- 1Department of Ultrasound, Changsha Hospital for Maternal & Child Health Care Affiliated to Hunan Normal University, Changsha, China
- 2State Key Laboratory of Oncology in South China, Collaborative Innovation Center for Cancer Medicine, Sun Yat-sen University Cancer Center, Guangzhou, China
- 3Institute of Clinical Medicine, The First Affiliated Hospital of University of South, Hengyang, Hunan, China
- 4Department of Ultrasound, The People’s Hospital of Guangxi Zhuang Autonomous Region, Nanning, China
Background: Previous models for differentiating benign and malignant thyroid nodules(TN) have predominantly focused on the characteristics of the nodules themselves, without considering the specific features of the thyroid gland(TG) in patients with Hashimoto’s thyroiditis(HT). In this study, we analyzed the clinical and ultrasound radiomics(USR) features of TN in patients with HT and constructed a model for differentiating benign and malignant nodules specifically in this population.
Methods: We retrospectively collected clinical and ultrasound data from 227 patients with TN and concomitant HT(161 for training, 66 for testing). Two experienced sonographers delineated the TG and TN regions, and USR features were extracted using Python. Lasso regression and logistic analysis were employed to select relevant USR features and clinical data to construct the model for differentiating benign and malignant TN. The performance of the model was evaluated using area under the curve(AUC), calibration curves, and decision curve analysis(DCA).
Results: A total of 1,162 USR features were extracted from TN and the TG in the 227 patients with HT. Lasso regression identified 14 features, which were used to construct the TN score, TG score, and TN+TG score. Univariate analysis identified six clinical predictors: TI-RADS, echoic type, aspect ratio, boundary, calcification, and thyroid function. Multivariable analysis revealed that incorporating USR scores improved the performance of the model for differentiating benign and malignant TN in patients with HT. Specifically, the TN+TG score resulted in the highest increase in AUC(from 0.83 to 0.94) in the clinical prediction model. Calibration curves and DCA demonstrated higher accuracy and net benefit for the TN+TG+clinical model.
Conclusion: USR features of both the TG and TN can be utilized for differentiating benign and malignant TN in patients with HT. These findings highlight the importance of considering the entire TG in the evaluation of TN in HT patients, providing valuable insights for clinical decision-making in this population.
Summary
Previous models for differentiating benign and malignant thyroid nodules(TN) have predominantly focused on the characteristics of the nodules themselves, without considering the specific features of the thyroid gland(TG) in patients with Hashimoto’s thyroiditis(HT). it is worth further investigating whether US features of TN and TG play an important role in the benign-malignant discrimination of TN in patients with HT. In this study, clinical and US data were retrospectively collected from 227 patients with HT accompanied by TN. A total of 1,162 USR features were extracted from TN and the TG in the 227 patients with HT. Lasso regression identified 14 features, which were used to construct the TN score, TG score, and TN+TG score. Multivariable analysis revealed that incorporating USR scores improved the performance of the model for differentiating benign and malignant TN in patients with HT. Specifically, the TN+TG score resulted in the highest increase in AUC(from 0.83 to 0.94) in the clinical prediction model. Calibration curves and DCA demonstrated higher accuracy and net benefit for the TN+TG+clinical model. In conclusion, USR features of both the TG and TN can be utilized for differentiating benign and malignant TN in patients with HT.
1 Introduction
Hashimoto’s thyroiditis (HT), an autoimmune disease, is the most common cause of hypothyroidism, characterized by diffuse lymphocytic infiltration and progressive autoimmune reactions leading to chronic inflammation and thyroid dysfunction (1, 2). On the other hand, thyroid cancer (TC) is the most common malignancy of the endocrine system, with rapidly increasing incidence rates globally, ranging from 4.5% to 6.6% per year (3, 4). Thyroid nodules (TN) are a common presentation of TC, but TN are not always malignant (5). Differentiating between benign and malignant TN is crucial for detecting TC, which has significant implications for guiding treatment decisions, improving patients’ quality of life, and optimizing healthcare resources (6, 7). Numerous etiological and epidemiological studies have indicated a higher coexistence rate of HT and TC, estimated at approximately 23% (ranging from 10% to 58%) (8). However, the current assessment systems used to distinguish between benign and malignant conditions often overlook the impact of HT on TN, which could lead to a lower detection rate of TC in HT patients.
Ultrasound (US) is widely used in the evaluation of TN because it is a non-invasive and radiation-free imaging technique that provides detailed structural information (9, 10). The American College of Radiology Thyroid Imaging Reporting and Data System (ACR TI-RADS) is currently the most commonly used tool in clinical practice for risk stratification of TN. This system encompasses five ultrasound features, including composition, echogenicity, shape, margins, and echogenic foci (11). It has been reported that ACR TI-RADS has a sensitivity of approximately 88% and specificity of around 49%. However, some malignant TNs exhibit benign features in ultrasound images, such as smooth margins and absence of calcification. Therefore, the evaluation value of ACR TI-RADS for these types of TNs is limited (12). To improve the accuracy of US diagnosis of TN, researchers are constantly exploring new image features and classification algorithms (13). For example, Zhao et al. proposed a local and global feature disentanglement network to classify the benign and malignant nature of thyroid nodules, achieving an accuracy of 89.33% (14). Recently, radiomics based on US image analysis has shown superior performance compared to other conventional methods (15). Radiomics can automatically extract a large number of quantitative image features from medical images, which are often difficult to identify by the naked eye (16, 17). Radiomics can provide complementary information to image features and, in combination with clinical information and US image features, improve model performance (18–20). Zheng et al., for instance, demonstrated the application of ultrasound radiomics (USR) to build a predictive model for better predicting the status of axillary lymph node metastasis in early-stage breast cancer patients prior to surgery (18).
HT and TN may be associated in certain cases. The chronic inflammation caused by HT can result in thyroid tissue damage and progressive structural changes, which may contribute to the formation of nodules (21). US imaging of HT presents with several unique features, including abnormal echogenicity patterns, abnormal blood flow signals, and diffuse changes (22). Previous studies on US features for benign-malignant discrimination of TN have primarily focused on the nodules themselves, while overlooking the US features of the thyroid gland (TG) which may indicate the differences between benign and malignant nodules (23–26). Jin et al. also reported that predictive models based on US features of TC and TG could effectively predict central lymph node metastasis (27). Therefore, it is worth further investigating whether US features of TN and TG play an important role in the benign-malignant discrimination of TN in patients with HT.
