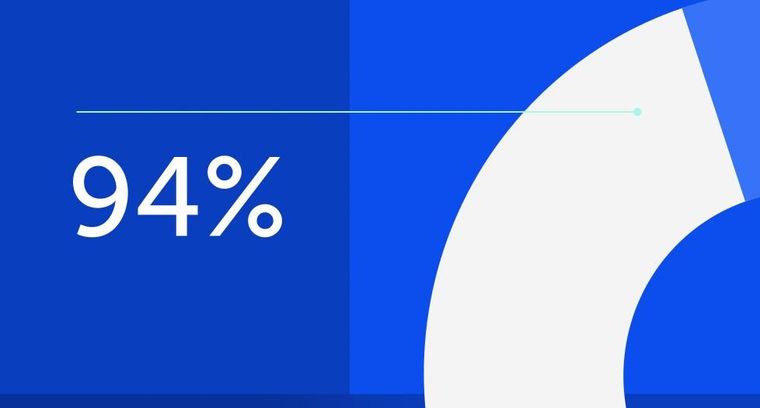
94% of researchers rate our articles as excellent or good
Learn more about the work of our research integrity team to safeguard the quality of each article we publish.
Find out more
ORIGINAL RESEARCH article
Front. Endocrinol., 20 July 2023
Sec. Clinical Diabetes
Volume 14 - 2023 | https://doi.org/10.3389/fendo.2023.1192491
Background: Type 2 diabetes mellitus (T2DM) is a disease of public health importance globally with an increasing burden of undiagnosed pre-diabetes and diabetes in low- and middle-income countries, Nigeria in particular. Pre-diabetes and diabetes are established risk factors for cardiovascular complications. However, data are scanty on the current prevalence of these conditions in Nigeria, based on haemoglobin A1c (HbA1c) diagnosis as recommended by the WHO in 2009. We aimed to determine the prevalence of pre-diabetes, diabetes, and undiagnosed diabetes among the adult population of Nigeria using HbA1c.
Methodology: A cross-sectional, multi-site population study was carried out in selected states in Nigeria (namely, Ekiti, Lagos, Osun, Oyo, and Kwara states) involving 2,708 adults (≥18 years) in rural and urban community dwellers, without prior diagnosis of pre-diabetes or diabetes. Participants with ongoing acute or debilitating illnesses were excluded. Data were collected using an interviewer-administered pretested, semi-structured questionnaire. Socio-demographic, clinical (weight, height, blood pressure, etc.), and laboratory characteristics of participants including HbA1c were obtained. Data were analysed using STATA version 16.
Results: The mean age of participants was 48.1 ± 15.8 years, and 65.5% were female. The overall prevalence of pre-diabetes and undiagnosed diabetes was 40.5% and 10.7%, respectively, while the prevalence of high blood pressure was 36.7%. The prevalence of pre-diabetes was the highest in Lagos (48.1%) and the lowest in Ekiti (36.7%), while the prevalence of diabetes was the highest in Kwara (14.2%) and the lowest in Ekiti (10%). There was a significant association between age of the participants (p< 0.001), gender (p = 0.009), educational status (p = 0.008), occupation (p< 0.001), tribe (p = 0.004), marital status (p< 0.001), blood pressure (p< 0.001), and their diabetic or pre-diabetic status. Independent predictors of diabetes and pre-diabetes include excess weight gain, sedentary living, and ageing. Participants within the age group 45–54 years had the highest total prevalence (26.6%) of pre-diabetes and diabetes.
Conclusion: Over half of the respondents had pre-diabetes and diabetes, with a high prevalence of undiagnosed diabetes. A nationwide screening campaign will promote early detection of pre-diabetes and undiagnosed diabetes among adult Nigerians. Health education campaigns could be an effective tool in community settings to improve knowledge of the risk factors for diabetes to reduce the prevalence of dysglycaemia.
The growing burden of diabetes mellitus (DM), particularly type 2 (T2DM), has become a thing of global concern. Approximately 537 million people are affected by DM with a projected increase to 783 million by 2045 (1). Africa is predicted to have the largest percentage rise (134%) in diabetes prevalence from 24 million in 2019 to 55 million in 2045 when compared to other regions of the world (1). This constitutes a great public health and economic concerns, as the African continent harbours many poorly resourced countries.
Impaired fasting glucose (IFG) and impaired glucose tolerance (IGT) are pre-diabetic states, which can exist undetected for many years with irreversible damage to vital organs of the body (2, 3).
Pre-diabetes will progress to overt T2DM in approximately 25% of patients within 3–5 years, and up to 70% of individuals with pre-diabetes will develop overt diabetes within their lifetime in the absence of intervention (2). The current global prevalence of IGT is 10.6%, out of which about three-quarters live in low- and middle-income countries (LMICs), with a projected rise up to 11.4% by 2045 (1). A recent meta-analysis involving 15 studies in Nigeria, based on American Diabetes Association and World Health Organization criteria, reported the pooled prevalence of pre-diabetes as 13.2% (CI: 5.6%–23.2%) and 10.4% (CI: 4.3%–12.9%), respectively (4). This translates to 15.8 million and 12.5 million adult Nigerians living with pre-diabetes. Notably, there is a wide heterogeneity among the included studies, and only two of them used haemoglobin A1c (HbA1c) for the diagnosis of pre-diabetes. A study performed in Ibadan, southwest zone, on the prevalence and predictors of pre-diabetes among the administrative staff of a tertiary health centre reported a prevalence of 22.3%, suggesting there could be a wide variation depending on the socio-economic group studied (5). In that study, male sex, positive family history of diabetes mellitus, alcohol intake, and inadequate moderate-intensity physical activity were identified in the study as independent predictors.
Pre-diabetes (IGT and IFG) indicate an already heightened risk of cardiovascular disease (3, 6, 7), and their recognition compels urgent interventions that can lead to the prevention of type 2 diabetes and its complications (8). Fasting blood glucose and 2-h oral glucose tolerance test (OGTT) are the traditional methods of screening and diagnosis of diabetes. Following the recommendation by an expert committee in 2009, the use of HbA1c for the diagnosis of diabetes and pre-diabetes was also approved for the diagnosis of these dysglycaemic states (9). Also, potential limitations due to haemoglobinopathy have been minimised with newer standardised methods and assays (10).
