- Department of Anesthesiology, Shengjing Hospital of China Medical University, Shenyang, Liaoning, China
Background: Pancreatic adenocarcinoma (PAAD) is characterized by lower immunogenicity with a poor response rate to immune checkpoint inhibitors (ICIs) and exhibits the poorest prognosis of all solid tumors, which results in the highest tumor-related mortality among malignancies. However, the underlying mechanisms are poorly understood. In addition, diverse carbohydrate sulfotransferases (CHSTs), which are involved in the sulfation process of these structures, play an important role in the metastatic spread of tumor cells. Aberrant glycosylation is beginning to emerge as an influencing factor in tumor immunity and immunotherapy. Therefore, it might serve as a biomarker of the immunotherapeutic response in tumors. The purpose of the study was to evaluate the role of CHST12 in PAAD prognosis and its relevance to the immunotherapeutic response.
Methods: A comprehensive investigation of the interactions between CHST12 expression and the immune microenvironment as well as the clinical significance of CHST12 in PAAD was conducted. Data derived from the Cancer Genome Atlas (TCGA) database were analyzed using univariate and multivariate approaches, the Tumor Immune Estimation Resource (TIMER), and Tumor Immune Dysfunction and Exclusion (TIDE) algorithms. Publicly available datasets were analyzed in this study. These data can be found on websites such as http://www.xiantao.love and https://www.proteinatlas.org. An assessment of the predictive value of CHST12 for PAAD prognosis was conducted using univariate and multivariate Cox regression analysis, Kaplan–Meier analysis, and nomograms. The TIMER algorithm calculates the proportions of six types of immune cells. The TIDE algorithm was used to indicate the characteristics of tumors that respond to ICI therapy.
Results: The mRNA and protein levels of CHST12 showed the opposite trend. CHST12 mRNA expression was significantly upregulated in PAAD. According to Cox regression analysis, CHST12 RNA expression acts as a protective factor for overall survival [hazard ratio (HR), 0.617, P < 0.04]. Functional annotation indicated that CHST12-associated differentially expressed genes (DEGs) were related to the signaling activity of receptor tyrosine kinases and the regulation of ubiquitin-protein transferase. These are usually involved in tumor development and may be related to the treatment responses of immune checkpoint inhibitors (ICIs). There was significantly higher CHST12 mRNA expression in PAAD samples than in non-malignant samples.
Conclusions: In PAAD, elevated CHST12 mRNA expression might regulate immune cell infiltration into the tumor microenvironment (TME) and may predict clinical outcomes.
1 Introduction
Pancreatic adenocarcinoma, commonly referred to as pancreatic cancer, is a type of cancer that begins in the pancreas, an organ located deep within the abdomen (1, 2). The pancreas plays a crucial role in digestion and blood sugar regulation by producing enzymes and hormones, such as insulin and glucagon (3, 4). PAAD is a highly aggressive malignancy, exhibiting the poorest clinical outcome of all solid tumors. Generally, when patients come to the hospital, they are diagnosed with advanced PAAD. It is characterized by lower immunogenicity, with a lower number of predicted neoantigens, low immune infiltration, and poor response rates to ICI therapy, with a median survival of 6 months and growing incidence rates worldwide (5–8). The low tumor-infiltrating immune cell microenvironment features of PAAD consist of cancer cells, fibroblasts, myofibroblasts, endothelial cells, tumor-infiltrating lymphocytes (TILs), and extracellular matrix.
The majority of pancreatic cancers are adenocarcinomas, which start in the cells that line the ducts of the pancreas (9, 10). There are other less common types of pancreatic cancer, such as neuroendocrine tumors and acinar cell carcinomas, leading to drug resistance and great inertia in the response rates to immunotherapy (11–13). Therefore, it is particularly difficult to treat PAAD. Specific targeted therapies such as EGFR-targeted therapy confer a significant survival benefit in a minority of PAAD patients who are wild-type KRAS patients (14, 15). Approximately 90% of pancreatic cancers harbor oncogenic KRAS mutations (16, 17). With the improvement in many advanced medical diagnostic technologies, such as endoscopic ultrasound, the early diagnosis rate of PAAD has been greatly improved (18–20). However, given the limited effectiveness of current treatments for PAAD, new promising biomarkers are urgently needed to improve the prognosis and treatment of PAAD.
As a fundamental and prominent post-translational modification of proteins, the involvement of glycosylation in cellular functions includes transformation, adhesion, cell growth, differentiation, and tumor immune surveillance (21, 22). Abnormal glycosylation has been implicated as a crucial mechanism that triggers tumor progression (23, 24). Dysregulation in glycosylation can affect the function, sensitivity and expression of cell-surface receptors. These features make glycosylation a critical molecular event in cancer pathology (25). Glycoproteins are currently the most commonly used clinical tumor biomarkers (e.g., the AFP test for liver cancer, CA125 test for ovarian cancer, CEA, CA199 test for colon cancer, and PSA test for prostate cancer) (26, 27). Subsequently, the role of aberrant glycosylation influences tumorigenesis, which affects growth, immune surveillance, and immunotherapy.
Many recent publications have indicated that glycosylation can lead to an improvement in the immunotherapeutic effect and confer better treatment outcomes in cancer (28–30). As such, further elucidating the molecular mechanisms and consequently promoting cancer immune evasion is urgently required (31). As one of the important components of the tumor microenvironment, proteoglycans promote the progression of cancers, such as lung cancer, pancreatic cancer, and GBM (32–34). Multiple family members are implicated in tumor occurrence and development (35). The current evidence suggests that carbohydrate sulfotransferase 12 (CHST12) is a cancer-related enzyme and is a potential biomarker in some tumor subtypes. For instance, GBMs with high CHST12 expression have a reduced survival rate and positive associations with KI67 expression (36). Qiang Ren et al. found that CHST12 was expressed at a significantly higher level in pancreatic tumors, highlighting the potential diagnostic utility of the CHST12 expression profile in pancreatic cancer (37, 38). However, the specific functions and role of CHST12 in the prognosis of pancreatic cancer and the regulation of TME remain a mystery.
Here, we explored CHST12-related biological processes and CHST12-enhanced pathways to understand its functions in PAAD. The prognostic model based on univariate and multivariate Cox regression analysis revealed an independent prognosticator characteristic. Additionally, we used the TIDE algorithm to indicate the characteristics of CHST12 as a predictor for ICI therapy efficacy. The present study may help develop CHST12 as a prognostic biomarker and predict the efficacy of the immunotherapy treatment of PAAD patients.
2 Methods
2.1 Data processing
The level 3 HTSeq-FPKM (Fragments Per Kilobase per Million) sequencing RNA expression values were downloaded from the GTEx and TCGA datasets and log2-transformed [log2 (FPKM+1)]. The corresponding clinicopathological parameters were then obtained, including 171 normal tissue samples and 179 PAAD tumor samples. These databases are publicly open access. This study was approved by the Ethics Committee of the Shengjing Hospital of China Medical University (Ethical Approval Number: 2023PS700K).
2.2 Differentially expressed genes in PAAD
High-expression and low-expression groups of CHST12 were determined by the median expression from 178 PAAD patients. R (Version 3.6.3) was used to create plots with the ggplot2 package comparing the DEGs between the two groups using chi-square or Fisher exact tests (39). The following significance symbols were used: The ns value is equal to p≥0.05; * is equal to p< 0.05, ** is equal to p < 0.01, *** is equal to p < 0.001, and **** is equal to p < 0.0001.
2.3 Correlation analyses between CHST12 expression characteristics and the clinicopathology of PAAD
Detailed patient characteristics are shown in Table 1. Chi-square or Fisher exact tests were used to compare the clinicopathological characteristics of the 178 enrolled patients. Then, clinicopathological characteristics and CHST12 expression were analyzed using logistic regression.
