- 1The First Clinical Medical College of Guangzhou University of Chinese Medicine, Guangzhou, China
- 2The First Affiliated Hospital of Guangzhou University of Chinese Medicine, Guangzhou, China
- 3Lingnan Medical Research Center of Guangzhou University of Chinese Medicine, Guangzhou, China
Background: Coronavirus disease 2019 (COVID‐19) is a highly contagious respiratory disease that has posed a serious threat to people’s daily lives and caused an unprecedented challenge to public health and people’s health worldwide. Lung squamous cell carcinoma (LUSC) is a common type of lung malignancy with a highly aggressive nature and poor prognosis. Patients with LUSC could be at risk for COVID-19, We conducted this study to examine the potential for naringenin to develop into an ideal medicine and investigate the underlying action mechanisms of naringenin in COVID-19 and LUSC due to the anti-viral, anti-tumor, and anti-inflammatory activities of naringenin.
Methods: LUSC related genes were obtained from TCGA, PharmGKB, TTD,GeneCards and NCBI, and then the transcriptome data for COVID-19 was downloaded from GEO, DisGeNET, CTD, DrugBank, PubChem, TTD, NCBI Gene, OMIM. The drug targets of Naringenin were revealed through CTD, BATMAN, TCMIP, SymMap, Chemical Association Networks, SwissTargetPrediction, PharmMapper, ECTM, and DGIdb. The genes related to susceptibility to COVID-19 in LUSC patients were obtained through differential analysis. The interaction of COVID-19/LUSC related genes was evaluated and demonstrated using STRING to develop a a COX risk regression model to screen and evaluate the association of genes with clinical characteristics. To investigate the related functional and pathway analysis of the common targets of COVID-19/LUSC and Naringenin, KEGG and GO enrichment analysis were employed to perform the functional analysis of the target genes. Finally, The Hub Gene was screened and visualized using Cytoscape, and molecular docking between the drug and the target was performed using Autodock.
Results: We discovered numerous COVID-19/LUSC target genes and examined their prognostic value in LUSC patients utilizing a variety of bioinformatics and network pharmacology methods. Furthermore, a risk score model with strong predictive performance was developed based on these target genes to assess the prognosis of LUSC patients with COVID-19. We intersected the therapeutic target genes of naringenin with the LUSC, COVID-19-related targets, and identified 354 common targets, which could be used as potential target genes for naringenin to treat COVID-19/LUSC. The treatment of COVID-19/LUSC with naringenin may involve oxidative stress, anti-inflammatory, antiviral, antiviral, apoptosis, immunological, and multiple pathways containing PI3K-Akt, HIF-1, and VEGF, according to the results of the GO and KEGG enrichment analysis of these 354 common targets. By constructing a PPI network, we ascertained AKT1, TP53, SRC, MAPK1, MAPK3, and HSP90AA1 as possible hub targets of naringenin for the treatment of COVID-19/LUSC. Last but not least, molecular docking investigations showed that naringenin has strong binding activity in COVID-19/LUSC.
Conclusion: We revealed for the first time the pharmacological targets and potential molecular processes of naringenin for the treatment of COVID-19/LUSC. However, these results need to be confirmed by additional research and validation in real LUSC patients with COVID-19.
1 Introduction
An acute respiratory infectious illness called COVID-19 is brought on by the SARS-CoV-2 virus (1). Coughing, a sore throat, fever, arthralgias, myalgias, exhaustion, and headache are among the usual COVID-19 symptoms. Acute respiratory distress syndrome (2, 3), shock (4, 5), metabolic acidosis (6), and multiple organ failure (7, 8) may develop in patients with comorbidities. As of November 28, 2022, with 636,440,663 confirmed COVID-19 cases and 6,606,624 deaths reported globally (9). Despite the development of many COVID-19 vaccines and the initiation of mass vaccinations, the number of infections is still continuously increasing (10). Additionally, several antiviral medications have been used to treat COVID-19, such as remdesivir, but they have not been generally adopted because of their high cost and requirement for intravenous administration (11). Studies have demonstrated that cancer patients, including those with lung cancer (12), esophageal cancer (13), colorectal cancer (14), and breast cancer (15), among others, are more susceptible to SARS-CoV-2 infection and have a higher fatality rate (16, 17). Therefore, it is crucial to screen beneficial, affordable, and widely accessible drugs against COVID-19. Lung cancer is one of the most common malignant tumors in humans and has the highest mortality rate worldwide, with 1.6 million fatalities per year (18, 19). The hospital served as the primary infection source during the early stages of the outbreak, and patients with lung cancer who were admitted there for antitumor therapy significantly increased their risk of COVID-19 (20). The majority of patients with lung cancer are immunosuppressed (21), and there is a pressing need for effective drugs to treat lung cancer and COVID-19 with few side effects.
Naringenin is a common dietary flavanone found in citrus fruits such as oranges, bergamots, lemons, and grapefrui (22). Naringenin has a molecular formula C15H12O5 and is chemically named 2,3-dihydro-5,7-dihydroxy-2-(4-hydroxyphenyl)4H-1-benzopyran-4-one (23). Pharmacologically, it has anticancer, antimutagenic, antioxidant, antiproliferative, and antiatherogenic activities (24). Naringenin is Commonly used for the treatments of diabetic (25), cognition deficits (26), bronchial pneumonia (27), nonalcoholic steatohepatitis (28, 29), and neurodegenerative diseases (30–32). Naringin possesses antiviral and anti-inflammatory properties, which include lowering viral replication and cytokine production, according to recent studies (33). By entering human cells through the angiotensin-converting enzyme 2(ACE2) receptor and Transmembrane Serine Protease 2(TMPRSS2) (34), SARS-CoV-2 can cause infection. When a virus infects a host, it causes the host to produce and release more inflammatory cytokines, which can boost immune activity and cause tissue damage (35). A growing body of research suggests that antiviral therapy may help treat COVID-19 symptoms as well as those that reduce inflammatory responses (36, 37). Through both transcriptional and post-transcriptional processes, naringenin can reduce the generation of inflammatory molecules (38). Macrophages have a crucial role in the pathogenesis of COVID-19 because they can detect infections, react to them, and create inflammatory cytokines and chemokines (39). Without affecting the toll-like receptor(TLR) cascade, naringenin decreased the generation of TNF and IL-6 by macrophages and T cells in animal experimental models (39). A recent study suggests that Naringenin can function as having the potential inhibitor of SARS‐CoV‐2 main protease, naringenin may be considered as potential for preventing CoV replication (40, 41). In addition, Naringenin exhibits the role of treatment of lung cancer by reducing tumor cell proliferation, migration, and invasion while increasing apoptosis (42, 43). As far as we can tell, naringin’s molecular mechanisms and targeting have not been investigated in the treatment of COVID-19 in patients with LUSC. In this study, we examine the prognostic value of COVID-19-related genes in LUSC patients and further explore the potential anti-COVID-19/LUSC mechanisms of naringenin using network pharmacology and bioinformatics methods. Our findings offer some fresh perspectives on how naringenin works to treat COVID-19/LUSC. A clear graphical summary that detailed the entire study process was used in Figure 1.
2 Materials and methods
2.1 Ethics statement
Because the data were sourced from free databases, ethics committee approval was not necessary for this study.
2.2 Naringenin database building
PubChem (https://pubchem.ncbi.nlm.nih.gov/) comprises a wide range of chemical information from 750 data sources (44). The 2D structure, 3D structure, InChI, and canonical SMILES profiles of naringin were obtained from PubChem (44).
2.3 Identification of COVID-19/LUSC-associated genes
The transcriptome profiles of LUSC patients were downloaded from The Cancer Genome Atlas (TCGA) database (https://portal.gdc.cancer.gov/) on 19 November 2022, and the differentially expressed genes (DEGs) were screened and obtained using the ‘limma’ package of R-language(version 4.2.2)Bioconductor with P-value< 0.05 and |log2FC| >1 (45). DEGs were represented using volcano plots created with the R-language packages “ggpubr” and “ggthemes”. PharmGKB (https://www.pharmgkb.org), NCBI Gene (https://www.ncbi.nlm.nih.gov/) (46), Therapeutic Target Database (TTD, http://db.idrblab.net/), GeneCards (https://www.genecards.org/), and Online Mendelian Inheritance in Man (OMIM, https://www.omim.org/) (47) were also used to collect LUSC related targets.
The COVID-19-related targets were identified by examining the transcriptome RNA-seq data of COVID-19 (GSE171110 and GSE179850) from the Gene Expression Omnibus database (GEO, https://www.ncbi.nlm.nih.gov/geo) (48). After normalization and removal of the batch effect by using the “sva” package, the difference analysis was conducted with the criteria of adjusted P-value< 0.05 and |log2FC| > 1 by using the “limma” package (45). Furthermore, targets associated with COVID-19 were obtained by searching the following eight databases: 1) DisGeNET(http://www.disgenet.org/), 2) Comparative Toxicogenomics Database(CTD, http://ctdbase.org/), 3) DrugBank(https://go.drugbank.com/), 4) PubChem(https://pubchem.ncbi.nlm.nih.gov/), 5)Therapeutic Target Database, 6) GeneCards, 7) NCBI Gene, 8)Online Mendelian Inheritance in Man. Targets gathered from public databases and GEO datasets were combined. The targets of LUSC and COVID-19 were then intersected to create a gene set that is connected to COVID-19/LUSC.
2.4 Fishing of naringenin-related targets
From the following databases, many pharmacological targets connected to naringenin were gathered: 1) CTD (http://ctdbase.org/) (49), 2) Bioinformatics Analysis Tool for Molecular mechANism of Traditional Chinese Medicine(BATMAN, http://bionet.ncpsb.org.cn/batman-tcm/) (50), 3) Integrative Pharmacology-based Research Platform of Traditional Chinese Medicine (TCMIP, http://www.tcmip.cn/TCMIP/) (51), 4) Symptom Mapping (SymMap, https://www.Symmap.org/) (52), 5) Swiss Target Prediction (http://www.swisstargetprediction.ch/) (53), 6) Chemical Association Networks (STITCH, http://stitch.embl.de/) (53), 7) PharmMapper (http://www.lilabecust.cn/pharmmapper/) (54), 8) Encyclopedia of Traditional Chinese Medicine (ECTM, http://www.tcmip.cn/ETCM/) (51), and 9) Drug Gene Interaction Database (DGIdb, https://www.dgidb.org/) (55). The target genes were transformed to standard gene symbols by using the UniProt database (https://www.uniprot.org/) with the limitation of “Human species”. After deleting the duplicate data, there were 568 targets of naringenin.
2.5 Acquisition of naringenin targets in COVID-19/LUSC
Using the Venn diagram tool (http://bioinformatics.psb.ugent.be/webtools/Venn/) and Microsoft Excel, it was possible to further achieve the goal of removing the repeated targets among naringenin, LUSC, and COVID-19. Then, the intersection of naringenin-related targets and COVID-19/LUSC-related targets was then screened for common targets.
2.6 Construction of LUSC/COVID-19-related prognostic signature for lung squamous cell carcinoma patients
Univariate Cox analysis was performed on 354 common targets, and Lasso Cox regression was performed on significantly expressed genes based on a 1000 ten-fold cross-validation to identify COVID-19-related genes. Optimal prognostic genes were identified based on multivariate Cox regression analysis (P< 0.05), and the best model parameters were used for signature construction, followed by the calculation of risk scores.