The use of HbA1c as a means of diagnosis of dysglycaemia by clinicians is gradually increasing in Africa including Nigeria, but sparse data exist in our region about HbA1c-based prevalence of pre-diabetes and diabetes. Additionally, no large-scale A1c-based regional or national study exists to date on the burden of pre-diabetes and undiagnosed diabetes among Nigerians. Pre-diabetes is reversible with appropriate lifestyle interventions and selected pharmacology and thus can potentially reduce the burden of diabetes and its complications. To this end, we undertook a multistate large-scale cross-sectional study involving the adult population in southwestern and northcentral Nigeria, and here, we present the findings (Figure 1).
The study was a population-based descriptive cross-sectional study.
The study population included individuals aged ≥18 years and residents in the selected states without a prior diagnosis of pre-diabetes or diabetes mellitus. They had no ongoing acute or chronically debilitating illness.
Previous studies suggest that the prevalence of pre-diabetes in Nigeria ranged between 2.0% and 37.2%, while that of diabetes varied from 5% to 10%. 8 For a conservative sample size, we assumed the upper limits of the prevalence. Therefore, we assumed that the prevalence of the glycaemic disorder is 37.2%, at a 95% confidence level, 2.5% error margin, and a design effect of 1.5.
where Zα is the standard normal deviate at 95% confidence level = 1.96, P is the prevalence of pre-diabetes or diabetes (glycaemic disorder) = 37.2% = 0.372, q = 1 − p, and d is the degree of precision = 2.5% = 0.025.
A minimum sample size of 2,151 was obtained.
A non-response rate of 20% was estimated to give a sample size of 2,151/((1 − 0.2)) = 2,689.
A multistage sampling technique was used to select communities from all parts of each selected state to represent both the urban and rural settlements of the states. The states were purposively selected based on the availability of researchers and willingness to participate in the study.
Stage 1: each state was to select a minimum of two local government areas (LGA) by simple random sampling.
Stage 2: the selected LGAs were divided into rural and urban LGAs, and a minimum of 300 participants were assigned to each state.
Stage 3: a sampling frame listing all political wards in the selected LGAs was developed. Two wards were selected using a simple random sampling technique.
A pretested, semi-structured questionnaire was used to obtain the data. Data were collected electronically using Android phones loaded with the questionnaires and captured on REDCAP software version 5.19.7. The questionnaire was developed using information from literature, researchers’ knowledge of the topic, and adaptation of questions from past related studies. The draft questionnaire was face validated by experts to ensure the adequacy of the questions to provide answers to the study objectives. It was then pretested in a community that was excluded from the study sites to ensure the clarity, comprehensiveness, conciseness, and consistency of questions. The questionnaire was translated into the local language and back-translated into English to ascertain the accuracy of the translation. Information collected from the study participants included socio-demographic characteristics (age, gender, etc.), clinical (weight, height, blood pressure, etc.), and laboratory profiles of the individuals (glycated haemoglobin). Prior history of gestational diabetes, hypertension, family history of diabetes, dietary and lifestyle history, and selected disease screening practices including diabetes were collected.
Mobilisation campaigns were performed in the communities through walk-round sensitisation in the selected wards. Adult residents were invited to the primary health care centre nearest to them for interview and screening after written informed consent was obtained from each eligible individual. The interviewers were graduates of tertiary institutions and were well-versed in community-based quantitative data collection. They were trained to take anthropometric measurements: weight, height, waist circumference, hip circumference, and blood pressure. All interviewers were fluent in English and Yoruba languages. Weights were measured to the nearest 0.1 kg with portable bathroom scales (HARSON), which were validated with an object with a known weight.
The scales were also regularly checked and adjusted to zero after every 10th measurement. The participants were weighed wearing light clothing, with emptied pockets, no attachment to their clothing, and without shoes. Heights were measured with a mobile stadiometer placed on a level ground against a flat vertical surface, with participants standing erect without shoes and with the eyes looking horizontally and the feet together on a horizontal level. Heights were recorded to the nearest 0.1 cm. Standardisation checks on the height boards were performed periodically during the study period. Body mass index (BMI) was calculated using the following formula: BMI = weight/(height in meters)2 (kg/m2). BMI was classified as normal, 18.5–24.9 kg/m2; overweight, 25–29.9 kg/m2; class I obesity, 30–34.9 kg/m2; class II obesity, 35–39.9 kg/m2; class III obesity, 40 kg/m2 or higher.
Waist circumference was measured using a flexible tape measure, with the subject standing and breathing normally. The examiner stood on the right side of the subject, and then the inferior margin of the last rib and iliac crest were located and identified with a pen. A tape measure was placed at the midpoint between the landmarks in a way that the tape was in a horizontal plane parallel to the floor. The waist circumference was measured and read at the level of the tape to the nearest 0.1 cm. A waist circumference greater than or equal to 94 cm in men and greater than or equal to 80 cm in women was taken as indicative of truncal obesity.
Hip measurement was made while the participant was in a relaxed standing position with the feet together. The measuring tape was placed horizontally around the maximum circumference of the buttocks. The hip circumference was measured and read at this level to the nearest 0.1 cm.
Systolic and diastolic blood pressure were assessed using an automated digital blood pressure monitor (OMRON M3) with an appropriately sized cuff for participants’ arms. The measurements were taken after each participant had rested for at least 5 min, were well seated, resting their back with feet on the ground, and not distracted. Blood pressure was measured twice using the left arm, with an interval of 2 min. Blood pressure (BP) measured was categorised using standard guidelines such as The Seventh Report of the Joint National Committee on Prevention, Detection, Evaluation, and Treatment of High Blood Pressure: the JNC 7 report. In this study, hypertension was defined as an average of two measurements of systolic and/or diastolic blood pressure (second and third blood pressure measurement) that is ≥140/90 mmHg in any adult or self-reported treatment of hypertension with antihypertensive medication taken in the past weeks. Phlebotomists were recruited in each state to collect 2 mL of venous blood under an aseptic condition from the participants out of which drops were used to determine their random blood glucose (RBG). The remaining blood samples were stored in EDTA bottles, which were placed in cold boxes and transported to a central laboratory for the estimation of HbA1c.