2.4 Clinical significance of CHST12 expression in PAAD
Receiver operating characteristic (ROC) curve analysis was used to evaluate the diagnostic value of CHST12 for PAAD. Then, a prognostic analysis of CHST12 in PAAD patients was evaluated using the Kaplan–Meier method, univariate Cox proportional hazards regression, and multivariate Cox regression analysis, which were performed using the “survminer” and “survival” R packages. Published studies provide clinical outcomes data of PAAD patients, including OS, progression-free intervals (PFIs), and disease-specific survival (DDS). Analyses of statistical data were conducted using R (version 3.6.3). Significance values are defined as P-values less than 0.05.
2.5 Analysis of CHST12-associated differentially expressed genes in PAAD with functional annotations
DEGs identified between high and low expression groups of CHST12 mRNA were analyzed and processed through functional enrichment analysis with the Metascape database, Gene Ontology (GO) terms, and Kyoto Encyclopedia of Genes and Genomes (KEGG) pathways. In addition, reactome gene sets (https://metascape.org/gp/index.html#/main/step1) were included (40). The resultant terms were grouped into features based on attribute similarity, with a p-value threshold of 0.01 (< 0.01) and a minimum of three enriched genes.
2.6 Infiltration of immune cells into PAAD is associated with CHST12 expression
Tumor Immune Estimation Resource (TIMER) is an online database that uses a deconvolution statistical method, the single-sample GSEA (ssGSEA), to evaluate the TME immune cell infiltration levels from gene expression profiles (41–43). Via gene modules, TILs, including CD8+ T cells, B cells, CD4+ T cells, neutrophils, macrophages, and dendritic cells, were evaluated in PAAD in relation to CHST12 mRNA expression. The website (http://cis.hku.hk/TISIDB/) analyzes the relationship between tumors and the innate immune system (44). A database of tumor-immune system interactions called the Tumor-Immune System Interaction Database (TISIDB) was used to validate the relationship between CHST12 expression and TILs in PAAD using Pearson’s correlation analysis. Finally, a comparison of the distributions of immune checkpoint-associated genes (SIGLEC15, IDO1, CD274, HAVCR2, PDCD1, CTLA4, LAG3, and PDCD1LG2) was performed between the high and low expression groups of CHST12. Statistical significance was examined using the Wilcox test. The asterisks represent the degree of importance (*p < 0.05, **p < 0.01, ***p < 0.001).
2.7 Analysis of tumor immune dysfunction and exclusion
Using the gene exclusion model for tumor immune dysfunction, Tumor Immune Dysfunction and Exclusion (TIDE) model predicts cancer sensitivity to immune checkpoint therapy. The TIDE model includes dysfunctional T cells and the exclusion of T-cell mechanisms of immune evasion by tumors (45). The TIDE score indicates the potential for tumor immune escape. A high TIDE score suggests a bad curative effect of ICIs and a short survival time after ICI treatment in patients.
3 Results
3.1 Expression profiles of CHST12 and prognosis value in PAAD
In the TCGA+GTEx datasets, compared with normal tissues (N=171), tumor tissues (N=179, p < 0.001) expressed high levels of CHST12 mRNA. Likewise, in the paired analysis of normal and tumor tissues, CHST12 was highly expressed in pancreatic cancer tissues (N=41; p=0.038) (Figures 1A, B). We further analyzed the protein expression of CHST12 by immunohistochemistry. Interestingly, CHST12 protein levels from The Human Protein Atlas (THPA) database showed the opposite trend of mRNA levels, with lower levels of expression observed in PAAD tissues (Figure 1C). These results indicate an important post-transcriptional modification of CHST12.
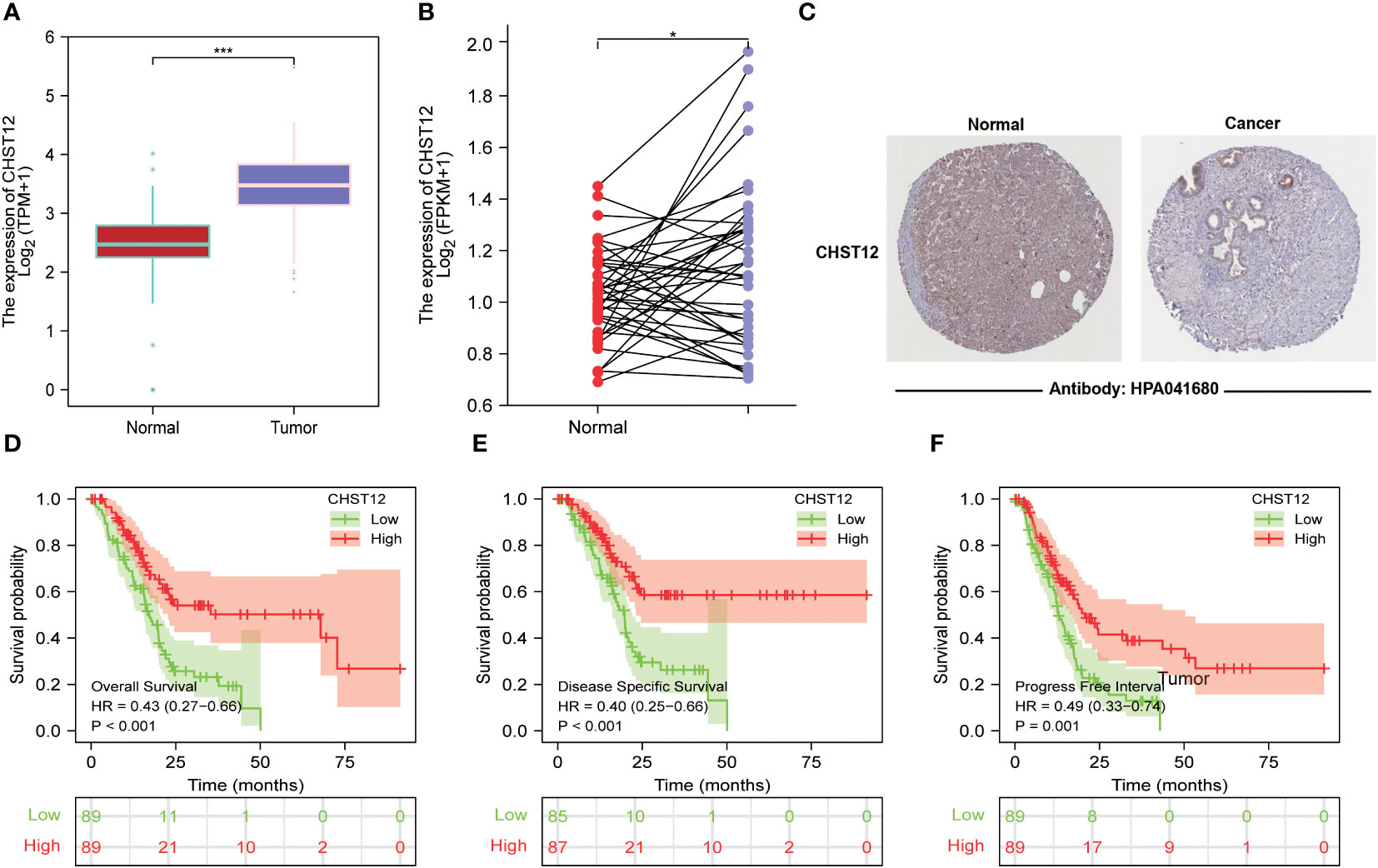
Figure 1 (A) Differential expression of CHST12 mRNA in normal and tumor tissues of patients with PAAD. The results show that CHST12 was highly expressed in pancreatic cancer tissues. (B) Differential expression of CHST12 mRNA in PAAD paired tumor and normal samples. The results show CHST12 was highly expressed in the PAAD paired tumor sample. (C) CHST12 proteins are differentially expressed in PAAD tumors and normal tissues according to THPA. The results show the opposite trend of mRNA levels, with lower levels of expression observed in PAAD tissues. (D) Survival curves comparing low to high expression groups of CHST12 mRNA for PAAD patients. (E) DSS. (F) PFI. The results show that low expression patients had a significantly shorter OS (p < 0.001, HR: 0.43), DSS (p< 0.001, HR: 0.40), and PFI (p = 0.001, HR: 0.49) than the high expression group. “*”:P<0.05, “***”:P<0.001.
