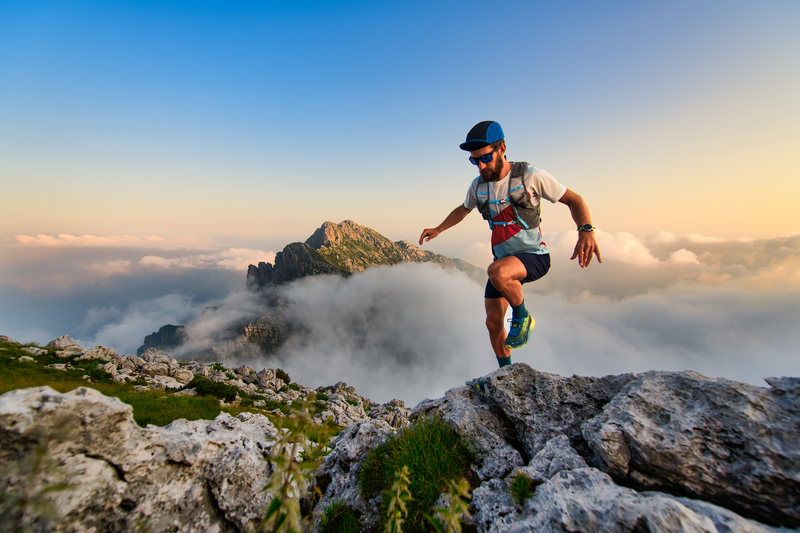
94% of researchers rate our articles as excellent or good
Learn more about the work of our research integrity team to safeguard the quality of each article we publish.
Find out more
ORIGINAL RESEARCH article
Front. Endocrinol. , 17 July 2023
Sec. Pediatric Endocrinology
Volume 14 - 2023 | https://doi.org/10.3389/fendo.2023.1199628
Background: It’s challenging for healthcare workers to detect neonatal hypoglycemia due to its rapid progression and lack of aura symptoms. This may lead to brain function impairment for the newborn, placing a significant care burden on the family and creating an economic burden for society. Tools for early diagnosis of neonatal hypoglycemia are lacking. This study aimed to identify newborns at high risk of developing neonatal hypoglycemia early by developing a risk prediction model.
Methods: Using a retrospective design, pairs (470) of women and their newborns in a tertiary hospital from December 2021 to September 2022 were included in this study. Socio-demographic data and clinical data of mothers and newborns were collected. Univariate and multivariate logistic regression were used to screen optimized factors. A neonatal hypoglycemia risk nomogram was constructed using R software, and the calibration curve and receiver operator characteristic curve (ROC) was utilized to evaluate model performance.
Results: Factors integrated into the prediction risk nomogram were maternal age (odds ratio [OR] =1.10, 95% CI: 1.04, 1.17), fasting period (OR=1.07, 95% CI: 1.03, 1.12), ritodrine use (OR=2.00, 95% CI: 1.05, 3.88), gestational diabetes mellitus (OR=2.13, 95% CI: 1.30, 3.50), gestational week (OR=0.80, 95% CI: 0.66, 0.96), fetal distress (OR=1.76, 95% CI: 1.11, 2.79) and neonatal body mass index (OR=1.50, 95% CI: 1.24, 1.84). The area under the curve (AUC) was 0.79 (95% confidence interval [CI]: 0.75, 0.82), specificity was 0.82, and sensitivity was 0.62.
Conclusion: The prediction model of this study demonstrated good predictive performance. The development of the model identifies advancing maternal age, an extended fasting period before delivery, ritodrine use, gestational diabetes mellitus diagnosis, fetal distress diagnosis and an increase in neonatal body mass index increase the probability of developing neonatal hypoglycemia, while an extended gestational week reduces the probability of developing neonatal hypoglycemia.
The fetus mainly obtains glucose from the mother and stabilizes its plasma glucose level. However, some newborns may develop hypoglycemia as they transit from intrauterine to extrauterine life. Neonatal hypoglycemia (NH) is a common neonatal metabolic disorder related to alterations in maternal and fetal metabolism, as well as disruptions in insulin production and function (1). The main clinical manifestations of NH are irritability, shortness of breath, decreased muscular tone, feeding difficulties, hypothermia, convulsion, or lethargy. Sometimes, neonatal hypoglycemia can be asymptomatic or accompanied by nonspecific manifestations (2). The incidence of NH is 10-20% (3–5), while in high-risk newborns, it can be as high as 50% (6). The incidence is still rising along with the increase of high-risk factors (7).