Glycated haemoglobin was determined using a mobile point-of-care equipment (DCA Vantage Analyzer by Siemens, Erlangen, Germany) certified by the National Glycohemoglobin Standardization Program (NGSP) and International Federation of Clinical Chemistry and Laboratory Medicine (IFCC). In diabetes, the glucose moiety is gradiently added to the haemoglobin chain of the red blood cells, and this can be retrospectively assessed using the HbA1c, reflecting blood glucose level corresponding to the lifespan of a red blood cell. Siemens’ DCA HbA1c test is based on an immunoassay technique and is standardised to both NGSP and IFCC reference methods. HbA1c tests using the device demonstrated tight precision, with CVs of 2.0% or less in the important clinical ranges for diagnosing and monitoring. These results exceed the acceptance criteria for precision as specified in the Clinical and Laboratory Standards Institute (CLSI) guidelines. They also demonstrated excellent correlation (R = 0.99) of the DCA HbA1c test with the NGSP-certified Tosoh G7 central laboratory HbA1c method. Although potential limitation due to haemoglobinopathy has been minimised with newer standardised methods and assays (9), the possibility of error in those with haemoglobinopathy cannot be completely eliminated (1). In addition, participants had RBG levels estimated using a glucometer (Accu-Chek Active glucometer and strips). An RBG level of 200 mg/dL or higher indicates diabetes.
Phlebotomists were recruited in each state to collect 2 mL of venous blood under an aseptic condition from the participants out of which drops were used to determine their RBG. The remaining blood samples were stored in EDTA bottles, which were placed in cold boxes and transported to the laboratory for the estimation of HbA1c. Pre-diabetes is defined with glycated haemoglobin levels 5.7%–6.4% and undetected diabetes ≥6.5% (1, 3, 11). An RBG level of 200 mg/dL or higher indicate diabetes (1).
Data were analysed using the STATA version 16 package program. Descriptive statistics such as means and standard deviation were used to summarize categorical variables, while proportions were used for qualitative variables. The associations between the categorical variables were performed using the chi-square test. The comparison of means was tested using Student’s t-test for quantitative variables. Associations between continuous variables were determined using correlation analysis. Multiple logistic regression was used to determine predictors of pre-diabetes and diabetes.
All statistical analyses were carried out at a level of significance<0.05.
Ethical approval was obtained from the Ethical Committees in all the selected states and the National Health Research Ethics Committee of Nigeria (NHREC) [Ref No.: NHREC/01/01/2007]. Permission was obtained from the local heads of the communities and the primary health care unit of the LGA secretariats. Written informed consent was obtained from each participant before commencing with the data collection, and participants had the right to withdraw from the study at any time during data collection.
A total of 2,708 adults were studied in the selected five states. Out of the 2,708 participants, 1,759 (65.5%) were female, 2,325 (86.6%) were Yoruba, and 1,917 (71.4%) were married. The mean age of the participants was 48.1 ± 15.8 years. A total of 872 (32.5%) participants had up to tertiary education, while 393 (14.7%) never attended school (Table 1). Participants who had never smoked were 2,465 (92.3%), while 141 (67.1%) of those that signified to have smoked before were current smokers. A total of 1,994 (74.8%) of the participants had never taken alcohol before, and 209 (53.2%) out of those who consumed alcohol were occasional drinkers. Also, 2,474 (92.7%) of the participants consumed fruits at least once weekly. The overall prevalence of high blood pressure was 36.7%. The prevalence of pre-diabetes was the highest in Lagos (48.1%) and the lowest in Ekiti (36.7%), while the prevalence of diabetes was the highest in Kwara (14.2%) and the lowest in Ekiti (10%) (Table 2 and Figure 2). Out of the 290 participants with diabetes, 244 (84.1%) reaffirmed no prior knowledge of having diabetes, giving a true prevalence of undiagnosed diabetes as 9.6%.
The ratio of previously diagnosed to newly diagnosed diabetes was 1.11/9.60 = 0.11. The prevalence of pre-diabetes and diabetes was the highest in Lagos State at 29.7% and 25.2%, respectively (Figure 2). The study showed statistically significant associations between the age of the participants (p< 0.001), gender (p = 0.009), educational status (p = 0.008), occupation (p< 0.001), tribe (p = 0.004), marital status (p< 0.001), blood pressure status (p< 0.001), and their diabetic or pre-diabetic status. Participants within the age group 45–54 years had the highest total prevalence (26.6%) of pre-diabetes and diabetes, and 765 (56.2%) of participants who were either pre-diabetic or diabetic had high blood pressure. No participant within the age range ≤24 years had diabetes as shown in Table 3.
There was a significant association between their age (p< 0.001), gender (p = 0.003), educational status (p = 0.025), occupation (p< 0.001), marital status (p< 0.001), blood pressure status (p< 0.001), and their knowledge of whether they have diabetes or not. Participants within the age range 55–64 years had the highest prevalence of undiagnosed diabetes (30.7%), and 57.1% of the participants with high blood pressure had undiagnosed diabetes (Table 4). The multivariable logistic regression analysis is shown in Table 5, demonstrating the relationship between predictor variables and diabetes (Table 5A) and pre-diabetes (Table 5B). Compared to the reference categories, women, those who had secondary or higher education, retired and unemployed, not having high blood pressure, and overweight/obese have higher odds of developing diabetes. For pre-diabetes, the predictor variables were 45, 64, or >75 years of age, married, widowed, obese, retired, and those with occupations such as civil servants, petty trading/business, and professionals.
Table 5 Multivariable analysis showing relationship between predictor variables and (a) diabetes and (b) pre-diabetes.
Although it is well established that there is an increasing burden of pre-diabetes and undiagnosed diabetes in sub-Saharan Africa (1), most of the evidence is based on blood glucose as a diagnostic method. This study provides evidence, perhaps for the first time on a large scale, that the prevalence of A1c-based pre-diabetes and diabetes among Nigerians is disturbingly high: 40.5% and 10.7%, respectively. Also, the majority (84.2%) of those with diabetes were previously undiagnosed, implying that the actual prevalence of diabetes was 9.6%. To the best of our knowledge, this is the first study in Nigeria with such large scope to investigate dysglycaemia using HbA1c across states in a region of the country.
The prevalence rate of pre-diabetes in this study is higher than estimates from the International Diabetes Federation (IDF) and those reported from different parts of Africa. Our prevalence rate for diabetes is similar to the finding by Bashir and colleagues in a meta-analysis involving 15 studies in Nigeria (4) and the study by Ekpeyong and co-workers (12). The latest diabetes atlas by the IDF estimated the overall prevalence of pre-diabetes and diabetes in sub-Saharan Africa (SSA) as 17.7% and 4.5%, respectively (1). Studies from different countries in SSA indicate a wide variation in the prevalence of pre-diabetes and diabetes (4, 13–20). Similarly, there are wide variations in the prevalence of pre-diabetes and diabetes reported within Nigeria, ranging between 6.3% and 29% for pre-diabetes and 0.8% and 9.3% for diabetes (4, 5, 21–25). However, these studies used glucose testing methods and not HbA1c. Data on studies based on the latter are very scarce in Nigeria and even the SSA continent. One A1c-based study in Botswana (26) reported a higher prevalence rate of pre-diabetes and diabetes (54.3% and 14.4%, respectively) than our findings.