In this study, clinical and US data were retrospectively collected from 227 patients with HT accompanied by TN. By outlining the target areas and extracting US features of the TG and TN, we constructed a specific diagnostic model for TN benign-malignant discrimination, taking into account the patients’ clinical information.
2 Method
2.1 Patient selection
The study was conducted in accordance with the Declaration of Helsinki (as revised in 2013). From January 2012 to December 2022, we retrospectively collected 5,478 patients with TN from Changsha Hospital for Maternal & Child Health Care Affiliated to Hunan Normal University and People’s Hospital of Guangxi Zhuang Autonomous Region. The inclusion criteria are as follows: (1) TN Patients have HT. (2)All patients have undergone thyroid surgery and have tissue pathology results. (3) The diagnosis of HT and the benign or malignant nature of TN were confirmed by post-operative pathological examination. (4)ACR TI-RADS score≥4. The exclusion criteria are as follows: (1) Patients with two or more TN. (2) Lacking complete clinical data and high-quality US images. (3) Lacking pathological data for the diagnosis of TN and HT. Finally, there were 227 patients enrolled in this study.
2.2 Data collection
All included patients in this study had their clinical data collected, including preoperative basic clinical information, conventional ultrasound results, thyroid function indicators, and other serological markers. The basic clinical information comprised age, gender, BMI, tumor size (long diameter), and location. The conventional ultrasound results were assessed by experienced sonographers, and the obtained features included echoic type (iso/hyper/hypo/marked hypo echoic), margin (well/ill defined), calcification (NO/macro/micro calcification), vascularity (NO/low/median/high). Thyroid function indicators encompassed total triiodothyronine (TT3), free triiodothyronine (FT3), total tetraiodothyronine (TT4), free tetraiodothyronine (FT4), thyroid stimulating hormone (TSH), parathyroid hormone (PTH), anti-thyroid peroxidase (anti-TPO), thyroid globulin, anti-thyroid globulin (anti-TG) and calcitonin. Other serological markers primarily reflected inflammation and nutritional status, such as neutrophil count, lymphocyte count, platelet count, calcium levels, lactate dehydrogenase, albumin, and others.
2.3 Segmentation and feature extraction of US
In this study, preoperative ultrasound data in DICOM format were collected from patients. After excluding low-quality data, the high-quality ultrasound data were imported into ITK-SNAP software (Version 3.8). Segmentation of the regions of interest (ROIs) was performed using a double-blind method, with two experienced ultrasound specialists independently delineating the ROIs. The delineated target areas were compared by the two ultrasound specialists, and any discrepancies in the regions were adjusted. In cases of disagreement, a third physician provided confirmation. The ROIs delineation included two parts: TN and TG. The delineated target areas were saved in NIFF format. Finally, radiomics data were extracted using the Python package pyradiomics (V1.3.0), and a total of 1,162 USR features were extracted from the thyroid (531 from TN and 531 from TG).
2.4 USR feature selection and model establishment
The ROIs from the TG and TN were analyzed together. To identify the most relevant and significant features, we employed statistical methods such as independent t-test and least absolute shrinkage and selection operator (LASSO) regression. These methods helped us select a subset of features that had the strongest correlation with the target variable, and we calculated USR scores using regression techniques. Besides, logistic regression analysis was used to conduct univariate analysis on clinical and serum markers, and markers significantly associated with malignant nodule were included in the multivariate analysis. We combined the USR scores with clinically significant information, thyroid function indicators, and serum markers to perform a comprehensive multivariable analysis and establish multiple predictive models for malignant nodule.
2.5 Statistical analysis
All statistical analyses were performed using R software (Version 4.1.3). Continuous variables were reported as medians and interquartile ranges (IQRs), and categorical variables as frequencies and percentages. The Wilcoxon signed-rank test was used in two sets of related samples. Logistic regression analysis was used to build the lymph node prediction model and calculate the odds ratios (ORs) with relative 95% confidence intervals (95%CI) to determine the relevance of all potential predictors. In logistic regression analysis, univariate analysis was first conducted to screen for statistically significant predictive factors, and then statistically significant predictors were included in the multivariable model. In the ROC curve, the area under the curve (AUC) was used to evaluate the differences between different models. Thousand bootstrap resamples were used to internal validation of novel diagnostic models. Decision curve analysis (DCA) was performed to determine the net benefit associated with the models (28). The discrimination and DCA were corrected for overfitting using leave-one-out cross-validation. All tests were two-tailed and p<0.05 was considered statistically significant.
3 Results
In this study, a total of 5,478 patients with TN who underwent US examination were reviewed. Patients without HT and those with a TI-RADS score less than 3 were excluded, resulting in 956 patients with HT and TN. Further screening based on pathological results, presence of multiple nodules, ultrasound image quality, and completeness of clinical data excluded 729 patients. Finally, there was a sample size of 227 patients for inclusion including 161 patients for training and 66 patients for testing (Figure 1).
