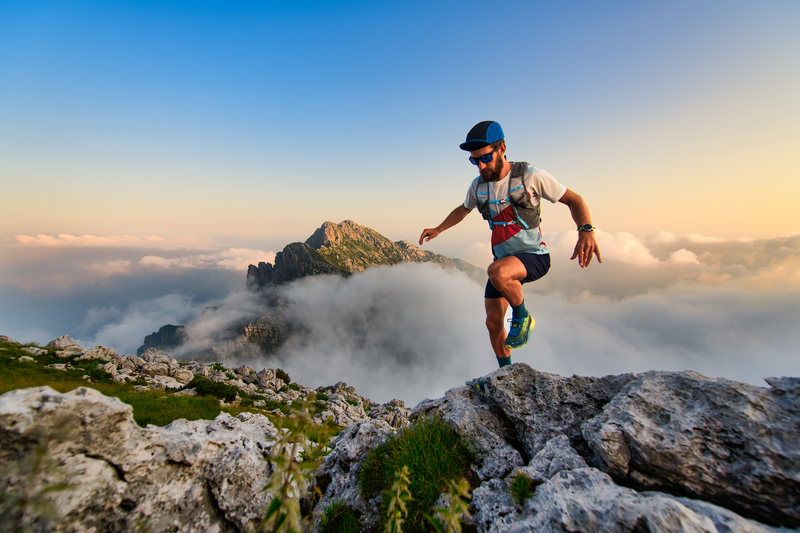
94% of researchers rate our articles as excellent or good
Learn more about the work of our research integrity team to safeguard the quality of each article we publish.
Find out more
ORIGINAL RESEARCH article
Front. Endocrinol. , 04 January 2024
Sec. Experimental Endocrinology
Volume 14 - 2023 | https://doi.org/10.3389/fendo.2023.1293749
This article is part of the Research Topic Interplay Between Regulatory Mechanisms of Stress and Feeding in Fish View all 4 articles
Objective: As one of the most important environmental signals, photoperiod plays a crucial role in regulating the growth, metabolism, and survival of organisms. The photoperiod shifts with the transition of the seasons. The difference in photoperiod between summer and winter is the greatest under natural conditions. However, the effect of photoperiod on Huanghe carp (Cyprinus carpio haematopterus) was paid little attention. We investigated the impact of artificial manipulation of seasonal photoperiod on Huanghe carp by integrating growth performance, intestinal flora, and intestinal metabolome.
Method: We conducted an 8-week culture experiment with summer photoperiod (14 h light:10 h dark, n = 60) as the control group and winter photoperiod (10 h light:14 h dark, n = 60) based on the natural laws.
Results: Winter photoperiod provokes significant weight increases in Huanghe carp. The altered photoperiod contributed to a significant increase in triglyceride and low-density lipoprotein cholesterol levels and the gene expressions of lipid metabolism in the intestine of Huanghe carp. 16s rDNA sequencing revealed that winter photoperiod diminished intestinal flora diversity and altered the abundance. Specifically, the relative abundances of Fusobacteria and Acidobacteriota phyla were higher but Proteobacteria, Firmicutes, and Bacteroidetes phyla were reduced. Analogously, photoperiodic changes induced a significant reduction in the Pseudomonas, Vibrio, Ralstonia, Acinetobacter, and Pseudoalteromonas at the genus level. Additionally, metabolomics analysis showed more than 50% of differential metabolites were associated with phospholipids and inflammation. Microbiome and metabolome correlation analyses revealed that intestinal microbe mediated lipid metabolism alteration.
Conclusion: The winter photoperiod induced intestinal flora imbalance and lipid metabolism modification, ultimately affecting the growth of Huanghe carp. This study provides new insights into the effects of seasonal photoperiodic alteration on the well-being of fish.
The rotational and translational movement of the Earth triggers variations in luminance that fluctuate over a 24-hour or seasons of the year period (1). Most organisms exhibit rhythms in their physiology and behavior. Since identical fish can exhibit circadian behaviors, the circadian rhythm system in fish exhibits profound flexibility (2). Evidence suggested that fish circadian mechanisms include photosensitive central nervous system-related clock organs, peripheral photosensitive tissues with autonomic circadian clocks, and entrainable circadian oscillators. The structurally and functionally complex fish circadian system maintains coordination in order to ensure physiological adaptation to a periodically changing environment (3). Photoperiod is an important factor for fish, involving numerous biological processes such as growth performance, foraging, and survival. Hence, photoperiod is also significant in the long-term cultivation of artificial aquaculture systems.
The gut microbiota has been identified as an environmental factor that significantly impacts digestion absorption and physiological metabolism in animals (4, 5). Its composition and proportions are dynamic, depending on both internal and external factors of the host (6). Different photoperiod regimes have the capacity to shape the microbiota (7–9). Bailey et al. demonstrated that prolonged daylight significantly enhanced the relative abundance of bacteria in the phylum Proteobacteria (10). Reproductive hormone and gene expression levels as well as the gut microbiota of voles (Lasiopodomys brandtii) were also impacted by long-day and short-day photoperiod (11). In a study simulating seasonal changes in light and dark cycles, the ratio of Firmicutes to Bacteroidetes in the cecum and large intestine was significantly higher in winter-simulated conditions than in summer-simulated conditions (9). Wu et al. found that continuous darkness suppressed gut microbial rhythmic oscillations and induced a concomitant increase in the abundance of Clostridia, suggesting that light is a necessary factor for the normal rhythm of intestinal flora (12). Photoperiod manipulation not only affects mammalian gut flora, but also strongly shapes the microbial community in fish. Under extended darkness, gut microbial changes induced impair lipid metabolism in the gut of zebrafish (Danio rerio) and provoke apoptosis, compromising fish health (8). Given this, we speculate that photoperiod may influence carp physiological health by adjusting the composition of the gut microbiota, but such studies are still lacking.
