- 1School of Medicine and Doctoral Program of Clinical and Experimental Medicine, College of Medicine and Center of Excellence for Metabolic Associated Fatty Liver Disease, National Sun Yat-sen University, Kaohsiung, Taiwan
- 2Division of Cardiology, Department of Internal Medicine, Chi-Mei Medical Center, Tainan, Taiwan
- 3Department of Biotechnology, Southern Taiwan University of Science and Technology, Tainan, Taiwan
- 4Department of Anesthesiology, E-Da Hospital, I-Shou University, Kaohsiung, Taiwan
- 5Department of Nursing, College of Medicine, I-Shou University, Kaohsiung, Taiwan
- 6School of Medicine, I-Shou University, Kaohsiung, Taiwan
- 7Department of Surgery, National Cheng Kung University Hospital, College of Medicine, National Cheng Kung University, Tainan, Taiwan
- 8Department of Nutrition, Chi Mei Medical Center, Tainan, Taiwan
- 9Department of Neurology, Chi-Mei Medical Center, Tainan, Taiwan
- 10Department of Anesthesiology, Chi Mei Medical Center, Tainan, Taiwan
- 11School of Medicine, College of Medicine, National Sun Yat-sen University, Kaohsiung, Taiwan
- 12Department of Anesthesiology, Chi Mei Medical Center, Liouying, Tainan, Taiwan
Introduction: Contrast-induced nephropathy (CIN) is a common complication of percutaneous coronary intervention (PCI). Identifying patients at high CIN risk remains challenging. The triglyceride-glucose (TyG) index may help predict CIN but evidence is limited. We conducted a meta-analysis to evaluate the diagnostic value of TyG index for CIN after PCI.
Methods: A systematic literature search was performed in MEDLINE, Cochrane, and EMBASE until August 2023 (PROSPERO registration: CRD42023452257). Observational studies examining TyG index for predicting CIN risk in PCI patients were included. This diagnostic meta-analysis aimed to evaluate the accuracy of the TyG index in predicting the likelihood of CIN. Secondary outcomes aimed to assess the pooled incidence of CIN and the association between an elevated TyG index and the risk of CIN.
Results: Five studies (Turkey, n=2; China, n=3) with 3518 patients (age range: 57.6 to 68.22 years) were included. The pooled incidence of CIN was 15.3% [95% confidence interval (CI) 11-20.8%]. A high TyG index associated with increased CIN risk (odds ratio: 2.25, 95% CI 1.82-2.77). Pooled sensitivity and specificity were 0.77 (95% CI 0.59-0.88) and 0.55 (95% CI 0.43-0.68) respectively. Analysis of the summary receiver operating characteristic (sROC) curve revealed an area under the curve of 0.69 (95% CI 0.65-0.73). There was a low risk of publication bias (p = 0.81).
Conclusion: The TyG index displayed a noteworthy correlation with the risk of CIN subsequent to PCI. However, its overall diagnostic accuracy was found to be moderate in nature. While promising, the TyG index should not be used in isolation for CIN screening given the heterogeneity between studies. In addition, the findings cannot be considered conclusive given the scarcity of data. Further large-scale studies are warranted to validate TyG cutoffs and determine how to optimally incorporate it into current risk prediction models.
Systematic Review Registration: https://www.crd.york.ac.uk/prospero/display_record.php?ID=CRD42023452257, identifier CRD42023452257.
1 Introduction
Contrast-induced nephropathy (CIN), characterized by rapidly declining renal function following the administration of iodinated contrast agents, is the most commonly encountered dilemma during percutaneous coronary intervention (PCI) (1–4). CIN is associated with poor clinical outcomes including long-term deterioration in kidney function and increased mortality risk (5–7). Therefore, identifying patients at high risk of CIN is crucial to optimize prevention strategies and clinical management. Predicting CIN involved assessing risk factors and identifying high-risk patients, which remains challenging (1, 3, 8). To date, although pre-existing kidney disease, diabetes, advanced age, hypertension, and heart failure are reported as risk factors of CIN, whether other index also contribute to the development of CIN is still largely unknown (1, 2, 8).
Research interest has recently focused on exploring whether certain metabolic markers could further refine CIN risk stratification. The triglyceride–glucose (TyG) index, which is determined by fasting triglyceride and glucose levels, has been regarded as a marker representing insulin resistance and metabolic status (9–11). TyG index was calculated as the Ln[fasting triglycerides(mg/dl) × fasting glucose (mg/dL)/2]. Studies have suggested that the TyG index could serve as a predictive marker for cardiovascular risk such as coronary artery disease, heart attack, and stroke (9, 10, 12). In addition, some studies have explored the potential connection between the TyG index and nephropathy, particularly in diabetic nephropathy (13–17). A higher TyG index, indicative of insulin resistance, can contribute to inflammation, oxidative stress, and vascular dysfunction (9, 15, 18). In addition, the TyG index exhibits a positive correlation with a high risk of developing diabetic nephropathy and other renal-related complications (15, 17).
Emerging evidence suggests that the TyG index may predict the risk of CIN (13, 14). For instance, Aktas et al. found that the TyG index was an independent predictor of CIN in 272 patients without diabetes who were undergoing PCI for non-ST elevation myocardial infarction (13). In another study of 350 patients without diabetes who were undergoing PCI for acute coronary syndrome, Gursoy and Baydar observed that the incidence of CIN was significantly higher in those with high TyG indices (≥8.65) than in those with lower indices (<8.65) (14). Importantly, the predictive value of the TyG index for CIN appears to be present even after adjusting for potential confounders such as diabetes status (13, 14). While promising, research on the TyG index and CIN risk remains limited. Only a few studies, mainly from China and Turkey, with relatively small sample sizes, have examined this association (13–17). Moreover, the diagnostic accuracy of the TyG index remains unclear. Further investigation through meta-analyses could help synthesize the current evidence regarding the utility of the TyG index as a predictor of CIN in patients undergoing PCI. Thus, in this diagnostic meta-analysis, we aimed to investigate whether the TyG index could be used to predict the likelihood of CIN in patients receiving PCI.
2 Methods
2.1 Protocol registration
This report was presented in compliance with the PRISMA guidelines and registered in PROSPERO (CRD42023452257).
2.2 Literature search
Potentially relevant articles were identified following searches of electronic databases such as MEDLINE, Cochrane Library, and EMBASE, spanning from their inception to August 3, 2023. The search was conducted using relevant keywords: (“PCI” or “Left Main Disease” or “Coronary Angiograph*” or “coronary artery disease” or “Percutaneous Coronary Intervention” or “Myocardial infarction” or “MI”) and (“Triglyceride–Glucose Index” or “Triglyceride–Glucose Indices” or “TyG” or “insulin resistance”), and (“CI-AKI” or “CA-AKI” or “Contrast-induced acute kidney injury” or “Contrast-Associated Acute Kidney Injury” or “Contrast-Induced Nephropathy” or “Contrast-Associated Nephropathy” or “Acute Renal Insufficiency” or “Acute Kidney Insufficiency” or “Renal Insufficienc*” or “Kidney insufficienc*” or “Acute kidney injury” or “AKI” or “Nephropathy”). Using a comprehensive approach, controlled vocabulary search terms were used alongside keyword searches to enhance the breadth of the literature exploration. Furthermore, the reference lists from the acquired studies were manually checked to find potentially suitable articles. The search was comprehensive and was not limited by country or language of publication. Supplementary Table 1 provides detailed information of the search strategies.
