c (HbA1c) data, as well as complete history of diabetes mellitus from 2007–2018 were included in the study. We excluded 5,273 participants for the following reasons: (1) participants without HF questionnaire data; (2) participants without BMI, TG, and/or FBG data; and (3) participants without other covariates. Figure 1 shows the flow chart of the systematic selection process.
Diabetes mellitus and prediabetes mellitus were defined as follows: for diabetes mellitus, patients were informed by a doctor to have diabetes mellitus, or patients had FBG ≥126 mg/dl or HbA1c ≥6.5%. For prediabetes mellitus, patients had FBG 100–125 mg/dl or HbA1c 5.7%–6.4% (18). Finally, 7,472 participants were selected for analysis. The public database provided the data for this study, and written informed consent was obtained from the participants by NHANES.
Assessment of HF
The NHANES questionnaire asks: “Has a doctor or other health professional ever told you that you have been diagnosed with HF?”. The participants who answered “yes” were considered to have HF.
Data collection and measurements
Demographic data included age, gender, and race. Race included non-Hispanic White, Mexican American, other Hispanic, non-Hispanic Black, and other races. Questionnaire data included drinking status, smoking status, disease status (hypertension, coronary artery disease, and stroke), and medication status (ACE inhibitors, beta blocker, statin, and diuretics). Smoking was defined as smoking more than one hundred cigarettes in the past year (19). Drinking consumption was defined as having had 12 glasses of wine in the past year (20). The history of diseases was given to patients by a doctor. Measured data included BMI, systolic blood pressure (SBP), and diastolic blood pressure (DBP). Laboratory data collection was performed using fasting blood samples, including FBG, creatinine, HbA1c, estimated glomerular filtration rate (eGFR), low-density lipoprotein cholesterol (LDL-C), triglyceride (TG), high-density lipoprotein cholesterol (HDL-C), and total cholesterol (TC).
Definition of index
The formulas for calculating the indexes were as follows.
TyG index = Ln [TG (mg/dL) × FBG (mg/dL)/2
BMI = weight (kg)/height (m)2
TyG-BMI = TyG index × BMI
Statistical analyses
Qualitative variables were expressed as numbers (n) and percentages (%), and continuous variables were expressed as mean ± standard deviation. Participants were categorized into tertiles based on TyG-BMI. The chi-square test or Kruskal–Wallis H-test was used for analysis. Assessment of the nonlinear association between TyG-BMI and HF used restricted cubic spline curves. We used multiple logistic regression models to explore the correlation between TyG-BMI and HF. Model 1 was non-adjusted; model 2 was adjusted for age, gender, race, smoking status, and drinking consumption; model 3 was adjusted for model 2, LDL-C, eGFR, hypertension, coronary artery disease, ACE inhibitors, Beta blocker, stain, and diuretics.
In subgroups analyses, we stratified the analysis by hypertension (yes or no), gender (male or female) and age (< 50 or ≥ 50 years old), and diabetes mellitus status (diabetes mellitus or prediabetes mellitus) and assessed interactions by likelihood ratio tests. All analyses were performed using R version 3.6.1 (https://www.r-project.org/). Statistical tests were significant at P < 0.05.
Results
Baseline characteristics
In our study, our final analysis included 7,472 NHANES participants (weighted n = 111,808,357). The baseline characteristics of the patients according to the TyG-BMI tertiles: tertile 1 (TyG-BMI <232.72), tertile 2 (232.72 ≤ TyG-BMI < 286.33), and tertile 3 (TyG-BMI ≥286.33) are shown in Table 1. This study included 1,547 diabetes mellitus patients and 5,925 prediabetes patients, of which 329 (3.6%) had HF. The mean age of all individuals was 54.69 ± 16.10 years, with 46.2% males and 53.8% females. Participants of the T3 group showed higher total cholesterol, BMI, FBG, SBP, DBP, HbA1c, and TG than participants of the T1 group.