In Nigeria, a study was performed among 10 communities within a single northern state, mostly Hausa-Fulani ethnic groups, which compared both methods of blood glucose and HbA1c (27). The authors reported an HbA1c-based prevalence of pre-diabetes and diabetes at 9.3% and 3.5%, respectively. The OGTT-based results in the same population were 17.2% and 9.3% for prevalence rates of pre-diabetes and diabetes, respectively. In contrast to most reports, the study by Lawal and co-workers indicate higher prevalence rates of dysglycaemia using blood glucose than A1c (28–31). The reason for the wide disparities between the A1c-based values in that study and our findings could be ethnic variation. Studies have shown that race and ethnicity can influence the results of HbA1c (32–34). Some epidemiological studies among the Northern Hausa-Fulani ethnic groups have reported lower prevalence rates of dysglycaemia compared to ethnic tribes in the southern part of the country (35–39). Adeloye et al. in their meta-analytic work report the prevalence rates in the northern part of Nigeria to range between 2.0% and 4.6%, while that of Southern Nigeria ranges between 3.2% and 8.5% (39). Additionally, the differences could be due to the HbA1c assay methods used in both studies. While we used DCA Vantage analyser based on immunoassays in this study, the work by Lawal was performed using the boronate affinity method. Although none of these methods is the gold standard, both are certified by NGSP and IFCC. However, the A1c result from boronate affinity is based on a ratio of glycated to non-glycated haemoglobin regardless of species and hence could be falsely low in the presence of haemoglobin F (40). Finally, our findings could also reflect the rapid increase in the prevalence of diabetes in the country. Considering the southwest zone where most of the participants in this study resided, a prevalence rate of 0.8% was reported in 1998 from Ibadan (41). However, in 2013, Alebiosu et al. reported a prevalence rate of 5.05% from the southwest zone (42).
The International Expert Committee (IEC) in 2009 recommended a cutpoint of 6.5% for the diagnosis of diabetes and 5.7%–6.4% for pre-diabetes (10). However, this remains controversial due to inconsistent reports of a rise in the incidence of retinopathy at this threshold (43–46). The use of HbA1c and fasting blood glucose has been reported to have limited agreement with OGTT results (47). This limitation and under-performance of HbA1c in African Americans exist even when participants in the study did not have haemoglobinopathy and anaemia (48). Examination of the relationship between HbA1c and plasma glucose concentrations in the USA showed that the African Americans demonstrated a shift of the curve to the right by 0.65%, compared to the non-Hispanic Whites and Mexican Americans, implying a higher threshold of A1c in the African Americans (49, 50). Moreover, the sensitivity of HbA1c to a diagnosis of diabetes is the highest among the Chinese, Asian Indians, and Africans, compared to other races (51–53). The HbA1c-based high prevalence rates of pre-diabetes and diabetes in our study is probably supporting the widely reported high level of HbA1c in Blacks compared to Whites (54), such that with the same cutoff values, more Blacks will be diagnosed with dysglycaemia compared to Whites. As shown by Vega-Vazquez and colleagues, using A1c for diagnosis have more impact on the diagnosis of pre-diabetes than diabetes (31). Our study and the one from Botswana by Omech and co-authors (26) support this suggestion.
The magnitude of undiagnosed diabetes in this study (84.2%) is even higher than the 60% reported by IDF for the African region (1). These participants most likely have type 2 diabetes since that is the form that is insidious. As the prevalence rate of diabetes in Nigeria increases, there is no commensurate improvement in the health system, access to health care, and screening, further worsened by an abysmally low number of people covered by health insurance in the country (55). This is very disturbing considering the consequent risk for cardiovascular disease and other microvascular complications in sub-Saharan Africa (6, 56). When people lack knowledge of their health condition, the consequence is increased mortality and morbidity, which could have been prevented (57, 58). As indicated in our study, older age and hypertensive individuals have been reported as having a higher risk of undiagnosed diabetes in developing countries (59).
Consistent with established knowledge, our study supports that the commonest risk factor for glucose intolerance is excess weight gain (60). Overweight/obesity was common among the predictors for both pre-diabetes and diabetes. The next predictor is physical inactivity indicated in our study as those retired, unemployed, or professionals who require little expenditure of energy. Ageing is also associated with the development of glucose intolerance. The pre-diabetic and diabetic participants in our study were predominantly between 40 and 64 years old. However, age was only an independent predictor in the pre-diabetic group and not diabetes. This could be due to the stronger influence of HbA1c on pre-diabetes (31), underscored by the fact that HbA1c increases with age independent of glucose intolerance (45, 61). Age above 40 years, BMI > 30, and dyslipidaemia have been identified as predictors of pre-diabetes with a high level of certainty (62, 63). Insulin resistance is the pathophysiological mechanism underlying all these predictors (64, 65), while beta cell dysfunction accounts for the progression of pre-diabetes to diabetes and further deterioration of glycaemic control in those with established diabetes (66–68).
This study found a higher prevalence of pre-diabetes and diabetes among women compared to men. This result is similar to the findings of previous studies where more women than men had diabetes (27, 69). The occurrence of major life events has been shown through longitudinal studies to be associated with a rapid increase in HbA1c. For women, this could be attributed to stress, families, and other psychosocial variables (70, 71). Studies have also revealed that increased domestic duties and unpaid labour among women may result in conflicting feelings and possibly stress-induced diabetes (71–73). It could also be attributed to both physiological factors like rising rates of hypertension and obesity in women as well as unfavourable consequences of food and lifestyle choices. Direct causation of diabetes by stressors has not been established. More likely, the stressors unmasked symptoms in people who are already predisposed and possibly in the subclinical stage of the disease process (74, 75). Therefore, it is essential that women receive substantial health education on the risk factors for diabetes in a bid to reduce its prevalence.
This study was carried out in some selected states in the southwest and northcentral zones (only two of the six geopolitical zones in Nigeria); therefore, its findings might not be a representation of other states in Nigeria. In addition, the presence of sickle cell trait among the participants was not excluded, as the potential to cause interference in the HbA1c assay method used in this study could not be completely excluded.
Also, performing both HbA1c and fasting blood glucose simultaneously would have further reduced those that could have been falsely diagnosed as having diabetes or pre-diabetes. However, this approach is more practical and recommended in clinical practice rather than in a population research setting (61). Finally, the cross-sectional nature of the study also did not allow for causal inferences.