To better explore the prognostic value of CHST12, patients from the TCGA dataset were initially classified into high and low groups based on the CHST12 mRNA level. Kaplan–Meier curves showed that low expression patients had a significantly shorter OS (p < 0.001; HR, 0.43), DSS (p < 0.001; HR, 0.40), and PFI (p = 0.001; HR, 0.49) than the high expression group (Figures 1D–F). These data suggest that CHST12 is a potential clinical outcomes-protected factor.
3.2 Correlation between CHST12 expression and the clinicopathological characteristics of PAAD
Our study examined the clinicopathological characteristics of patients with PAAD who expressed high and low CHST12 mRNA expression, as shown in Table 1. In terms of the distribution of clinicopathological characteristics, there was no significant difference (gender, age, T-stage, N-stage, M-stage, pathologic stage, radiation therapy, and primary therapy outcome). Furthermore, by using logistics analysis, we determined the correlation between CHST12 expression and clinicopathological characteristics (T stage, N stage, M stage, pathologic stage, primary therapy outcome, gender, age, histologic grade, anatomic neoplasm subdivision, alcohol history, history of diabetes, and family history of cancer). Prominently positive correlations between CHST12 expression and anatomic neoplasm subdivision and alcohol history are presented in Table 2.
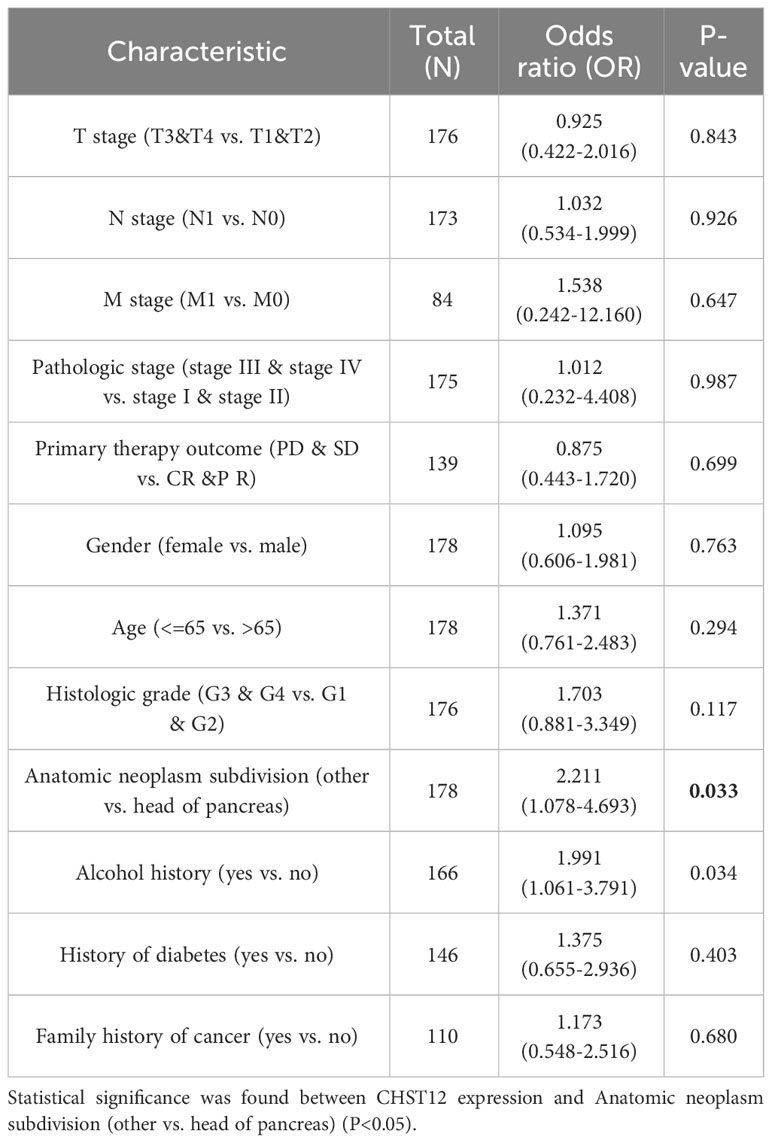
Table 2 Logistic regression analysis of the association between CHST12 expression and the clinicopathological characteristics in PAAD patients.
3.3 Diagnostic and prognostic predictive value of CHST12 in PAAD
To investigate the predictive value for PAAD diagnosis, ROC curves and the AUC of CHST12 were calculated in PAAD to demonstrate its discriminating value. As the AUC was 0.911 (95% CI = 0.879–0.943), CHST12 showed high diagnostic efficacy, with 82.1% sensitivity and 91.2% specificity and a high positive predictive value (PPV) (90.7%) and negative predictive value (NPV) (83.0%) for the diagnosis of PAAD (Figure 2A). Next, we performed univariate and multivariate Cox regression analyses to identify the potential prognostic value of CHST12 for clinical outcomes. CHST12 mRNA expression was shown to be an independent risk factor for OS in a multivariate Cox regression analysis (HR, 0.617; P = 0.040) (Table 3). Similarly, the clinical N stage displayed a meaningful predictive value for DSS in PAAD. Here, key prognostic factors in OS, DSS, and PFI were selected as parameters for constructing nomograms to present the prognostic status of PAAD patients (Figures 2B, D, F). Then, to test the efficacy of the nomograms, calibration curves were drawn for the 1-, 2-, and 3-year clinical outcomes. A C-index of 0.619 (0.585-0.654) was obtained for CHST12 in the nomogram to predict OS (Figure 2C), DSS (Figure 2E), and PFI (Figure 2G).
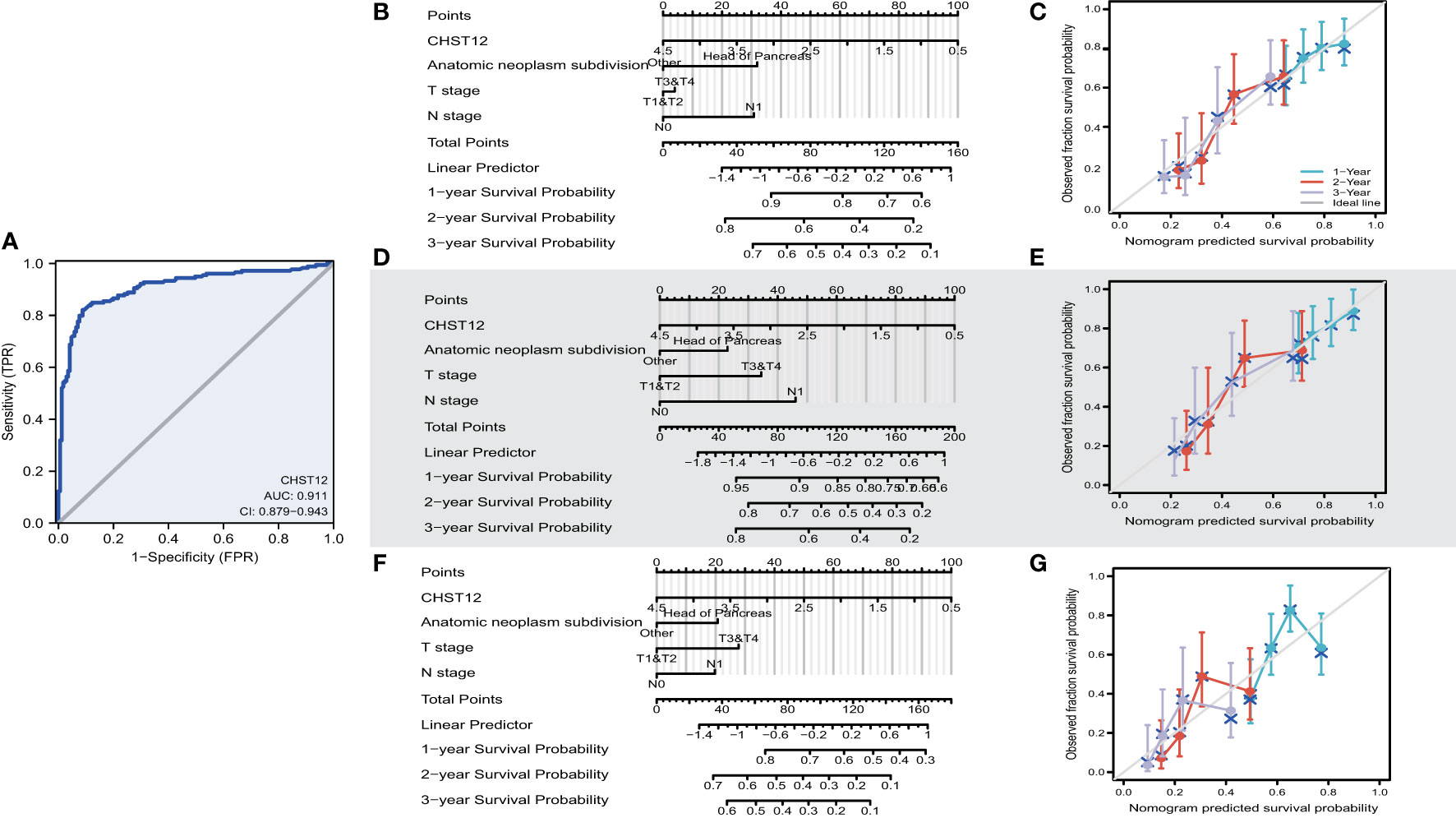
Figure 2 (A) ROC curve analysis for CHST12 clinical predictive values in PAAD patients. As the AUC was 0.911 (95% CI = 0.879–0.943), CHST12 showed high diagnostic efficacy, with 82.1% sensitivity and 91.2% specificity and a high positive predictive value (PPV) (90.7%) and negative predictive value (NPV) (83.0%) for the diagnosis of PAAD. (B) Nomograms of overall survival for CHSE12 expression-based risk scoring models predicting 1-, 2-, and 3-year clinical outcomes. (C) Calibration plots for the nomograms for overall survival. (D) Nomograms of the progress free interval (PFI) for CHSE12 expression-based risk scoring models predicting 1-, 2-, and 3-year clinical outcomes. (E) Calibration plots for the nomograms for PFI. (F) Nomograms of disease-specific survival (DSS) for CHSE12 expression-based risk scoring models predicting 1-, 2-, and 3-year clinical outcomes. (G) Calibration plots for the nomograms for DSS.