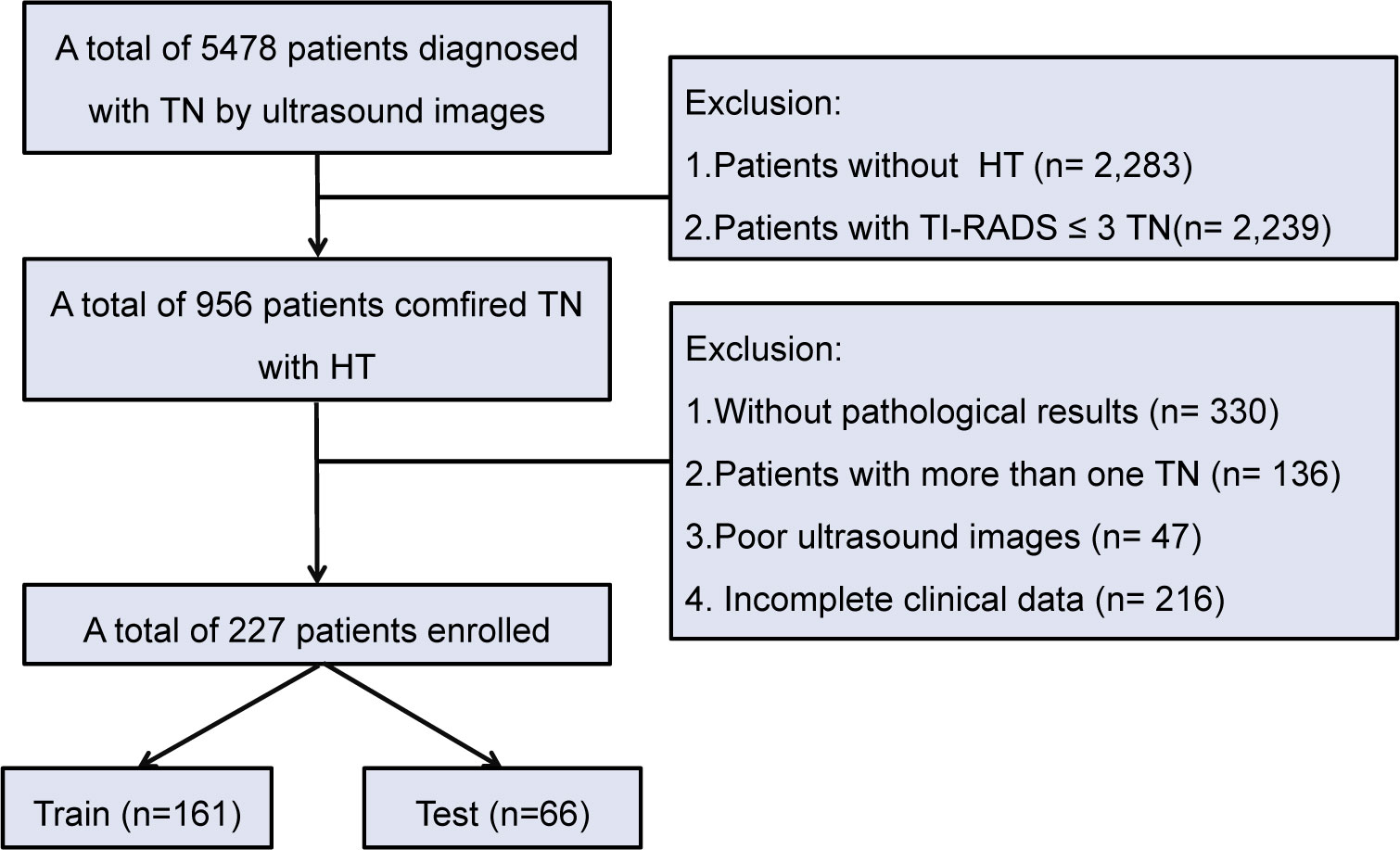
Figure 1 Flowchart of patient selection for TN patients with HT. TN, thyroid nodule; HT, Hashimoto’s thyroiditis.
As shown in Figure 2, we delineated the target regions of TN (highlighted in red) and the TG (highlighted in blue) on US images for the 227 patients. A total of 1,162 USR features were extracted from both the ROIs of TN and the TG using Python. By applying LASSO regression, we ultimately identified 14 USR features (4 from TG and 9 from TN) for distinguishing benign and malignant TN. Based on these 14 USR features, we use logistics analysis to construct the TN+TG score, TN score, and TG score, respectively.
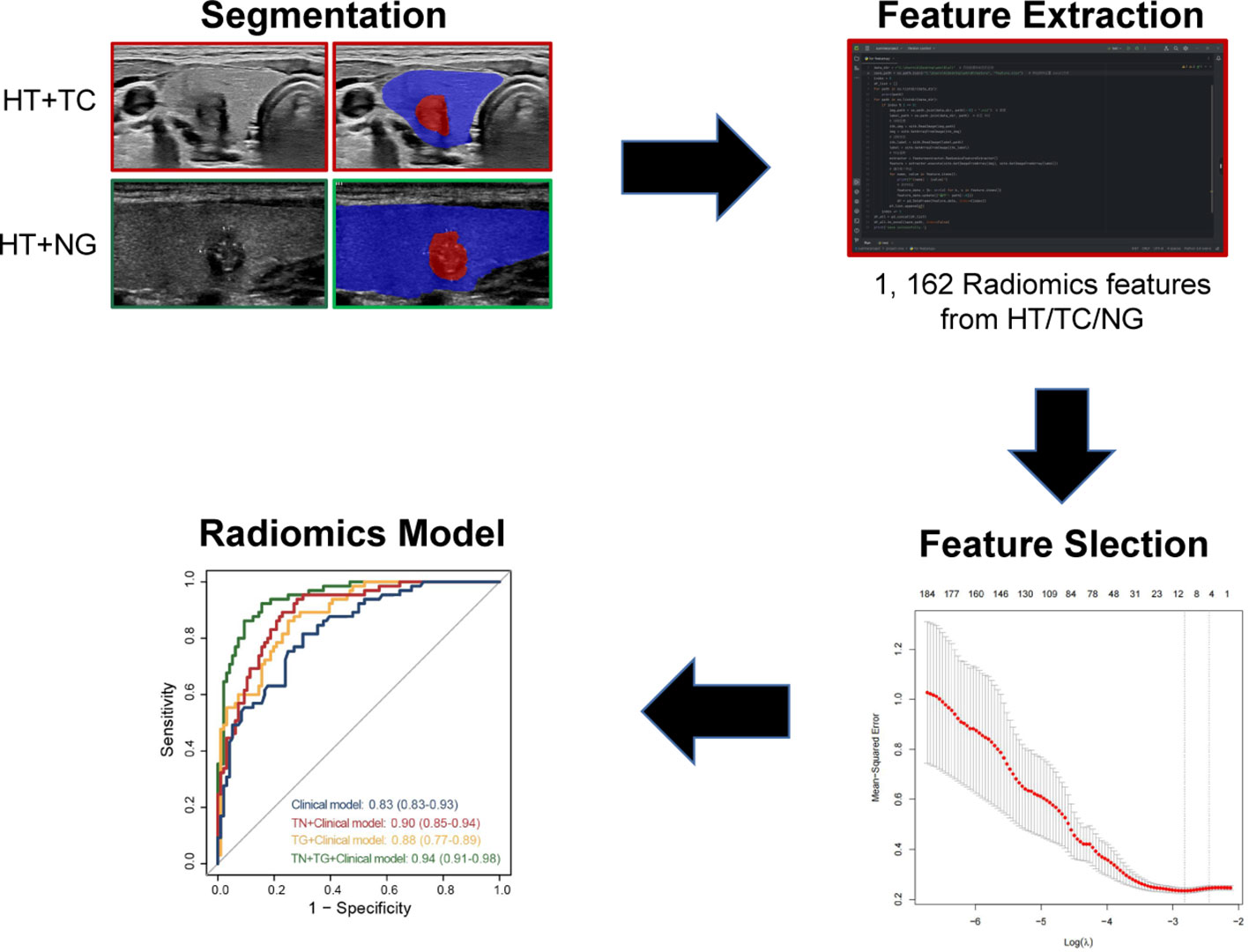
Figure 2 Flowchart of development of radiomics model for TN patients with HT. TN, thyroid nodule; HT, Hashimoto’s thyroiditis.