Although HbA1c is globally standardised, acceptable, convenient, and useful for screening even in population research, in view of the controversy about the recommended cutoff for dysglycaemia in some races including black Africans, a prospective country-wide study extended all over the country is needed to validate its usefulness in Nigerians and recommend an appropriate reference range for our population.
The burden of pre-diabetes and diabetes assessed by haemoglobin A1c among community dwellers in the southwestern and northeastern states of Nigeria is very high. Additionally, the proportion of those with undiagnosed diabetes is unacceptably high. Obesity and sedentary living are strong predictors of these abnormal glycaemic states. To promote early detection, screening of all adults for confirmation of their diabetic status should be encouraged to obtain true estimates of individuals with pre-diabetes among the Nigerian population. To achieve this nationwide estimate and achieve completeness of data, statistics on pre-diabetes and diabetes should be captured in the National Demographic Health Survey, and this should be regularly updated. Health education campaigns should be organised especially in community settings to improve knowledge of the risk factors for diabetes to achieve a significant fall in its prevalence. Health decision makers, governmental organisations, non-governmental organisations, and health care professionals in Nigeria must collaborate in this regard to reduce the burden of diabetes.
The raw data supporting the conclusions of this article will be made available by the authors, without undue reservation.
The studies involving human participants were reviewed and approved by Joint University of Ibadan and University College Hospital Ethical Committee; National Health Research Ethics Committee of Nigeria. The patients/participants provided their written informed consent to participate in this study.
IOA contributed to the conceptualisation, design of the study, overall project administration, and data collection, analysis, and interpretation and initiated the writing of the original draft and manuscript review. WB contributed to the conceptualisation and design of the study; assisted in the project administration and data collection, analysis, and interpretation; and contributed to the writing of the original draft, manuscript review, and editing. OBO, GA, DS, OB, MO, and BA-M contributed to the design of the study, data collection, and interpretation, manuscript review, and editing. OO, OF, FD, EO, IA, PA, AA, OA, AO, EG-A, IO, OE, TE, AL, FO, AY, TA, AO, AHA, AB, and SN contributed to the data collection and interpretation, and manuscript review and editing. All authors contributed to the article and approved the submitted version.
The authors thank the United Nations Children’s Education Fund through the International Institute for Tropical Agriculture for the donation of HbA1c kits and analysers. Also, the authors are grateful to the Epidemiology and Biostatistics Research Unit, Institute for Advanced Medical Research and Training College of Medicine, University of Ibadan, the Diabetes Epidemiological Group of Nigeria (DEGON), the Department of Medicine, College of Medicine, University of Ibadan, the Federal Teaching Hospital, Ido-Ekiti, and the Ministries of Health in Oyo, Lagos, Ekiti, Osun, and Kwara States.
The authors declare that the research was conducted in the absence of any commercial or financial relationships that could be construed as a potential conflict of interest.
All claims expressed in this article are solely those of the authors and do not necessarily represent those of their affiliated organizations, or those of the publisher, the editors and the reviewers. Any product that may be evaluated in this article, or claim that may be made by its manufacturer, is not guaranteed or endorsed by the publisher.
1. IDF. Diabetes atlas: international diabetes federation (2021). Available at: www.diabetesatlas.org.
2. Nathan DM, Davidson MB, DeFronzo RA, Heine RJ, Henry RR, Pratley R, et al. Impaired fasting glucose and impaired glucose tolerance: implications for care. Diabetes Care (2007) 30(3):753–9. doi: 10.2337/dc07-9920
3. Hostalek U. Global epidemiology of prediabetes - present and future perspectives. Clin Diabetes Endocrinol (2019) 5:5. doi: 10.1186/s40842-019-0080-0
4. Bashir MA, Yahaya AI, Muhammad M, Yusuf AH, Mukhtar IG. Prediabetes burden in Nigeria: a systematic review and meta-analysis. Front Public Health (2021) 9:762429. doi: 10.3389/fpubh.2021.762429
5. Martins SO, Folasire OF, Irabor AE. Prevalence and predictors of prediabetes among administrative staff of a tertiary health centre, southwestern Nigeria. Ann Ib Postgrad Med (2017) 15(2):114–23.
6. Gottwald-Hostalek U, Gwilt M. Vascular complications in prediabetes and type 2 diabetes: a continuous process arising from a common pathology. Curr Med Res Opin (2022) 38(11):1841–51. doi: 10.1080/03007995.2022.2101805
7. Honigberg MC, Zekavat SM, Pirruccello JP, Natarajan P, Vaduganathan M. Cardiovascular and kidney outcomes across the glycemic spectrum: insights from the UK biobank. J Am Coll Cardiol (2021) 78(5):453–64. doi: 10.1016/j.jacc.2021.05.004
8. Ghannam N, Alahmed S, Aldahash R, Aljohani N, Alshammary A, Amir A, et al. Addressing the continuum of dysglycaemia and vascular complications in prediabetes and type 2 diabetes: need for early and intensive treatment. Diabetes Metab Syndr Obes (2023) 16:105–15. doi: 10.2147/DMSO.S396621
9. Zechmeister B, Erden T, Kreutzig B, Weber M, Joly P, Erdmann J, et al. Analytical interference of 33 different hemoglobin variants on HbA1c measurements comparing high-performance liquid chromatography with whole blood enzymatic assay: a multi-center study. Clin Chim Acta (2022) 531:145–51. doi: 10.1016/j.cca.2022.03.028
10. Gillett MJ. International expert committee report on the role of the A1c assay in the diagnosis of diabetes. Diabetes Care (2009) 32(7):1327–34.
11. Sattar N, Gill JM. Type 2 diabetes in migrant south asians: mechanisms, mitigation, and management. Lancet Diabetes Endocrinol (2015) 3(12):1004–16. doi: 10.1016/S2213-8587(15)00326-5
12. Ekpenyong CE, Akpan U, Ibu JO, Nyebuk DE. Gender and age specific prevalence and associated risk factors of type 2 diabetes mellitus in uyo metropolis, south Eastern Nigeria. Diabetologia Croatica (2012) 41(1):17–28.