3.4 Functional annotation and identified CHST12-related signaling pathways in PAAD
A pathway enrichment analysis of CHST12-associated DEGs was conducted using the Metascape database, GO Biological Processes, KEGG Pathway, and Reactome Gene Set. These can help elucidate the functional role of CHST12-associated DEGs in PAAD patients with the criteria of false discovery rate (FDR) < 0.05 and |log2FC| > 1 (40). In the enrichment analysis, we found that the DEGs usually target tumor progression, immune-related signaling pathways, or biological processes that are usually involved in the tumor microenvironment. These may include immune system process, cellular response to growth factor stimulus, and signaling by receptor tyrosine kinases. (Figures 3A, B).
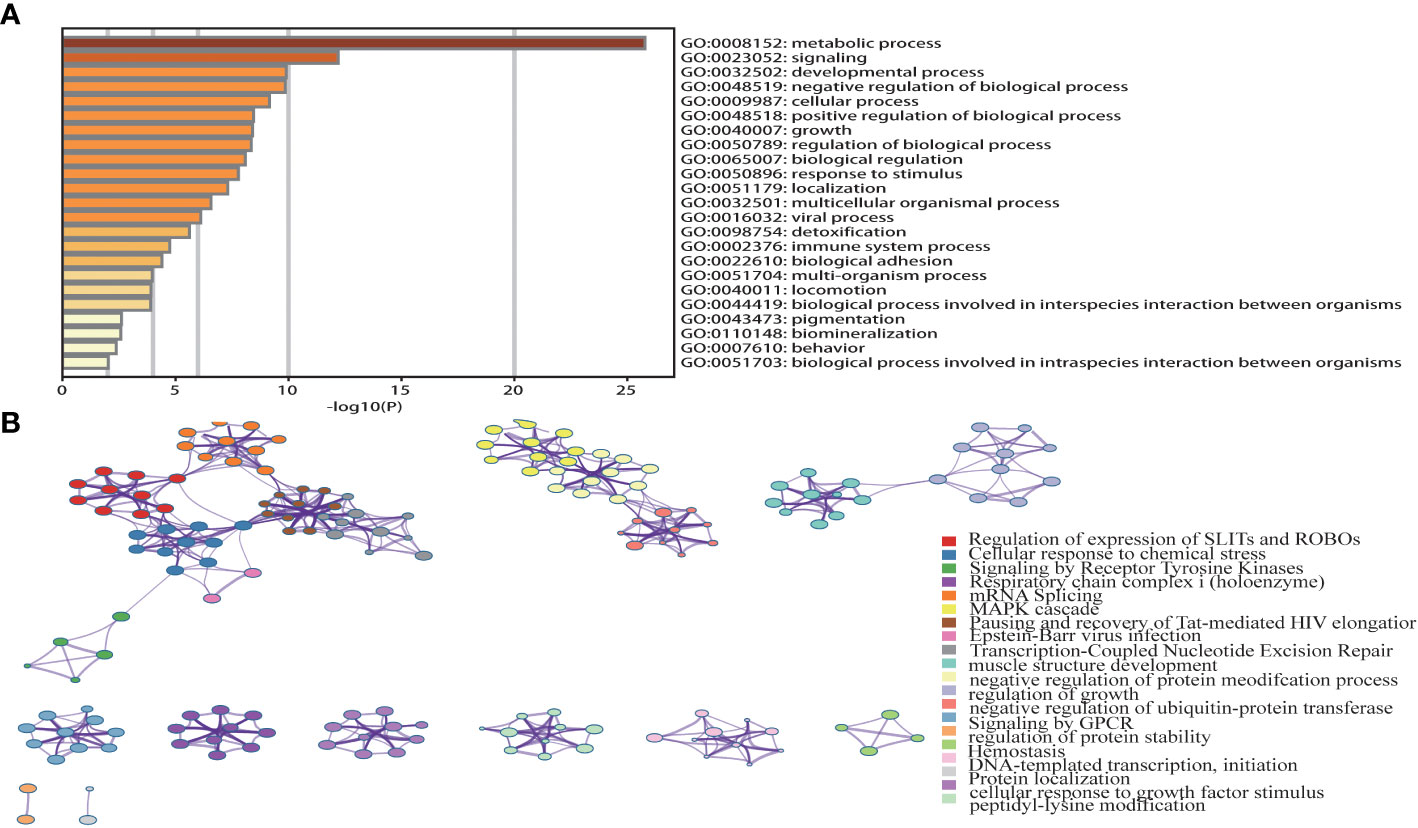
Figure 3 (A) Top 23 pathway clusters associated with CHST12-related DEGs enriched by Metascape. (B) TGraph of the top 20 enriched clustered terms. The term colors indicate nodes in close proximity to each other. An edge links terms that have a similarity score of >0.3 (the edge thickness corresponds to the similarity score). The darker the color, the greater the statistical significance. In the enrichment analysis, we found that the DEGs usually targeted tumor progression, immune-related signaling pathways, or biological processes that are usually involved in the tumor microenvironment.
3.5 CHST12 may have a certain correlation with the immune infiltration of TME in PAAD
A crucial role for glycosylation in modulating tumor immunity has been demonstrated and CHST12-associated DEG pathway analysis suggested that as a member of glycosylation, CHST12 may play a significant role in tumor microenvironment immunity in PAAD tumors. Hence, we tested whether there are correlations between CHST12 expression and TILs. We detected that higher CHST12 mRNA expression was associated with the increased infiltration of CD4+ T cells, macrophages, neutrophils, and dendritic cells. (Figure 4A). Using TISIDB analysis, our findings revealed that CHST12 mRNA was remarkably negatively correlated with CD4 and Type2 T-helper (Th2) cells, the infiltration of which was critical for immunotherapy producing anti-cancer immunity (Figures 4B, C). We further analyzed the relationship between CHST12 expression and the expression of immune checkpoints (CD274, CTLA4, HAVCR2, LAG3, PD-1 (PDCD1), PDCD1LG2, TIGIT, and SIGLEC15). Additionally, we proved that CHST12 mRNA expression was associated with several immune checkpoint distributions, including PD-1, CTLA-4, and LAG-3 in PAAD (Figure 4D). In summary, the expression pattern of CHST12 is closely related to immune cell infiltration in the TME of PAAD.