Due to the lack of specificity, diagnosis and intervention are difficult for healthcare providers, which may lead to newborns underwent continuous hypoglycemia, even irreversible brain injury and neurological dysfunction. In a follow-up study, 36% of newborns with NH had neurosensory impairment, including neurodevelopmental delay, visual impairment and behavioral problems (2, 8, 9). The separation of neonates with transitional hypoglycemia from their mothers to Neonatal Intensive Care Unit results in a great burden on both the family and society with family distress and increased medical costs (10, 11). Therefore, it is important to prevent its occurrence, minimize the risk factors and improve prenatal measures.
Previous studies have focused on the risk factors, management and prognosis of NH, the majority of which were focused on secondary prevention (12–15). An early identification tool to identify NH high-risk groups is lacking (6). This study aimed to develop a concise risk prediction model for early identification of NH by health professionals and to provide targeted management strategies to prevent NH.
Using a retrospective design, mothers and their newborns in a tertiary-level hospital from December 2021 to September 2022 in Wuhan, China were included in this study. Women were admitted to the hospital prior to labor or at the time of labor onset. Mothers and infants were discharged three to five days after delivery unless there were birth complications or other illnesses. Socio-demographic data and clinical data of mothers and newborns were recorded by the hospital information system (HIS) from admission to discharge. The study was reviewed and approved by the Ethics Committee of Wuhan University Medical Department, and the approval number is WHU-LFMD-IRB2023028.
To conduct multiple regression analyses, the sample size should be at least 15 times greater than the number of variables included (16). After conducting a thorough literature review and obtaining consensus among the researchers, a total of 26 variables were selected, encompassing socio-demographic data and clinical data (including previous medical history and complications) of mothers and newborns (Table 1) (17–21). Taking into account a potential attrition rate of 10%, a minimum of 429 pairs of mothers and newborns were required for the study.
Inclusion criteria were newborns: (1) meet the diagnostic criteria for NH; (2) without malformations and developmental abnormalities. Exclusion criteria were: newborns or mothers with incomplete data.
Currently, the definition of NH remains controversial. The American Academy of Pediatrics recommended that measured by a laboratory enzymatic method, any newborn with blood glucose less than 40 mg/dL (2.22 mmol/L) within four hours of birth should be considered as having NH and necessitate medical intervention (22, 23). In this study, blood glucose was measured by a heel-stick on the newborn within 30 minutes of birth using a glucose oxidase electrode type A blood glucometer manufactured by Bayer.
Data were collected from the HIS, including socio-demographic data and clinical data of mothers and newborns (see Table 1). As shown in Table 1, maternal body mass index (MBMI) was measured at admission, neonatal body mass index (NBMI) and the Apgar score were assessed at birth. The gravidity collected included this current pregnancy, while the parity did not. The fasting period was calculated from the start of fasting till the delivery of fetus. Based on the American Academy of Pediatrics (AAP) recommendations for interacting with neonates at risk of hypoglycemia (22), the following categories fall under this risk: low birth weight (LBW), which is defined as a birth weight equal to or less than 2500 grams, macrosomia that is defined as a birth weight equal to or greater than 4000 grams, small for gestational age (SGA) which is defined as a birth weight less than or equal to the 10th percentile, and finally large for gestational age (LGA) which is defined as a birth weight greater or equal to the 90th percentile.
A standardized data checklist was developed before data collection by the research team. Investigators reviewed the delivery record in the ward, and then filled the maternal and neonatal data in the checklist. Two investigators gathered the data independently and crosschecked each other’s work. Any disagreement about the data was resolved by checking the records with a third researcher.
Continuous variables were expressed by mean ± standard deviation (M ± SD) or median with interquartile range, and the discontinuous variables data were expressed as absolute numbers with percentages. For maternal and neonatal data, the Kolmogorov-Smirnov test was used to evaluate data normality.
Data analysis was conducted using SPSS (version 26.0) and R software (version 3.6.0). The process was divided into three stages: factors screening, model development and model evaluation. Using “glmnet” R packages, logistic regression analysis was used to identify risk factors from the 26 factors. Only variables with a P-value less than 0.05 in the univariate analysis were included in the multivariate regression analysis, and only variables with a P-value less than 0.05 in the multivariate analysis were selected for model development. Variables screened were entered into the model using “rms” R packages, allowing the nomogram to emerge. Using “rms” and “pROC” R packages, the calibration curve and the receiver operator characteristic curve (ROC) were depicted to evaluate the consistency and discrimination of the model.