2.3 Inclusion and exclusion criteria
In this meta-analysis, the selection of studies for inclusion adhered to predefined criteria: (1) the studies encompassed patients with coronary disease who had undergone coronary angiography or PCI, (2) the studies employed the TyG index as a predictive measure for CIN risk, and (3) these studies furnished comprehensive data concerning sensitivity, specificity, and count of patients with CIN. Randomized controlled trials or retrospective study designs (e.g., case–control studies) were deemed suitable for this meta-analysis.
Studies were excluded if (1) they were exclusively presented as conference papers, case series, abstracts, or review articles; (2) they concentrating on outcomes other than CIN; and (3) they did not provide full-text versions.
2.4 Data retrieval
Two team members extracted information separately from the individual research papers, and any differences were resolved through discussion. To gather any missing details, efforts were made to communicate with the corresponding author of the studies. The extracted data for each study included the country of origin, author’s details, sample size, study setting, age, sex, information on specificity/sensitivity values, details related to the TyG index, and count of patients with CIN.
2.5 Outcomes
This diagnostic meta-analysis was conducted to appraise the precision of the TyG index in anticipating the probability of CIN, as per the definitions employed in individual studies. Secondary outcomes sought to appraise the combined incidence of CIN and the relationship between a high TyG index and the risk of CIN.
2.6 Quality assessment
The Quality Assessment for Diagnostic Accuracy Studies-2 (QUADAS-2) tool was used to assess the risk of bias (19). Four main domains including patient selection, index test, reference standard, and flow and timing were systematically examined to identify sources of bias. Two authors conducted a subjective review of all eligible studies to establish consistency. By using the QUADAS-2 tool, the authors assigned each domain to one of three categories: ‘low risk,’ ‘some concerns,’ or ‘high risk. Discussions were held to resolve discrepancies.
2.7 Statistical analysis
For all statistical analyses, Stata version 15.0 and RevMan version 5.4 were used. The sensitivity and specificity were extracted from each study and pooled. Heterogeneity was assessed using the I2 statistic, where an I2 >50% signifies significant heterogeneity. Publication bias was evaluated through Deeks’ funnel plot asymmetry test. To summarize the diagnostic accuracy, a hierarchical summary receiver operating characteristic curve was constructed, and the area under the curve (AUC) was calculated. Fagan’s nomogram was utilized to estimate post-test probability based on likelihood ratios (LRs) (20). A positive LR in the range of 2–5 slightly increase the likelihood of disease presence following the test, whereas ratios ranging from 5 to 10 moderately enhance the likelihood; ratios >10 significantly amplify the probability of having the disease (21). The predictive utility of the TyG index was assessed by examining how the post-test probability changed from the pre-test probability. Two-sided tests in all statistical analyses were conducted at a significance level of 0.05.
3 Results
3.1 Database searching and study characteristics
Through a search on four electronic databases, 890 records were discovered (Figure 1). By eliminating duplicate studies (n = 130) and carrying out initial title and abstract examination (n = 760), 15 articles emerged as potentially aligned with the predefined inclusion criteria. Subsequent full-text reading led to the exclusion of 10 studies. Ultimately, five studies met the predetermined requirements and were included in the meta-analysis (13–17).
The characteristics of studies involving 3518 patients are summarized in Table 1. All studies were conducted in two countries, including Turkey (n = 2) (13, 14) and China (n = 3) (15–17). In these studies, the age range of the patients fell between 57.6 and 68.22 years. The number of participants varied across the studies, ranging from 272 to 1108, with male proportions ranging from 52.3% to 79.4%. Among the five studies, two on patients without diabetes mellitus (DM) (13, 14), while two targeted patients with DM (15, 17). However, one study did not explicitly specify this information (16). Regarding the definition of CIN, three studies adopted the same criteria, defining CIN as either a 25% increase or a 0.5 mg/dL increase in serum creatinine (Scr) levels from baseline within the first 48–72 h (13, 14, 16). In another study, CIN was defined as a 50% increase or a ≥0.3 mg/dL increase in Scr levels from baseline within 1 week (15). However, one study did not specify the criteria used to define CIN (17). The AUC, which reflects the accuracy of the predictive models, ranged from 0.662 to 0.811. The sensitivity varied between 59.3% and 94.9%, while the specificity ranged between 31.7% and 72%. Four studies (13, 14, 16, 17) reported cutoff values to predict CIN occurrence, with the range being 8.69–9.17, while one study did not provide this information (15).
3.2 Risk of bias
The risk of bias and applicability concerns for the five included studies are shown in Figure 2. Overall, the majority of studies displayed a low risk of bias across the assessed domains. The primary areas of concern were related to the measurement of the TyG index in one study (15) and the definition of CIN in another (17). Apart from these issues, patient selection, index tests, reference standards, and flow/timing exhibited a low risk of bias in the included studies.
3.3 Outcomes
3.3.1 Pooled incidence of CIN
The incidence of CIN varied among studies, with the lowest being 6.6% in a study from Turkey (13), and the highest reaching 21.2% in a study conducted in China (17). After pooling data from a total of 3518 patients, the combined incidence of CIN was 15.3% (95% confidence interval [CI] 11% to 20.8%) (Figure 3).
3.3.2 Association between the TyG index and CIN risk
Five studies consistently demonstrated a positive relationship between the TyG index and CIN risk. Upon calculation, a high TyG index was associated with an increased risk of CIN, with an odds ratio of 2.25 (95% CI 1.82–2.77, p < 0.00001) (Figure 4). The level of heterogeneity across these studies was moderate (I2 = 35%).
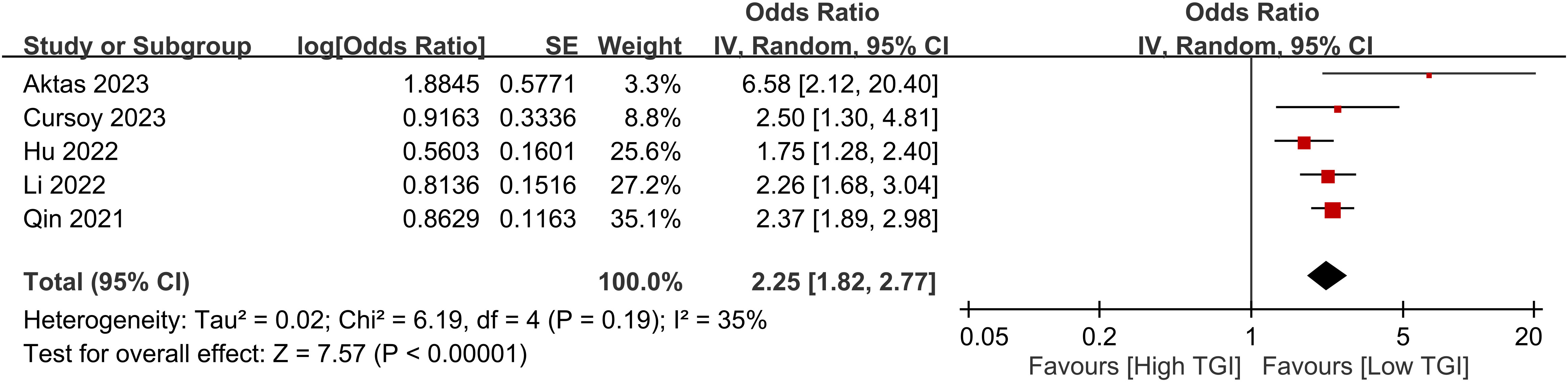
Figure 4 Forest plot showing a positive relationship between a high triglyceride–glucose (TyG) index and the risk of contrast-induced nephropathy.