13. Bigna JJ, Nansseu JR, Katte JC, Noubiap JJ. Prevalence of prediabetes and diabetes mellitus among adults residing in Cameroon: a systematic review and meta-analysis. Diabetes Res Clin Pract (2018) 137:109–18. doi: 10.1016/j.diabres.2017.12.005
14. Mayega RW, Guwatudde D, Makumbi F, Nakwagala FN, Peterson S, Tomson G, et al. Diabetes and pre-diabetes among persons aged 35 to 60 years in eastern Uganda: prevalence and associated factors. PloS One (2013) 8(8):e72554. doi: 10.1371/journal.pone.0072554
15. Mohamed SF, Mwangi M, Mutua MK, Kibachio J, Hussein A, Ndegwa Z, et al. Prevalence and factors associated with pre-diabetes and diabetes mellitus in Kenya: results from a national survey. BMC Public Health (2018) 18(3):1–11. doi: 10.1186/s12889-018-6053-x
16. Vinodhini R, Kebede L, Teka G, Bersisa A, Abel T. Prevalence of prediabetes and its risk factors among the employees of ambo university, oromia region, Ethiopia. Res Mol Med (2017) 5(3):11–20. doi: 10.29252/rmm.5.3.11
17. Pengpid S, Peltzer K. Prevalence and correlates of pre-diabetes and diabetes among a national population-based sample of adults in Zambia: results of the first national STEPS survey in 2017. Int J Diabetes Developing Countries (2021) 41:419–26. doi: 10.1007/s13410-020-00910-8
18. Adekanmbi VT, Uthman OA, Erqou S, Echouffo-Tcheugui JB, Harhay MN, Harhay MO. Epidemiology of prediabetes and diabetes in Namibia, Africa: a multilevel analysis. J Diabetes (2019) 11(2):161–72. doi: 10.1111/1753-0407.12829
19. Sosibo AM, Mzimela NC, Ngubane PS, Khathi A. Prevalence and correlates of pre-diabetes in adults of mixed ethnicities in the south African population: a systematic review and meta-analysis. PloS One (2022) 17(11):e0278347. doi: 10.1371/journal.pone.0278347
20. Nwafor A, Owhoji A. Prevalence of diabetes mellitus among nigerians in port Harcourt correlates with socio-economic status. J Appl Sci Environ Manage (2001) 5(1):75–7. doi: 10.4314/jasem.v5i1.54950
21. Sabir A, Ohwovoriole A, Isezuo S, Fasanmade O, Abubakar S, Iwuala S. Type 2 diabetes mellitus and its risk factors among the rural fulanis of northern Nigeria. Ann Afr Med (2013) 12(4):217. doi: 10.4103/1596-3519.122689
22. Enang O, Otu A, Essien O, Okpara H, Fasanmade O, Ohwovoriole A, et al. Prevalence of dysglycemia in calabar: a cross-sectional observational study among residents of calabar, Nigeria. BMJ Open Diabetes Res Care (2014) 2(1):e000032. doi: 10.1136/bmjdrc-2014-000032
23. Nwatu C, Ofoegbu E, Unachukwu C, Young E, Okafor C, Okoli C. Prevalence of prediabetes and associated risk factors in a rural Nigerian community. Int J Diabetes developing countries (2016) 36:197–203. doi: 10.1007/s13410-015-0401-5
24. Ubani BC, Young E, Ekrikpo UE, Agbonrofo-Eboigbe G, Nga CN, Unachukwu CN. Pre-diabetes and it’s predictors in abia state, Eastern Nigeria. Int J Diabetes Developing Countries (2022) 42:1–8. doi: 10.1007/s13410-021-01007-6
25. Aladeniyi I, Adeniyi OV, Fawole O, Adeolu M, Ter Goon D, Ajayi AI, et al. The prevalence and correlates of pre-diabetes and diabetes mellitus among public category workers in akure, Nigeria. Open Public Health J (2017) 10(1):167–76. doi: 10.2174/1874944501710010167
26. Omech B, Tshikuka J-G, Amone-P’Olak K, Mwita J, Tsima B, Nkomazana O. The utilization of HbA1c test in the screening and diagnosis of type 2 diabetes mellitus: an outpatient clinics survey in Botswana. J Diabetes Endo (2017) 8:1–8. doi: 10.5897/JDE2016.0102
27. Lawal Y, Anumah FE, Bakari AG. Is glycated haemoglobin an alternative to diagnose diabetes mellitus in a northern Nigerian population? Ann Med Health Sci Res (2018) 8(2):98–102.
28. Miranda JJ, Bernabe-Ortiz A, Stanojevic S, Malaga G, Gilman RH, Smeeth L. A1C as a diagnostic criteria for diabetes in low-and middle-income settings: evidence from Peru. PloS One (2011) 6(3):e18069. doi: 10.1371/journal.pone.0018069
29. Unwin N, Howitt C, Rose AM, Samuels TA, Hennis AJ, Hambleton IR. Prevalence and phenotype of diabetes and prediabetes using fasting glucose vs HbA1c in a Caribbean population. J Global Health (2017) 7(2):1–11. doi: 10.7189/jogh.07.020407
30. Murad NAA, Abdullah N, Kamaruddin MA, Abd Jalal N, Ismail N, Yusof NAM, et al. Discordance between fasting plasma glucose (FPG) and HbA1c in diagnosing diabetes and pre-diabetes in the Malaysian cohort. J ASEAN Fed Endocrine Societies (2021) 36(2):127. doi: 10.15605/jafes.036.02.02
31. Vega-Vázquez MA, Ramírez-Vick M, Muñoz-Torres FJ, González-Rodríguez LA, Joshipura K. Comparing glucose and hemoglobin A1c diagnostic tests among a high metabolic risk Hispanic population. Diabetes/metabolism Res Rev (2017) 33(4):e2874. doi: 10.1002/dmrr.2874
32. Booth RA, Jiang Y, Morrison H, Orpana H, Van Katwyk SR, Lemieux C. Ethnic dependent differences in diagnostic accuracy of glycated hemoglobin (HbA1c) in Canadian adults. Diabetes Res Clin practice (2018) 136:143–9. doi: 10.1016/j.diabres.2017.11.035
33. Herman WH, Ma Y, Uwaifo G, Haffner S, Kahn SE, Horton ES, et al. Differences in A1C by race and ethnicity among patients with impaired glucose tolerance in the diabetes prevention program. Diabetes Care (2007) 30(10):2453–7. doi: 10.2337/dc06-2003
34. Christensen DL, Witte DR, Kaduka L, Jørgensen ME, Borch-Johnsen K, Mohan V, et al. Moving to an A1C-based diagnosis of diabetes has a different impact on prevalence in different ethnic groups. Diabetes Care (2010) 33(3):580–2. doi: 10.2337/dc09-1843
35. Puepet F, Ohwovoriole A. Prevalence of risk factors for diabetes mellitus in a non-diabetic population in jos, Nigeria. Nigerian J Med (2008) 17(1):71–4. doi: 10.4314/njm.v17i1.37360
36. Sabir A, Isezuo S, Ohwovoriole A. Dysglycaemia and its risk factors in an urban Fulani population of northern Nigeria. West Afr J Med (2011) 30(5):325–30.