The baseline characteristics of the training and testing groups demonstrate good comparability (Table 1). Both groups exhibit significantly higher median levels of anti-TPO (>35 ng/mL) and anti-TG (>115 IU/mL) compared to normal levels. In both the training and validation groups, patients with TR4 and TR5 thyroid nodules each constitute around half of the total enrolled population. More than 60% of patients present with hypoechoic TN with indistinct borders. Over 50% of patients have an aspect ratio >1 and show microcalcifications in the TN. Around one-third of patients in both groups exhibit symptoms of either hyperthyroidism or hypothyroidism.
In training group, there were 96 benign nodules and 65 malignant nodules, while in testing group, there were 42 benign nodules and 65 malignant nodules (Table 2). In univariate analysis, we identified 6 predictive factors associated with TN malignancy in the training group: TI-RADS, echoic type, aspect ratio, boundary, calcification, and thyroid function (Supplementary Table 1). However, in the testing group, the correlations between boundary, calcification, and thyroid function with TN malignancy did not reach statistical significance. Both in the training and testing groups, the USR scores, including TN+TG score, TN score, and TG score, demonstrated significant statistical differences between benign and malignant TN.
We constructed four models for distinguishing benign and malignant TN in patients with HT based on the 6 clinical indicators and radiomic scores from the training group (Supplementary Table 2). The diagnostic performance of each model was evaluated using ROC analysis (Figure 3). In the training group, the clinical model had an AUC of 0.83 (95% CI: 0.83-0.93). Incorporating the TN score (AUC: 0.90, 95% CI: 0.85-0.94) and TG score (AUC: 0.88, 95% CI: 0.77-0.89) into the model both improved the AUC. The highest AUC (0.94, 95% CI: 0.91-0.98) was achieved when both the TN-USR score and TG-USR score were included in the model. Similar results were obtained when validating the models in the training group. In the Training group, there were significant differences between TN+TG+Clinical model and Clinical model, TN+Clinical model, TG+Clinical model. In the Testing group, only TN+TG+Clinical model exhibited a significant difference when compared to the Clinical model. There were no statistically significant differences observed among the Clinical model, TN+Clinical model, and TG+Clinical model (Supplementary Table 3).
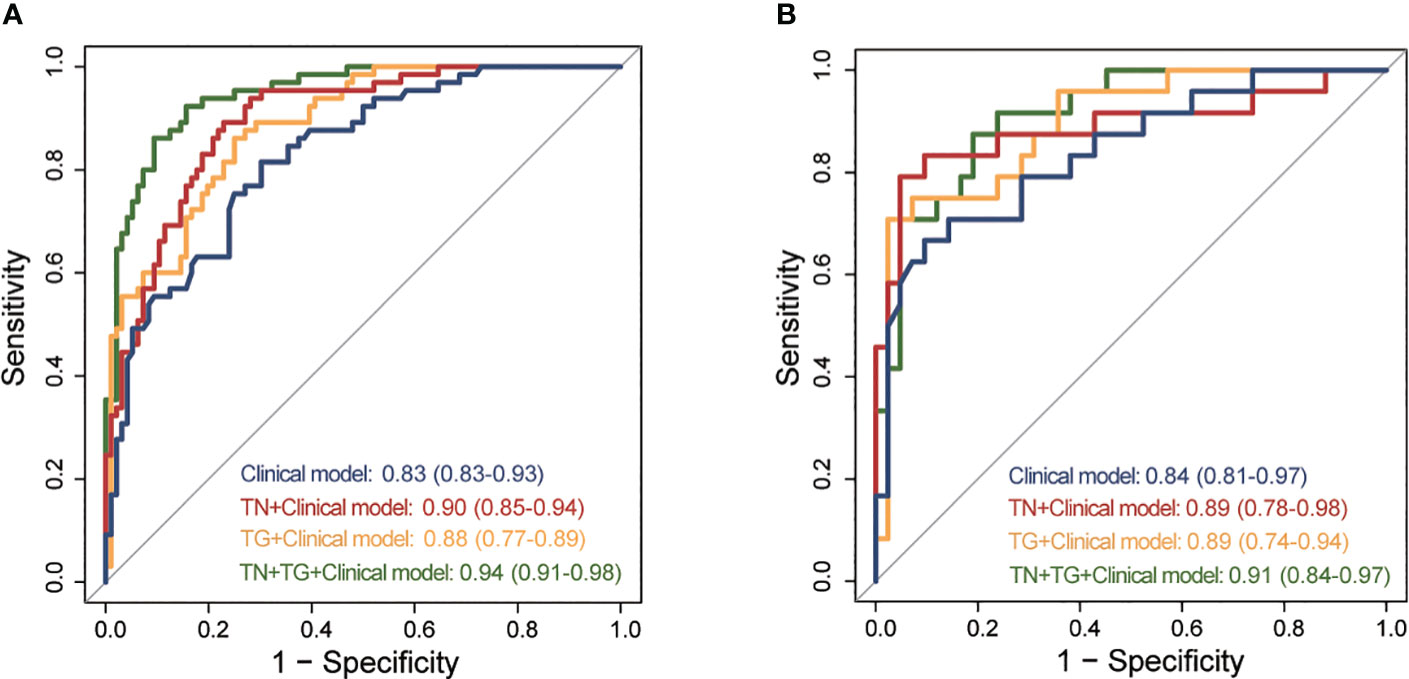
Figure 3 ROC of different predictive models for predicting TC in training and testing group. (A) ROC of different predictive models in training group. (B) ROC of different predictive models in testing group. ROC, receiver operating curves; TC, thyroid cancer; TN, thyroid nodule; TG, thyroid gland.