3.3.3 Diagnostic efficacy of the TyG index for CIN
In relation to the diagnostic effectiveness of the TyG index, the combined sensitivity and specificity values were 0.77 (95% CI 0.59–0.88) and 0.55 (95% CI 0.43–0.68), respectively, accompanied by I2 values of 95.1% for sensitivity and 98.29% for specificity (Figure 5). The aggregated AUC was 0.69 (95% CI 0.65–0.73) (Figure 6). Fagan’s nomogram plot, presented in Figure 7, provides a visualization of post-test probabilities derived from the LRs. Specifically, an LR of 2 translates to a marginal increment in the probability of a positive outcome, while an LR of 0.42 signifies a minor reduction in the likelihood of a negative outcome. Notably, Deek’s funnel plot asymmetry test demonstrated a low susceptibility to publication bias (p = 0.81) (Figure 8).
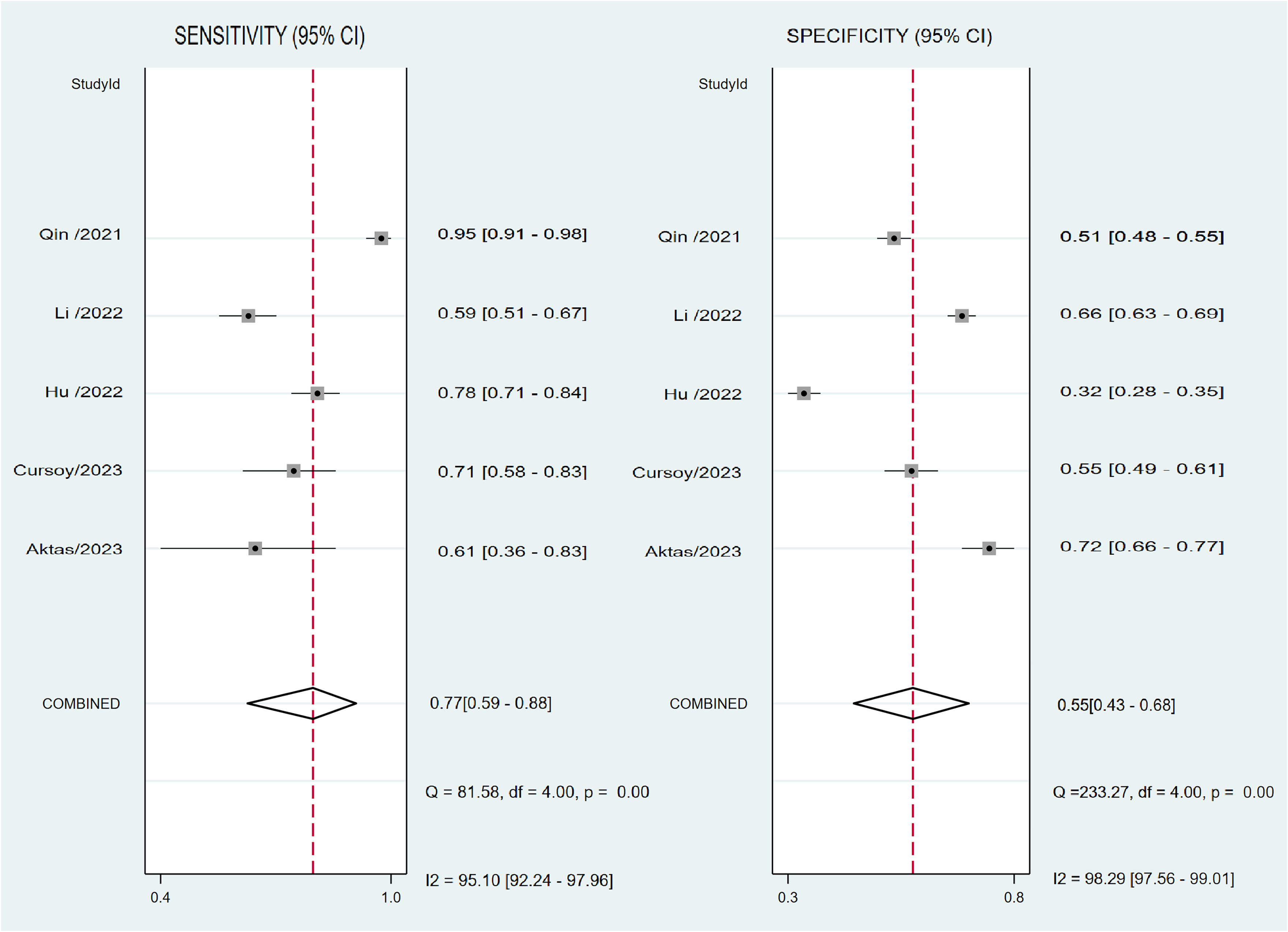
Figure 5 Forest plot depicting the combined sensitivity and specificity of the triglyceride–glucose (TyG) index in predicting contrast-induced nephropathy.