37. Okesina A, Oparinde D, Akindoyin K, Erasmus R. Prevalence of some risk factors of coronary heart disease in a rural Nigerian population. East Afr Med J (1999) 76(4):212–6.
38. Dahiru T, Jibo A, Hassan A, Mande A. Prevalence of diabetes in a semi-urban community in northern Nigeria. Nigerian J Med (2008) 17(4):414–6. doi: 10.4314/njm.v17i4.37423
39. Adeloye D, Ige JO, Aderemi AV, Adeleye N, Amoo EO, Auta A, et al. Estimating the prevalence, hospitalisation and mortality from type 2 diabetes mellitus in Nigeria: a systematic review and meta-analysis. BMJ Open (2017) 7(5):e015424. doi: 10.1136/bmjopen-2016-015424
40. Little RR, Roberts WL. A review of variant hemoglobins interfering with hemoglobin A1c measurement. J Diabetes Sci Technol (2009) 3(3):446–51. doi: 10.1177/193229680900300307
41. Olatunbosun ST, Ojo PO, Fineberg NS, Bella AF. Prevalence of diabetes mellitus and impaired glucose tolerance in a group of urban adults in Nigeria. J Natl Med Assoc (1998) 90(5):293–301.
42. Alebiosu OC, Familoni OB, Ogunsemi OO, Raimi T, Balogun WO, Odusan O, et al. Community based diabetes risk assessment in ogun state, Nigeria (World diabetes foundation project 08-321). Indian J Endocrinol Metab (2013) 17(4):653. doi: 10.4103/2230-8210.113756
43. Unwin N, Shaw J, Zimmet P, Alberti KG. Impaired glucose tolerance and impaired fasting glycaemia: the current status on definition and intervention. Diabetes Med (2002) 19(9):708–23. doi: 10.1046/j.1464-5491.2002.00835.x
44. Genuth S, Alberti KG, Bennett P, Buse J, Defronzo R, Kahn R, et al. Follow-up report on the diagnosis of diabetes mellitus. Diabetes Care (2003) 26(11):3160–7. doi: 10.2337/diacare.26.11.3160
45. Pani LN, Korenda L, Meigs JB, Driver C, Chamany S, Fox CS, et al. Effect of aging on A1C levels in individuals without diabetes: evidence from the framingham offspring study and the national health and nutrition examination survey 2001-2004. Diabetes Care (2008) 31(10):1991–6. doi: 10.2337/dc08-0577
46. Abdul-Ghani MA, Jenkinson CP, Richardson DK, Tripathy D, DeFronzo RA. Insulin secretion and action in subjects with impaired fasting glucose and impaired glucose tolerance: results from the veterans administration genetic epidemiology study. Diabetes (2006) 55(5):1430–5. doi: 10.2337/db05-1200
47. Skinner S, Diaw M, Ndour Mbaye M, Joly P, Renoux C, Masson C, et al. Evaluation of agreement between hemoglobin A1c, fasting glucose, and fructosamine in Senegalese individuals with and without sickle-cell trait. PloS One (2019) 14(2):e0212552. doi: 10.1371/journal.pone.0212552
48. Briker SM, Aduwo JY, Mugeni R, Horlyck-Romanovsky MF, DuBose CW, Mabundo LS, et al. A1C underperforms as a diagnostic test in africans even in the absence of nutritional deficiencies, anemia and hemoglobinopathies: insight from the africans in America study. Front Endocrinol (Lausanne) (2019) 10:533. doi: 10.3389/fendo.2019.00533
49. Guo F, Moellering DR, Garvey WT. Use of HbA1c for diagnoses of diabetes and prediabetes: comparison with diagnoses based on fasting and 2-hr glucose values and effects of gender, race, and age. Metab Syndr Relat Disord (2014) 12(5):258–68. doi: 10.1089/met.2013.0128
50. Ford CN, Leet RW, Kipling L, Rhee MK, Jackson SL, Wilson PW, et al. Racial differences in performance of HbA1c for the classification of diabetes and prediabetes among US adults of non-Hispanic black and white race. Diabetic Med (2019) 36(10):1234–42. doi: 10.1111/dme.13979
51. Sumner AE, Duong MT, Aldana PC, Ricks M, Tulloch-Reid MK, Lozier JN, et al. A1C combined with glycated albumin improves detection of prediabetes in africans: the africans in America study. Diabetes Care (2016) 39(2):271–7. doi: 10.2337/dc15-1699
52. Xu Y, Zhao W, Wang W, Bi Y, Li J, Mi S, et al. Plasma glucose and hemoglobin A1c for the detection of diabetes in Chinese adults. J Diabetes (2016) 8(3):378–86. doi: 10.1111/1753-0407.12305
53. Paddock E, Hohenadel MG, Piaggi P, Vijayakumar P, Hanson RL, Knowler WC, et al. One-hour and two-hour postload plasma glucose concentrations are comparable predictors of type 2 diabetes mellitus in southwestern native americans. Diabetologia (2017) 60(9):1704–11. doi: 10.1007/s00125-017-4332-1
54. Ziemer DC, Kolm P, Weintraub WS, Vaccarino V, Rhee MK, Twombly JG, et al. Glucose-independent, black-white differences in hemoglobin A1c levels: a cross-sectional analysis of 2 studies. Ann Intern Med (2010) 152(12):770–7. doi: 10.7326/0003-4819-152-12-201006150-00004
55. Fasanmade OA, Dagogo-Jack S. Diabetes care in Nigeria. Ann Global Health (2015) 81(6):821–9. doi: 10.1016/j.aogh.2015.12.012
56. Huang YQ, Liu L, Huang JY, Chen CL, Yu YL, Lo K, et al. Prediabetes and risk for all-cause and cardiovascular mortality based on hypertension status. Ann Transl Med (2020) 8(23):1580. doi: 10.21037/atm-20-1155
57. Kenkel DS. Health behavior, health knowledge, and schooling. J Political Economy (1991) 99(2):287–305. doi: 10.1086/261751
58. Ruhm CJ. Health effects of economic crises. Health Economics (2016) 25:6–24. doi: 10.1002/hec.3373
59. Chi JH, Lee BJ. Risk factors for hypertension and diabetes comorbidity in a Korean population: a cross-sectional study. PloS One (2022) 17(1):e0262757. doi: 10.1371/journal.pone.0262757
60. Yang B, Li J, Haller MJ, Schatz DA, Rong L. Modeling the progression of type 2 diabetes with underlying obesity. PloS Comput Biol (2023) 19(2):e1010914. doi: 10.1371/journal.pcbi.1010914
61. Bergman M, Abdul-Ghani M, DeFronzo RA, Manco M, Sesti G, Fiorentino TV, et al. Review of methods for detecting glycemic disorders. Diabetes Res Clin practice (2020) 165:108233. doi: 10.1016/j.diabres.2020.108233
62. Nwatu CB, Young EE. Prediabetes in Sub−Saharan Africa: pathophysiology, predictors, and prevalence. Nigerian J Med (2020) 29(3):343–50.