The association of TyG-BMI with HF
As shown in Table 2, logistic regression models showed the association of TyG-BMI with HF. When TyG-BMI was analyzed as a continuous variable, patients were more likely to have HF (OR, 1.009; 95% CI, 1.006–1.012). T3-group patients had higher odds of developing HF than T1-group patients in the fully adjusted model (odds ratio [OR], 2.645; 95% CI, 1.529–4.576). When TyG-BMI was converted to a categorical variable, the P for trend was also significant in unadjusted (P for trend, <0.001) or adjusted (P for trend, <0.001) models. In Figure 2, using a multiple logistic regression model with cubic spline functions, we observed a dose-response relationship of TyG-BMI with HF (non-linear P < 0.05), and a higher TyG-BMI (>258.26) was associated with an increased HF risk.
Subgroup analyses
The results of the subgroup analyses are shown in Table 3 and Figure 3. For participants aged ≥50 years, TyG-BMI was significantly associated with a higher HF prevalence. Subgroup analyses of gender and hypertension showed significant associations between TyG-BMI and HF prevalence. Finally, a higher TyG-BMI associated with a greater HF prevalence in diabetes mellitus (OR, 1.008; 95% CI, 1.003–1.012) and prediabetes mellitus (OR, 1.009; 95% CI, 1.005–1.012) patients. No obvious interactions between TyG-BMI and HF in all subgroup analyses (P for interaction, >0.05) were noted.
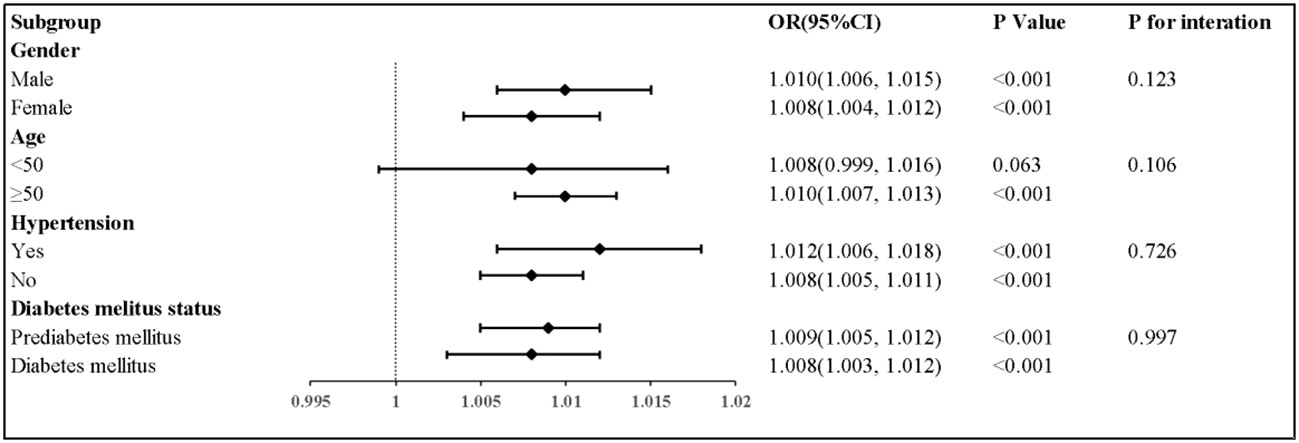
Figure 3 Subgroup analyses for the association between TyG-BMI with heart faliure. Adjusted for gender, age, race, smoking status, and drinking consumption, LDL-C, eGFR, hypertension, coronary artery disease, ACE inhibitors, Beta blocker, stain, and diuretics.
Discussion
This cross-sectional study explored the association of TyG-BMI with HF in diabetic and prediabetic populations from NHANES 2007–2018. Our study confirmed that diabetic or prediabetic participants with high TyG-BMI were more likely to have HF. When the index was used as a categorical or continuous variable, TyG-BMI was significantly associated with increased HF risk. Restricted cubic spline analysis indicated that high TyG-BMI had a dose-response relationship with HF development. The risk is higher when the index is greater than 258.26.