The participants’ inclusion process is shown in Figure 1. Of 1,250 mothers and newborns identified in HIS, 478 pairs were screened. Eight pairs were excluded due to congenital heart disease (two mothers), syndactyly (five infants) and stillbirth (one infant). A total of 470 pairs were eligible for participation and 176 (37.4%) infants had experienced NH.
Socio-demographic characteristics of participants are shown in Table 1. The maternal age was [30.00 (28.00-33.00)] years, and 55 participants (11.7%) were elderly parturient women. Forty-seven percent (221) were pregnant for the first time. Most of the infants (364, 77.4%) were delivered by cesarean birth, and about half of them (244, 51.9%) were female. The gestational week was [39.00 (38.00-40.00)] weeks.
Thirteen variables were found to be statistically significant using univariate analysis (P <0.05) (Table 2). Seven of the 13 factors showed statistically significant differences employed by multivariate analysis. Of these predictors, maternal age (odds ratio [OR] =1.10, 95% CI: 1.04, 1.17), fasting period (OR=1.07, 95% CI: 1.03, 1.12), ritodrine use (OR=2.00, 95% CI: 1.05, 3.88), gestational diabetes mellitus (GDM) (OR=2.13, 95% CI: 1.30, 3.50), fetal distress (OR=1.76, 95% CI: 1.11, 2.79) and NBMI (OR=1.50, 95% CI: 1.24, 1.84) were risk factors for NH. Gestational week (GW) (OR=0.80, 95% CI: 0.66, 0.96) was a protective factor for NH (Figure 2).
A risk nomogram was developed using the model that included all seven predictors, and it is a concise tool to predict the probability of developing NH. As shown in Figure 3, the nomogram comprises three parts, including the predictive factors, points and predicted probabilities. The top line is the point reference line for each factor, and the penultimate line is the total points of all the factors. To calculate the risk of developing NH, a vertical line is drawn for each predictor, with the vertical line corresponds to the points from the top line. The relevant scores are then summed to determine the total points (the penultimate line), which corresponded to the probability of experiencing NH (bottom line).
A dynamic nomogram is intuitive and straightforward, requiring no manual computation. To obtain a predictive probability, healthcare providers need to input variables into the programmer, set x-axis ranges and click predict (Figure 4).
In this study, the calibration curve of the risk nomogram regarding prediction of NH showed good consistency (Figure 5). The uncorrected reference curve of the model is highly consistent and closely approximates the actual situation. The ROC curve was used to assess model differentiation and determine sensitivity and specificity, where the area under the ROC curve was 0.79 (95% CI: 0.75, 0.82). The specificity was 0.82, and the sensitivity was 0.62 (Figure 6).
In this study, univariate analysis revealed 13 significant predictors out of 26 variables, while only seven predictors ultimately qualified for inclusion in the prediction model using multivariate analysis. The findings of the model fit and performance evaluation are in line with what was anticipated and show good predictive performance. Previous studies investigated risk factors of NH, but most of them only focused on a few or a single factor. This study developed a novel nomogram of NH for healthcare providers’ use in primary prevention.
To evaluate the risk of maternal age accurately, it was defined as a continuous variable rather than a binary variable in this research. The results of this article show that maternal age is a risk factor for NH, there have no studies reported an association between maternal age and NH. However, a meta-analysis showed a strong positive relationship between maternal age and GDM risk (24), especially for women aged 35 years and older (25). GDM is an independent risk factor for NH (26–28). It happens as a result of GDM have been shown to increase insulin resistance and fetal hyperinsulinemia in reaction to the mother’s uterine hyperglycemia (29). Therefore, it is necessary to pay attention to advanced maternal age. Having regular prenatal check-ups can also help to detect abnormal conditions early for further intervention. Maintaining stable blood glucose and body mass index is vital for them.
This study demonstrates that the risk of NH increases as the maternal fasting period lengthens, which is consistent with a previous study (30). Li et al. found that the preoperative fasting of two hours for clear fluids and six to eight hours for solid food not only reduced the incidence of NH but also reduced the incidence of vomiting. When fasting, some metabolic pathways work together to maintain normal blood glucose concentrations, which are regulated by hormones and autonomic nerves. In pregnancy, hormone changes that induce abnormal glucose metabolism may lead to maternal hypoglycemia, resulting in NH. Fasting is required for women undergoing cesarean delivery to prevent gastric reflux and aspiration caused by anesthesia. As the Enhanced Recovery After Surgery Guidelines recommended, two-hour fasting for liquids and a small meal six hours prior to cesarean delivery is appropriate, but in clinical practice the period is eight hours (31). Hence, limiting the duration of fasting is a way to maintain high glucose, especially for some women with metabolic diseases.