63. Paprott R, Scheidt-Nave C, Heidemann C. Determinants of change in glycemic status in individuals with prediabetes: results from a nationwide cohort study in Germany. J Diabetes Res (2018) 2018:5703652. doi: 10.1155/2018/5703652
64. Ferrannini E. The stunned beta cell: a brief history. Cell Metab (2010) 11(5):349–52. doi: 10.1016/j.cmet.2010.04.009
65. Fonseca VA. Defining and characterizing the progression of type 2 diabetes. Diabetes Care (2009) 32 Suppl 2(Suppl 2):S151–6. doi: 10.2337/dc09-S301
66. Weyer C, Bogardus C, Mott DM, Pratley RE. The natural history of insulin secretory dysfunction and insulin resistance in the pathogenesis of type 2 diabetes mellitus. J Clin Invest (1999) 104(6):787–94. doi: 10.1172/JCI7231
67. Weyer C, Tataranni PA, Bogardus C, Pratley RE. Insulin resistance and insulin secretory dysfunction are independent predictors of worsening of glucose tolerance during each stage of type 2 diabetes development. Diabetes Care (2001) 24(1):89–94. doi: 10.2337/diacare.24.1.89
68. Shen X, He S, Wang J, Qian X, Wang H, Zhang B, et al. Modifiable predictors of type 2 diabetes mellitus and roles of insulin resistance and β-cell function over a 6-year study and 30-year follow-up. J Endocrinol Invest (2023) 46(5):883–91. doi: 10.1007/s40618-022-01932-1
69. Tucker LA. Limited agreement between classifications of diabetes and prediabetes resulting from the OGTT, hemoglobin A1c, and fasting glucose tests in 7412 U.S. Adults J Clin Med (2020) 9(7):1–18. doi: 10.3390/jcm9072207
70. Norberg M, Stenlund H, Lindahl B, Andersson C, Eriksson JW, Weinehall L. Work stress and low emotional support is associated with increased risk of future type 2 diabetes in women. Diabetes Res Clin Pract (2007) 76(3):368–77. doi: 10.1016/j.diabres.2006.09.002
71. Kroenke CH, Spiegelman D, Manson J, Schernhammer ES, Colditz GA, Kawachi I. Work characteristics and incidence of type 2 diabetes in women. Am J Epidemiol (2007) 165(2):175–83. doi: 10.1093/aje/kwj355
72. Wändell PE, Carlsson AC. Gender differences and time trends in incidence and prevalence of type 2 diabetes in Sweden–a model explaining the diabetes epidemic worldwide today? Diabetes Res Clin Pract (2014) 106(3):e90–2. doi: 10.1016/j.diabres.2014.09.013
73. Wells JC, Pomeroy E, Walimbe SR, Popkin BM, Yajnik CS. The elevated susceptibility to diabetes in India: an evolutionary perspective. Front Public Health (2016) 4:145. doi: 10.3389/fpubh.2016.00145
74. Wales JK. Does psychological stress cause diabetes? Diabetes Med (1995) 12(2):109–12. doi: 10.1111/j.1464-5491.1995.tb00439.x
Keywords: pre-diabetes, undiagnosed diabetes, prevalence, adult Nigerians, selected states, haemoglobin A1c
Citation: Ajayi IO, Balogun WO, Olopade OB, Ajani GO, Soyoye DO, Bolarinwa OA, Olamoyegun MA, Alatishe-Muhammad BW, Odeniyi IA, Odukoya O, Fasanmade OA, Diyaolu FP, Otrofanowei E, Akase I, Agabi PO, Adejimi A, Ajetunmobi OA, Durowade KA, Gabriel-Alayode EO, Ibrahim AO, Ezekpo OO, Elegbede TO, Lamidi AO, Owolabi FA, Yusuf AO, Adetunji TA, Ogunmodede AJ, Ameen AH, Biliaminu AS and Nasiru S (2023) Prevalence of haemoglobin A1c based dysglycaemia among adult community dwellers in selected states in Nigeria: a descriptive cross-sectional study. Front. Endocrinol. 14:1192491. doi: 10.3389/fendo.2023.1192491
Received: 23 March 2023; Accepted: 14 June 2023;
Published: 20 July 2023.
Edited by:
Bo Zhu, Boston Children’s Hospital and Harvard Medical School, United StatesReviewed by:
Rosana De Morais Borges Marques, Universidade Federal de Goiás, BrazilCopyright © 2023 Ajayi, Balogun, Olopade, Ajani, Soyoye, Bolarinwa, Olamoyegun, Alatishe-Muhammad, Odeniyi, Odukoya, Fasanmade, Diyaolu, Otrofanowei, Akase, Agabi, Adejimi, Ajetunmobi, Durowade, Gabriel-Alayode, Ibrahim, Ezekpo, Elegbede, Lamidi, Owolabi, Yusuf, Adetunji, Ogunmodede, Ameen, Biliaminu and Nasiru. This is an open-access article distributed under the terms of the Creative Commons Attribution License (CC BY). The use, distribution or reproduction in other forums is permitted, provided the original author(s) and the copyright owner(s) are credited and that the original publication in this journal is cited, in accordance with accepted academic practice. No use, distribution or reproduction is permitted which does not comply with these terms.
*Correspondence: William O. Balogun, YmFsb2dlZUB5YWhvby5jb20=
Disclaimer: All claims expressed in this article are solely those of the authors and do not necessarily represent those of their affiliated organizations, or those of the publisher, the editors and the reviewers. Any product that may be evaluated in this article or claim that may be made by its manufacturer is not guaranteed or endorsed by the publisher.
Research integrity at Frontiers
Learn more about the work of our research integrity team to safeguard the quality of each article we publish.