Previous studies have confirmed that the TyG index is a surrogate marker for IR (16, 21). BMI is the simplest measure to assess body fat and metabolism (22). TyG-BMI is the multiplication of the TyG index and BMI. Er et al. indicated that TyG-BMI was better than the TyG index, BMI, conventional lipid levels, visceral obesity index, lipid accumulation products, and adipokine and lipid ratios in predicting IR (23). Liu et al. reported a significantly increased incidence of atherosclerotic cardiovascular disease in Taiwanese adults with increasing TyG-BMI (24). In another study, a linear relationship of TyG-BMI with ischemic stroke in the general population was demonstrated (25). A cross-sectional study has indicated that individuals with a high TyG-BMI are more likely to have prehypertension (26), whereas a prospective study using data from Hong Kong and Taiwan found that individuals with a high TyG index were more likely to have HF (27). Xu et al. also reported that the TyG index was significantly associated with the prevalence of HF (28). The differences between our study and these two studies may be related to the fact that TyG-BMI predicted IR better than the TyG index, and previous cohorts were from the general population. Our study included diabetic and prediabetic patients.
In our subgroup analyses, fully-adjusted models showed a positive relationship between TyG-BMI and HF in individuals aged ≥50 years but not in those aged <50 years, which is probably due to the fact that fewer individuals aged <50 years developed HF than those aged ≥50 years in our study. Meanwhile, our study indicated that males with elevated TyG-BMI had a higher risk of developing HF than females. Although females are less likely to develop IR than males (29), HF due to high BMI and diabetes mellitus is more common in females (30), and diabetic females are more likely to develop coronary heart disease than males (31, 32). In summary, our findings are inconsistent with previous studies.
The TyG-BMI was associated with increased HF risk in diabetes mellitus status subgroup analysis, and the P for interaction was not significant, indicating TyG-BMI can be used to reflect HF risk of prediabetic and diabetic populations. In prediabetic and diabetic populations, HF occurrence was more likely to be induced by IR. Currently, the association between IR and HF can be explained by several mechanisms. Firstly, IR leads to cardiac pressure overload or ischemia susceptibility, which is possibly caused by impaired glucose utilization and subsequent increased free fatty acid oxidation (33, 34). Secondly, IR leads to adverse activation of the sympathetic nervous system, with chronic hyperinsulinemia increasing angiotensinogen release from adipose tissues and angiotensin II receptor expression, ultimately leading to aberrant cardiac remodeling and cardiac dysfunction (35). However, the mechanism of action between IR and HF requires further study.
The current study has several limitations. Firstly, our study is a cross-sectional observational study. Further prospective cohort studies, which include a control group, are likely to provide more real-world evidence in the future. Secondly, the diagnosis of HF may have been under- or over-reported by individuals in the questionnaire. Thirdly, the impact of the oral glucose tolerance test results on the diagnosis of prediabetes or diabetes was not considered.
Conclusions
In conclusion, a high TyG-BMI was significantly associated with an increased HF risk in patients with diabetes mellitus or prediabetes mellitus. Treatments aimed at reducing TyG-BMI for HF prevention are urgently required.
Data availability statement
The datasets presented in this study can be found in online repositories. The names of the repository/repositories and accession number(s) can be found below: https://www.cdc.gov/nchs/nhanes.
Ethics statement
The present study received approval from the National Center for Health Statistics and database obtained consent from the participants. The studies were conducted in accordance with the local legislation and institutional requirements. The participants provided their written informed consent to participate in this study.
Author contributions
SY: Data curation, Methodology, Writing – original draft. XS: Data curation, Methodology, Writing – original draft. WL: Data curation, Methodology, Writing – review & editing. ZW: Data curation, Methodology, Writing – review & editing. RL: Data curation, Writing – original draft. XX: Data curation, Writing – original draft. CW: Data curation, Writing – original draft. LL: Writing – review & editing. RW: Writing – review & editing. TX: Writing – review & editing.
Funding
The author(s) declare financial support was received for the research, authorship, and/or publication of this article. This study was supported by the Jiangsu Provincial Health Commission Project Fund (Grant number: M2020015), Jiangsu Provincial Science and Technology Department Social Development Fund (Grant number: BE2019639), Jiangsu Traditional Chinese Medicine Science and Technology Development Plan Project (Grant number: YB201988), Xuzhou Innovative plan to Promote Science Technology (Grant number: KC21192), and Xuzhou Practical Innovation Program for University Students (Grant number: SJCX23_1389).
