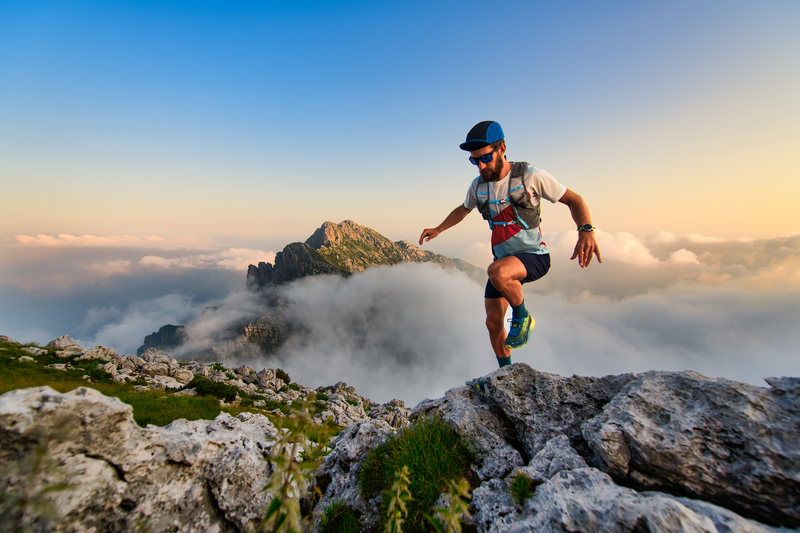
94% of researchers rate our articles as excellent or good
Learn more about the work of our research integrity team to safeguard the quality of each article we publish.
Find out more
SYSTEMATIC REVIEW article
Front. Endocrinol. , 31 August 2023
Sec. Thyroid Endocrinology
Volume 14 - 2023 | https://doi.org/10.3389/fendo.2023.1227339
This article is part of the Research Topic Radionuclide and Molecular Probes in the Diagnosis and Treatment of Thyroid Diseases View all 6 articles
Background: The performance in evaluating thyroid nodules on ultrasound varies across different risk stratification systems, leading to inconsistency and uncertainty regarding diagnostic sensitivity, specificity, and accuracy.
Objective: Comparing diagnostic performance of detecting thyroid cancer among distinct ultrasound risk stratification systems proposed in the last five years.
Evidence acquisition: Systematic search was conducted on PubMed, EMBASE, and Web of Science databases to find relevant research up to December 8, 2022, whose study contents contained elucidation of diagnostic performance of any one of the above ultrasound risk stratification systems (European Thyroid Imaging Reporting and Data System[Eu-TIRADS]; American College of Radiology TIRADS [ACR TIRADS]; Chinese version of TIRADS [C-TIRADS]; Computer-aided diagnosis system based on deep learning [S-Detect]). Based on golden diagnostic standard in histopathology and cytology, single meta-analysis was performed to obtain the optimal cut-off value for each system, and then network meta-analysis was conducted on the best risk stratification category in each system.
Evidence synthesis: This network meta-analysis included 88 studies with a total of 59,304 nodules. The most accurate risk category thresholds were TR5 for Eu-TIRADS, TR5 for ACR TIRADS, TR4b and above for C-TIRADS, and possible malignancy for S-Detect. At the best thresholds, sensitivity of these systems ranged from 68% to 82% and specificity ranged from 71% to 81%. It identified the highest sensitivity for C-TIRADS TR4b and the highest specificity for ACR TIRADS TR5. However, sensitivity for ACR TIRADS TR5 was the lowest. The diagnostic odds ratio (DOR) and area under curve (AUC) were ranked first in C-TIRADS.
Conclusion: Among four ultrasound risk stratification options, this systemic review preliminarily proved that C-TIRADS possessed favorable diagnostic performance for thyroid nodules.
Systematic review registration: https://www.crd.york.ac.uk/prospero, CRD42022382818.
Thyroid nodule is a common occurrence in clinical practice. Ultrasonography plays an irreplaceable role in the early detection of thyroid cancer with merits of being noninvasive, convenient, and affordable (1). As high-resolution ultrasound technology and the standard of living improve, the detection rate of thyroid nodules has increased gradually. Ultrasound examination can detect 30% to 67% of thyroid nodules, with malignant nodules accounting for 7% to 15% (2). In most cases, due to possibly overlapped imaging phenotypes between benign and malignant thyroid nodules, the interpretation of ultrasound images still relies on the subjective discrimination of radiologists (3). Thus, it cannot accurately determine the malignancy of thyroid tumors via dependence on single imaging features, which calls for making elaborate evaluation criteria (4). To address this, TIRADS was proposed in 2009, which was inspired by the Breast Imaging Reporting and Data System (BIRADS) of the ACR (5). Subsequently, numerous classifying systems suitable for various countries gradually progressed in later periods, whose versions released since 2017 encompassed Eu-TIRADS, ACR TIRADS, and C-TIRADS (6). Hence, application of TIRADS in worldwide ultrasound departments is relatively disorganized, which causes challenges for doctors in underdeveloped countries to choose the optimal diagnostic system (6). Moreover, advancement in medical artificial intelligence facilitates the establishment of computer-aided diagnosis (CAD) systems, whose classifying effectiveness has been initially verified in the examination of breast and thyroid nodules (7). S-Detect, a widely applied ultrasound CAD, is employed for recognizing thyroid nodules through early data learning and algorithm optimization (8). Although the Expert Committee agrees that each risk stratification option including TIRASD and CAD has its own advantages and disadvantages, there is a necessity to propose the most universal and efficient system for better clinical service (9).
Previous systematic reviews explored the diagnostic performance of various ultrasound risk stratification systems for thyroid nodules (10, 11), but traditional meta-analysis methods were not ideal for indirectly comparing multiple diagnostic techniques from separate studies. Network meta-analysis is a method that allows for indirect and direct comparison of multiple treatment or testing options by parsing data extracted from different studies to rank these regimens (12). Therefore, this systemic review carried out a comprehensive quantitative synthesis of discovered studies by using network meta-analysis to clarify clinical diagnostic performance among the latest risk stratification systems since 2017 and S-Detect in distinguishing benign from malignant thyroid nodules.
This network meta-analysis study was executed in accordance with the Preferred Reporting Items for Systematic Reviews and Meta-Analyses (PRISMA) guidelines. The study protocol was registered with PROSPERO (york.ac.uk) and assigned registration number CRD42022382818. A comprehensive literature search was performed by using the Population, Intervention, Comparison, and Outcome (PICO) format to answer the following questions: How to compare the diagnostic performance among ACR TIRADS, Eu-TIRADS, S-DETECT, and C-TIRADS by taking cytologic or histopathological tests as reference standards in patients who underwent ultrasound detection of thyroid nodules?
PubMed, EMBASE, and Web of Science databases were explored from the date of database creation to December 8, 2022, complying with the following search terms: ACR TIRADS, EU-TIRADS, C-TIRADS, S-detect, qualitative diagnosis, and thyroid nodule. The search strategy was mapped out by a researcher with six months of information retrieval experience. Two researchers independently screened the search results, and any discrepancies were resolved through discussion with a third investigator. After removing duplicates and non-research articles, such as reviews, conference reports, and case reports, as well as excluding articles that did not meet research question criteria, a final sample of studies was obtained. These studies provided sufficient data to assess the diagnostic performance of at least one of the four ultrasound risk stratification options.
Two investigators were responsible for data extraction, and any conflicting results were decided through discussion with a third investigator. Recorded information covered relevant demographic factors such as country, ethnicity, sex ratio, mean age, the number of patients and nodules, as well as the mean diameter of benign and malignant nodules. Additionally, ultrasound risk stratification methods, optimal cut-off values, and reference criteria in each study were noted. The investigators also extracted the number of true-positive, true-negative, false-positive, and false-negative nodules based on the reference criteria to calculate diagnostic performance of each risk stratification system.
In 2017, the TIRADS Committee of the ACR published a white paper introducing a new risk stratification format for thyroid nodules, known as ACR TIRADS. This system categorizes nodules as benign (TR1, 0 points), not suspicious (TR2, 2 points), mildly suspicious (TR3, 3 points), moderately suspicious (TR4, 4-6 points), or highly suspected malignant (TR5, 7 points) (13). Eu-TIRADS identifies thyroid nodules as benign (TR2), low risk (TR3), intermediate risk (TR4) or high risk (TR5) (14). C-TIRADS categorizes thyroid nodules as non-nodules (C-TIRADS TR1), benign (C-TIRADS TR2), potentially benign (C-TIRADS TR3), or varying levels of malignancy suspicion (C-TIRADS TR4a-c, TR5), with TR5 nodules having the highest malignant probability (>90%) (15). In addition to these options, S-Detect, a real-time CAD system software, is integrated into ultrasound systems that can automatically identify the region of interest associated with nodule, thereby calculating contour and quantifying various ultrasound manifestations of the nodule, including size, composition, shape, orientation, echogenicity, and spongiform. These quantitative features are then analyzed by the AI algorithm to diagnose whether the nodule is benign or malignant (16).
The risk of bias and applicability concerns of the included studies were assessed based on QUADAS-2 tool, which was routinely employed for analyzing validation studies of diagnostic criteria. Each item was rated as low, high, or unclear risk. This tool estimates the risk of bias across four domains: patient selection, index test, reference standard, as well as flow and timing. Additionally, it assesses applicability concerns, focusing on the first three domains. Two independent reviewers (C.L. and S.H.) commented on included studies, with any disagreement addressed by consensus. The QUADAS-2 scale was then completed based on commentary information.