2.7 Analysis of prognosis signature
Patients were divided into high- and low-risk groups based on median values of a risk score to determine the prognosis of the signature. We used the survival package to calculate overall survival (OS) for patients with LUSC in different groups and performed univariate and multivariate independent prognostic analyses to evaluate the independent prognostic value of the risk prediction signature. The pheatmap package was used to plot patient survival status and gene expression heatmap based on the risk scores. The survival ROC package was used to calculate the 1-, 3-, and 5-year area under the receiver operating characteristic curve(ROC) curve (AUC) of signature in the LUSC patients.
2.8 Construction of nomogram and validation of clinical subgroups
Nomograms were constructed for age, gender, T stage, N stage, M stage, and risk score using the survival and rms packages. Calibration curves were plotted to show the difference between the predicted and actual outcomes of the nomogram. Decision curve analyses are used to verify the accuracy of the signature in predicting the survival of patients with LUSC.
2.9 Principal component analysis
PCA analysis was performed using limma and scatterplot3d packages to explore the distribution of patients with the high and low-risk groups.
2.10 Analyses of the protein-protein interaction network and hub targets
PPI networks contribute to a better understanding of target-related pathogenesis at the protein level. Thus, the STRING 11.5b database (https://string-db.org/) was used to fabricate the PPI network and acquire hub targets. The organism was selected as “Homo sapiens” and the minimum required interaction score with a correlation degree ≥0.900 was the cut-off value (56). Subsequently, the PPI network was visualized and analyzed by Cytoscape 3.9.1 software (https://cytoscape.org/). The degree values in the PPI network were calculated by using the NetworkAnalyzer CytoNCA of Cytoscape 3.9.1 software. Then, targets with degree values higher than the median were filtered as hub targets (57).
2.11 Enrichment analyses for common targets
To investigate the related functional and pathway analysis of the common targets of COVID-19/LUSC and Naringenin, R packages such as “enrichplot”, “clusterProfiler” (58), “org.Hs.eg.db”, and “ggplot2” were used to perform GO functional analysis and KEGG pathway enrichment analysis (59).Biological processes (BP), cellular components (CC), and molecular functions(MF) were the three categories included in the GO analysis. For enrichment, q-value cutoff = 0.05 and p-value cutoff = 0.05 were set, and the output was utilized to construct the bubble chart (46, 60).
2.12 Molecular docking
The binding situation and interaction force of proteins and small molecules may be anticipated and acquired via molecular docking analysis. Naringenin was docked with the PPI network’s top six hub targets, which included RAC-alpha serine/threonine-protein kinase(AKT1), TP53-target gene 3 protein(TP53), Proto-oncogene tyrosine-protein kinase Src(SRC), Heat shock protein HSP 90-alpha(HSP90AA1), Mitogen-activated protein kinase 3(MAPK3), and Mitogen-activated protein kinase 1(MAPK1). Naringenin’s two-dimensional molecular structure was retrieved from the PubChem database (https://pubchem.ncbi.nlm.nih.gov/) (61), and its three-dimensional structure was created and optimized using the MM2 force field in ChemBioOffice software (version 2019) (62). Following that, Naringenin’s output ligand file was saved in mol2 format. The protein structures of the hub targets were obtained from the PDB database (https://www.rcsb.org/) (63). All water molecules and original ligands were removed from the structures using PyMOL software (https://pymol.org/2/) and saved as PDB files. AutoDockTools (Vina 1.5.6, http://autodock.scripps.edu/) was used to convert the ligand files and original protein receptor to PDBQT file format that be identified by the Autodock Vina software for further molecular docking. Lastly, PyMOL software was used to analyze and present all docking results (64).
3 Results
3.1 Targets identification of naringenin and COVID-19/LUSC
All targets of naringenin were obtained from Nine open-source databases, namely, BATMAN (1), CTD (326), DGIdb (6), ETCM (44), PharmMapper (299), STITCH (13), Swiss (65), SymMap (65), and TCMIP (44). And 568 targets related to naringenin were acquired after eliminating duplicate targets (Figure 2A). Subsequently, a total of 10649 DEGs (4042 upregulated and 6607 downregulated) of LUSC were identified from the TCGA. DEGs volcano plots for LUSC are displayed in Figure 3A. In addition, LUSC related genes collected from PharmGKB, NCBI Gene, TTD, GeneCards, and OMIM were 83, 2424, 7, 5062, and 438, respectively. By merging DEGs from the TCGA and LUSC-related genes from online platforms and removing duplication, we obtained 22232 LUSC related targets (Figure 2B). In total, 4533 DEGs (4561 upregulated and 17 downregulated) of COVID-19 were identified from the GEO. DEGs volcano plots for COVID-19 are displayed in Figure 3B. In addition, COVID-19 related genes retrieved from DisGeNET, CTD, DrugBank, PubChem, TTD, GeneCards, NCBI Gene, and OMIM were 1632, 9892, 40, 622, 73, 1972, 412, and 3, respectively. By merging DEGs from the GEO and LUSC-related genes from online platforms and removing duplication, we obtained 13422 COVID-19 related targets (Figure 2C). Finally, we acquired 354 common targets between naringenin, LUSC, and COVID-19, which were shown using the Venn diagram tool (Figure 2D).
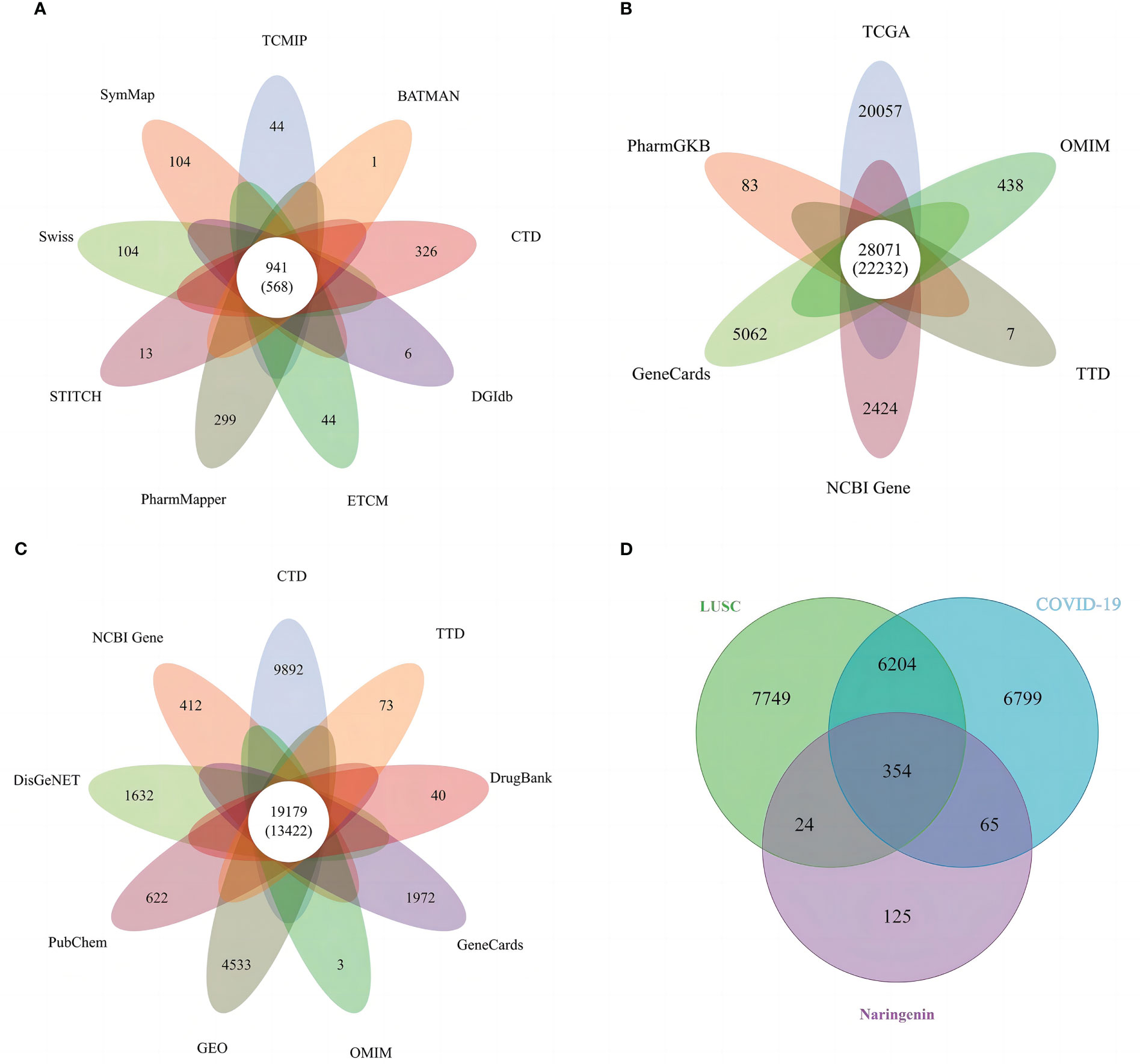
Figure 2 (A) Targets connected to naringenin in nine open-source databases. (B) Targets connected to LUSC in six open-source databases. (C) Targets connected to COVID-19 in nine open-source databases. (D) A Venn diagram demonstrating the common targets of naringenin, LUSC, and COVID-19.
3.2 Construction of LUSC/COVID-19-related prognostic signature
Cox regression analysis of 354 common target expression data and survival information was conducted using the coxph method in the “survival package” of the R language. The filtering standard was P<0.05, and 37 single-factor significant genes were obtained. Lasso regression analysis was performed on the above single-factor significant gene expression data, and Lasso regression plots and cross-validation plots were drawn (Figures 4A, B), and there were 20 Lasso regression significant genes based on the λ minimum value of LASSO Cox regression (0.03033484), Finally, 13 prognosis-related genes for constructing the model were identified using multifactorial Cox regression analysis, and forest plots were drawn (Figure 4C). Those involved in the model construction (BAD, CYP1A1, ESRRA, LRRC27, MMP9, NOS1, PDE5A, and SREBF1) with risk coefficients greater than 1 were defined as risk factors in LUSC, and their higher expression correlated with the worst OS of LUSC.