Acknowledgments
We would like to thank the NHANES database for providing data for the study and express our gratitude to all members of this study, including the study sponsors, and all participants. We also thank International Science Editing (http://www.internationalscienceediting.com) for editing this manuscript.
Conflict of interest
The authors declare that the research was conducted in the absence of any commercial or financial relationships that could be construed as a potential conflict of interest.
Publisher’s note
All claims expressed in this article are solely those of the authors and do not necessarily represent those of their affiliated organizations, or those of the publisher, the editors and the reviewers. Any product that may be evaluated in this article, or claim that may be made by its manufacturer, is not guaranteed or endorsed by the publisher.
Abbreviations
HF, heart failure; TyG-BMI, triglyceride glucose-body mass index; NHANES, National Health and Nutritional Examination Survey; IR, insulin resistance; BMI, body mass index; SBP, systolic blood pressure; DBP, diastolic blood pressure; eGFR, estimated glomerular filtration rate; LDL-C, low-density lipoprotein cholesterol; HDL-C, high-density lipoprotein cholesterol; TC, total cholesterol; TG, triglyceride; FBG, fasting blood glucose; HbA1c, hemoglobin A1c.
References
1. Marwick TH, Ritchie R, Shaw JE, Kaye D. Implications of underlying mechanisms for the recognition and management of diabetic cardiomyopathy. J Am Coll Cardiol (2018) 71(3):339–51. doi: 10.1016/j.jacc.2017.11.019
2. Davison B, Cotter G. Why is heart failure so important in the 21st century? Eur J Heart Failure (2015) 17(2):122–4. doi: 10.1002/ejhf.219
3. Abraham WT, Fonarow GC, Albert NM, Stough WG, Gheorghiade M, Greenberg BH, et al. Predictors of in-hospital mortality in patients hospitalized for heart failure: insights from the Organized Program to Initiate Lifesaving Treatment in Hospitalized Patients with Heart Failure (OPTIMIZE-HF). J Am Coll Cardiol (2008) 52(5):347–56. doi: 10.1016/j.jacc.2008.04.028
4. Adams KF Jr., Fonarow GC, Emerman CL, LeJemtel TH, Costanzo MR, Abraham WT, et al. Characteristics and outcomes of patients hospitalized for heart failure in the United States: rationale, design, and preliminary observations from the first 100,000 cases in the Acute Decompensated Heart Failure National Registry (ADHERE). Am Heart J (2005) 149(2):209–16. doi: 10.1016/j.ahj.2004.08.005
5. Loehr LR, Rosamond WD, Chang PP, Folsom AR, Chambless LE. Heart failure incidence and survival (from the Atherosclerosis Risk in Communities study). Am J Cardiol (2008) 101(7):1016–22. doi: 10.1016/j.amjcard.2007.11.061
6. Lloyd-Jones DM, Larson MG, Leip EP, Beiser A, D’Agostino RB, Kannel WB, et al. Lifetime risk for developing congestive heart failure: the Framingham Heart Study. Circulation (2002) 106(24):3068–72. doi: 10.1161/01.cir.0000039105.49749.6f
7. Kenny HC, Abel ED. Heart failure in type 2 diabetes mellitus. Circ Res (2019) 124(1):121–41. doi: 10.1161/circresaha.118.311371
8. Echouffo-Tcheugui JB, Masoudi FA, Bao H, Spatz ES, Fonarow GC. Diabetes mellitus and outcomes of cardiac resynchronization with implantable cardioverter-defibrillator therapy in older patients with heart failure. Circ Arrhythmia Electrophysiol (2016) 9(8):e004132. doi: 10.1161/circep.116.004132
9. Kannel WB, Hjortland M, Castelli WP. Role of diabetes in congestive heart failure: the Framingham study. Am J Cardiol (1974) 34(1):29–34. doi: 10.1016/0002-9149(74)90089-7