The common carp (Cyprinus carpio) is one of the most widely distributed freshwater fish species with high species diversity. Total production of carp aquaculture reached 4.2 million tons (Mt) in 2020, accounting for 8.6% of the world’s total aquaculture production (13). Huanghe carp (Cyprinus carpio haematopterus) is preferred by consumers because of its profound historical and cultural heritage (14). Photoperiod, as one of the most important environmental signals, impacts organism growth, metabolism, and survival (15, 16). However, the effect of photoperiodic changes was easily ignored on carps in experimental studies and culture. The difference in photoperiod between summer and winter is the greatest under natural conditions, and we were intrigued by the impact of photoperiod on carp in addition to temperature. Therefore, we selected experimental conditions with current seasonal [summer; 14 h light (L):10 h dark (D)] and winter (10L:14D) photoperiods to investigate the effect of seasonal photoperiod changes on the intestinal metabolism of Huanghe carp using gene variations, 16s rDNA sequencing, and metabolomic analyses.
A total of 120 juvenile Huanghe carp (6.5 ± 1.5 g) were selected from the Freshwater Fisheries Research Center, Chinese Academy of Fishery Sciences, and cultured in laboratory tanks for three weeks, after which they were transferred to tanks for acclimatization in the formal experiment for one week (14.5 ± 3 g). The entire experiment was conducted during the summer months. In order to conduct the protocols, the fish were kept in indoor 300-L tanks (20 fish per tank) with constantly aerated water in a recirculating system (temperature 24–28°C, pH 7.2–7.8, dissolved oxygen > 6 mg L−1). Fish were daily fed three times with the refined freshwater fish No. 1 compound feed (crude protein level 35%, crude fat level 3%) from Ningbo Tech-Bank Co., Ltd., slowly until there were no more fish feeding, and the residual bait and feces were removed.
Fish were divided into two groups: the control group (Con, n = 60), which was exposed to a summer lighting cycle (14L:10D); winter (short-period) photoperiod (SP, n = 60), which simulated the sunlight time rhythm of winter pattern in China (10L:14D). Each group had 3 replicates of 20 fish each. Fish were randomly allocated into the experimental tanks. A white light-emitting diode light (main peak 460 nm), simulating the natural light, was hung above the tank. The light intensity was 800 ± 20 luxes in all treatments during the whole experiment. To avoid interference, each tank was covered by light proof black material. In the eighth week of the trial, 10 fish per culture barrel were randomly selected (n = 30/group), euthanized with MS-222 (100 mg L−1), and weighed. Subsequently, these fish were dissected on a sterile bench to collect intestine samples for intestinal microbiota determination and metabolome analysis. All samples were immersed in liquid nitrogen and stored in an ultrafreezer (−80°C) for subsequent examination.
We collected intestinal samples of Huanghe carp for measuring parameters (n = 9/group), including triacylglycerol (TG), total cholesterol (CHOL), and low-density lipoprotein cholesterol (LDL-C) according to the manufacturer’s instructions. All the assay kits were purchased from Nanjing Jiancheng Bioengineering Institute, China. In detail, triacylglycerol and total cholesterol were determined by the GPO-PAP enzyme method, low-density lipoprotein cholesterol was determined by the microplate method.
Gut microbial analysis was performed using 16S rDNA amplicon sequencing to amplify the V4 variable regions of the 16S rRNA gene. Genomic DNA was first extracted from 12 intestinal samples using the CTAB/SDS method (n = 5/group). After detection, PCR amplification and product purification were performed, followed by library construction. Library quantification was followed by up-sequencing using Illumina NovaSeq 6000. The final Amplicon Sequence Variants (ASVs) were obtained by splicing, filtering, and noise reduction for the double-ended reads obtained from sequencing. Species annotation was performed using the classify-sklearn algorithm for each ASV using the naive bayes classifier to obtain the corresponding species information and species-based abundance distribution. The number of sequences of each sample at each taxonomic level (kingdom, phylum, order, family, genus, species) was counted based on the annotation results of ASVs and the characteristic table of each sample. Based on the species annotation results, the top ten species with maximum relative abundance at the taxonomic level of phylum and genus were selected for analysis for each sample. Alpha diversity was calculated using QIIME2 software (v2021.2), and beta diversity was assessed by principal coordinate analysis (PCoA) based on the calculation of Unifrac distance. P < 0.05 was considered a statistically significant difference. The original data analysis was completed by Novogene Co., Ltd. (Beijing, China).
Non-targeted metabolomic analysis using 12 intestinal samples was done on a liquid chromatography-mass spectrometry (LC-MS) (n = 6/group). Metabolites in intestinal tissue were extracted with 1000 μL of a pre-cooled mixture of methanol, acetonitrile, and water (v/v/v, 2/2/1), and then detected by LC-MS/MS. Detect as many molecular characteristic peaks in the sample as possible based on high-resolution mass spectrometry (HRMS) detection technology. Combined with the high-quality mzCloud database constructed from standard materials, mzVault and MassList databases, the molecular characteristic peaks were matched and identified. Spectral processing and database search were performed using Compound Discoverer software (3.1) to obtain qualitative and quantitative results of the metabolites, and then quality control was performed to ensure the accuracy and reliability of the data results. The data were transformed and subjected to partial least squares discriminant analysis (PLS-DA). Hierarchical cluster analysis (HCA) and metabolite correlation analysis were performed on all the differential metabolites obtained. The identified metabolites were annotated using the KEGG database. The screening criteria for differential metabolites were VIP > 1, P < 0.05. The original data analysis of omics was completed by Novogene Co., Ltd. (Beijing, China).