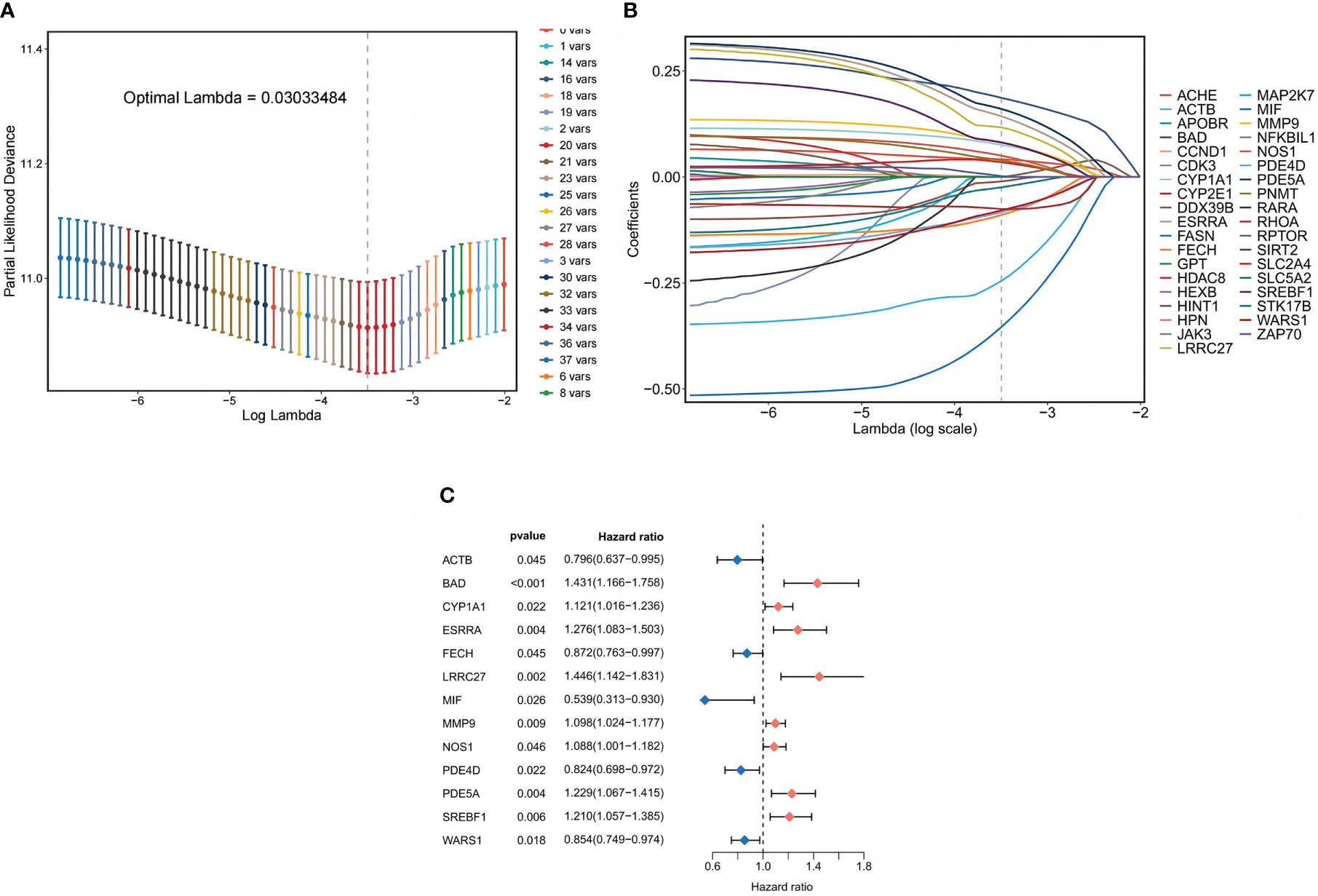
Figure 4 Prognostic model of LUSC patients. (A) Coefficient profiles plotted for LASSO regression analysis of LUSC. (B) Cross-validation error rate plotted for LASSO regression analysis of LUSC. (C) Partial presentation of prognostic related genes.
3.3 Analysis of prognosis signature
The patients were separated into high- and low-risk groups in order to better examine the prognostic value of the risk signature. The 13 genes in the constructed model were analyzed differentially for each clinical trait and high and low-risk groups, and heat maps were plotted (Figure 5A), demonstrating that (BAD, CYP1A1, ESRRA, LRRC27, MMP9, NOS1, PDE5A, and SREBF1) were highly expressed in the high-risk group. We discovered that patients in the high-risk group had a considerably shorter overall survival than those in the low-risk group in the Kaplan-Meier survival analysis. (Figure 5B). The risk curves show the relationship between LUSC patients’ risk scores and survival rates, and we discovered that mortality was higher in high-risk patients than in low-risk individuals. The heatmap showed high- and low-risk levels for 13 genes. For example, 8 genes (BAD, CYP1A1, ESRRA, LRRC27, MMP9, NOS1, PDE5A, and SREBF1) were highly expressed in the high-risk group, which was consistent with the prediction of the model (Figures 5C, D).
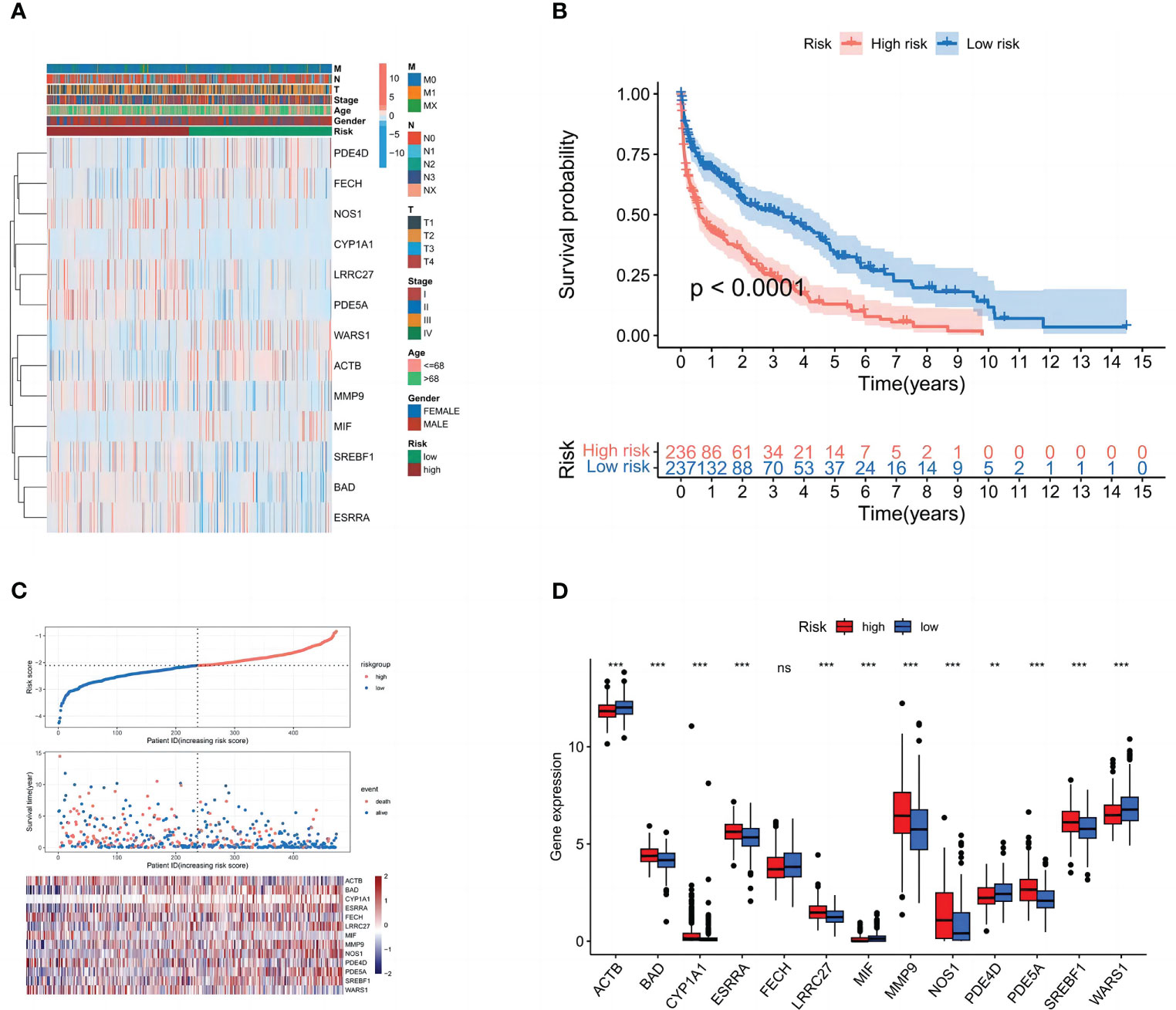
Figure 5 Subgroup correlation heat map and survival analysis of high- and low-risk groups. (A) Patient clinicopathological characteristics are distributed in different ways. (B) Box plot of model gene differential analysis: comparison of model genes differentially expressed in high- and low-risk groups.(C) Patient’s OS according to Kaplan-Meier curves for high- and low-risk groups. (D) The variation in immune checkpoint gene expression between various populations. ns, P=>0.05: **, P<0.01; ***, P<0.001.
3.4 Independent analysis of prognostic factors
Univariate and multivariate Cox regression analyses were performed to determine whether the risk signature has the potential to be a prognostic factor independent of other clinical parameters (Figures 6A, B). The risk score (HR = 2,805, 2.237–3.518; P<0.001) was significantly linked with OS in multivariate Cox regression, demonstrating that the risk signature is an independent prognostic factor for LUSC patients. Additionally, we evaluated the risk score’s predictive accuracy using ROC curves. The AUC for the risk score was 0.75, which was higher than those for age (0.55), gender (0.53), and stage (0.58). While in the LUSC cohort, the AUCs for 1-, 3-, and 5-year OS were 0.701, 0.747, and 0.757 (Figure 6C), indicating that the signature has trustworthy diagnostic applicability.
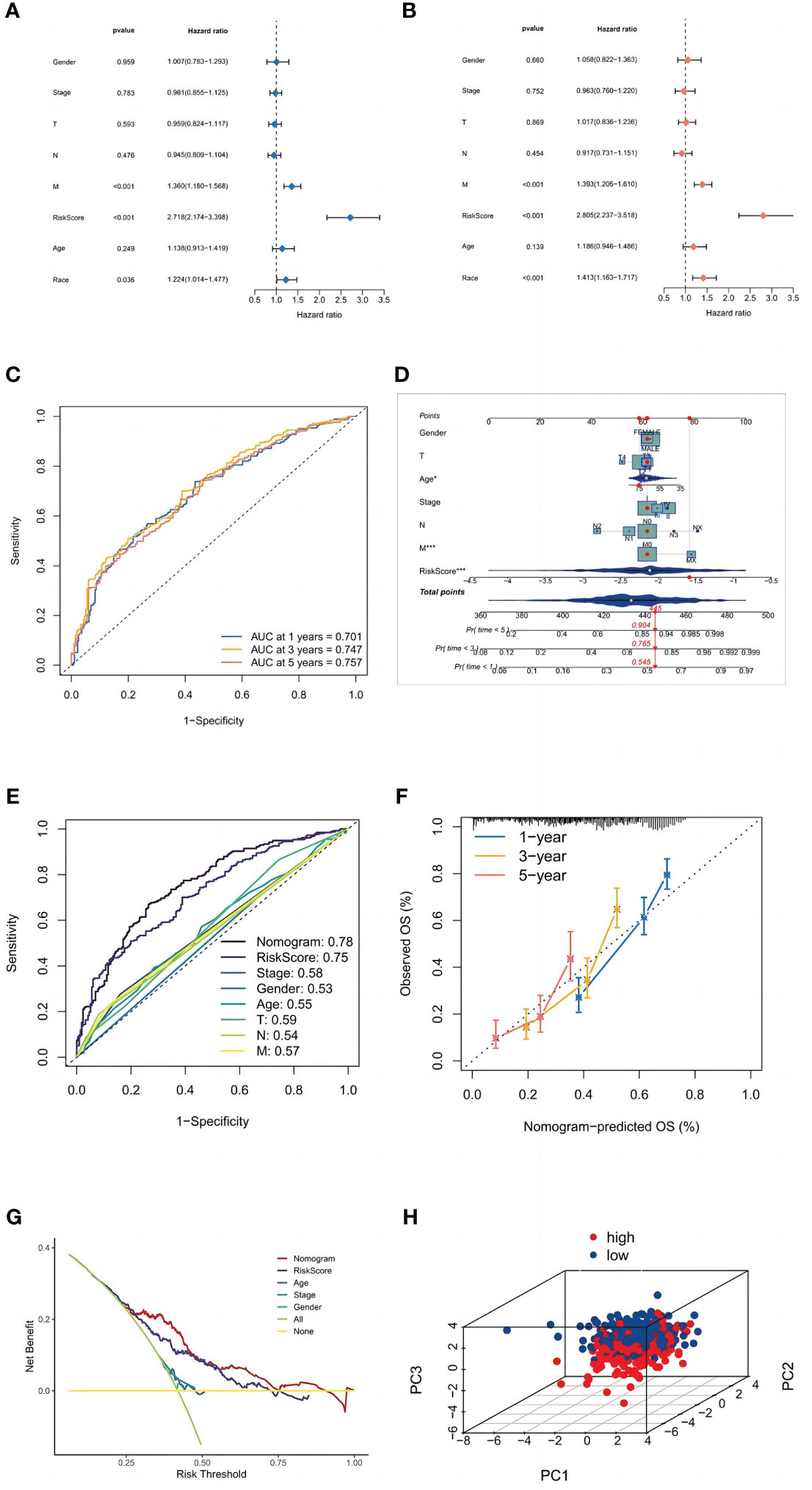
Figure 6 Validation of the model. (A) Univariate and (B) multivariate Cox regression analyses. (C) ROC curves of 1, 3, and 5 years. (D) The nomogram prediction model. (E) ROC curves of risk scores and clinical characteristics. (F) A calibration curve. (G) A DCA of the nomogram prediction model and the TNM staging system. (H) Principal component analysis of high and low-risk groups.