Further evaluation of the four models using calibration curves and DCA revealed that the TN+TG+clinical model demonstrated higher diagnostic performance and net benefit (Figure 4). Additionally, the TN+TG+Clinical model outperformed the other three models in terms of accuracy (ACC), sensitivity (SEN), specificity (SPE), positive predictive value (PPV), and negative predictive value (NPV) (Table 3). Bootstrap internal validation of the model parameters showed that TN+TG USR score, TI-RADS level, boundary, microcalcification, and thyroid function had resampling rates exceeding 50%, indicating their significant predictive value for distinguishing benign and malignant TN in HT patients (Table 4).
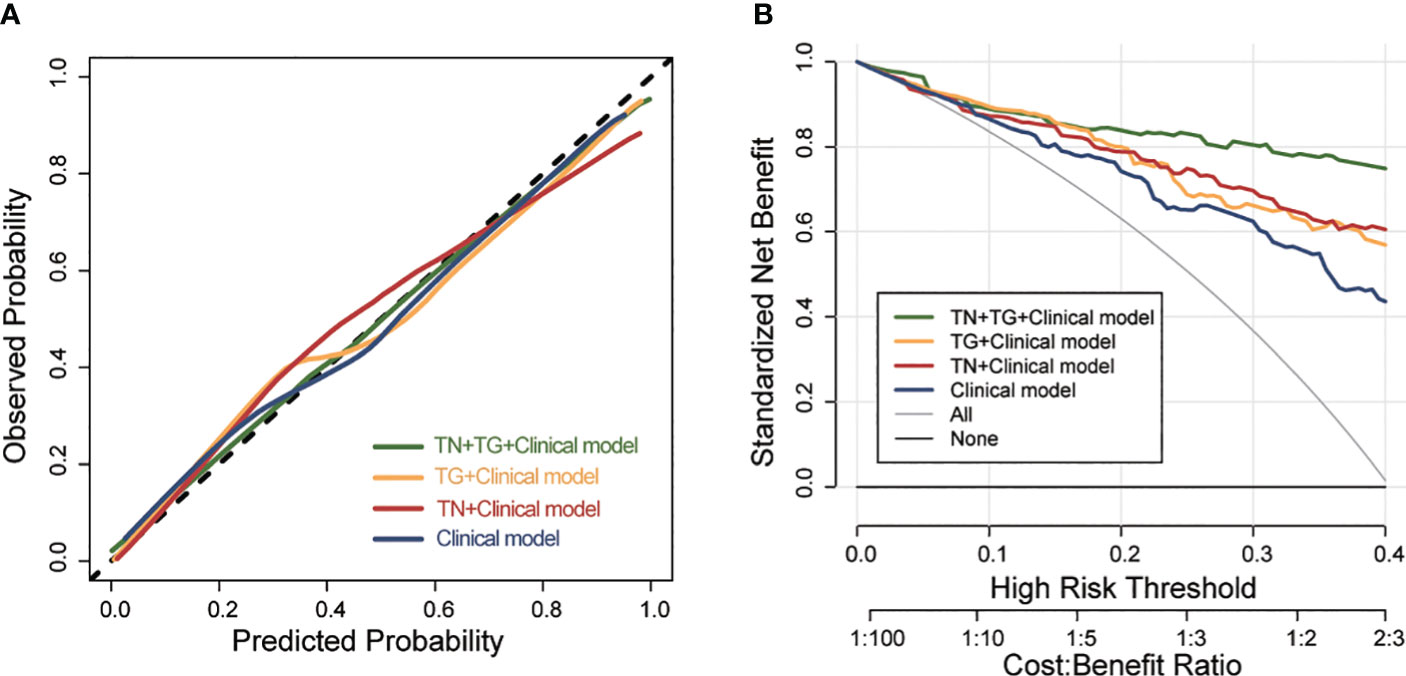
Figure 4 The calibration curve and DCA of different predictive models for predicting TC in training group. (A) the calibration curve of different predictive models. (B) DCA of different predictive models. DCA, Decision Curve Analysis; TC, thyroid cancer; TN, thyroid nodule; TG, thyroid gland.
4 Discussion
Nodules are a common manifestation of TC, however, not all TN are malignant, and the majority of them are benign. The benign-malignant discrimination of TN helps in the early detection of TC, guiding treatment decisions, improving patients’ quality of life, and effectively managing healthcare resources. USR can extract a plethora of image features that are not discernible to the naked eye, aiding in the benign-malignant diagnosis of TN. HT is a prevalent autoimmune disease that exhibits a higher coexistence rate with TC. Research suggests that the chronic inflammation associated with HT may contribute to nodule formation. Previous studies on USR features for benign-malignant discrimination of TN have primarily focused on the nodules themselves. However, in patients with HT and TN, both the US features of the nodules and the thyroid gland itself may possess distinct imaging characteristics that can assist in the benign-malignant diagnosis of TN.
USR holds immense promise and advantages in medical research. It not only enables the acquisition of multi-dimensional information but also offers non-invasiveness, real-time imaging, and applicability across various medical fields. Currently, ultrasound technology has been widely applied in the benign and malignant diagnosis of thyroid nodules, including screening models like ACR TI-RADS, European TI-RADS, Chinese TI-RADS, Horvath TI-RADS, and others (11, 29). However, the diagnostic models mentioned above, as reported in many studies, often exhibit a sensitivity and specificity of no more than 80% (29). Radiomic features, capturing tissue and lesion characteristics, can be integrated with histopathological, genomic, or proteomic data to address clinical challenges (30). A multicenter retrospective study revealed that a random forest model based on USR can distinguish endometrial cancer (31). For example, Feng et al. reported that the combined application of radiomics and pathomics could predict the response to neoadjuvant chemoradiotherapy in locally advanced rectal cancer, with high accuracy and specificity (32). Therefore, by advancing and refining the algorithms and techniques of UIR, we can better harness its potential in medical research, enhancing disease diagnosis, treatment, and prognostic evaluation, and promoting personalized medicine.
US is a commonly used diagnostic modality for TC, and USR has been widely studied and explored in the context of TC. US assists in the early diagnosis and screening, malignant risk assessment, preoperative evaluation and surgical guidance, as well as follow-up and prognostic evaluation of TC by assessing the morphological features of TN, internal echogenicity characteristics, and the presence of lymph node metastasis (7, 9, 33, 34). Although there may be subjectivity in the analysis of nodule features, leading to inconsistencies in interpretation among different physicians, extensive research and exploration in the field of USR are addressing this issue (13). Yu et al. identified that the combination of USR features, US features, and clinical factors enables non-invasive preoperative differentiation between thyroid follicular carcinoma and adenoma, potentially reducing unnecessary diagnostic thyroidectomy in patients with benign follicular adenomas (35). Currently, there has been progress in the application of USR in TC and TN, but challenges remain regarding the accuracy of malignant risk assessment, nodule classification and boundary delineation, establishment and sharing of datasets, and clinical validation (13). Through further research and efforts, we can gradually overcome these challenges. Additionally, our study can expand the application of USR in patients with TN associated with HT, thereby advancing the clinical application of USR in thyroid diseases.