Total RNA from the intestine was extracted with TRIzol reagent (Vazyme Biotechnology Co., LTD., Nanjing, China) (n = 12/group). The quality and quantity of the extracted RNA were evaluated by the OD values and their ratios at 260 and 280 nm (1.8-2.1 for A260/A280). cDNA was synthesized using 1 μg of extracted RNA through a 20 μl reverse transcription reaction system using the HiScript III RT SuperMix for RT-qPCR (+gDNA wiper) kit (Vazyme). RT-qPCR was performed in a 20 μL reaction system containing specific primers and SYBR premixes (Vazyme), and cycling conditions as follows: 95°C pre-denaturation was performed for 30 s, followed by 40 cycles of 95°C for 5 s and 59–62°C for 1 min. Amplification was performed in a Thermal Cycler Dice Real Time System TP800 system. The final concentration of primers used for RT-qPCR was 0.4 μM. We used melting curves to quantify and assess the specificity of their RT-qPCR, and single peaks appeared, which indicated amplicons were specific for the genes. We used No Template Control and Endogenous Control as negative and positive controls to ensure that there was no contamination. Using gapdh as an internal reference (17), the expression levels of target genes were normalized. The expression of relevant RNA was analyzed by 2-ΔΔCT. The primer information of the selected genes is shown in Table 1. Primers for RT-qPCR were designed using primer premier 5.0.
Based on the Spearman correlation coefficient, correlation analysis of the significantly different metabolites was performed based on the LC/MS non-targeted metabolomic analysis. Integrated analysis between differential metabolites and different bacteria was conducted to measure the correlation between species diversity and metabolites in intestinal samples. The range of the correlation coefficient is (−1, +1).
All statistical analyses were performed using IBM SPSS Statistics for Windows, version 27.0 (IBM Corp., Armonk, N.Y., USA). Kolmogorov-Smirnov and Levene’s test were utilized to test the normality of distribution of the data and homogeneity of variances, respectively. Student’s t-test was used to test the significance between the Con and SP groups in cases where the data obeyed a normal distribution and exhibit homoscedasticity. Mann-Whitney U-test was used when the data did not conform to normal distribution or homoscedastic. P < 0.05 was considered statistically significant. Data were expressed as the mean ± standard error (SEM).
Compared with the Con, the carp in SP group had a significant increase in body weight (P = 0.048) (Figure 1A). Seasonal photoperiodic exposure altered the expression of genes related to intestinal rhythm and lipid metabolism in carp, such as a significant up-regulation of nfil3 (nuclear factor interleukin-3-regulated protein) (P = 0.028) and rev-erbα (nuclear receptor subfamily 1) (P = 0.034) was significantly decreased in SP (Figure 1B). It has been shown that nfil3 is negatively regulated by upstream biological clock signaling (rev-erbα) and further affects intestinal lipid metabolism (18), so we examined the lipid levels in the intestine. The SP group showed significantly higher expression of intestinal adipogenesis-related genes including acsl1 (acyl-CoA synthetase long chain family member 1) (P = 0.017), scd (stearoyl-CoA desaturase) (P = 0.034), elovl1 (elongation of very long chain fatty acids protein 1) (P = 0.043), and c/ebpγ (CCAAT/enhancer-binding protein gamma) (P = 0.049) (Figure 1B). Similarly, TG (P = 0.016) and LDL-C (P = 0.009) levels were significantly higher in the intestine of Huanghe carp under winter photoperiod, but there was no significant difference in CHOL (Figure 1C).
Figure 1 Seasonal light interferes with the circadian rhythm of Huanghe carp and causes intestinal lipid metabolism disorder. (A) Body weight (g) in Con and SP groups (n = 30/group). (B) Expression of genes associated with circadian rhythm and lipid metabolism in intestine (n = 12/group). (C) Levels of TG, CHOL, and LDL-C in the intestine of carp in Con and SP groups (n = 9/group). rev-erbα, nuclear receptor subfamily 1; nfil3, nuclear factor interleukin-3-regulated protein; acsl1, acyl-CoA synthetase long chain family member 1; scd, stearoyl-CoA desaturase; elovl1, elongation of very long chain fatty acids protein 1; c/ebpγ, CCAAT/enhancer-binding protein gamma; TG, triglyceride; CHOL, cholesterol; LDL-C, low-density lipoprotein cholesterol. The values are mean ± SEM.
Experiments were conducted to assess the composition and diversity of the gut microbial community of Huanghe carp under seasonal photoperiod using high-throughput sequencing technology. To evaluate the differences in alpha diversity among the groups, we analyzed chao1, shannon, and simpson indicators (Figure 2A). Changes in photoperiod significantly altered the shannon index compared to Con (P = 0.011). Principal coordinate analysis (PCoA) was further performed to assess the degree of similarity of microbial communities among the groups (Figure 2B). The results showed that the microbial community composition of SP was significantly different from that of the Con. As shown in Figures 2C, D, the microbial abundance differed between the Con and SP groups at the phylum and genus levels, respectively. And both groups have similar dominant flora. At the phylum level, Proteobacteria, Fusobacteria, and Firmicutes were detected as the dominant phyla in carp intestine (Figure 2C). At the genus level, Aeromonas, Cetobacterium, Brevinema, Pseudomonas, Vibrio, and Ralstonia were the dominant genera in the intestine of Huanghe carp (Figure 2D).
Figure 2 Effects of seasonal photoperiod on the gut microbiota diversity of Huanghe carp. (A) Alpha diversity indexes results. (B) PCoA results. (C) Relative abundance of species at the phylum level. (D) Relative abundance of species at the genus level. The values are mean ± SEM, n = 5/group.
More specifically, we compared the abundance of different bacteria in the SP group with that of Con in Figure 3. At the phylum level (Figure 3A), photoperiod alteration significantly increased the abundance of Fusobacteria and Acidobacteriota phyla and decreased the abundance of Proteobacteria (P = 0.047), Firmicutes (P = 0.01), and Bacteroidetes phyla (P = 0.009). At the genus level (Figure 3B), the abundance of Pseudomonas (P = 0.016), Vibrio (P = 0.007), Ralstonia (P = 0.01), Acinetobacter (P = 0.009), and Pseudoalteromonas (P = 0.007) was significantly reduced, while at the same time the number of Cetobacterium (P = 0.046) was significantly increased.