3.5 Construction of nomogram and validation of clinical subgroups and PCA
Age, gender, TNM stage, T stage, M stage, N stage, and the risk score from the signature were used to construct a nomogram (Figure 6D) that could accurately predict the 1-, 3-, and 5-year OS of LUSC patients. Furthermore, ROC curves and decision curves were used to test the effectiveness of nomograms (Figures 6E, F). The nomogram’s AUC was 0.780, which was higher than the risk score of 0.75 and indicates that the nomogram has reliable diagnostic significance. Then, decision curve analysis(DCA) demonstrated that the nomogram outperformed the other six molecular categorization techniques in terms of clinical net benefit (Figure 6G). Finally, we used PCA to look at the distribution of risk genes among patients, and the results showed that these genes could be relied upon to generate the signature (Figure 6H).
3.6 Gene Ontology and Kyoto Encyclopedia of Genes and Genomes pathway analysis
GO and KEGG enrichment analyses on common targets were performed to investigate the biological activities and pathways of naringenin against COVID-19/LUSC. As a consequence, 3133 GO terms were highlighted (BP: 2793, CC: 92, and MF: 248), as well as 183 KEGG pathways. The top 10 GO terms of each ontology and the top 30 KEGG pathways are presented as bubble charts (Figures 7A, B). Representative BP terms included the response to xenobiotic stimulus, cellular response to chemical stress, response to oxidative stress, response to nutrient levels, response to lipopolysaccharide, response to molecule of bacterial origin, cellular response to xenobiotic stimulus, reactive oxygen species metabolic process, cellular response to oxidative stress, gland development, etc. Representative CC terms included protein kinase complex, cyclin-dependent protein kinase holoenzyme complex, vesicle lumen, secretory granule lumen, cytoplasmic vesicle lumen, etc. Representative MF terms included nuclear receptor activity, ligand-activated transcription factor activity, carboxylic acid binding, monocarboxylic acid binding, protein serine/threonine kinase activity, protein tyrosine kinase activity, etc. In addition, representative pathways included the PI3K-Akt signaling pathway, the VEGF signaling pathway, the HIF-1 signaling pathway, Pancreatic cancer, human cytomegalovirus infection, toxoplasmosis, prostate cancer, Chemical carcinogenesis—receptor activation, non-small cell lung cancer, chemical carcinogenesis—reactive oxygen species, Kaposi sarcoma-associated herpesvirus infection, proteoglycans in cancer, small cell lung cancer, endocrine resistance, apoptosis; colorectal cancer, thyroid hormone signaling pathway, tuberculosis, etc. In conclusion, Go and KEGG analysis highlighted that naringenin’s anti-inflammatory, antiviral, and anticancer properties are important targets/pathways in COVID-19/LUSC treatment.
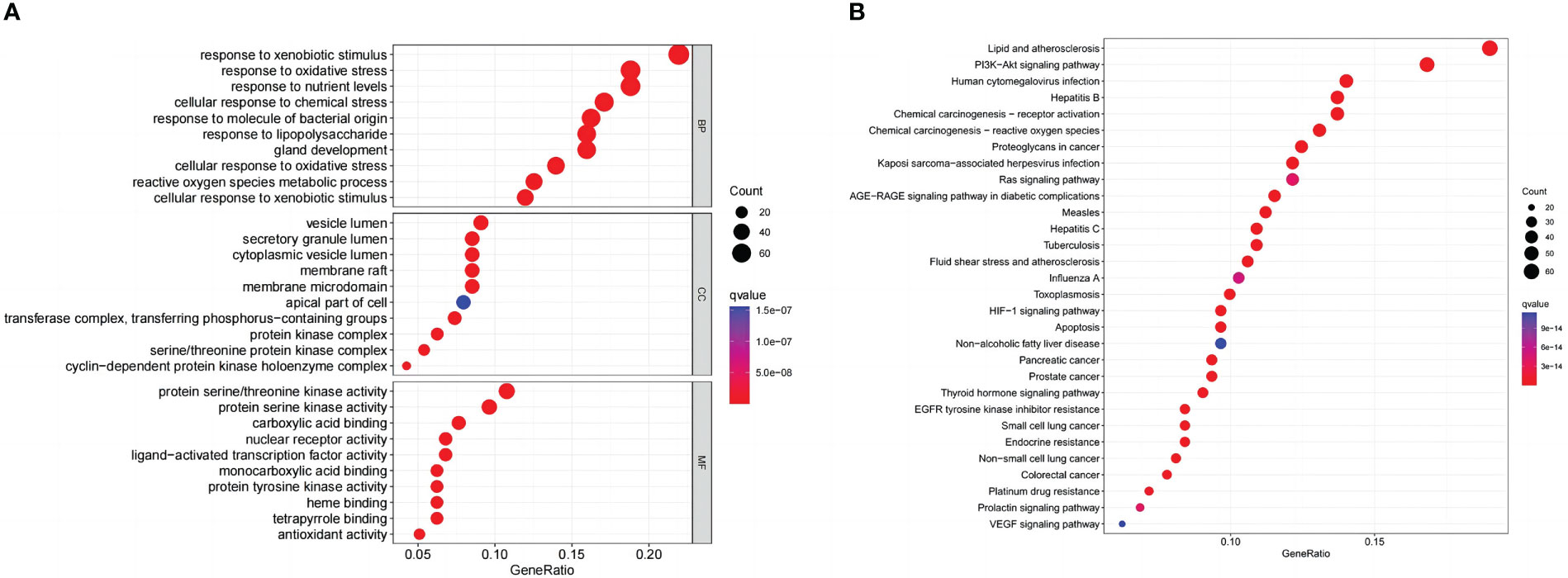
Figure 7 Naringenin functional characterization against COVID-19/LUSC. (A) GO analysis of naringenin and COVID-19/LUSC intersecting genes. (B) KEGG analysis of naringenin and COVID-19/LUSC intersecting genes.
3.7 PPI network analysis
The PPI network of common targets contained 268 nodes and 1,358 edges, which represented targets and interactions between targets, respectively. In the fight against COVID-19/LUSC, a node with a darker color and a larger form is more important. According to Figure 8, the top 6 targets with the highest degree values were AKT1 (degree = 55), TP53 (degree = 54), SRC (degree = 54), MAPK1 (degree = 49), MAPK3 (degree = 49), and HSP90AA1 (degree = 49). Consequently, molecular docking with naringenin was performed using AKT1, TP53, SRC, MAPK1, MAPK3, and HSP90AA1 as the hub targets for naringenin to cure COVID-19/LUSC.
3.8 Molecular docking
In general, stronger binding conformations and higher interaction probabilities result from lower binding energies. Related studies revealed that binding energy< 0 kJ/mol imply spontaneous binding, and -5.0 kJ/mol or lower indicates good binding activity (66). We analyzed the possible binding of naringenin with the six COVID-19/LUSC hub targets (AKT1, TP53, SRC, HSP90AA1, MAPK3, and MAPK1) identified previously and found that all of the docking results showed strong binding activity. Amino acid residues ASN-53, ILE-290, and THR-211 in AKT1, amino acid residues GLN-183, ASN-164, GLN-248, GLU-349, and ASN-345 in TP53, amino acid residues GLU-162, PTR-101, LYS-155, and LYS-198 in SRC, amino acid residues GLY-97 in HSP90AA1, amino acid residues LYS-131, ASP-128, ASN-171, ASP-184, and ASP-123 in MAPK3, amino acid residues ASN-144 in MAPK1, and naringenin form hydrogen bonds tightly. Overall, our findings demonstrated the high affinity between naringenin with AKT1, TP53, SRC, HSP90AA1, MAPK3, and MAPK1 (Figures 9A–F).
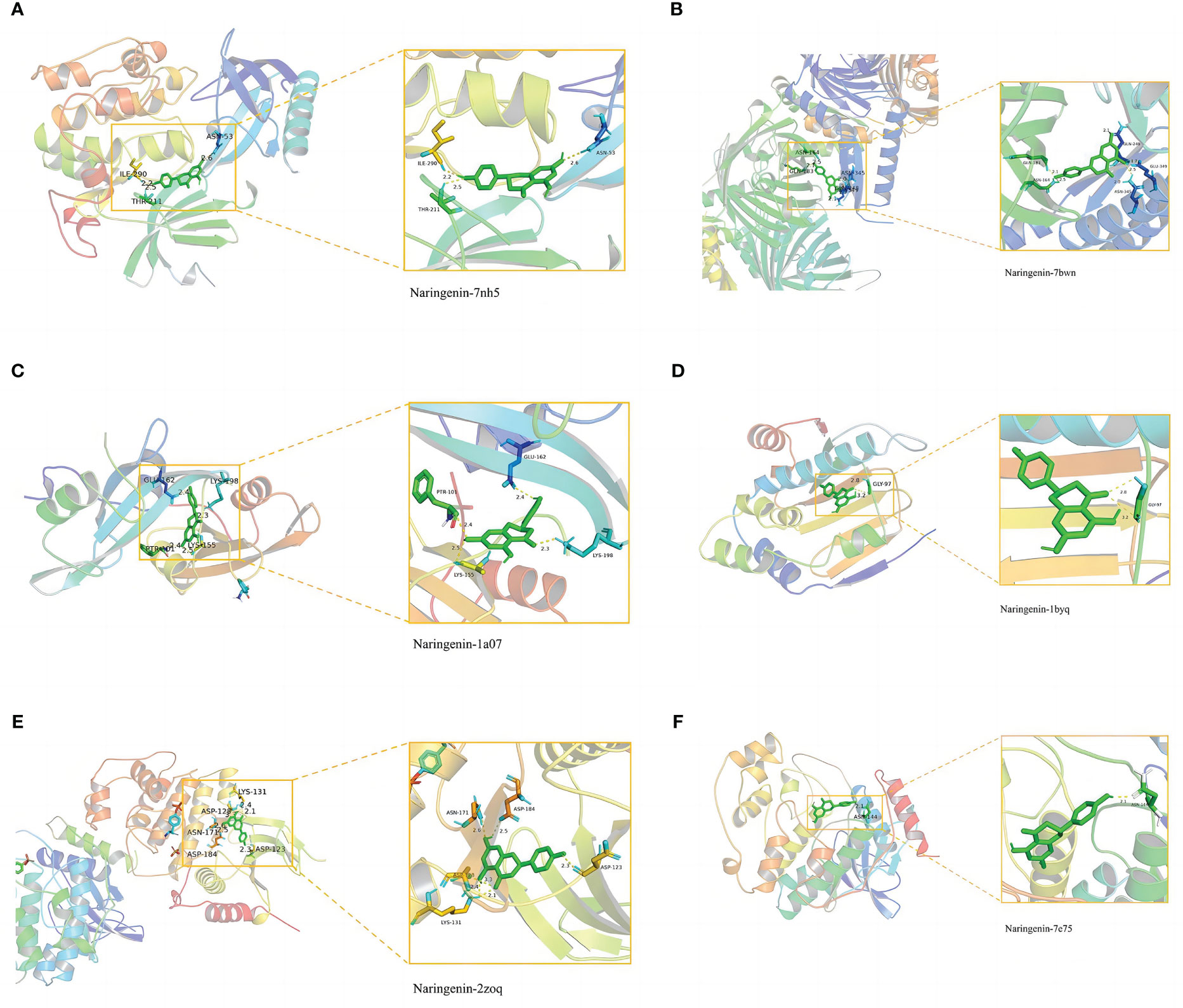
Figure 9 Molecular docking of naringenin and the targets AKT1, TP53, SRC, HSP90AA1, MAPK3, and MAPK1. (A) The binding of naringenin with the 7nh5 protein of AKT1. (B) The binding of naringenin with the 7bwn protein of TP53. (C) The binding of naringenin with the 1a07 protein of SRC. (D) The binding of naringenin with the 1byq protein of HSP90AA1. (E) The binding of naringenin with the 2zoq protein of MAPK3. (F) The binding of naringenin with the 7e75 protein of MAPK1.