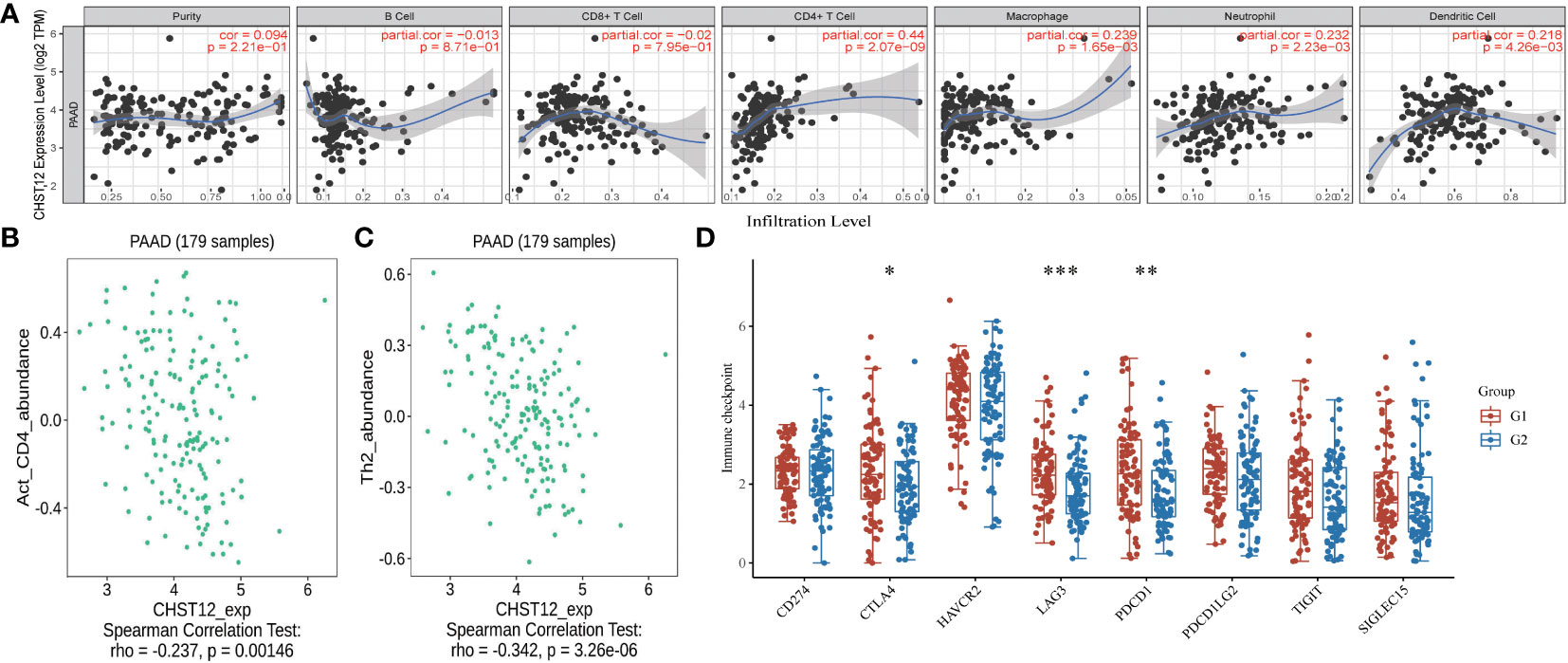
Figure 4 (A) A positive correlation was found between CHST12 expression and the infiltrating levels of T cells, macrophages, neutrophils, and dendritic cells; however, there was a negative correlation with CD8+ cells. (B) Correlation between CHST12 expression and the abundance of activated CD4 T cells in PAAD. (C) Correlation between CHST12 expression and the abundance of activated Th2 cells in PAAD. (D) CHST12 mRNA expression was associated with several immune checkpoint distributions, including PD-1, CTLA-4, and LAG-3 in PAAD.
3.6 CHST12 contributes to immune escape and resistance to ICIs
There is growing evidence that the tumor-infiltrating immune cells play a critical role in the TME and that higher levels of immune cell infiltration are associated with a better prognosis for tumor patients. Pearson correlation analysis using the TIDE web tool showed that increased CHST12 expression was associated with an increase in the number of cytotoxic T lymphocytes in two PAAD datasets (TCGA-PAAD and GSE21501@PRECOG, TCGA-PAAD; r = 0.185, p = 0.0147; GSE21501, r = 0.306, p = 0.00178) (Figures 5A, B). Moreover, we found that in the high CHST12 expression level group, high-level cytotoxic T lymphocyte (CTL) infiltration indicates better survival (Figure 5C); vice versa, in the low CHST12 expression level group, low CTL infiltration indicates better survival (Figure 5D). In the TIDE gene set prioritization module, tumor immune evasion phenotypes were not detected with a negative TIDE score. Furthermore, we examined the correlation between the CHST12 expression level and the efficacy of immunotherapy. Using the gene set prioritization module, we found that low CHST12 was related to an increase in the clinical efficacy of immunotherapy in melanoma and bladder cancer. These results suggest that CHST12 may be a potential candidate predictor for immunotherapy, especially ICIs.
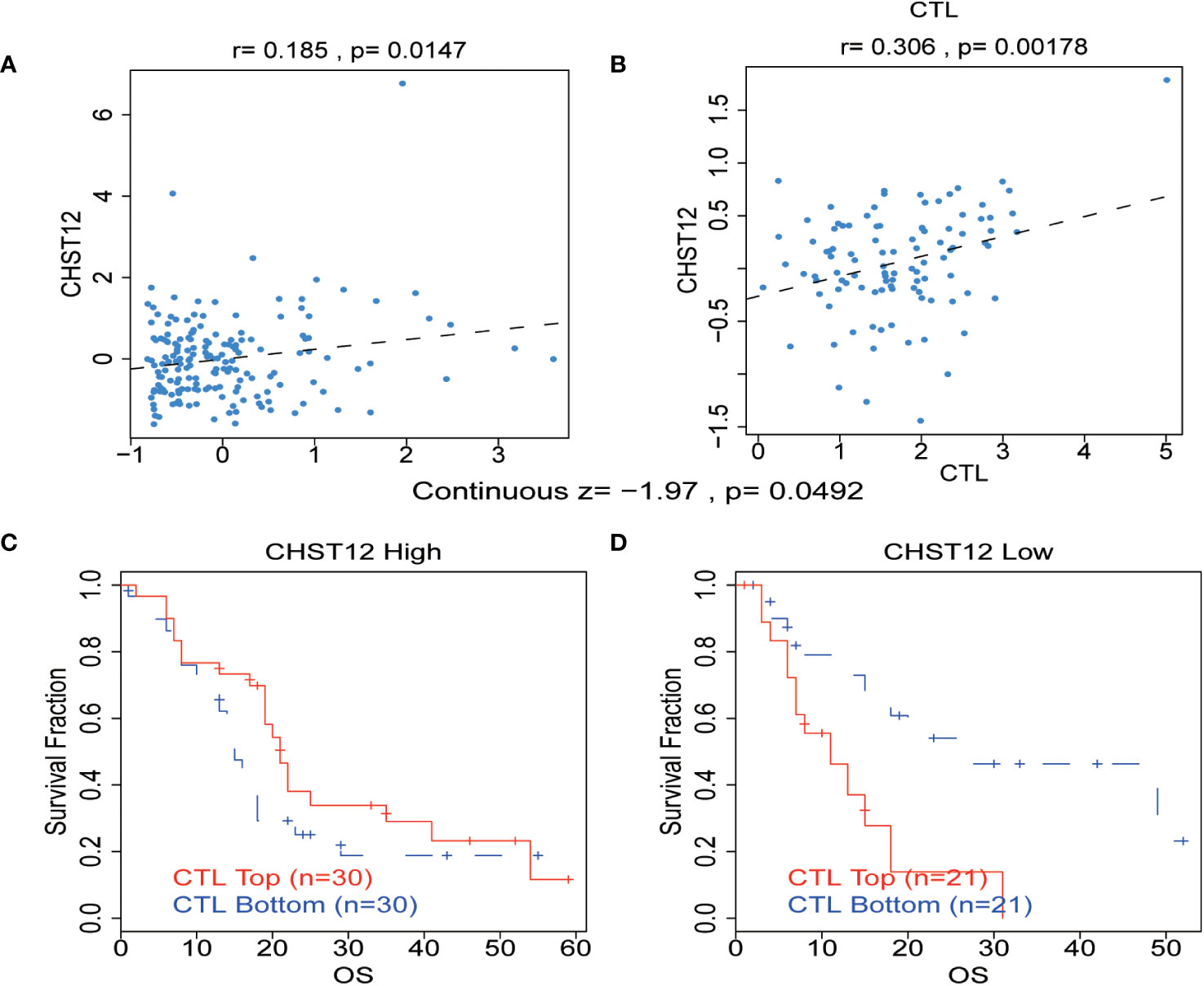
Figure 5 (A, B) Correlation between the levels of CHST12 and the CTL in the TME of PAAD from TCGA and GSE21501@PRECOG datasets. TCGA-PAAD: r = 0.185, p = 0.0147; GSE21501: r=0.306, p=0.00178) (C, D) The association between the CTL level and OS for PAAD with CHST12 high and low expression groups.