Belonging to the class of drugs known as β2-adrenergic receptor agonists, ritodrine’s primary mechanism of action is to stimulate β2-receptors on smooth muscles in the uterus, leading to relaxation and reduced contractions. However, its use has been linked to an increased risk of neonatal hypoglycemia due to its ability to inhibit insulin secretion from pancreatic beta cells, which may result in decreased glucose uptake by tissues (32, 33). Therefore, when considering the use of ritodrine during labor and delivery, it is critical to weigh its potential risks against benefits and make informed clinical decisions.
As one of the predictors, women with GDM were prone to give birth to infants with hypoglycemia due to abnormal fetal glucose and insulin homeostasis. This is consistent with the finding of a study that the risk of developing hypoglycemia increased 1.32-fold (95% CI: 1.25, 1.38, P<0.001) per gestational week at GDM diagnosis (34). Women with GDM produce more insulin than healthy pregnant women, maternal hyperglycemia stimulates fetal pancreatic beta cells to produce more insulin, which can lead to increased fetal nutrient uptake and growth. However, after birth, these infants are no longer exposed to the high glucose levels from their mothers, but continue to produce high levels of insulin, which can result in hypoglycemia. At present, the prevalence of GDM continues to rise globally, close monitoring of blood glucose levels in both mothers with GDM and their infants is essential to prevent and manage NH (28, 35, 36).
This study showed that the gestational week is a protective factor. Gestational week is negatively correlated with the risk of developing NH. This is consistent with a study that showed that premature infants are at higher risk of hypoglycemia than full-term infants (37). This may be due to insufficient glycogen and fat storage and reduced glucose formation in preterm infants. Hence, premature infants should be a focus of NH prevention.
Premature infants are at a high risk of developing NH due to their immature glucose-regulating mechanisms and limited glycogen stores (38). Close monitoring of blood glucose levels can help prevent and manage NH in premature infants. Breast milk provides the optimal source of nutrition for infants, and initiating breastfeeding within 30 to 60 minutes of birth, as advised by the Academy of Breastfeeding Medicine (23), can help prevent NH. Additional strategies hypoglycemia includes administering intravenous glucose solutions to women before or during childbirth and providing oral glucose and electrolyte supplements. Moreover, reducing the fasting period for mothers with premature infants can aid in glucose storage and promote speedy recuperation after delivery.
As for neonatal factors, infants who experienced fetal distress were 1.76 times more likely to develop NH as healthy infants (95% CI: 1.11, 2.79, P=0.016). Fetal distress triggers the release of stress hormones such as cortisol, factors contributing to hypoglycemia in “stressed” or SGA infants may include inadequacy of caloric/energy intake relative to energy expenditure, depletion of hepatic glycogen stores, defective gluconeogenesis, increased sensitivity to insulin, or adrenocortical insufficiency. The pathogenesis of transient hyperinsulinemic hypoglycemia in SGA infants is poorly understood but may be related in part to suppression of fetal insulin secretion by premature induction of cortisol and/or catecholamines (39, 40).
Infants with low body mass index were 1.50 times more likely to develop NH as healthy infants (95% CI: 1.24, 1.84, P<0.001). As an indicator to evaluate body proportion and nutritional status of newborns, NBMI can be calculated by evaluating weight and length via ultrasound during pregnancy, and measured immediately after birth, providing a basis for NH risk assessment. NBMI does reflect nutrition status growth status of newborns to some extent although in SGA and LGA infants were not found statistically significant in this model.
In this study, SGA was found not to be associated with the development of NH and LGA was significant only in the univariate analysis. It was consistent with the results of a cohort study, which showed that the probability of hypoglycemia was similar or even lower in SGA and LGA infants, or absolute high or low-weight infants than in infants without these risk factors (18). On the contrary, another previous study indicated that SGA was associated with a higher possibility of developing hypoglycemia (41). However, LGA was also mentioned as a controversial risk factor for NH in a clinical report (22). Mainly because it is challenging to rule out maternal diabetes or hyperglycemia. NH most frequently occurs in infants with impaired glucogenesis or ketogenesis, which may occur with excessive insulin production, altered production of counterregulatory hormones, a lack of substrate supply, or a disorder of fatty acid oxidation. Plasma glucose homeostasis requires glucogenesis and ketogenesis to maintain normal rates of fuel use. One of the reasons for no association with gestational age may be that infants can absorb adequate nutrition from the diet of mothers.