Figure 3 Phylum and genus level differential flora due to seasonal photoperiod. (A) Screened bacteria with significant differences by phylum level. (B) Screened bacteria with significant differences by genus level. ASVs, Amplicon Sequence Variants. The values are mean ± SEM, n = 5/group.
In conjunction with the previous findings, we performed non-targeted metabolomic analyses of carp intestinal samples exposed to seasonal photoperiod to further explore the effects of seasonal photoperiodic changes on the gut. To maximize the metabolite coverage, both positive ion (pos) and negative ion (neg) modes were used in the assay analysis. According to the PLS-DA plot, it can be shown that metabolites are largely separated between SP and Con (Figure 4A). Under simulated winter light duration conditions, 43 differential metabolites (DMs) were screened of which 18 were up-regulated and 25 were down-regulated (Figure 4B). Additionally, these 43 DMs separated positive and negative metabolites were clustered into different subclusters according to their relative content in the heatmap (Figure 4C).
Figure 4 Characteristics of gut metabolites between the Con and SP groups (n = 6/group). (A) PLS-DA scores plots. (B) Volcanic map of differential metabolites. Red represents the up-regulation; blue represents the down-regulation; gray represents no significant change. (C) Hierarchical cluster analysis of differential metabolites. pos. and neg. stand for positive and negative ion modes applied to detection analysis.
We observed DMs induced by winter light mainly associated with phospholipids and inflammation (Figure 5). We found significant reductions in phospholipid-related metabolites, including phosphatidylcholine (PC), phosphatidylethanolamine (PE), lysophosphatidic acids (LPA), and lysophosphatidylethanolamine (LPE) (P < 0.05) (Figure 5A). This suggests that the reduction in light duration altered phospholipid levels and triggered abnormal lipid metabolism in intestine. Notably, arachidonic acid metabolism intermediates such as Prostaglandin F2α (PGF2α) (P = 0.028), 8-Iso prostaglandin A2 (8-Iso PGA2) (P = 0.04), 11-HETE (P = 0.044), and 16(R)-HETE (P = 0.02) were upregulated (Figure 5B). Subsequently, we assessed whether photoperiodically altered carp intestinal lipid-related metabolites were associated with inflammation-related metabolites. Spearman correlation analysis revealed that PCs, PE, and LPA were negatively correlated with most of the inflammation-related metabolites such as PGF2α, 8-Iso PGA2, 11-HETE, and 16(R)-HETE. The opposite was cis-gondoic acid, which revealed a positive significant correlation with the lipid-related metabolites, totally opposite to the rest of inflammation-related metabolites (Figure 5C).
Figure 5 Correlation analysis of intestinal differential metabolites in Huanghe carp between Con and SP groups. (A) Phospholipid-related differential metabolites. (B) Inflammatory-related differential metabolites. (C) Spearman correlation between phospholipid related metabolites and inflammation related metabolites under winter (short-period) photoperiod. PC, phosphatidylcholine; PE, phosphatidylethanolamine; LPA, lysophosphatidic acids; LPE, lysophosphatidylethanolamine; PGF2α, Prostaglandin F2α; 8-Iso PGA2, 8-Iso prostaglandin A2. The values are mean ± SEM, n = 6/group. *P < 0.05, **P < 0.01.
Correlation analysis was used to analyze the correlation between differential flora and lipid- and inflammation-related DMs to determine the relationship between intestinal metabolites and microorganisms. As shown in Figure 6, Firmicutes and Bacteroidetes were positively correlated with PC(16:2/24:4), PC(20:1/22:6), PC(20:3/20:3), and LPA19:0 at phylum level. Whereas PC(18:1/18:1) was only positively correlated with Firmicutes, but not with Bacteroidetes. Additionally, there was a highly positive correlation between Proteobacteria and PC(20:1/22:6), Fusobacteriota was significantly negatively correlated with PE(19:1/20:5), and Acidobacteriota was negatively correlated with PC(20:3/20:3) and LPA19:0. At the genus level, PC(20:1/22:6) was significantly positively correlated with Pseudomonas, Vibrio, Ralstonia, and Pseudoalteromonas, and PC(20:3/20:3) was positively correlated with Pseudomonas, Ralstonia, and Acinetobacter. PE(19:1/20:5) was positively correlated with Acinetobacter and significantly negatively correlated with Cetobacterium. Moreover, arachidonic acid metabolism intermediate 16(R)-HETE had a markedly negative correlation with Bacteroidetes and Pseudomonas. Overall, Firmicutes, Bacteroidetes, and Acinetobacter were the shared flora for metabolites PC and LPA. Most of the intestinal flora and inflammatory metabolites had no obvious direct correlation, but we found an association through the correlation of lipid-related metabolites and inflammation-related metabolites (Figure 5C).
Figure 6 Heatmap of correlation between the gut differential metabolites and differential microbiota under the influence of winter (short-period) photoperiod. Orange indicates positive correlations and green indicates negative correlations. *P < 0.05, **P < 0.01.