Due to its high sensitivity, non-ionizing radiation, ease operating, and rapid diagnosis, US is the preferred method for screening of TN. In recent years, new US techniques such as contrast-enhanced US and US elastography have greatly improved the diagnostic accuracy of TN (36). For example, Liang et al. found that the diagnostic performance of USR score derived from US image were not worse than the ACR TI-RADS (37). However, diagnosing TC in HT patients can be challenging, as HT itself causes inflammation and nodular formation in the thyroid tissue, making differentiation from malignant lesions on US images difficult (38, 39). Several studies have demonstrated the significant predictive value of US features and USR in HT patients with TC. Feng et al. found that US grayscale ratio was independently associated with central compartment lymph node metastasis in patients with HT (40), while Jin et al. developed a prediction model for central compartment lymph node metastasis in patients with HT based on USR (27). Clearly, USR features play an important role in distinguishing the benign and malignant nature of TN in HT patients, and further exploration is needed.
Our study has shown that USR features of glands combined nodules in patients with HT can improve the accuracy of benign-malignant discrimination of TN. This may be attributed to the close association between certain USR features and the pathological processes of TC in the presence of HT. Firstly, the immunological characteristics of HT, such as the production of autoantibodies, T-cell mediated immune responses, and immune tolerance abnormalities, might be reflected by USR features (21). Previous studies have demonstrated that radiomic features of immune cells, particularly tumor-infiltrating lymphocytes, can predict the prognosis of tumor treatment (41–43). Furthermore, certain USR features have been found to correlate with the presence of malignant gene mutations in TC. Wang et al. reported that a radiomics model based on grayscale and elastography ultrasound had good predictive value for the BRAF-V600E gene mutation in patients with TC (44). Therefore, in future research, integrating radiomics with pathology, genetics, and immunology would greatly enhance our understanding of the correlation between radiomics features and the benign-malignant nature of TC in the presence of HT.
The study has several limitations. Firstly, it is a small-sample retrospective study, and selection bias is inevitable. To validate the research findings and provide stronger evidence, standardized protocols and larger prospective studies are needed. Secondly, the focus on collecting TN images in clinical imaging may lead to inconsistency in US images of the TG affected by HT, which could impact the extraction of radiomic features for the TG in HT. Lastly, the correlation between TC and HT in terms of disease occurrence is still a matter of debate, and it remains unknown whether the radiomic features can be linked to the pathological process of TC induced by HT. In conclusion, further clinical and mechanistic studies are still needed in this research direction to guide the clinical diagnosis of TC.
5 Conclusion
Our study provides compelling evidence that integrating the USR features of TN with the specific features of the TG in patients with HT significantly enhances the differentiation between benign and malignant TN. The TN+TG+clinical model exhibited superior performance compared to other models, demonstrating higher accuracy and net benefit. These findings underscore the critical importance of considering the entire TG, alongside TN characteristics, in the evaluation of TN in HT patients. This comprehensive approach holds valuable implications for clinical decision-making, facilitating more accurate diagnosis and management strategies in this specific patient population. Further research and validation are warranted to confirm the robustness and generalizability of our findings.
Data availability statement
The raw data supporting the conclusions of this article will be made available by the authors, without undue reservation.
Ethics statement
The studies were conducted in accordance with the local legislation and institutional requirements. The participants provided their written informed consent to participate in this study.
Author contributions
MF: Conceptualization, Data curation, Investigation, Software, Writing – original draft. ML: Data curation, Formal Analysis, Methodology, Project administration, Resources, Software, Writing – original draft. XC: Data curation, Formal Analysis, Investigation, Methodology, Project administration, Supervision, Writing – original draft. HC: Methodology, Project administration, Resources, Validation, Writing – original draft. XD: Formal Analysis, Methodology, Resources, Software, Supervision, Writing – original draft. HY: Supervision, Validation, Writing – review & editing. LG: Funding acquisition, Resources, Supervision, Validation, Visualization, Writing – review & editing.
Funding
The author(s) declare financial support was received for the research, authorship, and/or publication of this article. This study is A Project Supported by Scientific Research Fund of Hunan Provincial Education Department (21C0010).
Conflict of interest
The authors declare that the research was conducted in the absence of any commercial or financial relationships that could be construed as a potential conflict of interest.
Publisher’s note
All claims expressed in this article are solely those of the authors and do not necessarily represent those of their affiliated organizations, or those of the publisher, the editors and the reviewers. Any product that may be evaluated in this article, or claim that may be made by its manufacturer, is not guaranteed or endorsed by the publisher.