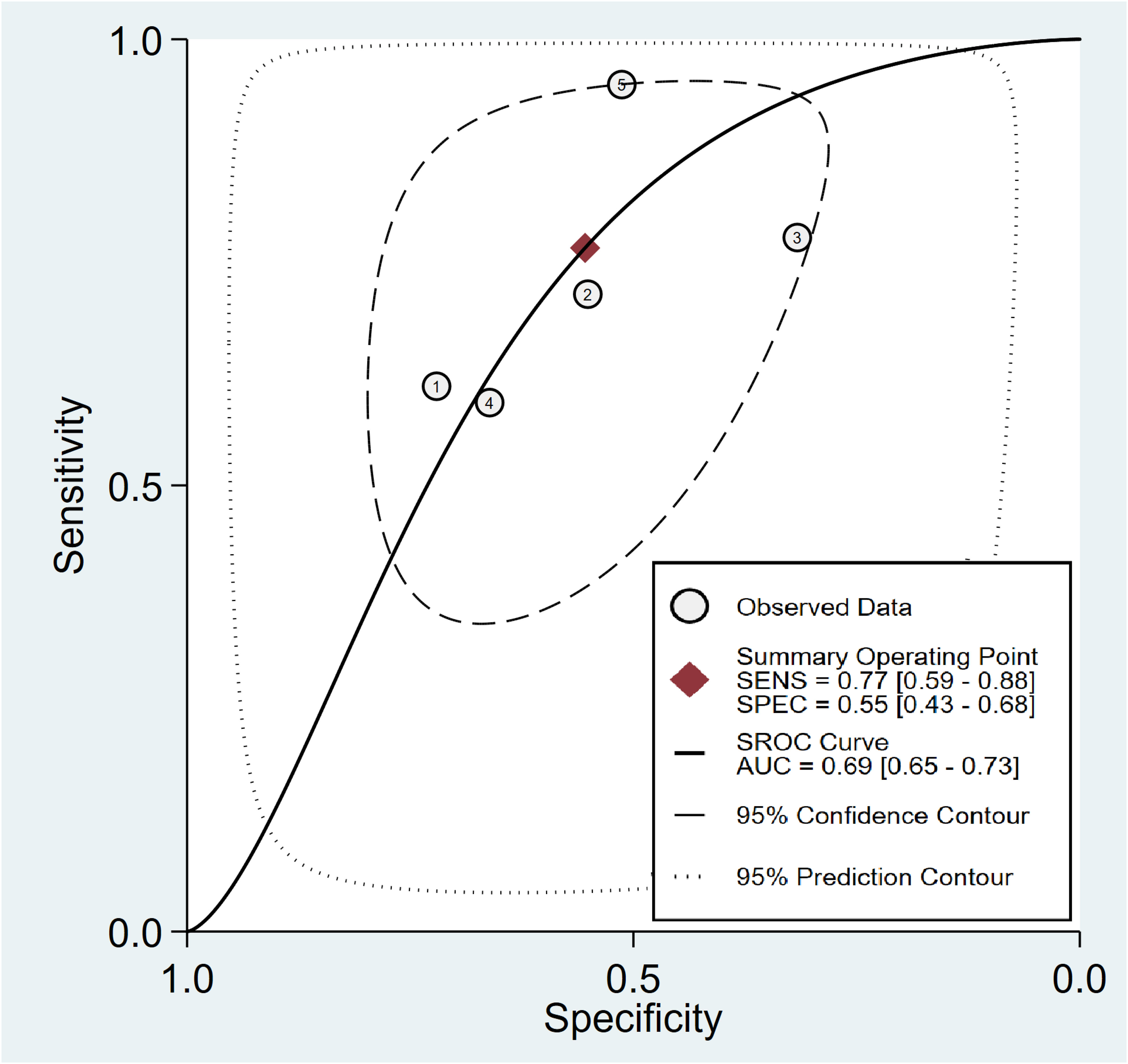
Figure 6 Analysis on summary receiver operating characteristic (sROC) curve demonstrating the predictive effectiveness of the triglyceride–glucose Index concerning contrast-induced nephropathy.
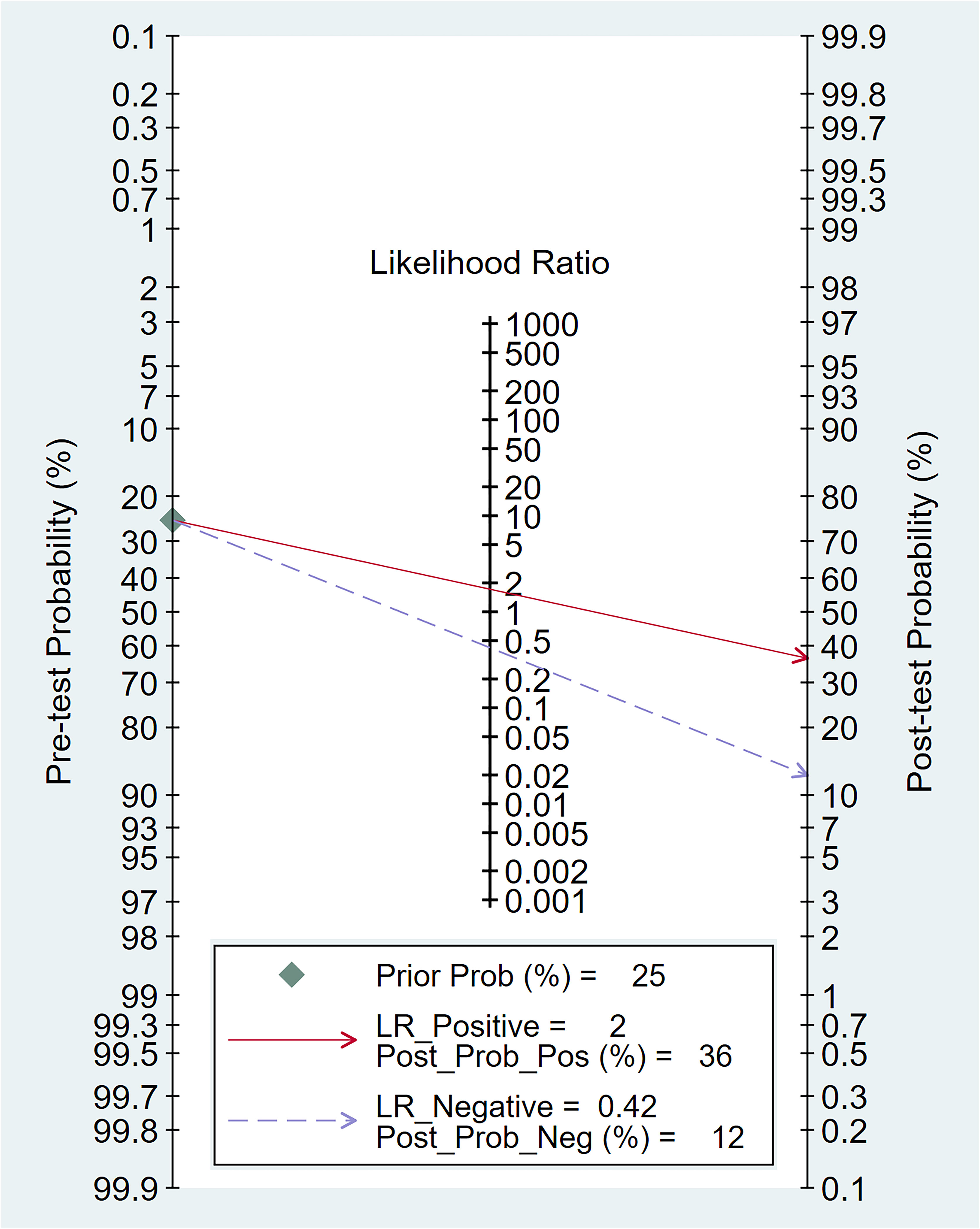
Figure 7 The clinical applicability of the triglyceride–glucose (TyG) index in forecasting the occurrence of contrast-induced nephropathy illustrated through Fagan’s nomogram plot.