4 Discussion
Carbohydrate sulfotransferases are enzymes that transfer sulfate to carbohydrate groups in glycoproteins, which have been implicated in the metastatic spread of tumor cells through the modulation of the TME (46–49). The deregulated expression of CHST family members has been reported in a wide range of human cancer types (36). Here, according to our findings, CHST12 mRNA expression was upregulated in PAAD, and low CHST12 expression predicted a favorable outcome for PAAD patients.
Through transcriptome analysis, we found that CHST12 mRNA was highly expressed in tumors, but CHST12 protein showed lower expression in PAAD tumor tissues than in normal tissues. The contradiction between the upregulation of CHST12 mRNA and downregulation of the protein may involve potential post-transcriptional modification mechanisms, such as RNA splicing or protein degradation. This result highly suggests that CHST12 has experienced post-transcriptional modification. Moreover, pancreatic cancer patients with low CHST12 protein expression have a worse prognosis, which is consistent with the findings of Han Nie et al. (50). Through multivariate regression analysis, we identified CHST12 and N staging as independent prognostic risk factors for OS in PAAD patients, which indicates that CHST12 may be useful in clinical settings as a prognostic biomarker and possesses potential clinical utility in PAAD patients. The ROC curve shows the diagnostic performance of CHST12 in discriminating PAAD diagnosis with an AUC of 0.911, suggesting that CHST12 is a high-performance biomarker for PAAD diagnosis. These results agree with previously reported results that other tumor patients with high CHST12 mRNA levels have shorter survival times (51, 52). Considering the key value of CHST12 in clinical diagnosis, we further investigated the potential functions and molecular mechanisms of CHST12 in PAAD. In our study, functional enrichment analysis of CHST12-associated DEGs showed that they are significantly enriched in tumor progression and immune-related signaling pathways. CHST12 has shown a similar effect in hepatocellular carcinoma (53). All the above analyses indicate that CHST12 may be involved in the regulation of the TME in PAAD.
Glycosylation plays a significant role in immunity and endows unique properties to glycoproteins (54, 55). Moreover, our functional studies have found that CHST12 plays a vital role in the TME. Hence, the effects of CHST12 expression on the TME and immunotherapy of pancreatic cancer is a key aspect of our study. According to our findings, CHST12 expression is positively correlated with the infiltration of immune cells (CD4+ T cells, macrophages, neutrophils, and dendritic cells), which contributes to immunosurveillance, the elimination of tumor cells, and the slowing of immune evasion in the PAAD microenvironment (56, 57). Additionally, we proved that CHST12 mRNA expression was associated with several immune checkpoint distributions, including PD-1, CTLA-4, and LAG-3, in PAAD. Using the gene set prioritization module, we found that low CHST12 was related to the increase in the clinical efficacy of immunotherapy in melanoma and bladder cancer. These results suggest that CHST12 may be a potential candidate predictor for immunotherapy, especially ICIs. To test the prediction, we will next conduct a verification experiment clinicopathologically. Combined with the results of the previous discovery of the post-transcriptional modification of CHST12, our study supports the notion that the decreased expression of CHST12 protein in the TME may produce an anti-tumor effect by acting on the recruitment of immune cells to the TME. Notably, in terms of CTLA4, LAG3, and PDCD1, the low expression CHST12 group differed significantly from the high expression CHST12 group. CTLA4, LAG3, and PDCD1 are important targets for cancer ICI therapy. This finding prompted us to investigate whether CHST12 expression impacts clinical outcomes when treated with ICIs. Using the TIDE algorithm, we predicted the response of patients to immunotherapy. We found that high CHST12 mRNA expression is related to the increased efficacy of immunotherapy in melanoma and bladder cancer. These data imply that CHST12 may be a predictor for the efficacy of immunotherapy.
Our findings uncover CHST12 as a new independent predictor of PAAD prognosis and immunotherapeutic efficacy, but it has its limitations. First, CHST12 expression and its prognostic significance were identified through bioinformatic analysis. Hence, further study with clinical samples for such applications is needed to verify the above findings. In addition, in vivo and in vitro experiments are needed to provide additional information about how CHST12 impacts immune infiltration in the PAAD TME.
Conclusively, in this study, we have shown that CHST12 mRNA expression is upregulated in PAAD, whereas CHST12 protein expression is downregulated. The expression of CHST12 is an independent predictor of a good prognosis in PAAD. In addition, CHST12 may affect the infiltration of immune cells in the microenvironment of PAAD. Our data highlight the potential of CHST12 in predicting ICI efficacy. In the future, we hope to further investigate the regulatory mechanisms of CHST12 through animal trials to test its validation. Additionally, we hope to conduct clinical trials as well. Overall, CHST12 may be a novel prognosis biomarker and a potential predictor of the response to ICI treatment in PAAD patients.
Data availability statement
The data sets used and/or analyzed during the current study arec available from the following public data sources: the TCGA-PAAD (https://xenabrowser.net/datapages/) and GSE21501 (https://ncbi.nlm.nih.gov/geo/query/acc.cgi?acc=GSE21501) data sets. Publicly available datasets were analyzed in this study. These data can be obtained from the following sites: http://www.xiantao.love; https://www.proteinatlas.org/; https://metascape.org/; http://tide.dfci.harvard.edu/; http://cis.hku.hk/TISIDB/.
Ethics statement
The studies involving humans were approved by Shengjing Hospital of China Medical University. The studies were conducted in accordance with the local legislation and institutional requirements. The participants provided their written informed consent to participate in this study. Written informed consent was obtained from the individual(s) for the publication of any potentially identifiable images or data included in this article.
Author contributions
Interpretation or analysis of the data: KL and LL; Preparation of the manuscript: KL and LL; Revision for important intellectual content: GH; Supervision: GH. All authors contributed to the article and approved the submitted version.
Funding
The author(s) declare financial support was received for the research, authorship, and/or publication of this article. This study was funded by the National Natural Science Foundation of China (82071242) and the 345 Talent Project of Shengjing Hospital.
Conflict of interest
The authors declare that the research was conducted in the absence of any commercial or financial relationships that could be construed as a potential conflict of interest.
Publisher’s note
All claims expressed in this article are solely those of the authors and do not necessarily represent those of their affiliated organizations, or those of the publisher, the editors and the reviewers. Any product that may be evaluated in this article, or claim that may be made by its manufacturer, is not guaranteed or endorsed by the publisher.