Annual variation of photoperiod is the temporal organization of seasonal activities in the biological community, which plays an important role in regulating physiological processes such as growth, reproduction, and sex determination in fish (19, 20). Periodic environmental changes coordinate biological functions through the complex circadian system of fish (3). The variation in nfil3 and rev-erbα in the present study also indicated that photoperiodic changes contribute to the regulation of clock genes, which further affects lipid metabolism (18). Various studies have demonstrated that circadian rhythm disruption broadly affects host lipid metabolism (21–24). It is well documented that seasonal variation such as photoperiod affect lipid deposition in farmed fish as well as the metabolic status of fish (25). Similarly, we observed increased body weight and intestinal TG and LDL-C in the SP group, which is consistent with the study by Xie et al. (26). Also, the expression of intestinal lipid synthesis-related genes acsl1, scd, elovl1, and c/ebpγ were higher under intestinal winter photoperiod in comparison to the control group. Together, winter photoperiod enhanced the expression of lipid synthesis-related genes, and thus lipid accumulation may be the crucial reason for weight gain under winter photoperiod.
Dysbiosis of the intestinal flora interferes with fish growth and development, nutrient metabolism, and immune regulation, ultimately affecting fish health (27). Moreover, intestinal flora modulates intestinal epithelial development and stimulates the immune system to regulate fish physiological functions (27, 28). Gut microbes are highly susceptible to changes in the external environment. The external environment transforms the composition and diversity of fish intestinal flora, resulting in modifications of metabolic functions and thus affecting the growth of fish (29). To our knowledge, there are few reports on the effects of photoperiod on the gut microbes of aquatic animals. We observed a decrease in microbial diversity in the SP group. It was shown that the alpha diversity of fecal microbiota was significantly lower under a 6L:18D photoperiod compared to the normal 12L:12D cycle, suggesting that photoperiod helps to maintain a higher species of gut microbiota (30). However, short photoperiod exposure reduces the number of bacterial species and remodels the distribution of intestinal flora (31). Thus, it’s difficult to maintain higher number of gut bacteria species throughout the winter photoperiod, which mean that the intestinal flora homeostasis was disrupted, possibly mediating metabolic shifts in Huanghe carp.
In addition to diversity, the relative abundance of intestinal flora directly reveals the function of specific gut microbes. In general, under normal conditions, the composition of the intestinal flora tends to be dynamically balanced and relatively stable (32, 33). However, the winter photoperiod showed significantly different gut microbial composition. Photoperiodic changes caused the differences in the abundance of intestinal flora (8). Arreaza-Gil et al. found that photoperiodic effects exhibited a trend toward decreased relative abundance of Firmicutes and increased Bacteroidetes level under 18L:6D (30). In the present experiment, the winter photoperiod showed lower abundance of Firmicutes and Bacteroidetes. In fact, members of Firmicutes such as Lactobacillus are involved in various carbon sources fermentation (34). Additionally, Bacteroidetes contains some bacteria with fermentative function on carp intestinal food (35), acting mainly on the metabolism of polysaccharides and bile acids (36). Thus, the winter photoperiod affects the intestinal digestion of Huanghe carp. Moreover, our experiments revealed a significant increase in the abundance of Fusobacteria in SP. Elevated Fusobacteria produces lipopolysaccharides (LPS) and endotoxins, involving inflammatory responses in the intestine (37). At the genus level, most of the bacterial genera altered by photoperiod belong to Proteobacteria phyla. For example, the relative abundance of Pseudomonas, Vibrio, Ralstonia, Acinetobacter, and Pseudoalteromonas was significantly reduced. It has been shown that Vibrio, Pseudomonas, and Acinetobacter contribute to some extent to intestinal digestion in fish (35, 38). Pseudomonas as a potential probiotic has important functions on intestine health, and Vibrio is one of the cellulose degrading microorganisms from the intestine of grass carp (39). In a study on sea bass (Dicentrarchus labrax), Acinetobacter, Pseudomonas, and Vibrio genera in the gut produced lipases and proteases (40). MacDonald et al. reported that strains belonging to Acinetobacter and Vibrio in the gut microbiota of Dover sole (Solea solea L.) were able to degrade chitin (41). Thus, it is evident that the winter photoperiod adversely affects the intestinal flora by modifying digestion and metabolism of nutrients. These changes in flora structure may also be a trigger of the body weight gain under winter photoperiod.
Crosstalk between the gut microbiota and its host depends in part on the production of metabolites, which have a significant impact on host physiology (42). Arachidonic acid related metabolites and phospholipids such as PE, PCs, and LPA exhibited obvious variations in Huanghe carp under the winter photoperiod. Winter photoperiod decreased almost all of the phospholipid levels, indicating that phospholipid metabolism in the intestine of Huanghe carp is extremely sensitive to seasonal photoperiod. In the present study, bacteria such as Firmicutes, Bacteroidetes, and Fusobacteria phyla showed a significant correlation with PC and PE. PC is the main bioactive phospholipid in the intestine (43). Alterations in microbial communities may play an important role in PC utilization (44). However, PE is involved in mitochondrial respiration and autophagy. In addition to its role in membrane structure formation, PE as a substrate is implicated in specific biosynthesis such as PC (44, 45). PE is a major precursor of endogenous cannabinoids with regulatory intestinal barrier function and anti-inflammatory effects, and its reduced levels can have a negative impact on mitochondrial energy metabolism and impair cell survival and growth (46–48). Down-regulated Proteobacteria, Firmicutes and Bacteroidetes were positively correlated with many PCs. However, up-regulated Fusobacteria was negatively correlated with PE. The results demonstrate that the changes in phospholipid metabolism under winter photoperiod are inextricably linked to gut microbe alterations in Huanghe carp. Additionally, PC and PE were mostly negatively correlated with arachidonic acid metabolism intermediates such as PGF2α, 8-Iso PGA2, 11-HETE, 16(R)-HETE. PC hydrolysis leads to the formation of fatty acids such as arachidonic acid, which activates inflammatory pathways. Arachidonic acid produces prostaglandins, thromboxaneA2, lipoxygenases, leukotrienes, and other metabolic products through three metabolic pathways regulated by cyclooxygenases (COX), lipoxygenases (LOX) and cytochrome P450 (CYP450) (49). These products are widely involved in organ function maintenance, inflammatory response and other physiological processes (50). Therefore, it is hypothesized that winter photoperiod affects lipid metabolism to trigger the production of inflammatory mediators.