4 Discussion
COVID-19 is a serious, rapidly spreading infectious disease that can be fatal (67). At this time, it is known that older age, male sex, diabetes mellitus, obesity, cardiovascular disease, and cancer are risk factors for COVID-19 infection and serious outcomes (68, 69). Cancer patients are vulnerable to respiratory viruses due to immunosuppression caused by the disease or therapy. This susceptibility has been demonstrated with influenza, which has been linked to a higher mortality rate in people with solid and hematologic cancer (70). Lung cancer patients from COVID-19 had the highest death risk compared to those with other cancers, which is most likely related to advancing age, a decline in lung reserve, concomitant conditions, and cancer treatment (71). We investigated the potential usefulness and molecular mechanisms of naringenin for COVID-19/LUSC using bioinformatics and system pharmacology methodologies based on the extensive biological properties of naringenin, including anti-inflammatory, anti-tumor, and antiviral. This might offer a fresh option for raising the survival rate of COVID-19/LUSC patients and stopping the spread of SARS-COV-2 through the digestive system.
In the present study, the potential mechanism and prognostic value of naringenin in COVID-19/LUSC were comprehensively investigated through network pharmacology and bioinformatic analyses, and we acquired 354 common targets of naringenin against COVID-19/LUSC. According to GO and KEGG enrichment analysis, the mechanisms for naringenin treatment of COVID-19/LUSC may be related to oxidative stress, immunoregulation, apoptosis, antiviral, anti-inflammatory, anti-cancer, and associated signaling pathways such as PI3K-Akt, HIF-1, and VEGF. Additionally, molecular docking demonstrated that naringenin and the top 6 COVID-19/LUSC related-target proteins had strong binding activity. As a result, the findings illustrate that naringenin holds a lot of promise as a treatment for COVID-19/LUSC.
4.1 Excellent prognostic analysis of LUSC patients with COVID-19
We first collected 13422 COVID-19 targets, 568 naringenin targets, and 22232 LUSC targets, and then further screened out 354 common targets, then selected 472 patient samples for follow-up prognostic analysis. Subsequently, a thirteen-gene signature containing ACTB, BAD, CYP1A1, ESRRA, FECH, LRRC27, MIF, MMP9, NOS1, PDE4D, PDE5A, SREBF1,and WARS1 was developed through univariate and multivariate Cox analyses, LUSC patients with COVID-19 may benefit from independent prognostic variables. Additionally, multivariate ROC analysis demonstrated that risk ratings were significantly more accurate than conventional pathological prognostic variables in predicting OS. Analysis of the nomogram revealed that the prognostic signature may be utilized to predict the outcomes of LUSC patients who have the COVID-19 mutation.
4.2 Hub targets could be set off by Naringenin to fight COVID-19/LUSC
In the PPI network of shared targets, which we first obtained, there were 268 nodes and 1,358 edges for the 354 common targets of naringenin and COVID-19/LUSC. In addition, we chose the top six targets in the PPI network as well as essential proteins connected to COVID-19/LUSC for molecular docking with naringenin. There were significant variations in the expression of the top six target genes, which included AKT1, TP53, SRC, MAPK1, MAPK3, and HSP90AA1. A member of the serine/threonine protein kinase subfamily known as Akt1 (72), viral protein synthesis is facilitated by overexpressed AKT1, and silencing of AKT1 results in reduced viral RNA expression, suppression of viral capsid protein synthesis, and virus release (73, 74). According to a study, which supports our hypothesis, AKT1 inhibition lowers viral yields in Huh7 cells infected with SARS-CoV-2 (75). An important aspect of the development of cancer is cell migration and motility, in different malignancies, Akt1 activation and expression leads to the advancement of carcinogenesis and metastasis (76). By controlling the expression of certain genes, such as those in the Akt signaling pathway, Akt1 plays a significant role in the development of tumors. Furthermore, AKT1 regulates innate immunity, which affects macrophage immunological function and the activated phenotype. AKT1 activation increases inflammatory and metabolic responses, making it a suitable target for COVID-19 therapy (57, 77, 78). The p53 protein, expressed by the TP53 gene, has been dubbed the “guardian of the genome” due to its involvement in responding to DNA damage by inducing cell cycle arrest, apoptosis, and/or senescence (79). The potential for TP53 gene therapy through SGT-53 to suppress viral infections against the many SARS-CoV-2 variants that have evolved or may develop throughout the COVID-19 pandemic (80). The gene that suppresses tumors One of the most frequently altered genes in human lung cancer is TP53 (81). In addition to causing tumor development, defects in TP53 function impair the response of malignant cells to anticancer drugs, especially those that induce DNA damage (82) and TP53 mutations are more common than average in LUSC (83). Therefore, it is anticipated that TP53 would be crucial in the fight against LUSC (84). When MAPK family members and the MAPK-STAT3 axis are activated, inflammatory factors such as IL-1, TNF-, and IL-6 are overexpressed (85). The MAPK1 signaling pathway has been associated to ALI/ARDS inflammation (86). Many cytokines, including IL-1, TNF-, and IL-6, play important roles in ALI/ARDS, primarily via the MPAK1 signal transduction pathway (87), The study discovered that an inhibitor of MAPK3/MAPK1 following carrageenan induced a reduction in all inflammation parameters assessed, which could be effective in the treatment of numerous inflammatory illnesses (88),Therefore, we have reason to believe that regulating MAPK pathway has positive significance for improving the expression of inflammatory factors in COVID-19. Meanwhile, MAPK1 restoration inhibited the proliferation, migration, and invasion of NSCLC cells (89). HSP90AA1 is a gene that promotes squamous cell lung cancer progression (90) and has an important regulatory role in non-small cell lung cancer (91). Simultaneously, the study discovered that decreasing HSP90AA1 expression can lower inflammatory factors, ROS generation, cell apoptosis rate, and autophagy-related proteins (92). These results further suggested that naringenin could be an effective pharmaceutical target for these intersecting genes against LUSC and COVID-19.
4.3 The critical mechanisms for naringenin to combat COVID-19/LUSC
The mechanism of naringenin against COVID-19/LUSC is strongly linked to oxidative stress, immunoregulation, apoptosis, antiviral, anti-inflammatory, anti-cancer, and related pathways including PI3K-Akt, HIF-1, and VEGF signaling pathway, according to the findings of GO and KEGG enrichment study. Excessive oxidative stress impairs immune system performance, increasing the risk of SARS-CoV-2 viral invasion in the body (93). Cancer, neurodegeneration, cardiovascular conditions, diabetes, and other illnesses also can be brought on by abnormal oxidative stress (94). The major characteristic of cancer cells is reduced apoptosis, which is accomplished by modifying important signaling molecules or pathways. The proto-oncogene Akt, whose expression and activation are increased in a variety of cancers, including LUSC, contributes to the resistance of cancer cells to chemotherapy and radiation therapy (95, 96). High basal levels of PI3K-Akt activation in clinical samples suggested an aggressive type of LUSC (97). The PI3K-Akt signaling pathway is specifically activated by CD147, which is crucial in the entry of SARS-CoV-2 into cells, and its shutting down will prevent some viruses from entering cells (98). Furthermore, by inhibiting the PI3K/AKT/mTOR signaling pathway, SARS-CoV-2 spike pseudovirions promote the appearance of autophagy, which in turn initiates apoptosis (99). Another hallmark of COVID-19 is tissue hypoxia, which is related to overexpression of the HIF-1along with their immunometabolic and immune-response implications (100). HIF-1, as one of the hypoxia signal transcription factors, regulates the expression of genes involved in metabolism in macrophages and T cells, promoting an inflammatory response (101, 102). The HIF-1 pathway regulates oxidative stress, hypoxia, and inflammation, and its activity may promote SARS-CoV-2 infection and affect a variety of physiological processes (103). HIF-1 is a fibroblast master regulator of lipid metabolism that contributes to a tumor-promoting phenotype in lung fibroblasts (104). Therapy for lung cancer may benefit from focusing on the HIF-1/SCD1 axis in CAFs (65). HIF-1 is thus a potential target in research against COVID-19/LUSC due to the importance of HIF-1 stabilization in tumor progression. As is generally known, VEGF is the best-characterized mediator of angiogenesis at the molecular level (105). Cancer cells that overexpress VEGF exhibit enhanced tumorigenicity, invasiveness, proliferation, and EMT features (106). Therefore, VEGF coordinates non-angiogenic events that are crucial for the early spread of tumors (107). When VEGF levels are elevated, it results in high permeability, edema, and tissue injury, which are the pathophysiologic causes of acute lung injury in COVID-19 patients (108, 109). The VEGF signaling pathway, on the other hand, raises angiotensin II(Ang II) levels to promote inflammation, while Ang II can also raise VEGF to promote the release of inflammatory cytokines (110). In conclusion, Naringenin may have an antiviral, anti-inflammatory, and anti-cancer effect by regulating oxidative stress, apoptosis, HIF-1, PI3K-Akt, and VEGF signaling pathways in order to alleviate the clinical symptoms of COVID-19/LUSC patients.
Last but not least, molecular docking data showed that naringenin has strong binding capabilities with the six COVID-19/LUSC targets, demonstrating that naringenin can effectively bind to specific proteins connected to COVID-19/LUSC. According to network pharmacology, naringenin can be used to treat SARS-CoV-2-infected patients with LUSC. More experimental investigation, however, is required to confirm and investigate the expected targets and their regulatory processes.