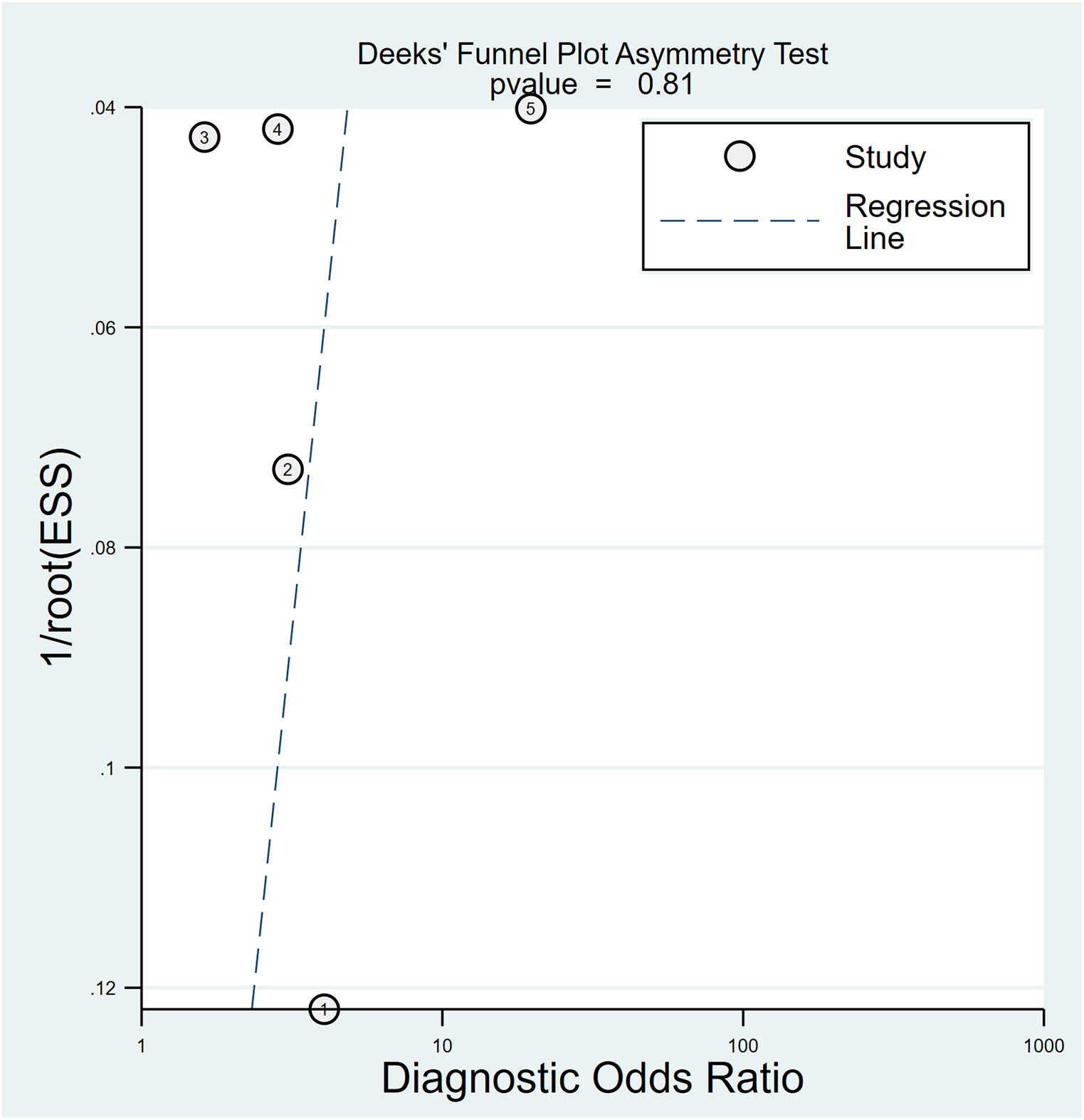
Figure 8 Utilizing Deek’s funnel plot asymmetry test, the probability of publication bias was deemed minimal (p = 0.81).
4 Discussion
On five studies involving 3518 patients with or without DM, patients with a high TyG index were prone to CIN than those with a low index. Regarding the diagnostic efficacy of TyG, the pooled AUC was 0.69, and the pooled sensitivity and specificity were 0.77 and 0.55, respectively. Although several risk factors correlated with CIN development, such as pre-existing kidney disease, diabetes, and heart failure (22–24), whether insulin resistance-associated index could predict CIN following PCI remains unknown. Hereby, this diagnostic meta-analysis highlighted that the TyG index could be a marker that reflects the risk of CIN development in patients receiving PCI.
To date, several risk scores have been established to predict CIN development. For instance, the Mehran contrast nephropathy risk score including hypotension, intra-aortic balloon pump (IABP), heart failure, chronic renal failure, diabetes, old age (>75 years), anemia, and contrast volume, was reported to sensitively predict CIN post-PCI (22). In another study of CIN in patients with ST-segment elevation myocardial infarction who received primary PCI, Koowattanatianchai et al. attempted to set up a simpler predictive model than the Mehran risk score (23). They found that three final predictors, which were ejection fraction of <40%, triple-vessel disease, and use of IABP were the most powerful predictors for the risk stratification of CIN (23). Upon reviewing 16 studies, including 12 prediction models, Silver et al. identified pre-existing chronic kidney disease, age, diabetes, heart failure or impaired ejection fraction, and shock as risk factors for CIN (24). In another meta-analysis, Yin et al. observed that the Maioli score had the best discrimination for CIN occurrence (25, 26). Nevertheless, most studies were based on single-center setting and lacked external validations (25). Thus, a comprehensive review including multiple institutes are necessary to evaluate and discover novel predictive markers for the risk stratification of CIN.
In a study of 1108 patients undergoing PCI for non-ST elevation acute coronary syndrome, the TyG index was identified as an independent predictor of CIN in the multivariate analysis, with a J-shaped association observed between the TyG index and the CIN risk after adjusting for confounders such as DM and kidney function (16). Our pooled meta-analysis also confirmed a significant association between the TyG index and CIN risk (odds ratio: 2.25; I2: 35%). However, our meta-analysis, which encompasses five studies with a total of 3518 patients, provides a more substantial evidence base compared to the single study involving 1108 patients (16). This larger pooled dataset enhances the generalizability of the observed association and allows for a more robust analysis of the TyG index’s predictive capacity for CIN. Compared with the TyG index, a recent meta-analysis of four studies involving 1346 patients found that gamma-glutamyl transferase (GGT) levels could potentially predict CIN in patients undergoing cardiac catheterization, showing a significantly higher GGT levels in patients with CIN (odds ratio: 3.21; I2:91.93%) (27). In our previous meta-analysis, patients with a low prognostic nutritional index (PNI) demonstrated a higher risk of CIN than those with a high PNI, with an odds ratio of 3.362 (I2 = 89.6%) (28). Although both GGT levels and PNI appear to have a stronger relationship with CIN than the TyG index, the relatively low heterogeneity in the current meta-analysis highlighted that the TyG index may be a reliable predictor of CIN.
Our meta-analysis found that the TyG index had the modest diagnostic accuracy based on the pooled sensitivity of 0.77 and specificity of 0.55. These results may be attributed to the complex, multifactorial mechanisms driving CIN, which are not yet fully elucidated. Proposed pathways for CIN include renal ischemia and hypoxia from altered hemodynamics, direct tubular cytotoxicity, and inflammation/oxidative stress (4, 29, 30). More specifically, contrast agents can reduce renal blood flow through the effects on vasoactive mediators such as nitric oxide and endothelin-1, leading to ischemic injury (4). Contrast media may directly induce tubular epithelial cell damage by increasing intracellular calcium levels, disrupting the mitochondria, and generating reactive oxygen species (29). In addition, the high osmolality and viscosity of some agents can impair tubular cell function and morphology (29). Finally, contrast-mediated CIN may involve free radical formation that damages cell membranes and activation of inflammatory and immunologic cascades (30). Consistently, the observed moderate diagnostic efficacy of the TyG index in predicting CIN might be attributed to the presence of multifactorial mechanisms contributing to CIN. Although the TyG index may not be used in isolation for screening, it may be a supplemental predictor to improve CIN detection.