Our study has shown that artificially imposed winter photoperiod can significantly increase body weight and induce lipid metabolism disorder in Huanghe carp. The changes in intestinal lipid metabolism in carp may be closely related to intestinal flora and various metabolites. Winter photoperiod reduced the relative abundance of some bacteria on nutrient digestion and metabolism in fish, such as the Firmicutes and Bacteroidetes phyla, and Pseudomonas, Vibrio, and Acinetobacter genera. The decreased phospholipids such as intestinal PC and PE were associated with an increase in inflammatory mediators such as prostaglandins and intermediates of arachidonic acid metabolism.
The data presented in the study are deposited in the National Center for Biotechnology Information (NCBI) repository, accession number PRJNA982570.
The animal study was approved by Nanjing Agricultural University. The study was conducted in accordance with the local legislation and institutional requirements.
WW: Conceptualization, Data curation, Formal Analysis, Methodology, Software, Validation, Visualization, Writing – original draft. SS: Conceptualization, Methodology, Software, Visualization, Writing – review & editing. PD: Investigation, Software, Validation, Writing – review & editing. WF: Formal Analysis, Project administration, Software, Writing – review & editing. JL: Data curation, Formal Analysis, Investigation, Software, Writing – review & editing. CZ: Formal Analysis, Software, Writing – review & editing. YT: Conceptualization, Funding acquisition, Methodology, Project administration, Resources, Supervision, Writing – review & editing.
The author(s) declare financial support was received for the research, authorship, and/or publication of this article. This research was supported by the earmarked fund for CARS (CARS-45), National Key Research and Development Program (2022YFF0608203), Central Public-interest Scientific Institution Basal Research Fund, CAFS (2020TD37, 2023XT0303).
The authors thank the students and staff of the Aquatic Genetic Laboratory, Freshwater Fisheries Research Center of Chinese Academy of Fishery Sciences for their kind assistance in the study. Additionally, the authors gratefully acknowledge Jianxiang Chen from Nanjing Agricultural University for his assistance during the experimental and writing period.
The authors declare that the research was conducted in the absence of any commercial or financial relationships that could be construed as a potential conflict of interest.
All claims expressed in this article are solely those of the authors and do not necessarily represent those of their affiliated organizations, or those of the publisher, the editors and the reviewers. Any product that may be evaluated in this article, or claim that may be made by its manufacturer, is not guaranteed or endorsed by the publisher.
1. Ruiz de Azua MJ, Manocchio F, Cruz-Carrión Á, Arola-Arnal A, Torres-Fuentes C, Bernal CA, et al. Fatty acid metabolism in liver and muscle is strongly modulated by photoperiod in Fischer 344 rats. J Photochem Photobiol B Biol (2023) 238:112621. doi: 10.1016/j.jphotobiol.2022.112621
2. Idda ML, Bertolucci C, Vallone D, Gothilf Y, Sánchez-Vázquez FJ, Foulkes NS. Circadian clocks: lessons from fish. Prog Brain Res (2012) 199:41–57. doi: 10.1016/B978-0-444-59427-3.00003-4
3. Irina Z, Stéphan R. Circadian rhythms in fish. In: Fish physiology 24: behaviour and physiology of fishes. New York: Elsevier (2006). p. 197–238.
4. Lacroix S, Pechereau F, Leblanc N, Boubertakh B, Houde A, Martin C, et al. Rapid and concomitant gut microbiota and endocannabinoidome response to diet-induced obesity in mice. mSystems. (2019) 4(6):e00407–19. doi: 10.1128/mSystems.00407-19
5. Zhao K, Ge Q, Zhang X, Shao X, Wei Y, Wang H, et al. Genomic analysis of intestinal flora and liver genes in mice with circadian rhythm disorders fed with flavonoids from Sedum aizoon L. Food Biosci (2022) 50:102067. doi: 10.1016/j.fbio.2022.102067
6. Hasan N, Yang H. Factors affecting the composition of the gut microbiota, and its modulation. PeerJ. (2019) 7:e7502. doi: 10.7717/peerj.7502
7. Liang X, Bushman FD, FitzGerald GA. Time in motion: the molecular clock meets the microbiome. Cell. (2014) 159:469–70. doi: 10.1016/j.cell.2014.10.020
8. Basili D, Lutfi E, Falcinelli S, Balbuena-Pecino S, Navarro I, Bertolucci C, et al. Photoperiod manipulation affects transcriptional profile of genes related to lipid metabolism and apoptosis in zebrafish (Danio rerio) larvae: potential roles of gut microbiota. Microb Ecol (2020) 79:933–46. doi: 10.1007/s00248-019-01468-7
9. Matsumoto S, Ren L, Iigo M, Murai A, Yoshimura T. Mimicking seasonal changes in light-dark cycle and ambient temperature modulates gut microbiome in mice under the same dietary regimen. PloS One (2023) 18:e0278013. doi: 10.1371/journal.pone.0278013
10. Bailey MT, Walton JC, Dowd SE, Weil ZM, Nelson RJ. Photoperiod modulates gut bacteria composition in male Siberian hamsters (Phodopus sungorus). Brain Behav Immun (2010) 24:577–84. doi: 10.1016/j.bbi.2009.12.010