To sum up, We created a trustworthy predictive model for patients with LUSC and COVID-19 as well as many possible treatment targets of COVID-19/LUSC. Further, through the pharmacological actions and possible targets that have been identified, naringenin may be used to treat COVID-19/LUSC, including immunomodulation, antivirals, anti-inflammation, etc. Additionally, we were able to find direct binding sites that had a strong affinity for naringenin against COVID-19/LUSC, which gave the justification for further clinical trials as well as the proof for its use in clinical settings.
4.4 Strengths and limitations
Notably, our study offered some novel insights into naringenin in the therapy of COVID-19/LUSC and suggested plausible molecular pathways and prospective pharmacological targets of naringenin for the first time. However, a few remaining issues with our study’s limitations must be resolved. Since the results of this study were not verified in actual LUSC patients with COVID-19, future confirmation of these findings will need the recruitment of actual LUSC patients with COVID-19. Second, additional in vivo and in vitro studies are necessary to confirm the hypothesized mechanisms and pharmacological targets in order to confirm the potential therapeutic application of naringenin for COVID-19/LUSC.
Data availability statement
The datasets presented in this study can be found in online repositories. The names of the repository/repositories and accession number(s) can be found in the article/Supplementary Material.
Author contributions
S-fZ, KW, and X-fH conceived and designed this research. W-yW and XJ wrote the manuscript and participated in the design of the study. W-yW and XJ were responsible for the bioinformatics analysis and network construction. P-qX and W-xS carried out the data analysis and data interpretation. PW helped to modify the manuscript. All authors contributed to the article and approved the submitted version.
Funding
This work was supported by the National Natural Science Foundation of China (Grant no. 81973814), Special projects in key fields of ordinary colleges and universities in Guangdong Province (Grant no. 2022ZDZX2014), Guangdong Postgraduate Education Innovation Plan Project (Grant no. 2022ANLK025), Guangdong Traditional Chinese Medicine Research Project (Grant no. 20231169), Science, Technology and Innovation Commission of Shenzhen Municipality (Grant No. JSGG20220226090550002), and Sanming Project of Medicine in Shenzhen (No. SZZYSM202206013).
Acknowledgments
We thank the distinguished investigators for generously publishing their research data on COVID-19 and LUSC. The authors thank S-fZ for his enthusiastic help and selfless assistance in initiating this study.
Conflict of interest
The authors declare that the research was conducted in the absence of any commercial or financial relationships that could be construed as a potential conflict of interest.
Publisher’s note
All claims expressed in this article are solely those of the authors and do not necessarily represent those of their affiliated organizations, or those of the publisher, the editors and the reviewers. Any product that may be evaluated in this article, or claim that may be made by its manufacturer, is not guaranteed or endorsed by the publisher.
Supplementary material
The Supplementary Material for this article can be found online at: https://www.frontiersin.org/articles/10.3389/fendo.2023.1187882/full#supplementary-material
References
1. Struyf T, Deeks JJ, Dinnes J, Takwoingi Y, Davenport C, Leeflang MM, et al. Signs and symptoms to determine if a patient presenting in primary care or hospital outpatient settings has covid-19 disease. Cochrane Database Syst Rev (2020) 7(7):Cd013665. doi: 10.1002/14651858.Cd013665
2. Berlin DA, Gulick RM, Martinez FJ. Severe covid-19. N Engl J Med (2020) 383(25):2451–60. doi: 10.1056/NEJMcp2009575
3. Hariri L, Hardin CC. Covid-19, angiogenesis, and Ards endotypes. N Engl J Med (2020) 383(2):182–3. doi: 10.1056/NEJMe2018629
4. Riphagen S, Gomez X, Gonzalez-Martinez C, Wilkinson N, Theocharis P. Hyperinflammatory shock in children during covid-19 pandemic. Lancet (2020) 395(10237):1607–8. doi: 10.1016/s0140-6736(20)31094-1
5. Noval Rivas M, Porritt RA, Cheng MH, Bahar I, Arditi M. Covid-19-Associated multisystem inflammatory syndrome in children (Mis-c): a novel disease that mimics toxic shock syndrome-the superantigen hypothesis. J Allergy Clin Immunol (2021) 147(1):57–9. doi: 10.1016/j.jaci.2020.10.008
6. Li J, Wang X, Chen J, Zuo X, Zhang H, Deng A. Covid-19 infection may cause ketosis and ketoacidosis. Diabetes Obes Metab (2020) 22(10):1935–41. doi: 10.1111/dom.14057
7. Kim JS, Lee JY, Yang JW, Lee KH, Effenberger M, Szpirt W, et al. Immunopathogenesis and treatment of cytokine storm in covid-19. Theranostics (2021) 11(1):316–29. doi: 10.7150/thno.49713
8. Iwasaki M, Saito J, Zhao H, Sakamoto A, Hirota K, Ma D. Inflammation triggered by sars-Cov-2 and Ace2 augment drives multiple organ failure of severe covid-19: molecular mechanisms and implications. Inflammation (2021) 44(1):13–34. doi: 10.1007/s10753-020-01337-3
9. WHO. Who coronavirus (Covid-19) dashboard. Available at: https://covid19.who.int/ (Accessed December 3, 2022).
10. Rabaan AA, Al-Ahmed SH, Sah R, Tiwari R, Yatoo MI, Patel SK, et al. Sars-Cov-2/Covid-19 and advances in developing potential therapeutics and vaccines to counter this emerging pandemic. Ann Clin Microbiol antimicrobials (2020) 19(1):40. doi: 10.1186/s12941-020-00384-w
11. Wang Y, Zhang D, Du G, Du R, Zhao J, Jin Y, et al. Remdesivir in adults with severe covid-19: a randomised, double-blind, placebo-controlled, multicentre trial. Lancet (2020) 395(10236):1569–78. doi: 10.1016/s0140-6736(20)31022-9
12. Luo J, Rizvi H, Preeshagul IR, Egger JV, Hoyos D, Bandlamudi C, et al. Covid-19 in patients with lung cancer. Ann Oncol (2020) 31(10):1386–96. doi: 10.1016/j.annonc.2020.06.007
13. Turkington RC, Lavery A, Donnelly D, Cairnduff V, McManus DT, Coleman HG. The impact of the covid-19 pandemic on barrett's esophagus and esophagogastric cancer. Gastroenterology (2021) 160(6):2169–71.e1. doi: 10.1053/j.gastro.2021.01.208
14. Patel S, Issaka RB, Chen E, Somsouk M. Colorectal cancer screening and covid-19. Am J Gastroenterol (2021) 116(2):433–4. doi: 10.14309/ajg.0000000000000970
15. Parmar HS, Nayak A, Gavel PK, Jha HC, Bhagwat S, Sharma R. Cross talk between covid-19 and breast cancer. Curr Cancer Drug Targets (2021) 21(7):575–600. doi: 10.2174/1568009621666210216102236
16. Han HJ, Nwagwu C, Anyim O, Ekweremadu C, Kim S. Covid-19 and cancer: from basic mechanisms to vaccine development using nanotechnology. Int Immunopharmacol (2021) 90:107247. doi: 10.1016/j.intimp.2020.107247
17. Grivas P, Khaki AR, Wise-Draper TM, French B, Hennessy C, Hsu CY, et al. Association of clinical factors and recent anticancer therapy with covid-19 severity among patients with cancer: a report from the covid-19 and cancer consortium. Ann Oncol (2021) 32(6):787–800. doi: 10.1016/j.annonc.2021.02.024
18. Siegel RL, Miller KD, Fuchs HE, Jemal A. Cancer statistics, 2021. CA Cancer J Clin (2021) 71(1):7–33. doi: 10.3322/caac.21654
19. Sun D, Li H, Cao M, He S, Lei L, Peng J, et al. Cancer burden in China: trends, risk factors and prevention. Cancer Biol Med (2020) 17(4):879–95. doi: 10.20892/j.issn.2095-3941.2020.0387
20. Wang X, Fang J, Zhu Y, Chen L, Ding F, Zhou R, et al. Clinical characteristics of non-critically ill patients with novel coronavirus infection (Covid-19) in a fangcang hospital. Clin Microbiol Infect (2020) 26(8):1063–8. doi: 10.1016/j.cmi.2020.03.032
21. Kong Q, Xiang Z, Wu Y, Gu Y, Guo J, Geng F. Analysis of the susceptibility of lung cancer patients to sars-Cov-2 infection. Mol Cancer (2020) 19(1):80. doi: 10.1186/s12943-020-01209-2
22. Zaidun NH, Thent ZC, Latiff AA. Combating oxidative stress disorders with citrus flavonoid: naringenin. Life Sci (2018) 208:111–22. doi: 10.1016/j.lfs.2018.07.017
23. Nouri Z, Fakhri S, El-Senduny FF, Sanadgol N, Abd-ElGhani GE, Farzaei MH, et al. On the neuroprotective effects of naringenin: pharmacological targets, signaling pathways, molecular mechanisms, and clinical perspective. Biomolecules (2019) 9(11). doi: 10.3390/biom9110690
24. Patel K, Singh GK, Patel DK. A review on pharmacological and analytical aspects of naringenin. Chin J Integr Med (2018) 24(7):551–60. doi: 10.1007/s11655-014-1960-x
25. Den Hartogh DJ, Tsiani E. Antidiabetic properties of naringenin: a citrus fruit polyphenol. Biomolecules (2019) 9(3). doi: 10.3390/biom9030099
26. Zhou T, Liu L, Wang Q, Gao Y. Naringenin alleviates cognition deficits in high-fat diet-fed Samp8 mice. J Food Biochem (2020) 44(9):e13375. doi: 10.1111/jfbc.13375
27. Yao W, Zhang X, Xu F, Cao C, Liu T, Xue Y. The therapeutic effects of naringenin on bronchial pneumonia in children. Pharmacol Res Perspect (2021) 9(4):e00825. doi: 10.1002/prp2.825
28. Hua YQ, Zeng Y, Xu J, Xu XL. Naringenin alleviates nonalcoholic steatohepatitis in middle-aged apoe(-/-)Mice: role of Sirt1. Phytomedicine (2021) 81:153412. doi: 10.1016/j.phymed.2020.153412
29. Wang Q, Ou Y, Hu G, Wen C, Yue S, Chen C, et al. Naringenin attenuates non-alcoholic fatty liver disease by down-regulating the Nlrp3/Nf-κb pathway in mice. Br J Pharmacol (2020) 177(8):1806–21. doi: 10.1111/bph.14938