The best sensitivity, specificity, and accuracy of each stratification option were determined using Stata13.1 “midas” packages, based on the number of true-positive, false positives, true-negative, and false-negative assessments extracted from the individual studies. I2 was computed to quantify the heterogeneity among inter-studies in terms of sensitivity, specificity, and accuracy for each system, and was considered to be substantial when at least 75%. Then, through included one-arm/two-arm/three-arm studies, direct and indirect comparisons of performance metrics (e.g., sensitivity, specificity, et al.) among four options were performed through random effect network meta-analysis within the frequentist framework, based on risk category with the highest diagnostic accuracy in each option. Next, within-group heterogeneity and between-group heterogeneity were calculated. Finally, the risk stratification system evaluated by included medium-quantity studies was identified as reference option, and odds ratios (ORs) and 95% confidence intervals (CIs) were used to express the sensitivity, specificity, and accuracy with respect to that system for the remaining three systems.
This network meta-analysis was conducted by R package “rstan” (version 4.1.3; R Foundation for Statistical Computing, Vienna, Austria). Analysis of Variance (ANOVA) model based on Bayesian algorithm was applied to exhibit network meta-analysis among four systems by utilizing two independent binomial distributions to describe the true positive and true negative rates between benign and malignant thyroid nodules and meantime considering the correlation between sensitivity and specificity (17). There were 4 trials to be evaluated and 88 included studies. In certain study i, (Yi1k, Yi2k) referred to true positive and true negative, respectively; (Ni1k, Ni2k) referred to malignant and benign thyroid nodules, respectively; and (πi1k, πi2k) referred to “unobserved” sensitivity and specificity, respectively. The binomial distribution describing true positive and true negative between malignant and benign thyroid nodules was as follows:
I = 1,… I; j = 1, 2, k = 1,… K; xi refers to covariate affecting πijk.
Monte Carlo chain number, pre-iterations number, iteration number and step size were set at 3, 10000, 1000, and 5, respectively. Subsequently, absolute effect sizes including sensitivity and specificity, as well as relative effect sizes including risk ratio (RR), OR, and DOR were calculated.
Besides, we employed various statistical packages in R4.1.3 to analyze extracted data. Specifically, we utilized “mada” and “reshape” packages to conduct the Summary Receiver Operating Characteristic (SROC) analysis of four optimal categories from risk stratification systems. The forest plots respectively related to ORs of sensitivity and specificity were created using “forestplot” package. To visualize publication bias, we used the “meta” and “metafor” packages.
A total of 1998 articles were initially identified through multiple database searches, of which 147 duplicate articles were removed, leaving 1,851 articles for further review. After excluding 1,613 articles based on title and abstract screening, 238 articles were reviewed in full, where 61 articles lacked sufficient data to determine diagnostic performance, and 45 articles were irrelevant to the research topic. Ultimately, 132 studies were included in meta-analysis, comprising 90,451 nodules. Figure 1 showed screening process. Specifically, 76 studies only analyzed ACR TIRADS, 10 studies only analyzed Eu-TIRADS, 5 studies only analyzed C-TIRADS and 5 studies only analyzed S-Detect. Furthermore, there were 21, 5, 2, and 4 studies comparing diagnostic effectiveness of ACR TIRADS and Eu-TIRADS, ACR TIRADS and C-TIRADS, Eu-TIRADS and S-Detect, as well as ACR TIRADS and S-Detect, respectively. In addition, only 3 studies examined the diagnostic performance of all three diagnostic systems including ACR-TIRADS, Eu-TIRADS, and C-TIRADS, and only 1 study examined ACR TIRADS, Eu-TIRADS, and S-Detect.
A summary of the risk of bias and applicability concerns for each domain was exhibited in Supplementary Figure 1. Table 1 presented a detailed assessment for each domain. Most studies showed a low or unclear risk of bias in each domain. 33 enrolled studies had low risk of bias, while 14 studies were considered to have high risk of bias due to non-consecutive patient selection and inappropriate exclusions. The remaining studies with non-consecutive patient selection were considered to have an unclear risk of bias. In index test domain, most of studies had a low or unclear risk of bias because of the prespecified thresholds and blinding. The reference standard domain had a low risk of bias for most studies since they were based on cytological and pathological golden standards. In the flow and timing domain, 2 studies had high risk of bias due to introduced bias of patient flow, while the others were considered to have low or unclear risk of bias. In terms of applicability concerns, at high concern, there were 6 studies in one domain, 3 studies in two domains, and only 1 study in all domains, while the other studies had low or unclear concerns.
Table 1 Summary of risk stratification systems for evaluating ultrasound thyroid nodules in network meta-analysis.
Table 2 shows the diagnostic performance of the four stratification systems at each system’s risk category thresholds. 107 studies evaluated ACR TIRADS (18–124). After meta-analysis, in the best risk category threshold TR5 of ACR TIRADS, sensitivity ranged from 65% to 76%; specificity ranged from 84% to 89%; and accuracy ranged from 77% to 80%. 37 studies evaluated Eu-TIRADS (20, 22, 28, 48, 55, 65, 66, 70, 73, 74, 78, 80–82, 85, 86, 92, 93, 95, 99–101, 115, 118, 121, 125–136). After meta-analysis, in the best risk category threshold TR5 of Eu-TIRADS, sensitivity ranged from 66% to 82%; specificity ranged from 75% to 87%; and accuracy ranged from 74% to 82%. 13 studies evaluated C-TIRADS (20, 69, 70, 72, 80, 87, 120, 137–142), with sensitivity ranging from 70% (C-TIRADS TR4c) to 95% (C-TIRADS TR4a); specificity ranging from 54% (C-TIRADS TR4a) to 92% (C-TIRADS TR4c); and accuracy ranging from 75% (C-TIRADS TR4a) to 88% (C-TIRADS TR4b). 12 studies evaluated S-Detect (45, 46, 65, 112, 114, 133, 134, 143–147), with sensitivity ranging from 73% to 88%; specificity ranging from 66% to 86%; accuracy ranging from 72% to 83%. Heterogeneity was found to be significant across all evaluated risk category thresholds for all systems in terms of sensitivity, specificity, and accuracy (all I2>75%).
Table 2 Results of meta-analysis of diagnostic performance of individual risk stratification systems.
As displayed in Table 3, the network meta-analysis was conducted on threshold categories with the highest accuracy, specifically TR5 for Eu-TIRADS, TR5 for ACR TIRADS, and possible malignancy for S-Detect. Owing to similar accuracy performance among TR4a, TR4b, and TR4c, these threshold categories of C-TIRADS were respectively compared with Eu-TIRADS TR5, ACR TIRADS TR5, and S-Detect possible malignancy. Finally, a total of 88 studies including 59,304 nodules were included in network meta-analysis based on the best cut-off category in each system. Figure 2 depicted the direct comparisons within the network meta-analysis, where the width of the lines between systems represented the number of studies included in each comparison. Eu-TIRADS was chosen as reference system for network meta-analysis, given that an appropriate number of studies can partially ensure results’ reliability. Figure 3 graphically displayed the results of the network meta-analysis in terms of the OR for comparisons of sensitivity, specificity, and accuracy of other systems with Eu-TIRADS.
Table 3 Comparisons of sensitivity, specificity, and DOR of risk classification systems, based on network meta-analysis.
Figure 2 Schematic of network meta-analysis of 36 studies containing more than one risk classification system for thyroid nodules on ultrasound. Direct comparisons within individual studies were indicated by lines connecting pairs of systems. Number of studies involved in each pairwise comparison was indicated by width of lines. ACR TIRADS, American College of Radiology Thyroid Imaging Reporting and Data System; Eu-TIRADS, European Thyroid Association Thyroid Imaging Reporting and Data System; C-TIRADS, Chinese version of Thyroid Imaging Reporting and Data System; S-Detect: Computer aided diagnosis system using deep learning.
Figure 3 Summary of sensitivity (A) and specificity (B) from network meta-analysis among the best cut-offs in each system. Tick marks indicate ORs, blue boxes around tick marks are proportional to precision of estimates, and horizontal lines indicate 95% CIs. Eu-TIRADS TR5 served as reference for ORs. ACR TIRADS, American College of Radiology Thyroid Imaging Reporting and Data System; Eu-TIRADS, European Thyroid Association Thyroid Imaging Reporting and Data System; C-TIRADS, Chinese version of Thyroid Imaging Reporting and Data System; S-Detect, Computer aided diagnosis system using deep learning; ABM, Arm-based model; OR, Odds ratio.
