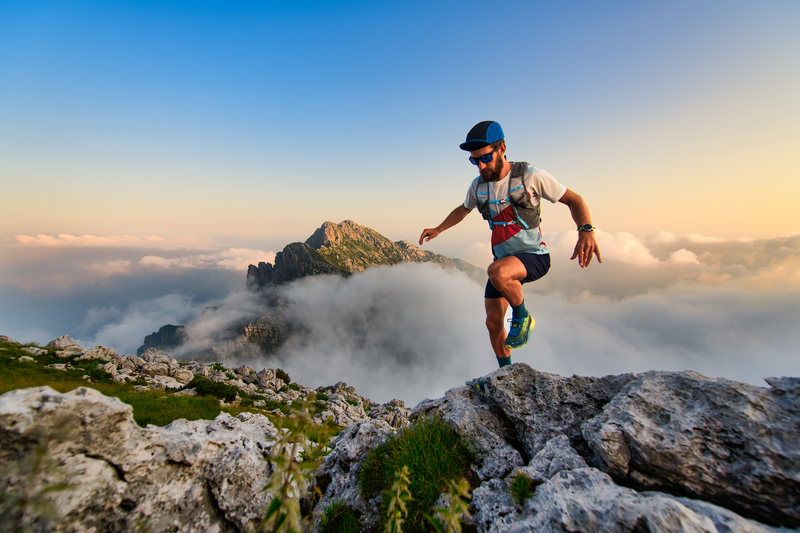
94% of researchers rate our articles as excellent or good
Learn more about the work of our research integrity team to safeguard the quality of each article we publish.
Find out more
EDITORIAL article
Front. Endocrinol. , 14 April 2023
Sec. Thyroid Endocrinology
Volume 14 - 2023 | https://doi.org/10.3389/fendo.2023.1188250
This article is part of the Research Topic Advances of Imaging Techniques in Identifying Malignancy in Thyroid Nodules View all 10 articles
Editorial on the Research Topic
Advances of imaging techniques in identifying malignancy in thyroid nodules
The management of thyroid nodules (TNs) has gained attention due to its increasingly high incidence rate (1). It was estimated that over 586,000 people were diagnosed with TNs in 2020 (2). TNs have been identified more frequently in recent years due to increased sensitivity in imaging techniques (3). TNs patients are diagnosed by history taking and physical examination, ultrasonography, Thyroid -stimulating hormone (TSH) serum measurement, fine needle aspiration, and scintigraphy (4). Most TNs proved benign; however, 7 to 15% of TNs are malignant, causing mortality if not treated properly (5, 6). Hence, diagnostic tools are most necessary to differentiate benign and malignant TNs to provide optimal treatment.
We invited researchers worldwide to address advanced imaging techniques to differentiate TNs and innovations in thyroid imaging approaches for therapy. We received diverse and insightful nine manuscripts involving 68 authors from various backgrounds. The Research Topic was divided into three areas of research: 1) Artificial intelligence prediction models compared to real-world evidence; 2) Advanced ultrasonography from grey-scale to contrast-enhanced ultrasonography; and 3) Ultrasonography (US) characterization for TNs and lymph node metastasis. This research aimed to assess the malignancy risk, identify poor prognostic factors, and determine the optimal management plan for patients.
The US is an operator-dependent technique, and the imaging results’ interpretation is affected by the radiologists’ experience (5). To reduce a variation of US interpretations between operators, recent studies have investigated the role of a deep-learning prediction model to assess thyroid nodule malignancy risk and metastatic lymph nodes. Zhang et al. invented a deep learning prediction model for diagnosing papillary thyroid carcinoma (PTC) malignancy that incorporated many variables – demographic, serological, ultrasound, and biopsy data of 2,029 patients. The study reported an excellent predictive ability to differentiate between benign and malignant PTC. Furthermore, Chang et al. developed a deep learning model integrated with clinical and ultrasound factors to predict central lymph node metastasis (CLNM) in PTC by using the dataset of 3,359 CLNM patients, and the study may have superior clinical predictive tools compared to other deep learning-based models.
Even though the deep learning prediction model has promising predictive capabilities, the US remains the conservative diagnostic modality of TNs malignancy, especially in areas with limited healthcare facilities (5). Feng et al. explored the effectiveness of ultrasound grayscale ratio (UGSR) to differentiate benign and malignant TNs in patients with Hashimoto’s thyroiditis (HT). The UGSR showed an excellent diagnostic result to determine TNs malignancy in HT patients. Wang et al. studied the US to detect partially cystic thyroid nodules (PCTNs). The study described US characteristic features for malignant and benign PCTNs with reliable diagnostic ability. Chen et al. examined the role of contrast-enhanced ultrasonography (CEUS) to differentiate mummified TNs from other malignant TNs. However, a large-scale population with a multicenter prospective study is needed to verify the specificity and sensitivity of the CEUS findings.