In current meta-analysis, while studies adjusted for potential confounders such as diabetes and kidney function, other factors like contrast volume and hemodynamic instability were not consistently adjusted for across studies included. Failure to control for all potential mediating and moderating variables may weaken the observed TyG-CIN association. In addition, the heterogeneity between studies regarding patient characteristics, TyG cutoffs, and CIN definitions may have reduced the predictive value of the TyG index. Future studies should focus on optimally adjusting for possible confounders to elucidate the independent contribution of the TyG index to CIN occurrence.
Regarding the pathophysiological link between the TyG index and the risk of CIN, while our study did not directly investigate the underlying mechanisms, we can speculate based on existing literature. Metabolic syndrome, which is characterized by a cluster of conditions including insulin resistance, hypertension, dyslipidemia, and obesity, has been reported in several studies to be a stronger predictor of CIN (31, 32). Given that the TyG index is a recognized surrogate marker for insulin resistance and has been associated with metabolic syndrome (33, 34), it is plausible to consider the TyG index as a potential predictive marker for CIN.
The TyG index has garnered attention because of its potential clinical applications, particularly in the assessment of insulin resistance (9, 12). High TyG index levels are linked to reduced insulin sensitivity (9). This metric can be an initial screening tool for identifying individuals at risk of insulin resistance (9). Furthermore, the TyG index allows for monitoring alterations in insulin resistance and metabolic well-being in response to interventions such as lifestyle modification and medications (9, 12). A low TyG index could indicate enhanced insulin sensitivity and an improved metabolic status (35). The homeostasis model assessment of insulin resistance (HOMA-IR) is another commonly used marker of insulin resistance (36). However, the TyG index has some potential advantages over HOMA-IR. The TyG index requires only fasting glucose and triglyceride levels, which are routinely measured in clinical practice. In contrast, HOMA-IR calculation requires fasting insulin levels, which may not be routinely available. The simpler calculation and widespread availability of the components make the TyG index easily applicable. Furthermore, recent research highlights the TyG index as a superior alternative to HOMA-IR for predicting metabolic syndrome or type 2 diabetes, emphasizing its enhanced efficacy as a surrogate marker of insulin resistance (37, 38).
Several systematic reviews and meta-analyses have examined the relationship between the TyG index and cardiovascular diseases (39–41). A recent meta-analysis of seven studies, contrasting the highest-TyG group with the lowest-TyG group, revealed a notable rise in the risk of heart failure for the higher-TyG group (i.e., HR: 1.21) (39). Another meta-analysis reported that individuals with elevated TyG index levels face an increased risk of cardiovascular disease [i.e., odds ratio: 1.94], more pronounced coronary artery lesions [odds ratio: 3.49], and a less favorable prognosis than those with lower TyG index levels (40). These studies highlight the growing evidence linking the TyG index to increased risks of various cardiovascular diseases. In addition, studies have explored the potential link between the TyG index and nephropathy, particularly in the context of diabetic nephropathy (23, 25, 26). Thus, while the TyG index holds promise across various clinical applications, our meta-analysis suggested that it should not be solely relied upon as a diagnostic tool. Its interpretation should be complemented with other clinical data, including patient history, physical examinations, and supplementary laboratory tests.
Compared with other metabolic predictors, the TyG index had better diagnostic performance for predicting CIN compared with HbA1c, fasting glucose, or triglycerides alone based on the AUC analysis (16). However, the ideal approach for integrating the TyG index into CIN prediction remains unclear, as our meta-analysis relied on aggregate study-level data. A previous study found that adding TyG to the Mehran risk score increased its predictive accuracy from 62.3% to 71.2% and incorporating TyG improved risk reclassification compared with adding fasting blood glucose alone (16). This suggests that the TyG index may have incremental value when combined with established risk factors or prediction models.
This meta-analysis is the first to specifically assess and quantify the TyG index’s overall diagnostic accuracy in predicting the risk of CIN in patients undergoing PCI. While previous research has primarily concentrated on the TyG index’s relationship with diabetic nephropathy, our meta-analysis extends the scope by focusing on its utility in predicting CIN post-PCI. We provide pooled estimates for the sensitivity and specificity of the TyG index, offering a comprehensive view of its diagnostic performance as a predictor for CIN. This meta-analysis not only yields a more accurate assessment of the link between a heightened TyG index and the risk of CIN but also underscores the need for more extensive studies to determine optimal TyG thresholds and to confirm its predictive value across diverse populations.
This meta-analysis has several limitations. First, while showing promise, the evidence base remains limited to just a handful of studies conducted mainly in two countries. Larger scale and more geographically diverse studies are needed to confirm the association between the TyG index and CIN risk. Second, as our meta-analysis found significant heterogeneity between studies, optimal TyG index cutoff values for predicting CIN risk may differ based on patient population characteristics. Careful calibration is necessary before applying proposed cutoffs such as 8.69–9.17 from these studies. Third, this study only investigated the predictive utility of baseline or pre-procedural TyG index. Changes in the TyG index during hospitalization may also correlate with CIN but were not examined here. Finally, the lack of individual patient data precluded more detailed assessment of how the TyG index could enhance existing CIN prediction models. Future pooled analyses should focus on optimally incorporating the TyG index into the risk stratification.
5 Conclusion
The results of this meta-analysis showed that patients who received PCI with a higher TyG index were at an increased risk to develop CIN than those with a low index. In addition to the conventional risk factors, the TyG index may be used as an additional predictor of CIN. Nevertheless, CIN can stem from various factors. Therefore, clinical practitioners must take into account all potential risks holistically and evaluate each patient’s situation individually. In addition, the small number of studies identified makes the results preliminary and precludes definitive conclusions. Larger studies are needed to validate the utility of the TyG index and determine appropriate cutoffs.
Data availability statement
The original contributions presented in the study are included in the article/Supplementary Material. Further inquiries can be directed to the corresponding author.
Author contributions
W-TC: Conceptualization, Writing – original draft, Writing – review & editing. C-CL: Conceptualization, Investigation, Writing – original draft. Y-TH: Data curation, Formal Analysis, Investigation, Software, Writing – original draft. J-YW: Formal Analysis, Methodology, Software, Writing – original draft. W-WT: Investigation, Methodology, Resources, Writing – original draft. K-CH: Writing – original draft, Writing – review & editing. I-WC: Supervision, Writing – original draft, Writing – review & editing. P-HF: Supervision, Writing – original draft, Writing – review & editing.
Funding
The author(s) declare financial support was received for the research, authorship, and/or publication of this article. This research was funded by Chi Mei Medical Center, Tainan, Taiwan, grant number CMOR11203. This work was supported by Chi Mei Medical Center, Liouying branch.