11. Zhu H, Li G, Liu J, Xu X, Zhang Z. Gut microbiota is associated with the effect of photoperiod on seasonal breeding in male Brandt's voles (Lasiopodomys brandtii). Microbiome. (2022) 10:194. doi: 10.1186/s40168-022-01381-1
12. Wu G, Tang W, He Y, Hu J, Gong S, He Z, et al. Light exposure influences the diurnal oscillation of gut microbiota in mice. Biochem Biophys Res Commun (2018) 501:16–23. doi: 10.1016/j.bbrc.2018.04.095
13. FAO. The state of world fisheries and aquaculture 2022. Towards Blue Transformation. Rome: FAO. (2022). doi: 10.4060/cc0461en
14. Su S, Li H, Du F, Zhang C, Li X, Jing X, et al. Combined QTL and genome scan analyses with the help of 2b-RAD identify growth-associated genetic markers in a new fast-growing carp strain. Front Genet (2018) 9:592. doi: 10.3389/fgene.2018.00592
15. Fu X, Zou Z, Zhu J, Xiao W, Li D, Yu J, et al. Effects of different photoperiods on growth performance, daily rhythm of growth axis-related genes, and hormones in Nile tilapia (Oreochromis niloticus). Aquaculture. (2022) 553:738071. doi: 10.1016/j.aquaculture.2022.738071
16. Chen S, Liu J, Shi C, Migaud H, Ye Y, Song C, et al. Effect of photoperiod on growth, survival, and lipid metabolism of mud crab Scylla paramamosain juveniles. Aquaculture. (2023) 567:739279. doi: 10.1016/j.aquaculture.2023.739279
17. Zhang C, Su S, Li X, Li B, Yang B, Zhu J, et al. Comparative transcriptomics identifies genes differentially expressed in the intestine of a new fast-growing strain of common carp with higher unsaturated fatty acid content in muscle. PloS One (2018) 13:e0206615. doi: 10.1371/journal.pone.0206615
18. Wang Y, Kuang Z, Yu X, Ruhn KA, Kubo M, Hooper LV. The intestinal microbiota regulates body composition through NFIL3 and the circadian clock. Science. (2017) 357:912–6. doi: 10.1126/science.aan0677
19. Maitra SK, Seth M, Chattoraj A. Photoperiod, pineal photoreceptors and melatonin as the signal of photoperiod in the regulation of reproduction in fish. J Endocrinol Reprod (2006) 10:73–87.
20. Brown EE, Baumann H, Conover DO. Temperature and photoperiod effects on sex determination in a fish. J Exp Mar Biol Ecol (2014) 461:39–43. doi: 10.1016/j.jembe.2014.07.009
21. Paredes JF, López-Olmeda JF, Martínez FJ, Sánchez-Vázquez FJ. Daily rhythms of lipid metabolic gene expression in zebra fish liver: Response to light/dark and feeding cycles. Chronobiol. Int (2015) 32(10):1438–48. doi: 10.3109/07420528.2015.1104327
22. Li Y, Ma J, Yao K, Su W, Tan B, Wu X, et al. Circadian rhythms and obesity: Timekeeping governs lipid metabolism. J Pineal Res (2020) 69(3):e12682. doi: 10.1111/jpi.12682
23. Pan X, Mota S, Zhang B. Circadian clock regulation on lipid metabolism and metabolic diseases. Adv Exp Med Biol (2020) 1276:53–66. doi: 10.1007/978-981-15-6082-8_5
24. Schäbler S, Amatobi KM, Horn M, Rieger D, Helfrich-Förster C, Mueller MJ, et al. Loss of function in the Drosophila clock gene period results in altered intermediary lipid metabolism and increased susceptibility to starvation. Cell Mol Life Sci (2020) 77:4939–56. doi: 10.1007/s00018-019-03441-6
25. Weil C, Lefèvre F, Bugeon J. Characteristics and metabolism of different adipose tissues in fish. Rev Fish Biol Fisheries. (2013) 23:157–73. doi: 10.1007/s11160-012-9288-0
26. Xie X, Zhao B, Huang L, Shen Q, Ma L, Chen Y, et al. Effects of altered photoperiod on circadian clock and lipid metabolism in rats. Chronobiol. Int (2017) 34:1094–104. doi: 10.1080/07420528.2017.1341906
27. Larsen AM, Mohammed HH, Arias CR. Characterization of the gut microbiota of three commercially valuable warmwater fish species. J Appl Microbiol (2014) 116:1396–404. doi: 10.1111/jam.12475
28. Song W, Li L, Huang H, Jiang K, Zhang F, Chen X, et al. The gut microbial community of antarctic fish detected by 16S rRNA gene sequence analysis. Biomed Res Int (2016) 2016:3241529. doi: 10.1155/2016/3241529
29. Yukgehnaish K, Kumar P, Sivachandran P, Marimuthu K, Arshad A, Paray BA, et al. Gut microbiota metagenomics in aquaculture: factors influencing gut microbiome and its physiological role in fish. Rev Aquacult. (2020) 12:1903–27. doi: 10.1111/raq.12416
30. Arreaza-Gil V, Escobar-Martínez I, Suárez M, Bravo FI, Muguerza B, Arola-Arnal A, et al. Gut seasons: photoperiod effects on fecal microbiota in healthy and cafeteria-induced obese fisher 344 rats. Nutrients. (2022) 14(3):722. doi: 10.3390/nu14030722
31. Cui M, Xiao H, Luo D, Zhang X, Zhao S, Zheng Q, et al. Circadian rhythm shapes the gut microbiota affecting host radiosensitivity. Int J Mol Sci (2016) 17(11):1786. doi: 10.3390/ijms17111786
32. Lin Z, Wu J, Wang J, Levesque CL, Ma X. Dietary Lactobacillus reuteri prevent from inflammation mediated apoptosis of liver via improving intestinal microbiota and bile acid metabolism. Food Chem (2023) 404:134643. doi: 10.1016/j.foodchem.2022.134643
33. Yin H, Chai R, Qiu H, Tao C, Huang L, Wang H, et al. Effects of Isaria cicadae on growth, gut microbiota, and metabolome of Larimichthys crocea. Fish Shellfish Immunol (2023) 136:108719. doi: 10.1016/j.fsi.2023.108719