In the second section, sensitivity for ACR TIRADS TR5 [RR= 0.91 (95% CI, 0.85–0.98); OR=0.72 (95% CI, 0.57–0.93)] was significantly lower than Eu-TIRADS TR5, and lower (although not significantly) for S-Detect possible malignancy [RR= 0.97 (95% CI, 0.85–1.10); OR=0.94 (95% CI, 0.58–1.50)]. In contrast, the sensitivity of C-TIRADS [RR= 1.10 (95% CI, 1.00–1.22); OR=1.67 (95% CI, 1.01–2.91)] was significantly higher than Eu-TIRADS. The specificity was significantly higher than Eu-TIRADS for ACR TIRADS [RR= 1.11 (95% CI, 1.05–1.17); OR=1.59 (95% CI, 1.29–1.90)], and higher (although not significantly) for S-Detect [RR= 1.06 (95% CI, 0.95–1.17); OR=1.33 (95% CI, 0.84–1.95). On the other hand, the specificity was lower (although not significantly) than Eu-TIRADS for C-TIRADS [RR= 0.97 (95% CI, 0.86–1.07); OR=0.92 (95% CI, 0.60–1.32)]. DOR is commonly applied in systematic meta-analysis to comprehensively assess the performance of diagnostic tests in different studies by combining relationship between true positive rate and false positive rate. DOR close to 1 indicates less accuracy in the test. The DOR of C-TIRADS [12.36 (95% CI, 6.55–22.24)] ranked first, followed in order by S-Detect [9.87 (95% CI, 6.09–15.46)], ACR TIRADS [9.20 (95% CI, 7.58–10.97)], and Eu-TIRADS [8.22 (95% CI, 6.05–10.82)]. Figure 4 presented the results of SROC analysis among four risk stratification systems. It was found that C-TIRADS 4b had significantly higher AUC [0.93 (95% CI, 0.90–0.95)] compared to the other systems.
Figure 4 Significant separation of combined effect size point estimate of AUC of C-TIRADS TR4b, Eu-TIRADS TR5, ACR TIRADS TR5 and S-Detect possible malignancy. Additionally, the confidence interval of C-TIRADS did not overlap with those of the other diagnostic systems, indicating that C-TIRADS had the highest AUC in diagnosing the benign or malignant nature of thyroid nodules. ACR TIRADS, American College of Radiology Thyroid Imaging Reporting and Data System; Eu-TIRADS, European Thyroid Association Thyroid Imaging Reporting and Data System; C-TIRADS, Chinese version of Thyroid Imaging Reporting and Data System; S-Detect, Computer aided diagnosis system using deep learning; AUC, Area under curve.
In network meta-analysis, within-group heterogeneity and between-group heterogeneity were high (all I2>75%, p<0.1). Subgroup analysis found that publication year, age, gender, and race were not contributors to the heterogeneity (all I2>75%, p<0.1).
Figure 5 showed funnel plots of sensitivity and specificity. Visually, each point was evenly distributed on both sides. There were a few individual points with significant deviation, but they had minimal impact on the overall results. From the funnel plot, there was no obvious publication bias.
Figure 5 Assessment of publication bias by funnel plots with respect to (A) sensitivity, and (B) specificity. There was no providing evidence of publication bias.
This study took on network meta-analysis of four ultrasound-based risk stratification systems to compare their diagnostic performance in thyroid malignancy. It deemed that the highest accuracy threshold categories as TR5 for Eu-TIRADS, TR5 for ACR TIRADS, and possible malignancy for S-Detect. In the network meta-analysis respectively with C-TIRADS TR4a/4b/4c, the best threshold category of C-TIRADS was identified as TR4b. At the optimal category thresholds, the sensitivity and specificity of the risk stratification options ranged from 68–82% and 71–81%, respectively. Based on AUC determined for these category thresholds, SROC analysis also revealed the best diagnostic test performance for C-TIRADS TR4b.
Ultrasound is taken as the best imaging modality for determining malignant risk of thyroid nodules (125). However, in China, 82.3% of thyroid surgeons or endocrinologists express their concern regarding the overuse of surgery (22). However, each risk stratification system was proposed according to different racial populations and would vary in their assignment of risk categories to one nodule (37). Thus, in view of large population in China, it is necessary to explore system with relatively satisfactory diagnostic performance, consequently reducing medical care burden (45). In addition, acknowledging different diagnostic performances in various ultrasound-based risk stratification systems and combining their unique strengths will enable clinicians to make evidence-based choices about the most appropriate system for personal practice. Moreover, consistency and standardization of thyroid nodule evaluation across different healthcare settings will aid in muti-center validation of clinical trials (133).
Both C-TIRADS and ACR TIRADS are score-based systems that employ certain scores and weights to assess likelihood of nodule malignancy (22, 72). In contrast, Eu-TIRADS is a pattern-based system (126). On the other hand, S-Detect is a radiomics-based system that is driven by deep learning algorithms to automatically classify and evaluate thyroid nodules (112). Relative to Eu-TIRADS and C-TIRADS, ACR TIRADS has highly comprehensive and detailed reference entry at TR5 cutoff, which might lead to an increase in diagnostic specificity but decrease in sensitivity (51). C-TIRADS with higher sensitivity, diagnostic accuracy, and DOR than other systems might be due to more reasonable feature selection for malignancy diagnosis by TR4 subdivision. Based on the outcomes of multiple logistic regression and counting analysis, solid composition, microcalcification, marked hypoechoic features, blurred margins, irregular margins or extrathyroidal extension, and vertical orientation are considered suspicious malignant ultrasound features in C-TIRADS, while comet tail artifact is a benign feature (139). Through high-dimensional imaging features in ultrasound, S-Detect demonstrated a relatively high level of specificity, which was consistent with previous research findings (145). In addition, S-Detect, whose DOR ranked second among risk stratification systems, exhibited superior diagnostic performance, providing compelling support for its clinical utility (146).
There were some limitations in this study. Firstly, this network meta-analysis took histologic or cytologic tests as reference standards, and its results might not be extrapolated to other situations, like only clinical follow-up. Secondly, diagnostic performance might be affected by uncontrolled variables, such as scan technique, ultrasound equipment quality, and interpreter experience. The inclusion of studies conducted in various countries worldwide, with diverse populations, reference standards, and trial designs, may introduce some degree of uncertainty into direct/indirect comparisons. Thirdly, literature number of C-TIRADS and S-Detect was relatively small, which might introduce publication bias. Hence, this systemic review was a preliminary study to discuss C-TIRADS applicability (53). Fourthly, another limitation of the study is incomplete collection of clinical data, such as nodule size (≥10mm and <10mm) and age stratification, which may contribute to unobserved heterogeneity and limit the generalizability of results (16). Up to now, almost all literature focused on middle-aged and elderly populations, except several studies on children (81, 82, 114, 124), emphasizing more attention should be paid to pediatric patients in the future. Finally, it ought to be noted that comparisons of the systems were based on a single meta-analysis-derived best cutoff. It is important to recognize that our selected threshold per system might not fully reflect real-world experience in applying these systems with a range of risk categories. Interestingly, there is a 5% chance of malignancy at C-TIRADS TR4a in Chinese (137), which will require intimate monitoring or even puncture biopsy. How to balance the applicability between C-TIRADS TR4a and TR4b is of significance to avoid unnecessary invasive biopsy.
This network meta-analysis evaluated four risk stratification options on ultrasound for thyroid nodules. Sensitivity, DOC, and accuracy were the highest for C-TIRADS TR4b (moderate malignancy suspicion); sensitivity was lowest but specificity was highest for ACR TIRADS TR5 (highly suspicious). This tentative assessment of risk stratification systems for thyroid nodules may assist in future system updates and guide decisions regarding system implementation.
The raw data supporting the conclusions of this article will be made available by the authors, without undue reservation.
JL and LTY were responsible for the review concept and design. LTY, CL, ZC and SQH drafted the manuscript. JL and LTY provided critical revision of the manuscript for important intellectual content. All authors contributed to the article and approved the submitted version.
This work was supported by National Natural Science Foundation of China(61971451, U22A20303), Innovative Province special construction foundation of Hunan Province(2019SK2131), the Science and Technology Innovation Program of Hunan Province(2021RC4016), Clinical Research Center for Medical Imaging in Hunan Province in China(2020SK4001) and Hunan Cancer Hospital Climb Plan(ZX2021004, 2021).
The authors declare that the research was conducted in the absence of any commercial or financial relationships that could be construed as a potential conflict of interest.
All claims expressed in this article are solely those of the authors and do not necessarily represent those of their affiliated organizations, or those of the publisher, the editors and the reviewers. Any product that may be evaluated in this article, or claim that may be made by its manufacturer, is not guaranteed or endorsed by the publisher.