In the logistic regression analysis, delivery mode, maternal obesity, and hepatitis B were not found to have a significant impact. This finding is consistent with previous studies (37). However, there is ongoing debate regarding the relationship between maternal obesity and NH, with some evidence suggesting a correlation in a multicenter study (42). This relationship may be influenced by the limited sample size, highlighting the need for future research involving a larger cohort to investigate this further.
This study has limitations. Feeding status and birth temperature may be influencing factors of NH. Newborns who experience delayed or inadequate breastfeeding after birth are at high risk of developing NH, as the infant’s glucose reserves deplete rapidly without timely replenishment. However, kangaroo-mother care is now widely practiced in the clinical settings, with infants being carried by their mothers with skin-to-skin contact for warmth, breastfeeding, safety, and affection. This is one reason why the study did not include feeding condition and birth temperature as variables (43). In this study, we used a blood glucose meter to measure blood glucose, which is convenient and economical. However, its accuracy at low blood glucose levels is controversial (44, 45). Additional multi-centric studies in diverse populations are needed.
Considering the elevated prevalence, heightened risk, and asymptomatic manifestation of NH (46), it is particularly important to establish clinical prediction models for both patients and healthcare providers. The potency of a prediction model hinges on its ability to precisely forecast the development of illnesses, identify high-risk cohorts with precision, and offer a benchmark for clinical intervention that helps mitigate the likelihood of disease onset.
The ROC curve was used to assess the sensitivity and specificity of the model, and the AUC evaluated its consistency. Discrimination is defined as low (AUC between 0.5~0.7), good (AUC>0.7) and excellent (AUC>0.9) (47). In this study, the AUC was 0.79 (specificity=0.82; sensitivity=0.62), suggesting that the model has good predictive ability.
Early identify high-risk newborns can help to prevent the incidence of NH and related complications (48). A nomogram is a statistical model that is built on several variables. To deliver a more personalized diagnosis or prognosis, nomograms rely on user-friendly digital interfaces, greater accuracy and more readily comprehensible prognoses. This nomogram is a tool for healthcare providers to identify early and intervene with infants at high risk of developing NH before delivery, to reduce the incidence of NH and its series of complications, therefore reducing the family care burden and socio-economic burden. The dynamic nomogram proposed in this study does not require manual calculation of the probability of the disease, only the input of specific variables and the calculation of the probability of the event. The development of the nomogram identifies predictors and provides new insights into the prediction and primary prevention of NH.
In conclusion, the advancing maternal age, an extended fasting period before delivery, the use of ritodrine, diagnosis of GDM, diagnosis of fetal distress, and an increase in NBMI increase the probability of developing NH. Conversely, an extended GW reduces the probability of developing NH.
The original contributions presented in the study are included in the article/supplementary material. Further inquiries can be directed to the corresponding authors.
The study was reviewed and approved by the Ethics Committee of Wuhan University Medical Department, and the approval number is WHU-LFMD-IRB2023028. Written informed consent was obtained from the participant's legal guardian/next of kin.
Y-QO and W-PH is the corresponding author. TW and Y-YH contributed equally and share the first authorship. Y-QO and W-PH conceived the study. TW and Y-YH collected, analyzed the data and wrote the original draft of the manuscript. WS collected the data and provided support, and SR revised and edited the article. All authors contributed to the article and approved the submitted version.
The authors declare that the research was conducted in the absence of any commercial or financial relationships that could be construed as a potential conflict of interest.
All claims expressed in this article are solely those of the authors and do not necessarily represent those of their affiliated organizations, or those of the publisher, the editors and the reviewers. Any product that may be evaluated in this article, or claim that may be made by its manufacturer, is not guaranteed or endorsed by the publisher.