34. Ringø E, Gatesoupe FJ. Lactic acid bacteria in fish: a review. Aquaculture. (1998) 160:177–203. doi: 10.1016/S0044-8486(97)00299-8
35. van Kessel MA, Dutilh BE, Neveling K, Kwint MP, Veltman JA, Flik G, et al. Pyrosequencing of 16S rRNA gene amplicons to study the microbiota in the gastrointestinal tract of carp (Cyprinus carpio L.). AMB Express. (2011) 1:41. doi: 10.1186/2191-0855-1-41
36. Wang T, Han J, Dai H, Sun J, Ren J, Wang W, et al. Polysaccharides from Lyophyllum decastes reduce obesity by altering gut microbiota and increasing energy expenditure. Carbohydr. Polym. (2022) 295:119862. doi: 10.1016/j.carbpol.2022.119862
37. Sveen K. The capacity of lipopolysaccharides from bacteroides, fusobacterium and veillonella to produce skin inflammation and the local and generalized Shwartzman reaction in rabbits. J Periodont. Res (1977) 12:340–50. doi: 10.1111/j.1600-0765.1977.tb01525.x
38. Ray AK, Ghosh K, Ringø E. Enzyme-producing bacteria isolated from fish gut: a review. Aquacult. Nutr (2012) 18:465–92. doi: 10.1111/j.1365-2095.2012.00943.x
39. Wu S, Wang G, Angert ER, Wang W, Li W, Zou H. Composition, diversity, and origin of the bacterial community in grass carp intestine. PloS One (2012) 7:e30440. doi: 10.1371/journal.pone.0030440
40. Gatesoupe FJ, Infante JLZ, Cahu C, Quazuguel P. Early weaning of seabass larvae, Dicentrarchus labrax: the effect on microbiota, with particular attention to iron supply and exoenzymes. Aquaculture. (1997) 158:117–27. doi: 10.1016/S0044-8486(97)00179-8
41. MacDonald NL, Stark JR, Austin B. Bacterial microflora in the gastro-intestinal tract of Dover sole (Solea solea L.), with emphasis on the possible role of bacteria in the nutrition of the host. FEMS Microbiol Lett (1986) 35:107–11. doi: 10.1111/j.1574-6968.1986.tb01508.x
42. Levy M, Blacher E, Elinav E. Microbiome, metabolites and host immunity. Curr Opin Microbiol (2017) 35:8–15. doi: 10.1016/j.mib.2016.10.003
43. Schmitz MG, Renooij W. Phospholipids from rat, human, and canine gastric mucosa. Composition and metabolism of molecular classes of phosphatidylcholine. Gastroenterology. (1990) 99:1292–6. doi: 10.1016/0016-5085(90)91152-v
44. Boldyreva LV, Morozova MV, Saydakova SS, Kozhevnikova EN. Fat of the gut: epithelial phospholipids in inflammatory bowel diseases. Int J Mol Sci (2021) 22(21):11682. doi: 10.3390/ijms222111682
45. Böttinger L, Horvath SE, Kleinschroth T, Hunte C, Daum G, Pfanner N, et al. Phosphatidylethanolamine and cardiolipin differentially affect the stability of mitochondrial respiratory chain supercomplexes. J Mol Biol (2012) 423:677–86. doi: 10.1016/j.jmb.2012.09.001
46. DiPatrizio NV. Endocannabinoids in the gut. Cannabis Cannabinoid Res (2016) 1:67–77. doi: 10.1089/can.2016.0001
47. van der Veen JN, Kennelly JP, Wan S, Vance JE, Vance DE, Jacobs RL. The critical role of phosphatidylcholine and phosphatidylethanolamine metabolism in health and disease. Biochim Biophys Acta Biomembr. (2017) 1859:1558–72. doi: 10.1016/j.bbamem.2017.04.006
48. Tsuboi K, Uyama T, Okamoto Y, Ueda N. Endocannabinoids and related N-acylethanolamines: biological activities and metabolism. Inflamm Regen. (2018) 38:28. doi: 10.1186/s41232-018-0086-5
49. Wang B, Wu L, Chen J, Dong L, Chen C, Wen Z, et al. Metabolism pathways of arachidonic acids: mechanisms and potential therapeutic targets. Sig. Transduction Targeting Ther (2021) 6(1):94. doi: 10.1038/s41392-020-00443-w
Keywords: Cyprinus carpio, seasonal photoperiod, microbiota, metabolism, aquaculture
Citation: Wang W, Su S, Dong P, Feng W, Li J, Zhang C and Tang Y (2024) Effects of simulated winter short photoperiods on the microbiome and intestinal metabolism in Huanghe carp (Cyprinus carpio haematopterus). Front. Endocrinol. 14:1293749. doi: 10.3389/fendo.2023.1293749
Received: 29 September 2023; Accepted: 27 November 2023;
Published: 04 January 2024.
Edited by:
Nuria De Pedro, Complutense University of Madrid, SpainReviewed by:
Jesús M. Míguez, University of Vigo, SpainCopyright © 2024 Wang, Su, Dong, Feng, Li, Zhang and Tang. This is an open-access article distributed under the terms of the Creative Commons Attribution License (CC BY). The use, distribution or reproduction in other forums is permitted, provided the original author(s) and the copyright owner(s) are credited and that the original publication in this journal is cited, in accordance with accepted academic practice. No use, distribution or reproduction is permitted which does not comply with these terms.
*Correspondence: Yongkai Tang, dGFuZ3lrQGZmcmMuY24=
Disclaimer: All claims expressed in this article are solely those of the authors and do not necessarily represent those of their affiliated organizations, or those of the publisher, the editors and the reviewers. Any product that may be evaluated in this article or claim that may be made by its manufacturer is not guaranteed or endorsed by the publisher.
Research integrity at Frontiers
Learn more about the work of our research integrity team to safeguard the quality of each article we publish.