The Supplementary Material for this article can be found online at: https://www.frontiersin.org/articles/10.3389/fendo.2023.1227339/full#supplementary-material
1. Mai W, Zhou M, Li J, Yi W, Li S, Hu Y, et al. The value of the Demetics ultrasound-assisted diagnosis system in the differential diagnosis of benign from Malignant thyroid nodules and analysis of the influencing factors. Eur Radiol (2021) 31(10):7936–44. doi: 10.1007/s00330-021-07884-z
2. Sb F, Nd P. The incidental thyroid nodule. CA: Cancer J Clin (2018) 68(2):97–105. doi: 10.3322/caac.21447
3. Alexander LF, Patel NJ, Caserta MP, Robbin ML. Thyroid ultrasound: diffuse and nodular disease. Radiol Clin North Am (2020) 58(6):1041–57. doi: 10.1016/j.rcl.2020.07.003
4. Grani G, Sponziello M, Pecce V, Ramundo V, Durante C. Contemporary thyroid nodule evaluation and management. J Clin Endocrinol Metab (2020) 105(9):2869–83. doi: 10.1210/clinem/dgaa322
5. Horvath E, Majlis S, Rossi R, Franco C, Niedmann JP, Castro A, et al. An ultrasonogram reporting system for thyroid nodules stratifying cancer risk for clinical management. J Clin Endocrinol Metab (2009) 94(5):1748–51. doi: 10.1210/jc.2008-1724
6. Kim DH, Kim SW, Basurrah MA, Lee J, Hwang SH. Diagnostic performance of six ultrasound risk stratification systems for thyroid nodules: A systematic review and network meta-analysis. AJR Am J Roentgenol (2023) 220(6):791–803. doi: 10.2214/AJR.22.28556
7. Chen Y, Li D, Zhang X, Jin J, Shen Y. Computer aided diagnosis of thyroid nodules based on the devised small-datasets multi-view ensemble learning. Med Image Anal (2021) 67:101819. doi: 10.1016/j.media.2020.101819
8. Zhou L, Zheng LL, Zhang CJ, Wei HF, Xu LL, Zhang MR, et al. Comparison of S-Detect and thyroid imaging reporting and data system classifications in the diagnosis of cytologically indeterminate thyroid nodules. Front Endocrinol (2023) 14:1098031. doi: 10.3389/fendo.2023.1098031
9. Zhang Q, Ma J, Sun W, Zhang L. Comparison of diagnostic performance between the American College Of Radiology Thyroid imaging reporting and data system and american thyroid association guidelines: a systematic review. Endocr Pract (2020) 26(5):552–63. doi: 10.4158/EP-2019-0237
10. Li W, Wang Y, Wen J, Zhang L, Sun Y. Diagnostic performance of american college of radiology TI-RADS: A systematic review and meta-analysis. AJR Am J Roentgenol (2021) 216(1):38–47. doi: 10.2214/AJR.19.22691
11. Zhang D, Jiang F, Yin R, Wu G-G, Wei Q, Cui X-W, et al. A review of the role of the S-detect computer-aided diagnostic ultrasound system in the evaluation of benign and malignant breast and thyroid masses. Med Sci Monit (2021) 27:e931957. doi: 10.12659/MSM.931957
12. Rouse B, Chaimani A, Li T. Network meta-analysis: an introduction for clinicians. Intern Emerg Med (2017) 12(1):103–11. doi: 10.1007/s11739-016-1583-7
13. Tessler FN, Middleton WD, Grant EG. Thyroid imaging reporting and data system (TI-RADS): A user’s guide. Radiology (2018) 287(1):29–36. doi: 10.1148/radiol.2017171240
14. Russ G, Bonnema SJ, Erdogan MF, Durante C, Ngu R, Leenhardt L. European thyroid association guidelines for ultrasound Malignancy risk stratification of thyroid nodules in adults: the EU-TIRADS. Eur Thyroid J (2017) 6(5):225–37. doi: 10.1159/000478927
15. Zhou J, Yin L, Wei X, Zhang S, Song Y, Luo B, et al. Chinese guidelines for ultrasound Malignancy risk stratification of thyroid nodules: the C-TIRADS. Endocrine (2020) 70(2):256–79. doi: 10.1007/s12020-020-02441-y
16. Choi YJ, Baek JH, Park HS, Shim WH, Kim TY, Shong Y, et al. A computer-aided diagnosis system using artificial intelligence for the diagnosis and characterization of thyroid nodules on ultrasound: initial clinical assessment. Thyroid (2017) 27(4):546–52. doi: 10.1089/thy.2016.0372
17. Nyaga VN, Aerts M, Arbyn M. ANOVA model for network meta-analysis of diagnostic test accuracy data. Stat Methods Med Res (2018) 27(6):1766–84. doi: 10.1177/0962280216669182
18. Paker M, Goldman T, Masalha M, Shlizerman L, Mazzawi S, Ashkenazi D, et al. A comparison of two widely used risk stratification systems for thyroid nodule sonographic evaluation. Isr Med Assoc J (2021) 23(11):714–9.
19. Ahmadi S, Oyekunle T, Jiang X“Sara”, Scheri R, Perkins J, Stang M, et al. A DIRECT COMPARISON OF THE ATA AND TI-RADS ULTRASOUND SCORING SYSTEMS. Endocr Pract (2019) 25(5):413–22. doi: 10.4158/EP-2018-0369
20. Lu K, Wang L, Lai S, Chen Z, Cong S, Huang C, et al. A new discriminant strategy combined with four TIRADS screening procedures increases ultrasound diagnostic accuracy-focusing on “wrong diagnostic” thyroid nodules. Eur Radiol (2023) 33(2):784–96. doi: 10.1007/s00330-022-09126-2
21. Azab EA, Abdelrahman AS, Ibrahim MEA. A practical trial to use Thyroid Imaging Reporting and Data System (TI-RADS) in differentiation between benign and Malignant thyroid nodules. Egypt J Radiol Nucl Med (2019) 50(1):17. doi: 10.1186/s43055-019-0020-0
22. Eidt LB, Nunes de Oliveira C, Lagos YBBD, Solera GLM, Izquierdo R, Meyer El de S, et al. A prospective comparison of ACR-TIRADS and EU-TIRADS in thyroid nodule assessment for FNA-US. Clin Endocrinol (Oxf) (2023) 98(3):415–25. doi: 10.1111/cen.14799
23. Asya O, Yumuşakhuylu AC, Enver N, Gündoğdu Y, Abuzaid G, İncaz S, et al. A single-center multidisciplinary study analyzing thyroid nodule risk stratification by comparing the thyroid imaging reporting and data system (TI-RADS) and American thyroid association (ATA) risk of Malignancy for thyroid nodules. Auris Nasus Larynx (2022) 50(3):410–4. doi: 10.1016/j.anl.2022.08.006
24. Zheng Y, Xu S, Kang H, Zhan W. A single-center retrospective validation study of the American College of Radiology Thyroid imaging reporting and data system. Ultrasound Q (2018) 34(2):77–83. doi: 10.1097/RUQ.0000000000000350
25. Cao J, Huang W, Huang P, Huang Y. ACR TI-RADS and ATA ultrasound classifications are helpful for the management of thyroid nodules located in the isthmus. Clin Hemorheol Microcirc (2022) 80(4):463–71. doi: 10.3233/CH-211304
26. Barbosa TLM, Junior COM, Graf H, Cavalvanti T, Trippia MA, da Silveira Ugino RT, et al. ACR TI-RADS and ATA US scores are helpful for the management of thyroid nodules with indeterminate cytology. BMC Endocr Disord (2019) 19(1):112. doi: 10.1186/s12902-019-0429-5
27. Chen L, Zhan J, Diao X-H, Liu Y-C, Shi Y-X, Chen Y, et al. Additional value of superb microvascular imaging for thyroid nodule classification with the thyroid imaging reporting and data system. Ultrasound Med Biol (2019) 45(8):2040–8. doi: 10.1016/j.ultrasmedbio.2019.05.001
28. Freire da Silva P, Corrêa de Araújo Arcoverde L, de Siqueira Barbosa Arcoverde L, Tenório Wanderley Fernandes Lima G, Paes de Medeiros Lima T, José do Amaral F, et al. Agreement between american and european thyroid imaging, reporting, and data system (TIRADS) in the diagnosis of 473 thyroid nodules from a single center in Brazil. Endocr Pract (2021) 27(11):1108–13. doi: 10.1016/j.eprac.2021.06.008
29. Wu H, Zhang B, Cai G, Li J, Gu X. American College of Radiology thyroid imaging report and data system combined with K-RAS mutation improves the management of cytologically indeterminate thyroid nodules. PloS One (2019) 14(7):e0219383. doi: 10.1371/journal.pone.0219383
30. Biswas S, Sureka B, Kaushal D, Elhence P, Goyal A, Yadav T, et al. American college of radiology thyroid imaging reporting and data system score has high diagnostic value in the diagnosis of Malignant thyroid nodules: A prospective single-center cross-sectional study. Ann Afr Med (2022) 21(4):377–82. doi: 10.4103/aam.aam_123_21
31. Cui Y, Fu C, Si C, Li J, Kang Y, Huang Y, et al. Analysis and comparison of the Malignant thyroid nodules not recommended for biopsy in ACR TIRADS and AI TIRADS with a large sample of surgical series. J Ultrasound Med (2022) 42(6):1225–33. doi: 10.1002/jum.16132
32. Chen X, Kutaiba N, Pearce S, Digby S, Van Gelderen D. Application of Thyroid Imaging Reporting and Data System (TIRADS) guidelines to thyroid nodules with cytopathological correlation and impact on healthcare costs. Intern Med J (2022) 52(8):1366–73. doi: 10.1111/imj.15343