1. Casertano A, Rossi A, Fecarotta S, Rosanio FM, Moracas C, Di Candia F, et al. An overview of hypoglycemia in children including a comprehensive practical diagnostic flowchart for clinical use. Front Endocrinol (Lausanne) (2021) 12:684011. doi: 10.3389/fendo.2021.684011
2. Shah R, Dai DWT, Alsweiler JM, Brown GTL, Chase JG, Gamble GD, et al. Association of neonatal hypoglycemia with academic performance in mid-childhood. JAMA (2022) 327(12):1158–70. doi: 10.1001/jama.2022.0992
3. Kaiser JR, Bai SS, Gibson N, Holland G, Lin TM, Swearingen CJ, et al. Association between transient newborn hypoglycemia and fourth-grade achievement test proficiency a population-based study. JAMA Pediatr (2015) 169(10):913–21. doi: 10.1001/jamapediatrics.2015.1631
4. Edwards T, Harding JE. Clinical aspects of neonatal hypoglycemia: a mini review. Front Pediatr (2020) 8:562251. doi: 10.3389/fped.2020.562251
5. Dani C, Corsini I. Guidelines for management of neonatal hypoglycemia: are they actually applicable? JAMA Pediatr (2020) 174(7):638–9. doi: 10.1001/jamapediatrics.2020.0632
6. Harris DL, Weston PJ, Harding JE. Incidence of neonatal hypoglycemia in babies identified as at risk. J Pediatr (2012) 161(5):787–91. doi: 10.1016/j.jpeds.2012.05.022
7. Maria Kristina Chappe M APRN. NNP-BC. hypoglycemia in high-risk infants within the immediate postnatal period. Neonatal Network (2020) 39(5):263–7. doi: 10.1891/0730-0832.39.5.263
8. Harris DL, Alsweiler JM, Ansell JM, Gamble GD, Thompson B, Wouldes TA, et al. Outcome at 2 years after dextrose gel treatment for neonatal hypoglycemia: follow-up of a randomized trial. J Pediatr (2016) 170:54–9. e92. doi: 10.1016/j.jpeds.2015.10.066
9. Shah R, Harding J, Brown J, McKinlay C. Neonatal glycaemia and neurodevelopmental outcomes: a systematic review and meta-analysis. Neonatology (2019) 115(2):116–26. doi: 10.1159/000492859
10. Glasgow MJ, Edlin R, Harding JE. Cost burden and net monetary benefit loss of neonatal hypoglycaemia. BMC Health Serv Res (2021) 21(1):121. doi: 10.1186/s12913-021-06098-9
11. Sen S, Cherkerzian S, Turner D, Monthe-Dreze C, Abdulhayoglu E, Zupancic JAF. A graded approach to intravenous dextrose for neonatal hypoglycemia decreases blood glucose variability, time in the neonatal intensive care unit, and cost of stay. J Pediatr (2021) 231:74–80. doi: 10.1016/j.jpeds.2020.12.025
12. Bouchghoul H, Mokhtari DM, Letourneau A, Bouyer J, Senat MV. Risk of hypoglycemia by anthropometric measurements in neonates of mothers with diabetes. Eur J Pediatr (2022) 181(9):3483–90. doi: 10.1007/s00431-022-04532-6
13. Stanescu DL, Stanley CA. Advances in understanding the mechanism of transitional neonatal hypoglycemia and implications for management. Clin Perinatol (2022) 49(1):55–72. doi: 10.1016/j.clp.2021.11.007
14. Edwards T, Liu G, Hegarty JE, Crowther CA, Alsweiler J, Harding JE. Oral dextrose gel to prevent hypoglycaemia in at-risk neonates. Cochrane Database Syst Rev (2021) 5:CD012152. doi: 10.1002/14651858.CD012152.pub3
15. de Bruin R, van Dalen SL, Franx SJ, Ramaswamy VV, Simons SHP, Flint RB, et al. The risk for neonatal hypoglycemia and bradycardia after beta-blocker use during pregnancy or lactation: a systematic review and meta-analysis. Int J Environ Res Public Health (2022) 19(15):9616. doi: 10.3390/ijerph19159616
16. Stanford-Surgery Policy Improvement Research and Education Center. Estimating power and sample size (2016). Available at: https://med.stanford.edu/content/dam/sm/s-spire/d.