30. Yang W, Ma J, Liu Z, Lu Y, Hu B, Yu H. Effect of naringenin on brain insulin signaling and cognitive functions in icv-stz induced dementia model of rats. Neurol Sci (2014) 35(5):741–51. doi: 10.1007/s10072-013-1594-3
31. Kesh S, Kannan RR, Balakrishnan A. Naringenin alleviates 6-hydroxydopamine induced parkinsonism in Shsy5y cells and zebrafish model. Comp Biochem Physiol C Toxicol Pharmacol (2021) 239:108893. doi: 10.1016/j.cbpc.2020.108893
32. Lou H, Jing X, Wei X, Shi H, Ren D, Zhang X. Naringenin protects against 6-Ohda-Induced neurotoxicity Via activation of the Nrf2/Are signaling pathway. Neuropharmacology (2014) 79:380–8. doi: 10.1016/j.neuropharm.2013.11.026
33. Tutunchi H, Naeini F, Ostadrahimi A, Hosseinzadeh-Attar MJ. Naringenin, a flavanone with antiviral and anti-inflammatory effects: a promising treatment strategy against covid-19. Phytother Res (2020) 34(12):3137–47. doi: 10.1002/ptr.6781
34. Palmeira P, Barbuto JAM, Silva CAA, Carneiro-Sampaio M. Why is sars-Cov-2 infection milder among children? Clinics (Sao Paulo) (2020) 75:e1947. doi: 10.6061/clinics/2020/e1947
35. Conti P, Ronconi G, Caraffa A, Gallenga CE, Ross R, Frydas I, et al. Induction of pro-inflammatory cytokines (Il-1 and il-6) and lung inflammation by coronavirus-19 (Covi-19 or sars-Cov-2): anti-inflammatory strategies. J Biol Regul Homeost Agents (2020) 34(2):327–31. doi: 10.23812/conti-e
36. Monteleone G, Ardizzone S. Are patients with inflammatory bowel disease at increased risk for covid-19 infection? J Crohns Colitis (2020) 14(9):1334–6. doi: 10.1093/ecco-jcc/jjaa061
37. Stebbing J, Phelan A, Griffin I, Tucker C, Oechsle O, Smith D, et al. Covid-19: combining antiviral and anti-inflammatory treatments. Lancet Infect Dis (2020) 20(4):400–2. doi: 10.1016/s1473-3099(20)30132-8
38. Gupta SC, Tyagi AK, Deshmukh-Taskar P, Hinojosa M, Prasad S, Aggarwal BB. Downregulation of tumor necrosis factor and other proinflammatory biomarkers by polyphenols. Arch Biochem Biophys (2014) 559:91–9. doi: 10.1016/j.abb.2014.06.006
39. Jin L, Zeng W, Zhang F, Zhang C, Liang W. Naringenin ameliorates acute inflammation by regulating intracellular cytokine degradation. J Immunol (2017) 199(10):3466–77. doi: 10.4049/jimmunol.1602016
40. Tahir Ul Qamar M, Alqahtani SM, Alamri MA, Chen LL. Structural basis of sars-Cov-2 3cl(Pro) and anti-Covid-19 drug discovery from medicinal plants. J Pharm Anal (2020) 10(4):313–9. doi: 10.1016/j.jpha.2020.03.009
41. Chang KO, Kim Y, Lovell S, Rathnayake AD, Groutas WC. Antiviral drug discovery: norovirus proteases and development of inhibitors. Viruses (2019) 11(2). doi: 10.3390/v11020197
42. Chang HL, Chang YM, Lai SC, Chen KM, Wang KC, Chiu TT, et al. Naringenin inhibits migration of lung cancer cells Via the inhibition of matrix metalloproteinases-2 and -9. Exp Ther Med (2017) 13(2):739–44. doi: 10.3892/etm.2016.3994
43. Shi X, Luo X, Chen T, Guo W, Liang C, Tang S, et al. Naringenin inhibits migration, invasion, induces apoptosis in human lung cancer cells and arrests tumour progression in vitro. J Cell Mol Med (2021) 25(5):2563–71. doi: 10.1111/jcmm.16226
44. Kim S, Chen J, Cheng T, Gindulyte A, He J, He S, et al. Pubchem in 2021: new data content and improved web interfaces. Nucleic Acids Res (2021) 49(D1):D1388–d95. doi: 10.1093/nar/gkaa971
45. Gu S, Xue Y, Gao Y, Shen S, Zhang Y, Chen K, et al. Mechanisms of indigo naturalis on treating ulcerative colitis explored by geo gene chips combined with network pharmacology and molecular docking. Sci Rep (2020) 10(1):15204. doi: 10.1038/s41598-020-71030-w
46. Li R, Li Y, Liang X, Yang L, Su M, Lai KP. Network pharmacology and bioinformatics analyses identify intersection genes of niacin and covid-19 as potential therapeutic targets. Brief Bioinform (2021) 22(2):1279–90. doi: 10.1093/bib/bbaa300
47. Amberger JS, Bocchini CA, Schiettecatte F, Scott AF, Hamosh A. Omim.Org: online mendelian inheritance in man (Omim®), an online catalog of human genes and genetic disorders. Nucleic Acids Res (2015) 43(Database issue):D789–98. doi: 10.1093/nar/gku1205
48. Barrett T, Wilhite SE, Ledoux P, Evangelista C, Kim IF, Tomashevsky M, et al. Ncbi geo: archive for functional genomics data sets–update. Nucleic Acids Res (2013) 41(Database issue):D991–5. doi: 10.1093/nar/gks1193
49. Davis AP, Grondin CJ, Johnson RJ, Sciaky D, Wiegers J, Wiegers TC, et al. Comparative toxicogenomics database (Ctd): update 2021. Nucleic Acids Res (2021) 49(D1):D1138–d43. doi: 10.1093/nar/gkaa891
50. Liu Z, Guo F, Wang Y, Li C, Zhang X, Li H, et al. Batman-tcm: a bioinformatics analysis tool for molecular mechanism of traditional Chinese medicine. Sci Rep (2016) 6:21146. doi: 10.1038/srep21146
51. Xu HY, Zhang YQ, Liu ZM, Chen T, Lv CY, Tang SH, et al. Etcm: an encyclopaedia of traditional Chinese medicine. Nucleic Acids Res (2019) 47(D1):D976–d82. doi: 10.1093/nar/gky987
52. Wu Y, Zhang F, Yang K, Fang S, Bu D, Li H, et al. Symmap: an integrative database of traditional Chinese medicine enhanced by symptom mapping. Nucleic Acids Res (2019) 47(D1):D1110–d7. doi: 10.1093/nar/gky1021
53. Daina A, Michielin O, Zoete V. Swisstargetprediction: updated data and new features for efficient prediction of protein targets of small molecules. Nucleic Acids Res (2019) 47(W1):W357–w64. doi: 10.1093/nar/gkz382
54. Wang X, Shen Y, Wang S, Li S, Zhang W, Liu X, et al. Pharmmapper 2017 update: a web server for potential drug target identification with a comprehensive target pharmacophore database. Nucleic Acids Res (2017) 45(W1):W356–w60. doi: 10.1093/nar/gkx374
55. Freshour SL, Kiwala S, Cotto KC, Coffman AC, McMichael JF, Song JJ, et al. Integration of the drug-gene interaction database (Dgidb 4.0) with open crowdsource efforts. Nucleic Acids Res (2021) 49(D1):D1144–d51. doi: 10.1093/nar/gkaa1084
56. Szklarczyk D, Gable AL, Lyon D, Junge A, Wyder S, Huerta-Cepas J, et al. String V11: protein-protein association networks with increased coverage, supporting functional discovery in genome-wide experimental datasets. Nucleic Acids Res (2019) 47(D1):D607–d13. doi: 10.1093/nar/gky1131
57. Xia QD, Xun Y, Lu JL, Lu YC, Yang YY, Zhou P, et al. Network pharmacology and molecular docking analyses on lianhua qingwen capsule indicate Akt1 is a potential target to treat and prevent covid-19. Cell Prolif (2020) 53(12):e12949. doi: 10.1111/cpr.12949
58. Wu T, Hu E, Xu S, Chen M, Guo P, Dai Z, et al. Clusterprofiler 4.0: a universal enrichment tool for interpreting omics data. Innovation (Camb) (2021) 2(3):100141. doi: 10.1016/j.xinn.2021.100141
59. Kanehisa M, Furumichi M, Tanabe M, Sato Y, Morishima K. Kegg: new perspectives on genomes, pathways, diseases and drugs. Nucleic Acids Res (2017) 45(D1):D353–d61. doi: 10.1093/nar/gkw1092
60. Liang Y, Zhou R, Liang X, Kong X, Yang B. Pharmacological targets and molecular mechanisms of plumbagin to treat colorectal cancer: a systematic pharmacology study. Eur J Pharmacol (2020) 881:173227. doi: 10.1016/j.ejphar.2020.173227
61. Wang Y, Bryant SH, Cheng T, Wang J, Gindulyte A, Shoemaker BA, et al. Pubchem bioassay: 2017 update. Nucleic Acids Res (2017) 45(D1):D955–d63. doi: 10.1093/nar/gkw1118
62. Kerwin SM. Chembiooffice ultra 2010 suite. J Am Chem Soc (2010) 132(7):2466–7. doi: 10.1021/ja1005306
63. Rose PW, Prlić A, Altunkaya A, Bi C, Bradley AR, Christie CH, et al. The rcsb protein data bank: integrative view of protein, gene and 3d structural information. Nucleic Acids Res (2017) 45(D1):D271–d81. doi: 10.1093/nar/gkw1000
64. Seeliger D, de Groot BL. Ligand docking and binding site analysis with pymol and Autodock/Vina. J Comput Aided Mol Des (2010) 24(5):417–22. doi: 10.1007/s10822-010-9352-6
65. Barrios O, Sánchez BG, Rodríguez-Prieto T, Cano J, Bort A, Gómez R, et al. Alteration of the hif-1α/Vegf signaling pathway and disruption of the cell cycle by second generation carbosilan dendrimers. Biomacromolecules (2022) 23(12):5043–55. doi: 10.1021/acs.biomac.2c00899
66. Yuan C, Wang MH, Wang F, Chen PY, Ke XG, Yu B, et al. Network pharmacology and molecular docking reveal the mechanism of scopoletin against non-small cell lung cancer. Life Sci (2021) 270:119105. doi: 10.1016/j.lfs.2021.119105
67. Yuki K, Fujiogi M, Koutsogiannaki S. Covid-19 pathophysiology: a review. Clin Immunol (2020) 215:108427. doi: 10.1016/j.clim.2020.108427
68. Tian J, Yuan X, Xiao J, Zhong Q, Yang C, Liu B, et al. Clinical characteristics and risk factors associated with covid-19 disease severity in patients with cancer in wuhan, China: a multicentre, retrospective, cohort study. Lancet Oncol (2020) 21(7):893–903. doi: 10.1016/s1470-2045(20)30309-0
69. Huang C, Wang Y, Li X, Ren L, Zhao J, Hu Y, et al. Clinical features of patients infected with 2019 novel coronavirus in wuhan, China. Lancet (2020) 395(10223):497–506. doi: 10.1016/s0140-6736(20)30183-5
70. Francisci D, Labianca R, Roila F. Prevention and treatment of pandemic influenza in cancer patients. Ann Oncol (2010) 21(12):2301–3. doi: 10.1093/annonc/mdq351
71. Khoury E, Nevitt S, Madsen WR, Turtle L, Davies G, Palmieri C. Differences in outcomes and factors associated with mortality among patients with sars-Cov-2 infection and cancer compared with those without cancer: a systematic review and meta-analysis. JAMA Netw Open (2022) 5(5):e2210880. doi: 10.1001/jamanetworkopen.2022.10880
72. Manning BD, Cantley LC. Akt/Pkb signaling: navigating downstream. Cell (2007) 129(7):1261–74. doi: 10.1016/j.cell.2007.06.009