33. Richman DM, Benson CB, Doubilet PM, Wassner AJ, Asch E, Cherella CE, et al. Assessment of american college of radiology thyroid imaging reporting and data system (TI-RADS) for pediatric thyroid nodules. Radiology (2020) 294(2):415–20. doi: 10.1148/radiol.2019191326
34. Lim-Dunham JE, Toslak IE, Reiter MP, Martin B. Assessment of the american college of radiology thyroid imaging reporting and data system for thyroid nodule Malignancy risk stratification in a pediatric population. AJR Am J Roentgenol (2019) 212(1):188–94. doi: 10.2214/AJR.18.20099
35. Zhu Y, Wu H, Huang B, Shen X, Cai G, Gu X. BRAFV600E mutation combined with American College of Radiology thyroid imaging report and data system significantly changes surgical resection rate and risk of Malignancy in thyroid cytopathology practice. Gland Surg (2020) 9(5):1674–84. doi: 10.21037/gs-20-535
36. Yang GCH, Fried KO, Scognamiglio T. Can cytology and the Thyroid Imaging, Reporting, and Data System (TI-RADS) identify noninvasive follicular thyroid neoplasm with papillary-like nuclear features (NIFTP) before surgery? J Am Soc Cytopathol (2020) 9(3):159–65. doi: 10.1016/j.jasc.2020.01.002
37. Yang W, Fananapazir G, LaRoy J, Wilson M, Campbell MJ. Can the American thyroid association, K-tirads and Acr-tirads ultrasound classification systems be used to predict Malignancy in Bethesda category iv nodules? Endocr Pract (2020) 26(9):945–52. doi: 10.4158/EP-2020-0024
38. Dickey MV, Nguyen A, Wiseman SM. Cancer risk estimation using American College of Radiology Thyroid Imaging Reporting and Data System for cytologically indeterminate thyroid nodules. Am J Surg (2022) 224(2):653–6. doi: 10.1016/j.amjsurg.2022.02.061
39. Alqahtani SM, Alanesi SF, Mahmood WS, Moustafa YM, Moharram LM, Alharthi NF, et al. Clinical and ultrasonographic features in cancer risk stratification of indeterminate thyroid nodules. Saudi Med J (2022) 43(5):473–8. doi: 10.15537/smj.2022.43.5.20220045
40. Zhang Z, Lin N. Clinical diagnostic value of American College of Radiology thyroid imaging report and data system in different kinds of thyroid nodules. BMC Endocr Disord (2022) 22(1):145. doi: 10.1186/s12902-022-01053-z
41. Thedinger W, Raman E, Dhingra JK. Comparative study of ACR TI-RADS and ATA 2015 for ultrasound risk stratification of thyroid nodules. Otolaryngol Head Neck Surg (2022) 1 67(1):35–40. doi: 10.1177/01945998211064607
42. Gao L, Xi X, Jiang Y, Yang X, Wang Y, Zhu S, et al. Comparison among TIRADS (ACR TI-RADS and KWAK- TI-RADS) and 2015 ATA Guidelines in the diagnostic efficiency of thyroid nodules. Endocrine (2019) 64(1):90–6. doi: 10.1007/s12020-019-01843-x
43. Wu X-L, Du J-R, Wang H, Jin C-X, Sui G-Q, Yang D-Y, et al. Comparison and preliminary discussion of the reasons for the differences in diagnostic performance and unnecessary FNA biopsies between the ACR TIRADS and 2015 ATA guidelines. Endocrine (2019) 65(1):121–31. doi: 10.1007/s12020-019-01886-0
44. Zhang W-B, Xu W, Fu W-J, He B-L, Liu H, Deng W-F. Comparison of ACR TI-RADS, Kwak TI-RADS, ATA guidelines and KTA/KSThR guidelines in combination with SWE in the diagnosis of thyroid nodules. Clin Hemorheol Microcirc (2021) 78(2):163–74. doi: 10.3233/CH-201021
45. Watkins L, O’Neill G, Young D, McArthur C. Comparison of British Thyroid Association, American College of Radiology TIRADS and Artificial Intelligence TIRADS with histological correlation: diagnostic performance for predicting thyroid Malignancy and unnecessary fine needle aspiration rate. Br J Radiol (1123) 2021:94. doi: 10.1259/bjr.20201444
46. Liu Y, Li X, Yan C, Liu L, Liao Y, Zeng H, et al. Comparison of diagnostic accuracy and utility of artificial intelligence-optimized ACR TI-RADS and original ACR TI-RADS: a multi-center validation study based on 2061 thyroid nodules. Eur Radiol (2022) 32(11):7733–42. doi: 10.1007/s00330-022-08827-y
47. Huh S, Yoon JH, Lee HS, Moon HJ, Park VY, Kwak JY. Comparison of diagnostic performance of the ACR and Kwak TIRADS applying the ACR TIRADS’ size thresholds for FNA. Eur Radiol (2021) 31(7):5243–50. doi: 10.1007/s00330-020-07591-1
48. Shen Y, Liu M, He J, Wu S, Chen M, Wan Y, et al. Comparison of different risk-stratification systems for the diagnosis of benign and Malignant thyroid nodules. Front Oncol (2019) 9:378. doi: 10.3389/fonc.2019.00378
49. Di Fermo F, Sforza N, Rosmarin M, Morosan Allo Y, Parisi C, Santamaria J, et al. Comparison of different systems of ultrasound (US) risk stratification for Malignancy in elderly patients with thyroid nodules. Real World experience. Endocrine (2020) 69(2):331–8. doi: 10.1007/s12020-020-02295-4
50. Yavuz A, Akbudak İ, Üçler R, Özgökçe M, Arslan H, Batur A. Comparison of efficiencies between shear wave elastography, fine-needle aspiration biopsy and american college of radiology thyroid imaging reporting and data system scoring system in determining the Malignity potential of solid thyroid nodules. Ultrasound Q (2020) 37(2):155–60. doi: 10.1097/RUQ.0000000000000531
51. Kang S, Kwon SK, Choi HS, Kim MJ, Park YJ, Park DJ, et al. Comparison of korean vs. American thyroid imaging reporting and data system in Malignancy risk assessment of indeterminate thyroid nodules. Endocrinol Metab (Seoul) (2021) 36(5):1111–20. doi: 10.3803/EnM.2021.1208
52. Shreyamsa M, Mishra A, Ramakant P, Parihar A, Singh KR, Rana C, et al. Comparison of multimodal ultrasound imaging with conventional ultrasound risk stratification systems in presurgical risk stratification of thyroid nodules. Indian J Endocrinol Metab (2020) 24(6):537–42. doi: 10.4103/ijem.IJEM_675_20
53. Middleton WD, Teefey SA, Reading CC, Langer JE, Beland MD, Szabunio MM, et al. Comparison of performance characteristics of American College of Radiology TI-RADS, Korean society of thyroid radiology TIRADS, and American thyroid association guidelines. AJR Am J Roentgenol (2018) 210(5):1148–54. doi: 10.2214/AJR.17.18822
54. Zhang T, Bai BY, Wang SL, Wang JQ, Xue HH. [Comparison of stratification methods for Malignant risk of thyroid nodules]. Lin Chung Er Bi Yan Hou Tou Jing Wai Ke Za Zhi (2019) 33(5):458–60. doi: 10.13201/j.issn.1001-1781.2019.05.018
55. Ha EJ, Shin JH, Na DG, Jung SL, Lee YH, Paik W, et al. Comparison of the diagnostic performance of the modified Korean Thyroid Imaging Reporting and Data System for thyroid Malignancy with three international guidelines. Ultrasonography (2021) 40(4):594–601. doi: 10.14366/usg.21056
56. Gacayan RJ, Kasala R, Puno-Ramos P, Mojica DJ, Castro K. Comparison of the diagnostic performance of ultrasound-based thyroid imaging reporting and data system (TIRADS) classification with american thyroid association (ATA) guidelines in the prediction of thyroid Malignancy in a single tertiary center in Manila, Philippines. J ASEAN Fed Endocr Soc (2021) 36(1):69–75. doi: 10.15605/jafes.036.01.14
57. Liu J, Guo Y, Xiao J, Chen L, Liang Z. Comparison of the efficacy and safety of the American thyroid association guidelines and American college of radiology TI-RADS. Endocr Pract (2021) 27(7):661–7. doi: 10.1016/j.eprac.2020.11.013
58. Qi T-Y, Chen X, Liu H, Mao L, Chen J, He B-L, et al. Comparison of thyroid nodule FNA rates recommended by ACR TI-RADS, Kwak TI-RADS and ATA guidelines. Eur J Radiol (2022) 148:110152. doi: 10.1016/j.ejrad.2022.110152
59. Zhang W-B, Xu H-X, Zhang Y-F, Guo L-H, Xu S-H, Zhao C-K, et al. Comparisons of ACR TI-RADS, ATA guidelines, Kwak TI-RADS, and KTA/KSThR guidelines in Malignancy risk stratification of thyroid nodules. Clin Hemorheol Microcirc (2020) 75(2):219–32. doi: 10.3233/CH-190778
60. Abolhasani Foroughi A, Mokhtari M, Heidari E, Nazeri M, Rastgouyan H, Babaei A. Concordance between TIRADS and cytology in thyroid nodule. Iran J Otorhinolaryngol (2022) 34(125):295–302. doi: 10.22038/IJORL.2022.57663.2984
61. Huang Y, Hong Y, Xu W, Song K, Huang P. Contrast-enhanced ultrasound improves the accuracy of the ACR TI-RADS in the diagnosis of thyroid nodules located in the isthmus. Ultraschall Med (2022) 43(6):599–607. doi: 10.1055/a-1543-6033
62. Baz AAA, Mohamed AHI, El-Esawy YFG, El-kaffas KH. Conventional ultrasound, color Doppler, TI-RADS, and shear wave elastography for thyroid nodule differentiation: a study of efficacy compared with the histopathology results. Egyptian J Radiol Nucl Med (2021) 52(1):103. doi: 10.1186/s43055-021-00474-5