17. Butorac Ahel I, Lah Tomulic K, Vlasic Cicvaric I, Zuvic M, Baraba Dekanic K, Segulja S, et al. Incidence and risk factors for glucose disturbances in premature infants. Medicina (Kaunas) (2022) 58(9):1295. doi: 10.3390/medicina58091295
18. Bromiker R, Perry A, Kasirer Y, Einav S, Klinger G, Levy-Khademi F. Early neonatal hypoglycemia: incidence of and risk factors. a cohort study using universal point of care screening. J Matern Fetal Neonatal Med (2019) 32(5):786–92. doi: 10.1080/14767058.2017.1391781
19. Ogunyemi D, Friedman P, Betcher K, Whitten A, Sugiyama N, Qu L, et al. Obstetrical correlates and perinatal consequences of neonatal hypoglycemia in term infants. J Matern Fetal Neonatal Med (2017) 30(11):1372–7. doi: 10.1080/14767058.2016.1214127
20. Stomnaroska-Damcevski O, Petkovska E, Jancevska S, Danilovski D. Neonatal hypoglycemia: a continuing debate in definition and management. Pril (Makedon Akad Nauk Umet Odd Med Nauki) (2015) 36(3):91–7. doi: 10.1515/prilozi-2015-0083
21. Turner D, Monthe-Dreze C, Cherkerzian S, Gregory K, Sen S. Maternal obesity and cesarean section delivery: additional risk factors for neonatal hypoglycemia? J Perinatol (2019) 39(8):1057–64. doi: 10.1038/s41372-019-0404-z
22. Committee on F, Newborn, Adamkin DH. Postnatal glucose homeostasis in late-preterm and term infants. Pediatr (2011) 127(3):575–9. doi: 10.1542/peds.2010-3851
23. Wight NE, Academy of Breastfeeding M. ABM clinical protocol #1: guidelines for glucose monitoring and treatment of hypoglycemia in term and late preterm neonates, revised 2021. Breastfeed Med (2021) 16(5):353–65. doi: 10.1089/bfm.2021.29178.new
24. Li Y, Ren X, He L, Li J, Zhang S, Chen W. Maternal age and the risk of gestational diabetes mellitus: a systematic review and meta-analysis of over 120 million participants. Diabetes Res Clin Pract (2020) 162:108044. doi: 10.1016/j.diabres.2020.108044
25. Marozio L, Picardo E, Filippini C, Mainolfi E, Berchialla P, Cavallo F, et al. Maternal age over 40 years and pregnancy outcome: a hospital-based survey. J Matern Fetal Neonatal Med (2019) 32(10):1602–8. doi: 10.1080/14767058.2017.1410793
26. Alsweiler JM, Harris DL, Harding JE, McKinlay CJD. Strategies to improve neurodevelopmental outcomes in babies at risk of neonatal hypoglycaemia. Lancet Child Adolesc Health (2021) 5(7):513–23. doi: 10.1016/S2352-4642(20)30387-4
27. Cremona A, Saunders J, Cotter A, Hamilton J, Donnelly AE, O'Gorman CS. Maternal obesity and degree of glucose intolerance on neonatal hypoglycaemia and birth weight: a retrospective observational cohort study in women with gestational diabetes mellitus. Eur J Pediatr (2020) 179(4):653–60. doi: 10.1007/s00431-019-03554-x
28. Shepherd E, Gomersall JC, Tieu J, Han S, Crowther CA, Middleton P. Combined diet and exercise interventions for preventing gestational diabetes mellitus. Cochrane Database Syst Rev (2017) 11:CD010443. doi: 10.1002/14651858.CD010443.pub3
29. Catalano PM. Obesity, insulin resistance, and pregnancy outcome. Reproduction (2010) 140(3):365–71. doi: 10.1530/REP-10-0088
30. Li Y, Su D, Sun Y, Hu Z, Wei Z, Jia J. Influence of different preoperative fasting times on women and neonates in cesarean section: a retrospective analysis. BMC Pregnancy Childbirth (2019) 19(1):104. doi: 10.1186/s12884-019-2254-2
31. Wilson RD, Caughey AB, Wood SL, Macones GA, Wrench IJ, Huang J, et al. Guidelines for antenatal and preoperative care in cesarean delivery: enhanced recovery after surgery society recommendations (Part 1). Am J Obstet Gynecol (2018) 219(6):523 e1– e15. doi: 10.1016/j.ajog.2018.09.015
32. Shimokawa S, Sakata A, Suga Y, Soda K, Itai S, Nagase K, et al. Incidence and risk factors of neonatal hypoglycemia after ritodrine therapy in premature labor: a retrospective cohort study. J Pharm Health Care (2019) 5:7. doi: 10.1186/s40780-019-0137-3
33. Seltzer HS. Drug-induced hypoglycemia - a review of 1418 cases. Endocrinol Metab Clin North Am (1989) 18(1):163–83. doi: 10.1016/S0889-8529(18)30395-5
34. Meloncelli NJL, Barnett AG, D'Emden M, De Jersey SJ. Effects of changing diagnostic criteria for gestational diabetes mellitus in Queensland, Australia. Obstet Gynecol (2020) 135(5):1215–21. doi: 10.1097/AOG.0000000000003790
35. Falzone N, Harrington J. Clinical predictors of transient versus persistent neonatal hyperinsulinism. Horm Res Paediatr (2020) 93(5):297–303. doi: 10.1159/000511139