Conflict of interest
The authors declare that the research was conducted in the absence of any commercial or financial relationships that could be construed as a potential conflict of interest.
Publisher’s note
All claims expressed in this article are solely those of the authors and do not necessarily represent those of their affiliated organizations, or those of the publisher, the editors and the reviewers. Any product that may be evaluated in this article, or claim that may be made by its manufacturer, is not guaranteed or endorsed by the publisher.
Supplementary material
The Supplementary Material for this article can be found online at: https://www.frontiersin.org/articles/10.3389/fendo.2023.1282675/full#supplementary-material
References
1. Basta G, Chatzianagnostou K, Paradossi U, Botto N, Del Turco S, Taddei A, et al. The prognostic impact of objective nutritional indices in elderly patients with ST-elevation myocardial infarction undergoing primary coronary intervention. Int J Cardiol (2016) 221:987–92. doi: 10.1016/j.ijcard.2016.07.039
2. Kusirisin P, Chattipakorn SC, Chattipakorn N. Contrast-induced nephropathy and oxidative stress: mechanistic insights for better interventional approaches. J Transl Med (2020) 18:400. doi: 10.1186/s12967-020-02574-8
3. Wi J, Ko YG, Kim JS, Kim BK, Choi D, Ha JW, et al. Impact of contrast-induced acute kidney injury with transient or persistent renal dysfunction on long-term outcomes of patients with acute myocardial infarction undergoing percutaneous coronary intervention. Heart (2011) 97:1753–7. doi: 10.1136/hrt.2010.218677
4. McCullough PA, Choi JP, Feghali GA, Schussler JM, Stoler RM, Vallabahn RC, et al. Contrast-induced acute kidney injury. J Am Coll Cardiol (2016) 68:1465–73. doi: 10.1016/j.jacc.2016.05.099
5. James MT, Ghali WA, Tonelli M, Faris P, Knudtson ML, Pannu N, et al. Acute kidney injury following coronary angiography is associated with a long-term decline in kidney function. Kidney Int (2010) 78:803–9. doi: 10.1038/ki.2010.258
6. Ebert N, Schneider A, Huscher D, Mielke N, Balabanova Y, Brobert G, et al. Incidence of hospital-acquired acute kidney injury and trajectories of glomerular filtration rate in older adults. BMC Nephrol (2023) 24:226. doi: 10.1186/s12882-023-03272-5
7. Sun G, Chen P, Wang K, Li H, Chen S, Liu J, et al. Contrast-induced nephropathy and long-term mortality after percutaneous coronary intervention in patients with acute myocardial infarction. Angiology (2019) 70:621–6. doi: 10.1177/0003319718803677
8. Wu X, Ma C, Sun D, Zhang G, Wang J, Zhang E. Inflammatory indicators and hematological indices in contrast-induced nephropathy among patients receiving coronary intervention: A systematic review and meta-analysis. Angiology (2021) 72:867–77. doi: 10.1177/00033197211000492
9. Anto EO, Frimpong J, Boadu WIO, Korsah EE, Tamakloe V, Ansah E, et al. Cardiometabolic syndrome among general adult population in Ghana: The role of lipid accumulation product, waist circumference-triglyceride index, and triglyceride-glucose index as surrogate indicators. Health Sci Rep (2023) 6:e1419. doi: 10.1002/hsr2.1419
10. Boshen Y, Yuankang Z, Xinjie Z, Taixi L, Kaifan N, Zhixiang W, et al. Triglyceride-glucose index is associated with the occurrence and prognosis of cardiac arrest: a multicenter retrospective observational study. Cardiovasc Diabetol (2023) 22:190. doi: 10.1186/s12933-023-01918-0
11. Guerrero-Romero F, Simental-Mendía LE, González-Ortiz M, Martínez-Abundis E, Ramos-Zavala MG, Hernández-González SO, et al. The product of triglycerides and glucose, a simple measure of insulin sensitivity. Comparison with the euglycemic-hyperinsulinemic clamp. J Clin Endocrinol Metab (2010) 95:3347–51. doi: 10.1210/jc.2010-0288
12. Cai XL, Xiang YF, Chen XF, Lin XQ, Lin BT, Zhou GY, et al. Prognostic value of triglyceride glucose index in population at high cardiovascular disease risk. Cardiovasc Diabetol (2023) 22:198. doi: 10.1186/s12933-023-01924-2
13. Aktas H, Inci S, Gul M, Gencer S, Yildirim O. Increased triglyceride-glucose index predicts contrast-induced nephropathy in non-diabetic NSTEMI patients: A prospective study. J Investig Med (2023) 71(8):838–44. doi: 10.1177/10815589231182317
14. Gursoy E, Baydar O. The triglyceride-glucose index and contrast-induced nephropathy in non-ST elevation myocardial infarction patients undergoing percutaneous coronary intervention. Med (Baltimore) (2023) 102:e32629. doi: 10.1097/MD.0000000000032629
15. Hu Y, Wang X, Xiao S, Sun N, Huan C, Wu H, et al. A clinical nomogram based on the triglyceride-glucose index to predict contrast-induced acute kidney injury after percutaneous intervention in patients with acute coronary syndrome with diabetes mellitus. Cardiovasc Ther (2022) 2022:5443880. doi: 10.1155/2022/5443880
16. Li M, Li L, Qin Y, Luo E, Wang D, Qiao Y, et al. Elevated TyG index predicts incidence of contrast-induced nephropathy: A retrospective cohort study in NSTE-ACS patients implanted with DESs. Front Endocrinol (Lausanne) (2022) 13:817176. doi: 10.3389/fendo.2022.817176
17. Qin Y, Tang H, Yan G, Wang D, Qiao Y, Luo E, et al. A high triglyceride-glucose index is associated with contrast-induced acute kidney injury in Chinese patients with type 2 diabetes mellitus. Front Endocrinol (Lausanne) (2020) 11:522883. doi: 10.3389/fendo.2020.522883
18. Lin HY, Zhang XJ, Liu YM, Geng LY, Guan LY, Li XH. Comparison of the triglyceride glucose index and blood leukocyte indices as predictors of metabolic syndrome in healthy Chinese population. Sci Rep (2021) 11:10036. doi: 10.1038/s41598-021-89494-9
19. Whiting PF, Rutjes AW, Westwood ME, Mallett S, Deeks JJ, Reitsma JB, et al. QUADAS-2: a revised tool for the quality assessment of diagnostic accuracy studies. Ann Internal Med (2011) 155:529–36. doi: 10.7326/0003-4819-155-8-201110180-00009
20. Tsai WW, Hung KC, Huang YT, Yu CH, Lin CH, Chen IW, et al. Diagnostic efficacy of sonographic measurement of laryngeal air column width difference for predicting the risk of post-extubation stridor: A meta-analysis of observational studies. Front Med (2023) 10:1109681. doi: 10.3389/fmed.2023.1109681
21. Grimes DA, Schulz KF. Refining clinical diagnosis with likelihood ratios. Lancet (London England) (2005) 365:1500–5. doi: 10.1016/S0140-6736(05)66422-7