63. Kim GR, Lee E, Kim HR, Yoon JH, Park VY, Kwak JY. Convolutional neural network to stratify the Malignancy risk of thyroid nodules: diagnostic performance compared with the american college of radiology thyroid imaging reporting and data system implemented by experienced radiologists. AJNR Am J Neuroradiol (2021) 42(8):1513–9. doi: 10.3174/ajnr.A7149
64. Wu G-G, Lv W-Z, Yin R, Xu J-W, Yan Y-J, Chen R-X, et al. Deep learning based on ACR TI-RADS can improve the differential diagnosis of thyroid nodules. Front Oncol (2021) 11:575166. doi: 10.3389/fonc.2021.575166
65. Tan L, Tan YS, Tan S. Diagnostic accuracy and ability to reduce unnecessary FNAC: A comparison between four Thyroid Imaging Reporting Data System (TI-RADS) versions. Clin Imaging (2020) 65:133–7. doi: 10.1016/j.clinimag.2020.04.029
66. Orhan Soylemez UP, Gunduz N. Diagnostic accuracy of five different classification systems for thyroid nodules: A prospective, comparative study. J Ultrasound Med (2022) 41(5):1125–36. doi: 10.1002/jum.15802
67. Sharma P, el fatairy K, Gandhi D, Sawhney H, Osman M, Kochar P, et al. Diagnostic performance of ACR-TIRADS in differentiating benign from Malignant thyroid nodules in patients undergoing fine-needle aspiration biopsy: comparative study based on five international guidelines for management of thyroid nodules. J Endocrinol Metab (2021) 11:69–75. doi: 10.14740/jem735
68. Behbahaninia M, Nabahati M, Moazezi Z, Mehraeen R, Shirafkan H. Diagnostic performance of ACR-TIRADS, Korean TIRADS, and American Thyroid Association guidelines for risk stratification of thyroid nodules: a prospective study. J Ultrasound (2022) 25(4):887–94. doi: 10.1007/s40477-022-00669-0
69. Chen Z, Du Y, Cheng L, Zhang Y, Zheng S, Li R, et al. Diagnostic performance of simplified TI-RADS for Malignant thyroid nodules: comparison with 2017 ACR-TI-RADS and 2020 C-TI-RADS. Cancer Imaging (2022) 22(1):41. doi: 10.1186/s40644-022-00478-y
70. Yang J, Sun Y, Li X, Zhao Y, Han X, Chen G, et al. Diagnostic performance of six ultrasound-based risk stratification systems in thyroid follicular neoplasm: A retrospective multi-center study. Front Oncol (2022) 12:1013410. doi: 10.3389/fonc.2022.1013410
71. Ha EJ, Na DG, Moon W-J, Lee YH, Choi N. Diagnostic performance of ultrasound-based risk-stratification systems for thyroid nodules: comparison of the 2015 American thyroid association guidelines with the 2016 Korean thyroid association/Korean society of thyroid radiology and 2017 American college of radiology guidelines. Thyroid (2018) 28(11):1532–7. doi: 10.1089/thy.2018.0094
72. Zhu H, Yang Y, Wu S, Chen K, Luo H, Huang J. Diagnostic performance of US-based FNAB criteria of the 2020 Chinese guideline for Malignant thyroid nodules: comparison with the 2017 American College of Radiology guideline, the 2015 American Thyroid Association guideline, and the 2016 Korean Thyroid Association guideline. Quant Imaging Med Surg (2021) 11(8):3604–18. doi: 10.21037/qims-20-1365
73. Hekimsoy İ, Öztürk E, Ertan Y, Orman MN, Kavukçu G, Özgen AG, et al. Diagnostic performance rates of the ACR-TIRADS and EU-TIRADS based on histopathological evidence. Diagn Interv Radiol (2021) 27(4):511–8. doi: 10.5152/dir.2021.20813
74. Huh S, Lee HS, Yoon J, Kim E-K, Moon HJ, Yoon JH, et al. Diagnostic performances and unnecessary US-FNA rates of various TIRADS after application of equal size thresholds. Sci Rep (2020) 10(1):10632. doi: 10.1038/s41598-020-67543-z
75. Zhang C, Huang Y-Q, Liu Z-L. Diagnostic value of 2017 ACR Thyroid Imaging Reporting and Data System (TI-RADS) combined with fine needle aspiration biopsy in thyroid nodules. J Xray Sci Technol (2021) 29(6):1113–22. doi: 10.3233/XST-210949
76. Xiao J, Xiao Q, Cong W, Li T, Ding S, Shao C, et al. Discriminating Malignancy in thyroid nodules: the nomogram versus the kwak and ACR TI-RADS. Otolaryngol Head Neck Surg (2020) 163(6):1156–65. doi: 10.1177/0194599820939071
77. Durmaz F, Ozgokce M, Ozkacmaz S, Dundar I, Alay M, Goya C, et al. Efficacy of shear wave elastography in Malignity assessment of thyroid nodules with atypia of undetermined significance and comparison with TI-RADS. Ann Clin Anal Med (2022) 13(2):215–9. doi: 10.4328/ACAM.20949
78. Baş H, Üstüner E, Kula S, Konca C, Demirer S, Elhan AH. Elastography and doppler may bring a new perspective to TIRADS, altering conventional ultrasonography dominance. Acad Radiol (2022) 29(3):e25–38. doi: 10.1016/j.acra.2021.02.011
79. Xi X, Wang Y, Gao L, Jiang Y, Liang Z, Ren X, et al. Establishment of an ultrasound Malignancy risk stratification model for thyroid nodules larger than 4 cm. Front Oncol (2021) 11:592927. doi: 10.3389/fonc.2021.592927
80. Qi Q, Zhou A, Guo S, Huang X, Chen S, Li Y, et al. Explore the diagnostic efficiency of chinese thyroid imaging reporting and data systems by comparing with the other four systems (ACR TI-RADS, kwak-TIRADS, KSThR-TIRADS, and EU-TIRADS): A single-center study. Front Endocrinol (Lausanne) (2021) 12:763897. doi: 10.3389/fendo.2021.763897
81. Scappaticcio L, Maiorino MI, Iorio S, Docimo G, Longo M, Grandone A, et al. Exploring the performance of ultrasound risk stratification systems in thyroid nodules of pediatric patients. Cancers (Basel) (2021) 13(21):5304. doi: 10.3390/cancers13215304
82. Piccardo A, Fiz F, Bottoni G, De Luca C, Massollo M, Catrambone U, et al. Facing thyroid nodules in paediatric patients previously treated with radiotherapy for non-thyroidal cancers: are adult ultrasound risk stratification systems reliable? Cancers (Basel) (2021) 13(18):4692. doi: 10.3390/cancers13184692
83. Ruan J-L, Yang H-Y, Liu R-B, Liang M, Han P, Xu X-L, et al. Fine needle aspiration biopsy indications for thyroid nodules: compare a point-based risk stratification system with a pattern-based risk stratification system. Eur Radiol (2019) 29(9):4871–8. doi: 10.1007/s00330-018-5992-z
84. Wang B, Wan Z, Li C, Zhang M, Shi Y, Miao X, et al. Identification of benign and Malignant thyroid nodules based on dynamic AI ultrasound intelligent auxiliary diagnosis system. Front Endocrinol (Lausanne) (2022) 13:1018321. doi: 10.3389/fendo.2022.1018321
85. Popova NM, Radzina M, Prieditis P, Liepa M, Rauda M, Stepanovs K. Impact of the hypoechogenicity criteria on thyroid nodule Malignancy risk stratification performance by different TIRADS systems. Cancers (Basel) (2021) 13(21):5581. doi: 10.3390/cancers13215581
86. Phuttharak W, Boonrod A, Klungboonkrong V, Witsawapaisan T. Interrater reliability of various thyroid imaging reporting and data system (TIRADS) classifications for differentiating benign from Malignant thyroid nodules. Asian Pac J Cancer Prev (2019) 20(4):1283–8. doi: 10.31557/APJCP.2019.20.4.1283
87. Li N, He Y, Chang R. Is Chinese Thyroid Imaging Reporting and Data Systems superior to American College of Radiology or American Thyroid Association guidelines for consistency and efficacy in the diagnosis of thyroid cancer? Chin Med J (Engl) (2022) 135(15):1886–8. doi: 10.1097/CM9.0000000000002061
88. Chen L, Chen M, Li Q, Kumar V, Duan Y, Wu KA, et al. Machine learning-assisted diagnostic system for indeterminate thyroid nodules. Ultrasound Med Biol (2022) 48(8):1547–54. doi: 10.1016/j.ultrasmedbio.2022.03.020
89. Peng J-Y, Pan F-S, Wang W, Wang Z, Shan Q-Y, Lin J-H, et al. Malignancy risk stratification and FNA recommendations for thyroid nodules: A comparison of ACR TI-RADS, AACE/ACE/AME and ATA guidelines. Am J Otolaryngol (2020) 41(6):102625. doi: 10.1016/j.amjoto.2020.102625
90. Nabahati M, Moazezi Z. Malignancy risk stratification of thyroid nodules smaller than 10 mm with ACR-TIRADS, K-TIRADS, and ATA-2015 guidelines: a prospective study. Egyptian J Radiol Nucl Med (2022) 53(1):128. doi: 10.1186/s43055-022-00802-3
91. Buda M, Wildman-Tobriner B, Hoang JK, Thayer D, Tessler FN, Middleton WD, et al. Management of thyroid nodules seen on US images: deep learning may match performance of radiologists. Radiology (2019) 292(3):695–701. doi: 10.1148/radiol.2019181343
92. Yoon JH, Lee HS, Kim E-K, Moon HJ, Park VY, Kwak JY. Pattern-based vs. score-based guidelines using ultrasound features have different strengths in risk stratification of thyroid nodules. Eur Radiol (2020) 30(7):3793–802. doi: 10.1007/s00330-020-06722-y