73. Wang X, Zhang H, Abel AM, Young AJ, Xie L, Xie Z. Role of phosphatidylinositol 3-kinase (Pi3k) and Akt1 kinase in porcine reproductive and respiratory syndrome virus (Prrsv) replication. Arch Virol (2014) 159(8):2091–6. doi: 10.1007/s00705-014-2016-4
74. Esfandiarei M, Luo H, Yanagawa B, Suarez A, Dabiri D, Zhang J, et al. Protein kinase B/Akt regulates coxsackievirus B3 replication through a mechanism which is not caspase dependent. J Virol (2004) 78(8):4289–98. doi: 10.1128/jvi.78.8.4289-4298.2004
75. Appelberg S, Gupta S, Svensson Akusjärvi S, Ambikan AT, Mikaeloff F, Saccon E, et al. Dysregulation in Akt/Mtor/Hif-1 signaling identified by proteo-transcriptomics of sars-Cov-2 infected cells. Emerg Microbes Infect (2020) 9(1):1748–60. doi: 10.1080/22221751.2020.1799723
76. Bjerke GA, Yang CS, Frierson HF, Paschal BM, Wotton D. Activation of akt signaling in prostate induces a tgfβ-mediated restraint on cancer progression and metastasis. Oncogene (2014) 33(28):3660–7. doi: 10.1038/onc.2013.342
77. Zhang Y, Wang X, Yang H, Liu H, Lu Y, Han L, et al. Kinase akt controls innate immune cell development and function. Immunology (2013) 140(2):143–52. doi: 10.1111/imm.12123
78. Vergadi E, Ieronymaki E, Lyroni K, Vaporidi K, Tsatsanis C. Akt signaling pathway in macrophage activation and M1/M2 polarization. J Immunol (2017) 198(3):1006–14. doi: 10.4049/jimmunol.1601515
80. Harford JB, Kim SS, Pirollo KF, Chang EH. Tp53 gene therapy as a potential treatment for patients with covid-19. Viruses (2022) 14(4). doi: 10.3390/v14040739
81. Xu F, Lin H, He P, He L, Chen J, Lin L, et al. A Tp53-associated gene signature for prediction of prognosis and therapeutic responses in lung squamous cell carcinoma. Oncoimmunology (2020) 9(1):1731943. doi: 10.1080/2162402x.2020.1731943
82. Greathouse KL, White JR, Vargas AJ, Bliskovsky VV, Beck JA, von Muhlinen N, et al. Interaction between the microbiome and Tp53 in human lung cancer. Genome Biol (2018) 19(1):123. doi: 10.1186/s13059-018-1501-6
83. Jeong Y, Hoang NT, Lovejoy A, Stehr H, Newman AM, Gentles AJ, et al. Role of Keap1/Nrf2 and Tp53 mutations in lung squamous cell carcinoma development and radiation resistance. Cancer Discovery (2017) 7(1):86–101. doi: 10.1158/2159-8290.Cd-16-0127
84. Haakensen VD, Khadse A, Sandhu V, Halvorsen AR, Solberg SK, Jørgensen LH, et al. Molecular characterisation of Tp53 mutated squamous cell carcinomas of the lung to identify putative targets for therapy. Int J Cancer (2020) 147(10):2957–66. doi: 10.1002/ijc.33121
85. Bode JG, Ehlting C, Häussinger D. The macrophage response towards lps and its control through the P38(Mapk)-Stat3 axis. Cell Signal (2012) 24(6):1185–94. doi: 10.1016/j.cellsig.2012.01.018
86. Kumar S, Principe DR, Singh SK, Viswakarma N, Sondarva G, Rana B, et al. Mitogen-activated protein kinase inhibitors and T-Cell-Dependent immunotherapy in cancer. Pharm (Basel) (2020) 13(1). doi: 10.3390/ph13010009
87. Braicu C, Buse M, Busuioc C, Drula R, Gulei D, Raduly L, et al. A comprehensive review on mapk: a promising therapeutic target in cancer. Cancers (Basel) (2019) 11(10). doi: 10.3390/cancers11101618
88. Di Paola R, Crisafulli C, Mazzon E, Genovese T, Paterniti I, Bramanti P, et al. Effect of Pd98059, a selective Mapk3/Mapk1 inhibitor, on acute lung injury in mice. Int J Immunopathol Pharmacol (2009) 22(4):937–50. doi: 10.1177/039463200902200409
89. Zhang ZY, Gao XH, Ma MY, Zhao CL, Zhang YL, Guo SS. Circrna_101237 promotes nsclc progression Via the mirna-490-3p/Mapk1 axis. Sci Rep (2020) 10(1):9024. doi: 10.1038/s41598-020-65920-2
90. Wang L, Zhao H, Zhang L, Luo H, Chen Q, Zuo X. Hsp90aa1, Adrb2, Tbl1xr1 and Hspb1 are chronic obstructive pulmonary disease-related genes that facilitate squamous cell lung cancer progression. Oncol Lett (2020) 19(3):2115–22. doi: 10.3892/ol.2020.11318
91. Bhattacharyya N, Gupta S, Sharma S, Soni A, Bagabir SA, Bhattacharyya M, et al. Cdk1 and Hsp90aa1 appear as the novel regulatory genes in non-small cell lung cancer: a bioinformatics approach. J Pers Med (2022) 12(3). doi: 10.3390/jpm12030393
92. Zhang H, Huang J, Fan X, Miao R, Wang Y. Hsp90aa1 promotes the inflammation in human gingival fibroblasts induced by porphyromonas gingivalis lipopolysaccharide Via regulating of autophagy. BMC Oral Health (2022) 22(1):366. doi: 10.1186/s12903-022-02304-0
93. Iddir M, Brito A, Dingeo G, Fernandez Del Campo SS, Samouda H, La Frano MR, et al. Strengthening the immune system and reducing inflammation and oxidative stress through diet and nutrition: considerations during the covid-19 crisis. Nutrients (2020) 12(6). doi: 10.3390/nu12061562
94. Pisoschi AM, Pop A. The role of antioxidants in the chemistry of oxidative stress: a review. Eur J Med Chem (2015) 97:55–74. doi: 10.1016/j.ejmech.2015.04.040
95. Cheng H, Shcherba M, Pendurti G, Liang Y, Piperdi B, Perez-Soler R. Targeting the Pi3k/Akt/Mtor pathway: potential for lung cancer treatment. Lung Cancer Manag (2014) 3(1):67–75. doi: 10.2217/lmt.13.72
96. Balsara BR, Pei J, Mitsuuchi Y, Page R, Klein-Szanto A, Wang H, et al. Frequent activation of akt in non-small cell lung carcinomas and preneoplastic bronchial lesions. Carcinogenesis (2004) 25(11):2053–9. doi: 10.1093/carcin/bgh226
97. Kurgan N, Tsakiridis E, Kouvelioti R, Moore J, Klentrou P, Tsiani E. Inhibition of human lung cancer cell proliferation and survival by post-exercise serum is associated with the inhibition of akt, mtor, P70 S6k, and Erk1/2. Cancers (Basel) (2017) 9(5). doi: 10.3390/cancers9050046
98. Khezri MR. Pi3k/Akt signaling pathway: a possible target for adjuvant therapy in covid-19. Hum Cell (2021) 34(2):700–1. doi: 10.1007/s13577-021-00484-5
99. Li F, Li J, Wang PH, Yang N, Huang J, Ou J, et al. Sars-Cov-2 spike promotes inflammation and apoptosis through autophagy by ros-suppressed Pi3k/Akt/Mtor signaling. Biochim Biophys Acta Mol Basis Dis (2021) 1867(12):166260. doi: 10.1016/j.bbadis.2021.166260
100. Ferraro E, Germanò M, Mollace R, Mollace V, Malara N. Hif-1, the warburg effect, and Macrophage/Microglia polarization potential role in covid-19 pathogenesis. Oxid Med Cell Longev (2021) 2021:8841911. doi: 10.1155/2021/8841911
101. Zheng M, Karki R, Williams EP, Yang D, Fitzpatrick E, Vogel P, et al. Tlr2 senses the sars-Cov-2 envelope protein to produce inflammatory cytokines. Nat Immunol (2021) 22(7):829–38. doi: 10.1038/s41590-021-00937-x
102. Jiang P, Ye J, Jia M, Li X, Wei S, Li N. The common regulatory pathway of covid-19 and multiple inflammatory diseases and the molecular mechanism of cepharanthine in the treatment of covid-19. Front Pharmacol (2022) 13:960267. doi: 10.3389/fphar.2022.960267
103. Tian M, Liu W, Li X, Zhao P, Shereen MA, Zhu C, et al. Hif-1α promotes sars-Cov-2 infection and aggravates inflammatory responses to covid-19. Signal Transduct Target Ther (2021) 6(1):308. doi: 10.1038/s41392-021-00726-w
104. Zhang Y, Gu Z, Wan J, Lou X, Liu S, Wang Y, et al. Stearoyl-coa desaturase-1 dependent lipid droplets accumulation in cancer-associated fibroblasts facilitates the progression of lung cancer. Int J Biol Sci (2022) 18(16):6114–28. doi: 10.7150/ijbs.74924
105. Nagy JA, Dvorak AM, Dvorak HF. Vegf-a and the induction of pathological angiogenesis. Annu Rev Pathol (2007) 2:251–75. doi: 10.1146/annurev.pathol.2.010506.134925
106. Son M, Ryu B, Je JG, Jeon YJ, Kim DY. Ishophloroglucin a ameliorates vegf-induced epithelial-mesenchymal transition Via Vegfr2 pathway inhibition in microgravity-stimulated human retinal pigment epithelial cells. Antioxidants (Basel) (2022) 11(11). doi: 10.3390/antiox11112212
107. Gonzalez-Moreno O, Lecanda J, Green JE, Segura V, Catena R, Serrano D, et al. Vegf elicits epithelial-mesenchymal transition (Emt) in prostate intraepithelial neoplasia (Pin)-like cells Via an autocrine loop. Exp Cell Res (2010) 316(4):554–67. doi: 10.1016/j.yexcr.2009.11.020
108. Pine AB, Meizlish ML, Goshua G, Chang CH, Zhang H, Bishai J, et al. Circulating markers of angiogenesis and endotheliopathy in covid-19. Pulm Circ (2020) 10(4):2045894020966547. doi: 10.1177/2045894020966547
109. Patel BV, Arachchillage DJ, Ridge CA, Bianchi P, Doyle JF, Garfield B, et al. Pulmonary angiopathy in severe covid-19: physiologic, imaging, and hematologic observations. Am J Respir Crit Care Med (2020) 202(5):690–9. doi: 10.1164/rccm.202004-1412OC
Keywords: COVID-19, lung squamous cell carcinoma, naringenin, network pharmacology, bioinformatics
Citation: Wu W-y, Jiao X, Song W-x, Wu P, Xiao P-q, Huang X-f, Wang K and Zhan S-f (2023) Network pharmacology and bioinformatics analysis identifies potential therapeutic targets of Naringenin against COVID-19/LUSC. Front. Endocrinol. 14:1187882. doi: 10.3389/fendo.2023.1187882
Received: 16 March 2023; Accepted: 23 May 2023;
Published: 06 June 2023.
Edited by:
Jeff M. P. Holly, University of Bristol, United KingdomCopyright © 2023 Wu, Jiao, Song, Wu, Xiao, Huang, Wang and Zhan. This is an open-access article distributed under the terms of the Creative Commons Attribution License (CC BY). The use, distribution or reproduction in other forums is permitted, provided the original author(s) and the copyright owner(s) are credited and that the original publication in this journal is cited, in accordance with accepted academic practice. No use, distribution or reproduction is permitted which does not comply with these terms.
*Correspondence: Xiu-fang Huang, ODc5MTcyNTMxQHFxLmNvbQ==; Kai Wang, ODI3MTA4MzE3QHFxLmNvbQ==; Shao-feng Zhan, enNmc3RvbmVAMTI2LmNvbQ==
†These authors share first authorship