References
1. Li C, Lin L, Zhang L, Xu R, Chen X, Ji J, et al. Long noncoding RNA p21 enhances autophagy to alleviate endothelial progenitor cells damage and promote endothelial repair in hypertension through SESN2/AMPK/TSC2 pathway. Pharmacol Res (2021) 173:105920. doi: 10.1016/j.phrs.2021.105920
2. Zhu Y, Huang R, Wu Z, Song S, Cheng L, Zhu R. Deep learning-based predictive identification of neural stem cell differentiation. Nat Commun (2021) 12(1):2614. doi: 10.1038/s41467-021-22758-0
3. Han S, Chen C, Chen C, Wu L, Wu X, Lu C, et al. Coupling annealed silver nanoparticles with a porous silicon Bragg mirror SERS substrate and machine learning for rapid non-invasive disease diagnosis. Analytica Chimica Acta (2023) 1254:341116. doi: 10.1016/j.aca.2023.341116
4. Sun Q, Tao Q, Ming T, Tang S, Zhao H, Liu M, et al. Berberine is a suppressor of Hedgehog signaling cascade in colorectal cancer. Phytomedicine (2023) 114:154792. doi: 10.1016/j.phymed.2023.154792
5. Siegel RL, Miller KD, Jemal A. Cancer statistics, 2020. CA Cancer J Clin (2020) 70(1):7–30. doi: 10.3322/caac.21590
6. Carstens JL, Correa de Sampaio P, Yang D, Barua S, Wang H, Rao A, et al. Spatial computation of intratumoral T cells correlates with survival of patients with pancreatic cancer. Nat Commun (2017) 8:15095. doi: 10.1038/ncomms15095
7. Vogelstein B, Papadopoulos N, Velculescu VE, Zhou S, Diaz LA Jr, Kinzler KW. Cancer genome landscapes. Science (2013) 339(6127):1546–58. doi: 10.1126/science.1235122
8. Royal RE, Levy C, Turner K, Mathur A, Hughes M, Kammula US, et al. Phase 2 trial of single agent Ipilimumab (anti-CTLA-4) for locally advanced or metastatic pancreatic adenocarcinoma. J Immunother (2010) 33(8):828–33. doi: 10.1097/CJI.0b013e3181eec14c
9. Li Q, Miao Y, Zeng X, Tarimo CS, Wu C, Wu J. Prevalence and factors for anxiety during the coronavirus disease 2019 (COVID-19) epidemic among the teachers in China. J Affect Disord (2020) 277:153–8. doi: 10.1016/j.jad.2020.08.017
10. Xu H, van der Jeught K, Zhou Z, Zhang L, Yu T, Sun Y, et al. Atractylenolide I enhances responsiveness to immune checkpoint blockade therapy by activating tumor antigen presentation. J Clin Invest (2021) 131(10):e146832. doi: 10.1172/JCI146832
11. Abbas M, Alqahtani MS, Alshahrani MY, Alabdullh K. Aggressive and drug-resistant pancreatic cancer: challenges and novel treatment approaches. Discovery Med (2022) 34(173):158–64.
12. Liu G, Li B, Xu Z, Wang J, Ma S, Kan Y, et al. Bacillus calmette-guerin for the treatment of non-muscle invasive bladder cancer: history and current status. Discovery Med (2022) 33(169):85–92.
13. Hui L, Chen Y. Tumor microenvironment: Sanctuary of the devil. Cancer Lett (2015) 368(1):7–13. doi: 10.1016/j.canlet.2015.07.039
14. Qian Y, Gong Y, Fan Z, Luo G, Huang Q, Deng S, et al. Molecular alterations and targeted therapy in pancreatic ductal adenocarcinoma. J Hematol Oncol (2020) 13(1):130. doi: 10.1186/s13045-020-00958-3
15. Buscail L, Bournet B, Cordelier P. Role of oncogenic KRAS in the diagnosis, prognosis and treatment of pancreatic cancer. Nat Rev Gastroenterol Hepatol (2020) 17(3):153–68. doi: 10.1038/s41575-019-0245-4
16. Klimstra DS, Longnecker DS. K-ras mutations in pancreatic ductal proliferative lesions. Am J Pathol (1994) 145(6):1547–50.
17. Bailey P, Chang DK, Nones K, Johns AL, Patch AM, Gingras MC, et al. Genomic analyses identify molecular subtypes of pancreatic cancer. Nature (2016) 531(7592):47–52. doi: 10.1038/nature16965
18. Oh D, Seo DW, Song TJ, Park DH, Lee SK, Kim MH. Clinical outcomes of EUS-guided radiofrequency ablation for unresectable pancreatic cancer: A prospective observational study. Endosc Ultrasound Jan-Feb (2022) 11(1):68–74. doi: 10.4103/eus-d-21-00049
19. Rizza S, Maldi E, Laudi C, Mellano A, Pisacane A, Staiano T. A pancreatic metastasis from a colon carcinoma mimicking a primary tumor diagnosed by EUS-guided fine-needle biopsy. Endosc Ultrasound (2022) 12(1):162–3. doi: 10.4103/eus-d-21-00186
20. Nakai A, Kamata K, Hyodo T, Chikugo T, Hara A, Otsuka Y, et al. Utility of contrast-enhanced harmonic EUS for diagnosis of portal vein invasion by pancreatic cancer. Endosc Ultrasound (2022) 11(5):401–6. doi: 10.4103/eus-d-21-00185
21. Ohtsubo K, Marth JD. Glycosylation in cellular mechanisms of health and disease. Cell (2006) 126(5):855–67. doi: 10.1016/j.cell.2006.08.019
22. Sperandio M, Gleissner CA, Ley K. Glycosylation in immune cell trafficking. Immunol Rev (2009) 230(1):97–113. doi: 10.1111/j.1600-065X.2009.00795.x
23. Pinho SS, Reis CA. Glycosylation in cancer: mechanisms and clinical implications. Nat Rev Cancer (2015) 15(9):540–55. doi: 10.1038/nrc3982
24. Stowell SR, Ju T, Cummings RD. Protein glycosylation in cancer. Annu Rev Pathol (2015) 10:473–510. doi: 10.1146/annurev-pathol-012414-040438
25. Barkeer S, Chugh S, Batra SK, Ponnusamy MP. Glycosylation of cancer stem cells: function in stemness, tumorigenesis, and metastasis. Neoplasia (2018) 20(8):813–25. doi: 10.1016/j.neo.2018.06.001
26. Silsirivanit A. Glycosylation markers in cancer. Adv Clin Chem (2019) 89:189–213. doi: 10.1016/bs.acc.2018.12.005
27. Zhang Z, Wang L, Zheng W, Yin L, Hu R, Yang B. Endoscope image mosaic based on pyramid ORB. Biomed Signal Process control (2022) 71:103261. doi: 10.1016/j.bspc.2021.103261
28. RodrÍguez E, Schetters STT, van Kooyk Y. The tumour glyco-code as a novel immune checkpoint for immunotherapy. Nat Rev Immunol (2018) 18(3):204–11. doi: 10.1038/nri.2018.3
29. Peixoto A, Relvas-Santos M, Azevedo R, Santos LL, Ferreira JA. Protein glycosylation and tumor microenvironment alterations driving cancer hallmarks. Front Oncol (2019) 9:380. doi: 10.3389/fonc.2019.00380
30. Buettner MJ, Shah SR, Saeui CT, Ariss R, Yarema KJ. Improving immunotherapy through glycodesign. Front Immunol (2018) 9:2485. doi: 10.3389/fimmu.2018.02485
31. Nardy AF, Freire-de-Lima L, Freire-de-Lima CG, Morrot A. The sweet side of immune evasion: role of glycans in the mechanisms of cancer progression. Front Oncol (2016) 6:54. doi: 10.3389/fonc.2016.00054
32. Tzanakakis G, Neagu M, Tsatsakis A, Nikitovic D. Proteoglycans and immunobiology of cancer-therapeutic implications. Front Immunol (2019) 10:875. doi: 10.3389/fimmu.2019.00875
33. Cox TR. The matrix in cancer. Nat Rev Cancer (2021) 21(4):217–38. doi: 10.1038/s41568-020-00329-7
34. Yan Z, Wang S. Proteoglycans as therapeutic targets in brain cancer. Front Oncol (2020) 10:1358. doi: 10.3389/fonc.2020.01358