10. Kannel WB, McGee DL. Diabetes and cardiovascular disease. Framingham Study Jama (1979) 241(19):2035–8. doi: 10.1001/jama.241.19.2035
11. Han Y, Hu H, Li Q, Deng Z, Liu D. Triglyceride glucose-body mass index and the risk of progression to diabetes from prediabetes: A 5-year cohort study in Chinese adults. Front Public Health (2023) 11:1028461. doi: 10.3389/fpubh.2023.1028461
12. Cai X, Liu X, Sun L, He Y, Zheng S, Zhang Y, et al. Prediabetes and the risk of heart failure: A meta-analysis. Diabetes Obes Metab (2021) 23(8):1746–53. doi: 10.1111/dom.14388
13. Park JJ. Epidemiology, pathophysiology, diagnosis and treatment of heart failure in diabetes. Diabetes Metab J (2021) 45(2):146–57. doi: 10.4093/dmj.2020.0282
14. Son TK, Toan NH, Thang N, Le Trong Tuong H, Tien HA, Thuy NH, et al. Prediabetes and insulin resistance in a population of patients with heart failure and reduced or preserved ejection fraction but without diabetes, overweight or hypertension. Cardiovasc Diabetol (2022) 21(1):75. doi: 10.1186/s12933-022-01509-5
15. DeFronzo RA, Tobin JD, Andres R. Glucose clamp technique: a method for quantifying insulin secretion and resistance. Am J Physiol (1979) 237(3):E214–23. doi: 10.1152/ajpendo.1979.237.3.E214
16. Guerrero-Romero F, Simental-Mendía LE, González-Ortiz M, Martínez-Abundis E, Ramos-Zavala MG, Hernández-González SO, et al. The product of triglycerides and glucose, a simple measure of insulin sensitivity. Comparison with the euglycemic-hyperinsulinemic clamp. J Clin Endocrinol Metab (2010) 95(7):3347–51. doi: 10.1210/jc.2010-0288
17. Lee EY, Yang HK, Lee J, Kang B, Yang Y, Lee SH, et al. Triglyceride glucose index, a marker of insulin resistance, is associated with coronary artery stenosis in asymptomatic subjects with type 2 diabetes. Lipids Health Dis (2016) 15(1):155. doi: 10.1186/s12944-016-0324-2
18. Casagrande SS, Lee C, Stoeckel LE, Menke A, Cowie CC. Cognitive function among older adults with diabetes and prediabetes, NHANES 2011-2014. Diabetes Res Clin Practice (2021) 178:108939. doi: 10.1016/j.diabres.2021.108939
19. Eke PI, Dye BA, Wei L, Slade GD, Thornton-Evans GO, Borgnakke WS, et al. Update on prevalence of periodontitis in adults in the United States: NHANES 2009 to 2012. J Periodontol (2015) 86(5):611–22. doi: 10.1902/jop.2015.140520
20. Lin MP, Ovbiagele B, Markovic D, Towfighi A. “Life’s simple 7” and long-term mortality after stroke. J Am Heart Assoc (2015) 4(11):e001470. doi: 10.1161/jaha.114.001470
21. Bastard JP, Lavoie ME, Messier V, Prud’homme D, Rabasa-Lhoret R. Evaluation of two new surrogate indices including parameters not using insulin to assess insulin sensitivity/resistance in non-diabetic postmenopausal women: a MONET group study. Diabetes Metab (2012) 38(3):258–63. doi: 10.1016/j.diabet.2012.01.004
22. Ding J, Chen X, Bao K, Yang J, Liu N, Huang W, et al. Assessing different anthropometric indices and their optimal cutoffs for prediction of type 2 diabetes and impaired fasting glucose in Asians: The Jinchang Cohort Study. J Diabetes (2020) 12(5):372–84. doi: 10.1111/1753-0407.13000
23. Er LK, Wu S, Chou HH, Hsu LA, Teng MS, Sun YC, et al. Triglyceride glucose-body mass index is a simple and clinically useful surrogate marker for insulin resistance in nondiabetic individuals. PloS One (2016) 11(3):e0149731. doi: 10.1371/journal.pone.0149731
24. Huang YC, Huang JC, Lin CI, Chien HH, Lin YY, Wang CL, et al. Comparison of innovative and traditional cardiometabolic indices in estimating atherosclerotic cardiovascular disease risk in adults. Diagnostics (Basel Switzerland) (2021) 11(4):603. doi: 10.3390/diagnostics11040603