Supplementary material
The Supplementary Material for this article can be found online at: https://www.frontiersin.org/articles/10.3389/fendo.2023.1267886/full#supplementary-material
References
1. Ralli M, Angeletti D, Fiore M, D'Aguanno V, Lambiase A, Artico M, et al. Hashimoto's thyroiditis: An update on pathogenic mechanisms, diagnostic protocols, therapeutic strategies, and potential Malignant transformation. Autoimmun Rev (2020) 19(10):102649. doi: 10.1016/j.autrev.2020.102649
2. Caturegli P, De Remigis A, Rose NR. Hashimoto thyroiditis: clinical and diagnostic criteria. Autoimmun Rev (2014) 13(4-5):391–7. doi: 10.1016/j.autrev.2014.01.007
3. Siegel RL, Miller KD, Jemal A. Cancer statistics, 2015. CA: Cancer J Clin (2015) 65(1):5–29. doi: 10.3322/caac.21254
4. Cabanillas ME, McFadden DG, Durante C. Thyroid cancer. Lancet (London England) (2016) 388(10061):2783–95. doi: 10.1016/s0140-6736(16)30172-6
5. Dean DS, Gharib H. Epidemiology of thyroid nodules. Best Pract Res Clin Endocrinol Metab (2008) 22(6):901–11. doi: 10.1016/j.beem.2008.09.019
6. Alexander EK, Doherty GM, Barletta JA. Management of thyroid nodules. Lancet Diabetes Endocrinol (2022) 10(7):540–8. doi: 10.1016/s2213-8587(22)00139-5
7. Kobaly K, Kim CS, Mandel SJ. Contemporary management of thyroid nodules. Annu Rev Med (2022) 73:517–28. doi: 10.1146/annurev-med-042220-015032
8. Lee JH, Kim Y, Choi JW, Kim YS. The association between papillary thyroid carcinoma and histologically proven Hashimoto's thyroiditis: a meta-analysis. Eur J Endocrinol (2013) 168(3):343–9. doi: 10.1530/eje-12-0903
9. Alexander EK, Cibas ES. Diagnosis of thyroid nodules. Lancet Diabetes Endocrinol (2022) 10(7):533–9. doi: 10.1016/s2213-8587(22)00101-2
10. Durante C, Grani G, Lamartina L, Filetti S, Mandel SJ, Cooper DS. The diagnosis and management of thyroid nodules: A review. Jama (2018) 319(9):914–24. doi: 10.1001/jama.2018.0898
11. Tessler FN, Middleton WD, Grant EG. Thyroid imaging reporting and data system (TI-RADS): A user's guide. Radiology (2018) 287(1):29–36. doi: 10.1148/radiol.2017171240
12. Kim HG, Moon HJ, Kwak JY, Kim EK. Diagnostic accuracy of the ultrasonographic features for subcentimeter thyroid nodules suggested by the revised American Thyroid Association guidelines. Thyroid (2013) 23(12):1583–9. doi: 10.1089/thy.2012.0586
13. Cao Y, Zhong X, Diao W, Mu J, Cheng Y, Jia Z. Radiomics in differentiated thyroid cancer and nodules: explorations, application, and limitations. Cancers (Basel) (2021) 13(10):2436. doi: 10.3390/cancers13102436
14. Zhao SX, Chen Y, Yang KF, Luo Y, Ma BY, Li YJ. A local and global feature disentangled network: toward classification of benign-malignant thyroid nodules from ultrasound image. IEEE Trans Med Imag (2022) 41(6):1497–509. doi: 10.1109/tmi.2022.3140797
15. Gillies RJ, Kinahan PE, Hricak H. Radiomics: images are more than pictures, they are data. Radiology (2016) 278(2):563–77. doi: 10.1148/radiol.2015151169
16. Huang YQ, Liang CH, He L, Tian J, Liang CS, Chen X, et al. Development and validation of a radiomics nomogram for preoperative prediction of lymph node metastasis in colorectal cancer. J Clin Oncol (2016) 34(18):2157–64. doi: 10.1200/jco.2015.65.9128
17. Wu S, Zheng J, Li Y, Wu Z, Shi S, Huang M, et al. Development and validation of an MRI-based radiomics signature for the preoperative prediction of lymph node metastasis in bladder cancer. EBioMedicine (2018) 34:76–84. doi: 10.1016/j.ebiom.2018.07.029
18. Zheng X, Yao Z, Huang Y, Yu Y, Wang Y, Liu Y, et al. Deep learning radiomics can predict axillary lymph node status in early-stage breast cancer. Nat Commun (2020) 11(1):1236. doi: 10.1038/s41467-020-15027-z
19. Yu Y, Tan Y, Xie C, Hu Q, Ouyang J, Chen Y, et al. Development and validation of a preoperative magnetic resonance imaging radiomics-based signature to predict axillary lymph node metastasis and disease-free survival in patients with early-stage breast cancer. JAMA Netw Open (2020) 3(12):e2028086. doi: 10.1001/jamanetworkopen.2020.28086
20. Ji GW, Zhu FP, Xu Q, Wang K, Wu MY, Tang WW, et al. Radiomic features at contrast-enhanced CT predict recurrence in early stage hepatocellular carcinoma: A multi-institutional study. Radiology (2020) 294(3):568–79. doi: 10.1148/radiol.2020191470
21. Ehlers M, Schott M. Hashimoto's thyroiditis and papillary thyroid cancer: are they immunologically linked? Trends Endocrinol Metab (2014) 25(12):656–64. doi: 10.1016/j.tem.2014.09.001
22. Zhang Q, Zhang S, Pan Y, Sun L, Li J, Qiao Y, et al. Deep learning to diagnose Hashimoto's thyroiditis from sonographic images. Nat Commun (2022) 13(1):3759. doi: 10.1038/s41467-022-31449-3
23. Zhou H, Jin Y, Dai L, Zhang M, Qiu Y, Wang K, et al. Differential diagnosis of benign and Malignant thyroid nodules using deep learning radiomics of thyroid ultrasound images. Eur J Radiol (2020) 127:108992. doi: 10.1016/j.ejrad.2020.108992
24. Lu W, Zhang D, Zhang Y, Qian X, Qian C, Wei Y, et al. Ultrasound radiomics nomogram to diagnose sub-centimeter thyroid nodules based on ACR TI-RADS. Cancers (Basel) (2022) 14(19):4826. doi: 10.3390/cancers14194826
25. Park VY, Lee E, Lee HS, Kim HJ, Yoon J, Son J, et al. Combining radiomics with ultrasound-based risk stratification systems for thyroid nodules: an approach for improving performance. Eur Radiol (2021) 31(4):2405–13. doi: 10.1007/s00330-020-07365-9
26. Luo P, Fang Z, Zhang P, Yang Y, Zhang H, Su L, et al. Radiomics score combined with ACR TI-RADS in discriminating benign and Malignant thyroid nodules based on ultrasound images: A retrospective study. Diagnost (Basel) (2021) 11(6):1011. doi: 10.3390/diagnostics11061011