36. Voormolen DN, de Wit L, van Rijn BB, DeVries JH, Heringa MP, Franx A, et al. Neonatal hypoglycemia following diet-controlled and insulin-treated gestational diabetes mellitus. Diabetes Care (2018) 41(7):1385–90. doi: 10.2337/dc18-0048
37. Mitchell NA, Grimbly C, Rosolowsky ET, O'Reilly M, Yaskina M, Cheung PY, et al. Incidence and risk factors for hypoglycemia during fetal-to-Neonatal transition in premature infants. Front Pediatr (2020) 8:34. doi: 10.3389/fped.2020.00034
38. Sharma A, Davis A, Shekhawat PS. Hypoglycemia in the preterm neonate: etiopathogenesis, diagnosis, management and long-term outcomes. Transl Pediatr (2017) 6(4):335–48. doi: 10.21037/tp.2017.10.06
39. Palladino AA, Bennett MJ, Stanley CA. Hyperinsulinism in infancy and childhood: when an insulin level is not always enough. Clin Chem (2008) 54(2):256–63. doi: 10.1373/clinchem.2007.098988
40. Stanley CA, Thornton PS, De Leon DD. New approaches to screening and management of neonatal hypoglycemia based on improved understanding of the molecular mechanism of hypoglycemia. Front Pediatr (2023) 11:1071206. doi: 10.3389/fped.2023.1071206
41. Hosagasi NH, Aydin M, Zenciroglu A, Ustun N, Beken S. Incidence of hypoglycemia in newborns at risk and an audit of the 2011 American academy of pediatrics guideline for hypoglycemia. Pediatr Neonatol (2018) 59(4):368–74. doi: 10.1016/j.pedneo.2017.11.009
42. Vargas-Sanabria AP, Niño Tovar MA, Acosta Rodriguez AL, Acosta Rodriguez AL, Pérez LA. Relationship between gestational obesity and adverse perinatal outcomes: a multicenter study. Andes Pediatr (2021) 92(4):548–55. doi: 10.32641/andespediatr.v92i4.3302
43. Koreti M, Muntode Gharde P. A narrative review of kangaroo mother care (KMC) and its effects on and benefits for low birth weight (LBW) babies. Cureus (2022) 14(11):e31948. doi: 10.7759/cureus.31948
44. Kim HN, Yoon SY. Comparative study of i-SENS glucometers in neonates using capillary blood samples. Clin Chem Lab Med (2021) 59(6):1133–41. doi: 10.1515/cclm-2020-1367
45. Ho HT, Yeung WKY, Young BWY. Evaluation of ''point of care'' devices in the measurement of low blood glucose in neonatal practice. Arch Dis Child-Fetal (2004) 89(4):F356–F9. doi: 10.1136/adc.2003.033548
46. Adamkin DH. Low blood sugar levels in the newborn infant: do changing goal posts matter? Semin Fetal Neonatal Med (2021) 26(3):101202. doi: 10.1016/j.siny.2021.101202
47. Steyerberg EW, Vickers AJ, Cook NR, Gerds T, Gonen M, Obuchowski N, et al. Assessing the performance of prediction models: a framework for traditional and novel measures. Epidemiology (2010) 21(1):128–38. doi: 10.1097/EDE.0b013e3181c30fb2
Keywords: neonatal hypoglycemia, nomogram, predictive model, retrospective study, risk factors
Citation: Wu T, Huang Y-Y, Song W, Redding SR, Huang W-P and Ouyang Y-Q (2023) Development of a prediction model for neonatal hypoglycemia risk factors: a retrospective study. Front. Endocrinol. 14:1199628. doi: 10.3389/fendo.2023.1199628
Received: 03 April 2023; Accepted: 19 June 2023;
Published: 17 July 2023.
Edited by:
Michael Freemark, Duke University, United StatesReviewed by:
Paul Stephen Thornton, Cook Children’s Medical Center, United StatesCopyright © 2023 Wu, Huang, Song, Redding, Huang and Ouyang. This is an open-access article distributed under the terms of the Creative Commons Attribution License (CC BY). The use, distribution or reproduction in other forums is permitted, provided the original author(s) and the copyright owner(s) are credited and that the original publication in this journal is cited, in accordance with accepted academic practice. No use, distribution or reproduction is permitted which does not comply with these terms.
*Correspondence: Yan-Qiong Ouyang, b3V5YW5neXFAd2h1LmVkdS5jbg==; Wei-Peng Huang, aHVhbmc3ZG9jQDE2My5jb20=
†These authors have contributed equally to this work and share first authorship
Disclaimer: All claims expressed in this article are solely those of the authors and do not necessarily represent those of their affiliated organizations, or those of the publisher, the editors and the reviewers. Any product that may be evaluated in this article or claim that may be made by its manufacturer is not guaranteed or endorsed by the publisher.
Research integrity at Frontiers
Learn more about the work of our research integrity team to safeguard the quality of each article we publish.