The US features a high prediction tool for malignant TNs (1). Thyroid Imaging Reporting and Data System (TIRADS) provides US prediction tools for TNs malignancy – the most popular are ACR-TIRADS, EU-TIRADS, and K-TIRADS. A study by Chen et al. compared malignancy risk stratification of TNs for C-TIRADS, K-TIRADS, and ACR-TIRADS. Results showed that C-TIRADS has outstanding performance in the malignancy risk stratifications by optimized cut-off value compared with other TIRADS. Another interesting study was provided by Zhou et al. and Yao et al., where both studies support each other. Zhou et al. evaluated the sonographic characteristics and risk factors of CLNM in 2,376 patients. Although the US reported excellent sensitivity and specificity, Yao et al. suggested that a combination of US and CT-Scan showed better results in diagnosing CLNM rather than the US alone. Moreover, Liu et al. determined the optimal imaging time after Iodine -131 treatment; imaging sensitivity on day three detected residual thyroid tissue and on day seven and day ten detected cervical lymph nodes and lung metastases.
These findings highlight how advanced imaging techniques provide a more expansive view of TNs diagnosis. Although the artificial intelligence prediction model had better accuracy than the conventional ones, it may be limited to developing countries. We encourage more accessibility with exact accuracy tools such as a web-based deep learning model, which will be clinically applicable in iodine-deficit regions – mainly in developing countries. Nevertheless, we are confident that all selected studies on this Research Topic bring essential and enduring contributions to differentiate thyroid nodule malignancy.
HZ: conceptualizing, writing the original draft, writing–review, and editing. EB: writing–review, and editing. AE: writing–review, and editing. All authors approved the submitted version.
We would like to express our gratitude to all the authors who proposed their work and to all the researchers who reviewed the submission to this Research Topic.
The authors declare that the research was conducted in the absence of any commercial or financial relationships that could be construed as a potential conflict of interest.
All claims expressed in this article are solely those of the authors and do not necessarily represent those of their affiliated organizations, or those of the publisher, the editors and the reviewers. Any product that may be evaluated in this article, or claim that may be made by its manufacturer, is not guaranteed or endorsed by the publisher.
1. Ha EJ, Chung SR, Na DG, Ahn HS, Chung J, Lee JY, et al. Korean Thyroid imaging reporting and data system and imaging-based management of thyroid nodules: Korean society of thyroid radiology consensus statement and recommendations. Korean J Radiol (2021) 22(12):2094. doi: 10.3348/kjr.2021.0713
2. Siegel RL, Miller KD, Fuchs HE, Jemal A. Cancer statistics. CA Cancer J Clin (2022) 72(1):7–33. doi: 10.3322/caac.21708
3. Mu C, Ming X, Tian Y, Liu Y, Yao M, Ni Y, et al. Mapping global epidemiology of thyroid nodules among general population: a systematic review and meta-analysis. Front Oncol (2022) 12. doi: 10.3389/fonc.2022.1029926
4. Tamhane S, Gharib H. Thyroid nodule update on diagnosis and management. Clin Diabetes Endocrinol (2016) 2(1):17. doi: 10.1186/s40842-016-0035-7
5. Lee JY, Baek JH, Ha EJ, Sung JY, Shin JH, Kim Jh, et al. Imaging guidelines for thyroid nodules and differentiated thyroid cancer: Korean society of thyroid radiology. Korean J Radiol (2020) 22(5):840. doi: 10.3348/kjr.2020.0578
Keywords: thyroid nodules, artificial intelligence, deep learning, advanced thyroid imaging, real-world evidence, TIRADS
Citation: Zufry H, Barrenechea EA and Elliyanti A (2023) Editorial: Advances of imaging techniques in identifying malignancy in thyroid nodules. Front. Endocrinol. 14:1188250. doi: 10.3389/fendo.2023.1188250
Received: 17 March 2023; Accepted: 03 April 2023;
Published: 14 April 2023.
Edited and Reviewed by:
Terry Francis Davies, Icahn School of Medicine at Mount Sinai, United StatesCopyright © 2023 Zufry, Barrenechea and Elliyanti. This is an open-access article distributed under the terms of the Creative Commons Attribution License (CC BY). The use, distribution or reproduction in other forums is permitted, provided the original author(s) and the copyright owner(s) are credited and that the original publication in this journal is cited, in accordance with accepted academic practice. No use, distribution or reproduction is permitted which does not comply with these terms.
*Correspondence: Hendra Zufry, aGVuZHJhX3p1ZnJ5QHVuc3lpYWguYWMuaWQ=
Disclaimer: All claims expressed in this article are solely those of the authors and do not necessarily represent those of their affiliated organizations, or those of the publisher, the editors and the reviewers. Any product that may be evaluated in this article or claim that may be made by its manufacturer is not guaranteed or endorsed by the publisher.
Research integrity at Frontiers
Learn more about the work of our research integrity team to safeguard the quality of each article we publish.