25. Du Z, Xing L, Lin M, Sun Y. Estimate of prevalent ischemic stroke from triglyceride glucose-body mass index in the general population. BMC Cardiovasc Disord (2020) 20(1):483. doi: 10.1186/s12872-020-01768-8
26. Zeng ZY, Liu SX, Xu H, Xu X, Liu XZ, Zhao XX. Association of triglyceride glucose index and its combination of obesity indices with prehypertension in lean individuals: A cross-sectional study of Chinese adults. J Clin Hypertension (Greenwich Conn) (2020) 22(6):1025–32. doi: 10.1111/jch.13878
27. Li X, Chan JSK, Guan B, Peng S, Wu X, Lu X, et al. Triglyceride-glucose index and the risk of heart failure: Evidence from two large cohorts and a mendelian randomization analysis. Cardiovasc Diabetol (2022) 21(1):229. doi: 10.1186/s12933-022-01658-7
28. Xu L, Wu M, Chen S, Yang Y, Wang Y, Wu S, et al. Triglyceride-glucose index associates with incident heart failure: A cohort study. Diabetes Metab (2022) 48(6):101365. doi: 10.1016/j.diabet.2022.101365
29. Tramunt B, Smati S, Grandgeorge N, Lenfant F, Arnal JF, Montagner A, et al. Sex differences in metabolic regulation and diabetes susceptibility. Diabetologia (2020) 63(3):453–61. doi: 10.1007/s00125-019-05040-3
30. Gerdts E, Regitz-Zagrosek V. Sex differences in cardiometabolic disorders. Nat Med (2019) 25(11):1657–66. doi: 10.1038/s41591-019-0643-8
31. Tian X, Zuo Y, Chen S, Liu Q, Tao B, Wu S, et al. Triglyceride-glucose index is associated with the risk of myocardial infarction: an 11-year prospective study in the Kailuan cohort. Cardiovasc Diabetol (2021) 20(1):19. doi: 10.1186/s12933-020-01210-5
32. Peters SA, Huxley RR, Woodward M. Diabetes as risk factor for incident coronary heart disease in women compared with men: a systematic review and meta-analysis of 64 cohorts including 858,507 individuals and 28,203 coronary events. Diabetologia (2014) 57(8):1542–51. doi: 10.1007/s00125-014-3260-6
33. Kolwicz SC Jr., Purohit S, Tian R. Cardiac metabolism and its interactions with contraction, growth, and survival of cardiomyocytes. Circ Res (2013) 113(5):603–16. doi: 10.1161/circresaha.113.302095
34. Yazıcı D, Sezer H. Insulin resistance, obesity and lipotoxicity. Adv Exp Med Biol (2017) 960:277–304. doi: 10.1007/978-3-319-48382-5_12
Keywords: triglyceride glucose-body mass index, heart failure, diabetes mellitus, prediabetes mellitus, insulin resistance, NHANES
Citation: Yang S, Shi X, Liu W, Wang Z, Li R, Xu X, Wang C, Li L, Wang R and Xu T (2023) Association between triglyceride glucose-body mass index and heart failure in subjects with diabetes mellitus or prediabetes mellitus: a cross-sectional study. Front. Endocrinol. 14:1294909. doi: 10.3389/fendo.2023.1294909
Received: 15 September 2023; Accepted: 16 October 2023;
Published: 03 November 2023.
Edited by:
Lu Cai, University of Louisville, United StatesReviewed by:
Hong Zhu, Tianjin Medical University, ChinaHao Zheng, University of Louisville, United States
Copyright © 2023 Yang, Shi, Liu, Wang, Li, Xu, Wang, Li, Wang and Xu. This is an open-access article distributed under the terms of the Creative Commons Attribution License (CC BY). The use, distribution or reproduction in other forums is permitted, provided the original author(s) and the copyright owner(s) are credited and that the original publication in this journal is cited, in accordance with accepted academic practice. No use, distribution or reproduction is permitted which does not comply with these terms.
*Correspondence: Ruili Wang, eHpzbndybEAxNjMuY29t; Tongda Xu, eHV0b25nZGEzMDA0QDE2My5jb20=
†These authors share first authorship