93. Magri F, Chytiris S, Croce L, Molteni M, Bendotti G, Gruosso G, et al. Performance of the ACR TI-RADS and EU TI-RADS scoring systems in the diagnostic work-up of thyroid nodules in a real-life series using histology as reference standard. Eur J Endocrinol (2020) 183(5):521–8. doi: 10.1530/EJE-20-0682
94. Li X, Gao F, Li F, Han X-X, Shao S-H, Yao M-H, et al. Qualitative analysis of contrast-enhanced ultrasound in the diagnosis of small, TR3-5 benign and Malignant thyroid nodules measuring ≤1 cm. Br J Radiol (2020) 93(1111):20190923. doi: 10.1259/bjr.20190923
95. Grani G, Lamartina L, Ascoli V, Bosco D, Biffoni M, Giacomelli L, et al. Reducing the number of unnecessary thyroid biopsies while improving diagnostic accuracy: toward the “Right” TIRADS. J Clin Endocrinol Metab (2019) 104(1):95–102. doi: 10.1210/jc.2018-01674
96. Hoang JK, Middleton WD, Farjat AE, Langer JE, Reading CC, Teefey SA, et al. Reduction in thyroid nodule biopsies and improved accuracy with American college of radiology thyroid imaging reporting and data system. Radiology (2018) 287(1):185–93. doi: 10.1148/radiol.2018172572
97. Felder GJ, Naeem M, Shady W, Shetty AS, Fraum TJ, Itani M. Risk stratification of 18F-fluorodeoxyglucose-avid thyroid nodules based on ACR thyroid imaging reporting and data system. J Am Coll Radiol (2021) 18(3 Pt A):388–94. doi: 10.1016/j.jacr.2020.08.021
98. Clark TJ, McKinney K, Jensen A, Patel NU. Risk threshold algorithm for thyroid nodule management demonstrates increased specificity and diagnostic accuracy as compared with American college of radiology thyroid imaging, reporting and data system; society of radiologists in ultrasound; and American thyroid association management guidelines. Ultrasound Q (2019) 35(3):224–7. doi: 10.1097/RUQ.0000000000000420
99. Petersen M, Schenke SA, Firla J, Croner RS, Kreissl MC. Shear wave elastography and thyroid imaging reporting and data system (TIRADS) for the risk stratification of thyroid nodules-results of a prospective study. Diagnostics (Basel) (2022) 12(1):109. doi: 10.3390/diagnostics12010109
100. Yoon SJ, Na DG, Gwon HY, Paik W, Kim WJ, Song JS, et al. Similarities and differences between thyroid imaging reporting and data systems. AJR Am J Roentgenol (2019) 213(2):W76–84. doi: 10.2214/AJR.18.20510
101. Grani G, Brenta G, Trimboli P, Falcone R, Ramundo V, Maranghi M, et al. Sonographic risk stratification systems for thyroid nodules as rule-out tests in older adults. Cancers (Basel) (2020) 12(9):2458. doi: 10.3390/cancers12092458
102. Muhammed AS, Singh R, Suhaimi SNA, Latar NHM. Surgeon performed ultrasound in assessing thyroid nodule using American college of radiology tirads and total Malignancy score (TMS): A validation cross sectional study. IIUM Med J Malaysia (2021) 20(3). doi: 10.31436/imjm.v20i3.1832
103. Zhang W-B, Li J-J, Chen X-Y, He B-L, Shen R-H, Liu H, et al. SWE combined with ACR TI-RADS categories for Malignancy risk stratification of thyroid nodules with indeterminate FNA cytology. Clin Hemorheol Microcirc (2020) 76(3):381–90. doi: 10.3233/CH-200893
104. Zhu J, Li X, Wei X, Yang X, Zhao J, Zhang S, et al. The application value of modified thyroid imaging report and data system in diagnosing medullary thyroid carcinoma. Cancer Med (2019) 8(7):3389–400. doi: 10.1002/cam4.2217
105. Sakthisankari S, Vidhyalakshmi S, Shanthakumari S, Devanand B, Nagul U. The combination of ACR-Thyroid Imaging Reporting and Data system and The Bethesda System for Reporting Thyroid Cytopathology in the evaluation of thyroid nodules-An institutional experience. Cytopathology (2021) 32(4):472–81. doi: 10.1111/cyt.12967
106. Chen F, Sun Y, Chen G, Luo Y, Xue G, Luo K, et al. The diagnostic efficacy of the american college of radiology (ACR) thyroid imaging report and data system (TI-RADS) and the American thyroid association (ATA) risk stratification systems for thyroid nodules. Comput Math Methods Med (2022) 2022:9995962. doi: 10.1155/2022/9995962
107. Mao S, Zhao LP, Li XH, Sun YF, Su H, Zhang Y, et al. [The diagnostic performance of 2020 Chinese Ultrasound Thyroid Imaging Reporting and Data System in thyroid nodules]. Zhonghua Yi Xue Za Zhi (2021) 101(45):3748–53. doi: 10.3760/cma.j.cn112137-20210401-00799
108. Bora Makal G, Aslan A. The diagnostic value of the American college of radiology thyroid imaging reporting and data system classification and shear-wave elastography for the differentiation of thyroid nodules. Ultrasound Med Biol (2021) 47(5):1227–34. doi: 10.1016/j.ultrasmedbio.2021.01.023
109. Xu X, He XL, Guo LL. [The diagnostic value of the maximum value of Young’s modulus of shear-wave elastography and ACR TI-RADS for thyroid nodules]. Lin Chung Er Bi Yan Hou Tou Jing Wai Ke Za Zhi (2019) 33(8):764–7. doi: 10.13201/j.issn.1001-1781.2019.08.020
110. Grimmichová T, Pačesová P, Srbová L, Vrbíková J, Havrdová T, Hill M. The gold standard of thyroid nodule examination? Prospective validation of the ACR TI-RADS in a secondary referral center. Physiol Res (2020) 69(Suppl 2):S329–37. doi: 10.33549/physiolres.934515
111. Araruna Bezerra de Melo R, Menis F, Calsavara VF, Stefanini FS, Novaes T, Saieg M. The impact of the use of the ACR-TIRADS as a screening tool for thyroid nodules in a cancer center. Diagn Cytopathol (2022) 50(1):18–23. doi: 10.1002/dc.24904
112. Wei Q, Zeng S-E, Wang L-P, Yan Y-J, Wang T, Xu J-W, et al. The value of S-Detect in improving the diagnostic performance of radiologists for the differential diagnosis of thyroid nodules. Med Ultrason (2020) 22(4):415–23. doi: 10.11152/mu-2501
113. Grimmichova T, Pacesova P, Hill M, Pekova B, Vankova M, Moravcova J, et al. Thyroid cancer detection in a routine clinical setting: performance of ACR TI-RADS, FNAC, and molecular testing in prospective cohort study. Biomedicines (2022) 10(5):954. doi: 10.3390/biomedicines10050954
114. Yang J, Page LC, Wagner L, Wildman-Tobriner B, Bisset L, Frush D, et al. Thyroid nodules on ultrasound in children and young adults: comparison of diagnostic performance of radiologists’ Impressions, ACR TI-RADS, and a deep learning algorithm. AJR Am J Roentgenol (2023) 220(3):408–17. doi: 10.2214/AJR.22.28231
115. Borlea A, Borcan F, Sporea I, Dehelean CA, Negrea R, Cotoi L, et al. TI-RADS diagnostic performance: which algorithm is superior and how elastography and 4D vascularity improve the Malignancy risk assessment. Diagnostics (Basel) (2020) 10(4):180. doi: 10.3390/diagnostics10040180
116. Pei S, Zhang B, Cong S, Liu J, Wu S, Dong Y, et al. Ultrasound real-time tissue elastography improves the diagnostic performance of the ACR thyroid imaging reporting and data system in differentiating Malignant from benign thyroid nodules: A summary of 1525 thyroid nodules. Int J Endocrinol (2020) 2020:1749351. doi: 10.1155/2020/1749351
117. Gao L, Xi X, Wang J, Yang X, Wang Y, Zhu S, et al. Ultrasound risk evaluation of thyroid nodules that are “unspecified” in the 2015 American Thyroid Association management guidelines: A retrospective study. Med (Baltimore) (2018) 97(52):e13914. doi: 10.1097/MD.0000000000013914
118. Seminati D, Capitoli G, Leni D, Fior D, Vacirca F, Di Bella C, et al. Use of diagnostic criteria from ACR and EU-TIRADS systems to improve the performance of cytology in thyroid nodule triage. Cancers (Basel) (2021) 13(21):5439. doi: 10.3390/cancers13215439
119. Strieder DL, Cristo AP, Zanella AB, Faccin CS, Farenzena M, Graudenz MS, et al. Using an ultrasonography risk stratification system to enhance the thyroid fine needle aspiration performance. Eur J Radiol (2022) 150:110244. doi: 10.1016/j.ejrad.2022.110244
120. Chen Q, Lin M, Wu S. Validating and comparing C-TIRADS, K-TIRADS and ACR-TIRADS in stratifying the Malignancy risk of thyroid nodules. Front Endocrinol (Lausanne) (2022) 13:899575. doi: 10.3389/fendo.2022.899575
121. Xu T, Wu Y, Wu R-X, Zhang Y-Z, Gu J-Y, Ye X-H, et al. Validation and comparison of three newly-released Thyroid Imaging Reporting and Data Systems for cancer risk determination. Endocrine (2019) 64(2):299–307. doi: 10.1007/s12020-018-1817-8
122. Merhav G, Zolotov S, Mahagneh A, Malchin L, Mekel M, Beck-Razi N. Validation of TIRADS ACR risk assessment of thyroid nodules in comparison to the ATA guidelines. J Clin Imaging Sci (2021) 11:37. doi: 10.25259/JCIS_99_2021