35. Lanzi C, Cassinelli G. Receptor tyrosine kinases and heparan sulfate proteoglycans: Interplay providing anticancer targeting strategies and new therapeutic opportunities. Biochem Pharmacol (2020) 178:114084. doi: 10.1016/j.bcp.2020.114084
36. Wang J, Xia X, Tao X, Zhao P, Deng C. Knockdown of carbohydrate sulfotransferase 12 decreases the proliferation and mobility of glioblastoma cells via the WNT/β-catenin pathway. Bioengineered (2021) 12(1):3934–46. doi: 10.1080/21655979.2021.1944455
37. Ren Q, Wang J, Liu C, Meng LX, Qian RK, Gao HJ, et al. Exploring the sulfate patterns of chondroitin sulfate/dermatan sulfate and keratan sulfate in human pancreatic cancer. J Pharm BioMed Anal (2021) 205:114339. doi: 10.1016/j.jpba.2021.114339
38. Lu S, Yang B, Xiao Y, Liu S, Liu M, Yin L, et al. Iterative reconstruction of low-dose CT based on differential sparse. Biomed Signal Process Control (2023) 79:104204. doi: 10.1016/j.bspc.2022.104204
39. Love MI, Huber W, Anders S. Moderated estimation of fold change and dispersion for RNA-seq data with DESeq2. Genome Biol (2014) 15(12):550. doi: 10.1186/s13059-014-0550-8
40. Zhou Y, Zhou B, Pache L, Chang M, Khodabakhshi AH, Tanaseichuk O, et al. Metascape provides a biologist-oriented resource for the analysis of systems-level datasets. Nat Commun (2019) 10(1):1523. doi: 10.1038/s41467-019-09234-6
41. Subramanian A, Tamayo P, Mootha VK, Mukherjee S, Ebert BL, Gillette MA, et al. Gene set enrichment analysis: a knowledge-based approach for interpreting genome-wide expression profiles. Proc Natl Acad Sci U S A (2005) 102(43):15545–50. doi: 10.1073/pnas.0506580102
42. Li T, Fan J, Wang B, Traugh N, Chen Q, Liu JS, et al. TIMER: A web server for comprehensive analysis of tumor-infiltrating immune cells. Cancer Res (2017) 77(21):e108–10. doi: 10.1158/0008-5472.CAN-17-0307
43. Li B, Severson E, Pignon JC, Zhao H, Li T, Novak J, et al. Comprehensive analyses of tumor immunity: implications for cancer immunotherapy. Genome Biol (2016) 17(1):174. doi: 10.1186/s13059-016-1028-7
44. Ru B, Wong CN, Tong Y, Zhong JY, Zhong SSW, Wu WC, et al. TISIDB: an integrated repository portal for tumor-immune system interactions. Bioinformatics (2019) 35(20):4200–2. doi: 10.1093/bioinformatics
45. Jiang P, Gu S, Pan D, Fu J, Sahu A, Hu X, et al. Signatures of T cell dysfunction and exclusion predict cancer immunotherapy response. Nat Med (2018) 24(10):1550–8. doi: 10.1038/s41591-018-0136-1
46. Cooney CA, Jousheghany F, Yao-Borengasser A, Phanavanh B, Gomes T, Kieber-Emmons AM, et al. Chondroitin sulfates play a major role in breast cancer metastasis: a role for CSPG4 and CHST11 gene expression in forming surface P-selectin ligands in aggressive breast cancer cells. Breast Cancer Res (2011) 13(3):R58. doi: 10.1186/bcr2895
47. Ly M, Laremore TN, Linhardt RJ. Proteoglycomics: recent progress and future challenges. OMICS (2010) 14(4):389–99. doi: 10.1089/omi.2009.0123
48. Suwiwat S, Ricciardelli C, Tammi R, Tammi M, Auvinen P, Kosma VM, et al. Expression of extracellular matrix components versican, chondroitin sulfate, tenascin, and hyaluronan, and their association with disease outcome in node-negative breast cancer. Clin Cancer Res (2004) 10(7):2491–8. doi: 10.1158/1078-0432.ccr-03-0146
49. Shibata TK, Matsumura F, Wang P, Yu S, Chou CC, Khoo KH, et al. Identification of mono- and disulfated N-acetyl-lactosaminyl Oligosaccharide structures as epitopes specifically recognized by humanized monoclonal antibody HMOCC-1 raised against ovarian cancer. J Biol Chem (2012) 287(9):6592–602. doi: 10.1074/jbc.M111.305334
50. Nie H, Luo C, Liao K, Xu J, Cheng XX, Wang X. Seven glycolysis-related genes predict the prognosis of patients with pancreatic cancer. Front Cell Dev Biol (2021) 9:647106. doi: 10.3389/fcell.2021.647106
51. Matsumoto H, Minaga K, Nakano S, Uenoyama Y. Very late distant retroperitoneal metastasis of breast cancer diagnosed using EUS-guided tissue acquisition. Endosc Ultrasound (2022) 12(2):279–80. doi: 10.4103/eus-d-22-00050
52. Zhang L, Fan Y, Wang X, Yang M, Wu X, Huang W, et al. Carbohydrate sulfotransferase 4 inhibits the progression of hepatitis B virus-related hepatocellular carcinoma and is a potential prognostic marker in several tumors. Front Oncol (2020) 10:554331. doi: 10.3389/fonc.2020.554331
53. Xia X, Zhang H, Xia P, Zhu Y, Liu J, Xu K, et al. Identification of Glycolysis-Related lncRNAs and the Novel lncRNA WAC-AS1 Promotes Glycolysis and Tumor Progression in Hepatocellular Carcinoma. Front Oncol (2021) 11:733595. doi: 10.3389/fonc.2021.733595
54. Miller JL, de Wet BJ, Martinez-Pomares L, Radcliffe CM, Dwek RA, Rudd PM, et al. The mannose receptor mediates dengue virus infection of macrophages. PLoS Pathog (2008) 4(2):e17. doi: 10.1371/journal.ppat.0040017
55. Zhao J, Simeone DM, Heidt D, Anderson MA, Lubman DM. Comparative serum glycoproteomics using lectin selected sialic acid glycoproteins with mass spectrometric analysis: application to pancreatic cancer serum. J Proteome Res (2006) 5(7):1792–802. doi: 10.1021/pr060034r
56. Guillerey C, Ferrari de Andrade L, Vuckovic S, Miles K, Ngiow SF, Yong MC, et al. Immunosurveillance and therapy of multiple myeloma are CD226 dependent. J Clin Invest (2015) 125(7):2904. doi: 10.1172/JCI77181
Keywords: immune cell, infiltration, glycosylation (enzymatic conjugation with carbohydrates), prognostic signature, pancreatic adenocarcinoma (PAAD), tumor microenvironment
Citation: Liu K, Li L and Han G (2024) CHST12: a potential prognostic biomarker related to the immunotherapy response in pancreatic adenocarcinoma. Front. Endocrinol. 14:1226547. doi: 10.3389/fendo.2023.1226547
Received: 21 May 2023; Accepted: 06 December 2023;
Published: 25 January 2024.
Edited by:
Liang Liu, Fudan University, ChinaReviewed by:
Murad Ali Rahat, University of Swat, PakistanTianfeng Huang, Yangzhou University, China
Jingjing Yuan, First Affiliated Hospital of Zhengzhou University, China
Copyright © 2024 Liu, Li and Han. This is an open-access article distributed under the terms of the Creative Commons Attribution License (CC BY). The use, distribution or reproduction in other forums is permitted, provided the original author(s) and the copyright owner(s) are credited and that the original publication in this journal is cited, in accordance with accepted academic practice. No use, distribution or reproduction is permitted which does not comply with these terms.
*Correspondence: Guang Han, Y211X2hnQDE2My5jb20=
†ORCID: Guang Han, orcid.org/0000-0001-7117-4241