22. Mehran R, Aymong ED, Nikolsky E, Lasic Z, Iakovou I, Fahy M, et al. A simple risk score for prediction of contrast-induced nephropathy after percutaneous coronary intervention: development and initial validation. J Am Coll Cardiol (2004) 44:1393–9. doi: 10.1016/j.jacc.2004.06.068
23. Koowattanatianchai S, Chantadansuwan T, Kaladee A, Phinyo P, Patumanond J. Practical risk stratification score for prediction of contrast-induced nephropathy after primary percutaneous coronary intervention in patients with acute ST-segment elevation myocardial infarction. Cardiol Res (2019) 10:350–7. doi: 10.14740/cr939
24. Silver SA, Shah PM, Chertow GM, Harel S, Wald R, Harel Z. Risk prediction models for contrast induced nephropathy: systematic review. BMJ (2015) 351:h4395. doi: 10.1136/bmj.h4395
25. Yin W, Zhou G, Zhou L, Liu M, Xie Y, Wang J, et al. Validation of pre-operative risk scores of contrast-induced acute kidney injury in a Chinese cohort. BMC Nephrol (2020) 21:45. doi: 10.1186/s12882-020-1700-8
26. Maioli M, Toso A, Gallopin M, Leoncini M, Tedeschi D, Micheletti C, et al. Preprocedural score for risk of contrast-induced nephropathy in elective coronary angiography and intervention. J Cardiovasc Med (Hagerstown) (2010) 11:444–9. doi: 10.2459/JCM.0b013e328335227c
27. Javid M, Mirdamadi A, Javid M, Amini-Salehi E, Vakilpour A, Keivanlou MH, et al. Gamma glutamyl transferase as a biomarker to predict contrast-induced nephropathy among patients with acute coronary syndrome undergoing coronary interventions: a meta-analysis. Ann Med Surg (2023) 85:4033–40. doi: 10.1097/MS9.0000000000000967
28. Chang WT, Sun CK, Wu JY, Huang PY, Liu TH, Chang YJ, et al. Association of prognostic nutritional index with risk of contrast induced nephropathy: A meta-analysis. Front Nutr (2023) 10:1154409. doi: 10.3389/fnut.2023.1154409
29. Andreucci M, Faga T, Pisani A, Sabbatini M, Michael A. Acute kidney injury by radiographic contrast media: pathogenesis and prevention. BioMed Res Int (2014) 2014:362725. doi: 10.1155/2014/362725
30. Romano G, Briguori C, Quintavalle C, Zanca C, Rivera NV, Colombo A, et al. Contrast agents and renal cell apoptosis. Eur Heart J (2008) 29:2569–76. doi: 10.1093/eurheartj/ehn197
31. Amiri A, Ghanavati R, Riahi Beni H, Sezavar SH, Sheykhvatan M, Arab M. Metabolic syndrome and the iodine-dose/creatinine clearance ratio as determinants of contrast-induced acute kidney injury. Cardiorenal Med (2018) 8:217–27. doi: 10.1159/000488374
32. Ozcan OU, Adanir Er H, Gulec S, Ustun EE, Gerede DM, Goksuluk H, et al. Impact of metabolic syndrome on development of contrast-induced nephropathy after elective percutaneous coronary intervention among nondiabetic patients. Clin Cardiol (2015) 38:150–6. doi: 10.1002/clc.22364
33. Couto AN, Pohl HH, Bauer ME, Schwanke CHA. Accuracy of the triglyceride-glucose index as a surrogate marker for identifying metabolic syndrome in non-diabetic individuals. Nutr (Burbank Los Angeles County Calif) (2023) 109:111978. doi: 10.1016/j.nut.2023.111978
34. Raimi TH, Dele-Ojo BF, Dada SA, Fadare JO, Ajayi DD, Ajayi EA, et al. Triglyceride-glucose index and related parameters predicted metabolic syndrome in Nigerians. Metab syndrome related Disord (2021) 19:76–82. doi: 10.1089/met.2020.0092
35. Sanchez-Garcia A, Rodriguez-Gutierrez R, Mancillas-Adame L, Gonzalez-Nava V, Diaz Gonzalez-Colmenero A, Solis RC, et al. Diagnostic accuracy of the triglyceride and glucose index for insulin resistance: A systematic review. Int J Endocrinol (2020) 2020:4678526. doi: 10.1155/2020/4678526
36. Matthews DR, Hosker JP, Rudenski AS, Naylor BA, Treacher DF, Turner RC. Homeostasis model assessment: insulin resistance and beta-cell function from fasting plasma glucose and insulin concentrations in man. Diabetologia (1985) 28:412–9. doi: 10.1007/BF00280883
37. Park HM, Lee HS, Lee YJ, Lee JH. The triglyceride-glucose index is a more powerful surrogate marker for predicting the prevalence and incidence of type 2 diabetes mellitus than the homeostatic model assessment of insulin resistance. Diabetes Res Clin practice (2021) 180:109042. doi: 10.1016/j.diabres.2021.109042
38. Son DH, Lee HS, Lee YJ, Lee JH, Han JH. Comparison of triglyceride-glucose index and HOMA-IR for predicting prevalence and incidence of metabolic syndrome. Nutrition metabolism Cardiovasc diseases: NMCD. (2022) 32:596–604. doi: 10.1016/j.numecd.2021.11.017
39. Khalaji A, Behnoush AH, Khanmohammadi S, Ghanbari Mardasi K, Sharifkashani S, Sahebkar A, et al. Triglyceride-glucose index and heart failure: a systematic review and meta-analysis. Cardiovasc Diabetol (2023) 22:244. doi: 10.1186/s12933-023-01973-7
40. Liang S, Wang C, Zhang J, Liu Z, Bai Y, Chen Z, et al. Triglyceride-glucose index and coronary artery disease: a systematic review and meta-analysis of risk, severity, and prognosis. Cardiovasc Diabetol (2023) 22:170. doi: 10.1186/s12933-023-01906-4
Keywords: contrast-induced nephropathy, triglyceride-glucose index, meta-analysis, insulin resistance, cardiovascular disease
Citation: Chang W−T, Liu C-C, Huang Y-T, Wu J-Y, Tsai W-W, Hung K−C, Chen I−W and Feng P-H (2023) Diagnostic efficacy of the triglyceride–glucose index in the prediction of contrast-induced nephropathy following percutaneous coronary intervention. Front. Endocrinol. 14:1282675. doi: 10.3389/fendo.2023.1282675
Received: 24 August 2023; Accepted: 09 November 2023;
Published: 22 November 2023.
Edited by:
Seerapani Gopaluni, University of Cambridge, United KingdomReviewed by:
Amirmohammad Khalaji, Tehran University of Medical Sciences, IranAmir Hossein Behnoush, Tehran University of Medical Sciences, Iran
Copyright © 2023 Chang, Liu, Huang, Wu, Tsai, Hung, Chen and Feng. This is an open-access article distributed under the terms of the Creative Commons Attribution License (CC BY). The use, distribution or reproduction in other forums is permitted, provided the original author(s) and the copyright owner(s) are credited and that the original publication in this journal is cited, in accordance with accepted academic practice. No use, distribution or reproduction is permitted which does not comply with these terms.
*Correspondence: Ping-Hsun Feng, ZmVuZ2JlbnNvbkBnbWFpbC5jb20=
†These authors have contributed equally to this work