27. Jin P, Chen J, Dong Y, Zhang C, Chen Y, Zhang C, et al. Ultrasound-based radiomics nomogram combined with clinical features for the prediction of central lymph node metastasis in papillary thyroid carcinoma patients with Hashimoto's thyroiditis. Front Endocrinol (Lausanne) (2022) 13:993564. doi: 10.3389/fendo.2022.993564
28. Epstein JI, Zelefsky MJ, Sjoberg DD, Nelson JB, Egevad L, Magi-Galluzzi C, et al. A contemporary prostate cancer grading system: A validated alternative to the gleason score. Eur Urol (2016) 69(3):428–35. doi: 10.1016/j.eururo.2015.06.046
29. Ha EJ, Baek JH, Na DG. Risk stratification of thyroid nodules on ultrasonography: current status and perspectives. Thyroid (2017) 27(12):1463–8. doi: 10.1089/thy.2016.0654
30. Mayerhoefer ME, Materka A, Langs G, Häggström I, Szczypiński P, Gibbs P, et al. Introduction to radiomics. J Nucl Med (2020) 61(4):488–95. doi: 10.2967/jnumed.118.222893
31. Moro F, Albanese M, Boldrini L, Chiappa V, Lenkowicz J, Bertolina F, et al. Developing and validating ultrasound-based radiomics models for predicting high-risk endometrial cancer. Ultrasound Obstet Gynecol (2022) 60(2):256–68. doi: 10.1002/uog.24805
32. Feng L, Liu Z, Li C, Li Z, Lou X, Shao L, et al. Development and validation of a radiopathomics model to predict pathological complete response to neoadjuvant chemoradiotherapy in locally advanced rectal cancer: a multicentre observational study. Lancet Digit Health (2022) 4(1):e8–e17. doi: 10.1016/s2589-7500(21)00215-6
33. Brito JP, Gionfriddo MR, Al Nofal A, Boehmer KR, Leppin AL, Reading C, et al. The accuracy of thyroid nodule ultrasound to predict thyroid cancer: systematic review and meta-analysis. J Clin Endocrinol Metab (2014) 99(4):1253–63. doi: 10.1210/jc.2013-2928
34. Chen DW, Lang BHH, McLeod DSA, Newbold K, Haymart MR. Thyroid cancer. Lancet (London England) (2023) 401(10387):1531–44. doi: 10.1016/s0140-6736(23)00020-x
35. Yu B, Li Y, Yu X, Ai Y, Jin J, Zhang J, et al. Differentiate thyroid follicular adenoma from carcinoma with combined ultrasound radiomics features and clinical ultrasound features. J Digit Imag (2022) 35(5):1362–72. doi: 10.1007/s10278-022-00639-2
36. Gao X, Ran X, Ding W. The progress of radiomics in thyroid nodules. Front Oncol (2023) 13:1109319. doi: 10.3389/fonc.2023.1109319
37. Liang J, Huang X, Hu H, Liu Y, Zhou Q, Cao Q, et al. Predicting Malignancy in thyroid nodules: radiomics score versus 2017 American College of radiology thyroid imaging, reporting and data system. Thyroid (2018) 28(8):1024–33. doi: 10.1089/thy.2017.0525
38. Wu G, Zou D, Cai H, Liu Y. Ultrasonography in the diagnosis of Hashimoto's thyroiditis. Front Biosci (Landmark Ed) (2016) 21(5):1006–12. doi: 10.2741/4437
39. Mao L, Zheng C, Ou S, He Y, Liao C, Deng G. Influence of Hashimoto thyroiditis on diagnosis and treatment of thyroid nodules. Front Endocrinol (Lausanne) (2022) 13:1067390. doi: 10.3389/fendo.2022.1067390
40. Feng N, Wei P, Kong X, Xu J, Yao J, Cheng F, et al. The value of ultrasound grayscale ratio in the diagnosis of papillary thyroid microcarcinomas and benign micronodules in patients with Hashimoto's thyroiditis: A two-center controlled study. Front Endocrinol (Lausanne) (2022) 13:949847. doi: 10.3389/fendo.2022.949847
41. Khorrami M, Prasanna P, Gupta A, Patil P, Velu PD, Thawani R, et al. Changes in CT radiomic features associated with lymphocyte distribution predict overall survival and response to immunotherapy in non-small cell lung cancer. Cancer Immunol Res (2020) 8(1):108–19. doi: 10.1158/2326-6066.Cir-19-0476
42. Su GH, Xiao Y, Jiang L, Zheng RC, Wang H, Chen Y, et al. Radiomics features for assessing tumor-infiltrating lymphocytes correlate with molecular traits of triple-negative breast cancer. J Transl Med (2022) 20(1):471. doi: 10.1186/s12967-022-03688-x
43. Sun R, Limkin EJ, Vakalopoulou M, Dercle L, Champiat S, Han SR, et al. A radiomics approach to assess tumour-infiltrating CD8 cells and response to anti-PD-1 or anti-PD-L1 immunotherapy: an imaging biomarker, retrospective multicohort study. Lancet Oncol (2018) 19(9):1180–91. doi: 10.1016/s1470-2045(18)30413-3
44. Wang YG, Xu FJ, Agyekum EA, Xiang H, Wang YD, Zhang J, et al. Radiomic model for determining the value of elasticity and grayscale ultrasound diagnoses for predicting BRAF(V600E) mutations in papillary thyroid carcinoma. Front Endocrinol (Lausanne) (2022) 13:872153. doi: 10.3389/fendo.2022.872153
Keywords: thyroid nodules, Hashimoto’s thyroiditis, ultrasound, radiomics, diagnosis
Citation: Fang M, Lei M, Chen X, Cao H, Duan X, Yuan H and Guo L (2023) Radiomics-based ultrasound models for thyroid nodule differentiation in Hashimoto’s thyroiditis. Front. Endocrinol. 14:1267886. doi: 10.3389/fendo.2023.1267886
Received: 27 July 2023; Accepted: 25 September 2023;
Published: 23 October 2023.
Edited by:
Zia Moinuddin, Central Manchester University Hospitals NHS Foundation Trust, United KingdomReviewed by:
Dana I. Stoian, Victor Babes University of Medicine and Pharmacy, RomaniaJeehee Yoon, Chonnam National University Bitgoeul Hospital, Republic of Korea
Copyright © 2023 Fang, Lei, Chen, Cao, Duan, Yuan and Guo. This is an open-access article distributed under the terms of the Creative Commons Attribution License (CC BY). The use, distribution or reproduction in other forums is permitted, provided the original author(s) and the copyright owner(s) are credited and that the original publication in this journal is cited, in accordance with accepted academic practice. No use, distribution or reproduction is permitted which does not comply with these terms.
*Correspondence: Lili Guo, Z3VvbGlsaUBjc3NmeWJqeS5jb20=; Hongxia Yuan, MjQ1NzA0NTYyMkBxcS5jb20=; Xingxing Duan, ZHVhbnhpbmd4aW5nLjIwMDdAMTYzLmNvbQ==
†These authors have contributed equally to this work