123. Ha SM, Na DG, Kim J-H, Kim SC, Baek JH. Validation of web-based thyroid imaging reporting and data system in atypia or follicular lesion of undetermined significance thyroid nodules. Head Neck (2019) 41(7):2215–24. doi: 10.1002/hed.25677
124. Daniels KE, Shaffer AD, Garbin S, Squires JH, Vaughan KG, Viswanathan P, et al. Validity of the American college of radiology thyroid imaging reporting and data system in children. Laryngoscope (2022) 133(9):2394–401. doi: 10.1002/lary.30425
125. Trimboli P, Ngu R, Royer B, Giovanella L, Bigorgne C, Simo R, et al. A multicentre validation study for the EU-TIRADS using histological diagnosis as a gold standard. Clin Endocrinol (Oxf) (2019) 91(2):340–7. doi: 10.1111/cen.13997
126. Skowrońska A, Milczarek-Banach J, Wiechno W, Chudziński W, Żach M, Mazurkiewicz M, et al. Accuracy of the European Thyroid Imaging Reporting and Data System (EU-TIRADS) in the valuation of thyroid nodule Malignancy in reference to the post-surgery histological results. Pol J Radiol (2018) 83:e579–86. doi: 10.5114/pjr.2018.81556
127. Chambara N, Lo X, Chow TCM, Lai CMS, Liu SYW, Ying M. Combined shear wave elastography and EU TIRADS in differentiating Malignant and benign thyroid nodules. Cancers (Basel) (2022) 14(22):5521. doi: 10.3390/cancers14225521
128. Sharma P, Elfatairy K, Gandhi D, Sawhney H, Osman M, Kochar PS, et al. Diagnostic performance of ACR-TIRADS in differentiating benign from Malignant thyroid nodules in patients undergoing fine-needle aspiration biopsy: comparative study based on five international guidelines for management of thyroid nodules. J Endocrinol Metab (2021) 11(3–4):69–75. doi: 10.14740/jem.v11i3-4.735
129. Chambara N, Liu SYW, Lo X, Ying M. Diagnostic value of angioPLUS microvascular imaging in thyroid nodule diagnosis using quantitative and qualitative vascularity grading. Biomedicines (2022) 10(7):1554. doi: 10.3390/biomedicines10071554
130. Kovatcheva RD, Shinkov AD, Dimitrova ID, Ivanova RB, Vidinov KN, Ivanova RS. Evaluation of the diagnostic performance of EU-TIRADS in discriminating benign from Malignant thyroid nodules: A prospective study in one referral center. Eur Thyroid J (2021) 9(6):304–12. doi: 10.1159/000507575
131. Dobruch-Sobczak K, Adamczewski Z, Szczepanek-Parulska E, Migda B, Woliński K, Krauze A, et al. Histopathological verification of the diagnostic performance of the EU-TIRADS classification of thyroid nodules-results of a multicenter study performed in a previously iodine-deficient region. J Clin Med (2019) 8(11):1781. doi: 10.3390/jcm8111781
132. Słowińska-Klencka D, Klencki M, Duda-Szymańska J, Popowicz B. Optimization of the management of category III thyroid nodules using repeat FNA and TIRADS. Cancers (Basel) (2022) 14(18):4489. doi: 10.3390/cancers14184489
133. Szczepanek-Parulska E, Wolinski K, Dobruch-Sobczak K, Antosik P, Ostalowska A, Krauze A, et al. S-detect software vs. EU-TIRADS classification: A dual-center validation of diagnostic performance in differentiation of thyroid nodules. J Clin Med (2020) 9(8):2495. doi: 10.3390/jcm9082495
134. De Fiori E, Lanza C, Carriero S, Tettamanzi F, Frassoni S, Bagnardi V, et al. The european institute of oncology thyroid imaging reporting and data system for classification of thyroid nodules: A prospective study. J Clin Med (2022) 11(11):3238. doi: 10.3390/jcm11113238
135. Yeste Fernández D, Vega Amenabar E, Coma Muñoz A, Arciniegas Vallejo L, Clemente León M, Planes-Conangla M, et al. Ultrasound criteria (EU-TIRADS) to identify thyroid nodule Malignancy risk in adolescents. Correlation with cyto-histological findings. Endocrinol Diabetes Nutr (Engl Ed) (2021) S2530-0164(21)00078-1. doi: 10.1016/j.endinu.2020.11.009
136. Bukasa-Kakamba J, Bayauli P, Sabbah N, Bidingija J, Atoot A, Mbunga B, et al. Ultrasound performance using the EU-TIRADS score in the diagnosis of thyroid cancer in Congolese hospitals. Sci Rep (2022) 12(1):18442. doi: 10.1038/s41598-022-22954-y
137. Jin Z, Zhu Y, Lei Y, Yu X, Jiang N, Gao Y, et al. Clinical application of C-TIRADS category and contrast-enhanced ultrasound in differential diagnosis of solid thyroid nodules measuring ≥1 cm. Med Sci Monit (2022) 28:e936368. doi: 10.12659/MSM.936368
138. Gao X-Q, Ma Y, Peng X-S, Wang L-L, Li H-X, Zheng X-L, et al. Diagnostic performance of C-TIRADS combined with SWE for the diagnosis of thyroid nodules. Front Endocrinol (Lausanne) (2022) 13:939303. doi: 10.3389/fendo.2022.939303
139. Zhu T, Chen J, Zhou Z, Ma X, Huang Y. Differentiation of thyroid nodules (C-TIRADS 4) by combining contrast-enhanced ultrasound diagnosis model with Chinese thyroid imaging reporting and data system. Front Oncol (2022) 12:840819. doi: 10.3389/fonc.2022.840819
140. Cao H, Fan Q, Zhuo S, Qi T, Sun H, Rong X, et al. The value of chinese thyroid imaging report and data system combined with contrast-enhanced ultrasound scoring in differential diagnosis of benign and Malignant thyroid nodules. J Ultrasound Med (2022) 41(7):1753–61. doi: 10.1002/jum.15858
141. Zhou J, Song Y, Zhan W, Wei X, Zhang S, Zhang R, et al. Thyroid imaging reporting and data system (TIRADS) for ultrasound features of nodules: multicentric retrospective study in China. Endocrine (2021) 72(1):157–70. doi: 10.1007/s12020-020-02442-x
142. Cheng H, Zhuo S-S, Rong X, Qi T-Y, Sun H-G, Xiao X, et al. Value of contrast-enhanced ultrasound in adjusting the classification of Chinese-TIRADS 4 nodules. Int J Endocrinol (2022) 2022:5623919. doi: 10.1155/2022/5623919
143. Xia S, Yao J, Zhou W, Dong Y, Xu S, Zhou J, et al. A computer-aided diagnosing system in the evaluation of thyroid nodules-experience in a specialized thyroid center. World J Surg Oncol (2019) 17(1):210. doi: 10.1186/s12957-019-1752-z
144. Barczyński M, Stopa-Barczyńska M, Wojtczak B, Czarniecka A, Konturek A. Clinical validation of S-DetectTM mode in semi-automated ultrasound classification of thyroid lesions in surgical office. Gland Surg (2020) 9(Suppl 2):S77–85. doi: 10.21037/gs.2019.12.23
145. Yoo YJ, Ha EJ, Cho YJ, Kim HL, Han M, Kang SY. Computer-aided diagnosis of thyroid nodules via ultrasonography: initial clinical experience. Korean J Radiol (2018) 19(4):665–72. doi: 10.3348/kjr.2018.19.4.665
146. Huang P, Zheng B, Li M, Xu L, Rabbani S, Mayet AM, et al. The diagnostic value of artificial intelligence ultrasound S-detect technology for thyroid nodules. Comput Intell Neurosci (2022) 2022:3656572. doi: 10.1155/2022/3656572
Keywords: thyroid imaging reporting and data system, Eu-TIRADS, ACR TIRADS, C-TIRADS, S-Detect, diagnostic performance, network meta-analysis
Citation: Yang L, Li C, Chen Z, He S, Wang Z and Liu J (2023) Diagnostic efficiency among Eu-/C-/ACR-TIRADS and S-Detect for thyroid nodules: a systematic review and network meta-analysis. Front. Endocrinol. 14:1227339. doi: 10.3389/fendo.2023.1227339
Received: 23 May 2023; Accepted: 16 August 2023;
Published: 31 August 2023.
Edited by:
Dan Li, Sun Yat-sen Memorial Hospital, ChinaReviewed by:
Yuxuan Qiu, West China Hospital, Sichuan University, ChinaCopyright © 2023 Yang, Li, Chen, He, Wang and Liu. This is an open-access article distributed under the terms of the Creative Commons Attribution License (CC BY). The use, distribution or reproduction in other forums is permitted, provided the original author(s) and the copyright owner(s) are credited and that the original publication in this journal is cited, in accordance with accepted academic practice. No use, distribution or reproduction is permitted which does not comply with these terms.
*Correspondence: Zhiyuan Wang, d2FuZ3poaXl1YW5AaG5jYS5vcmcuY24=; Jun Liu, anVubGl1MTIzQGNzdS5lZHUuY24=
†These authors have contributed equally to this work
Disclaimer: All claims expressed in this article are solely those of the authors and do not necessarily represent those of their affiliated organizations, or those of the publisher, the editors and the reviewers. Any product that may be evaluated in this article or claim that may be made by its manufacturer is not guaranteed or endorsed by the publisher.
Research integrity at Frontiers
Learn more about the work of our research integrity team to safeguard the quality of each article we publish.