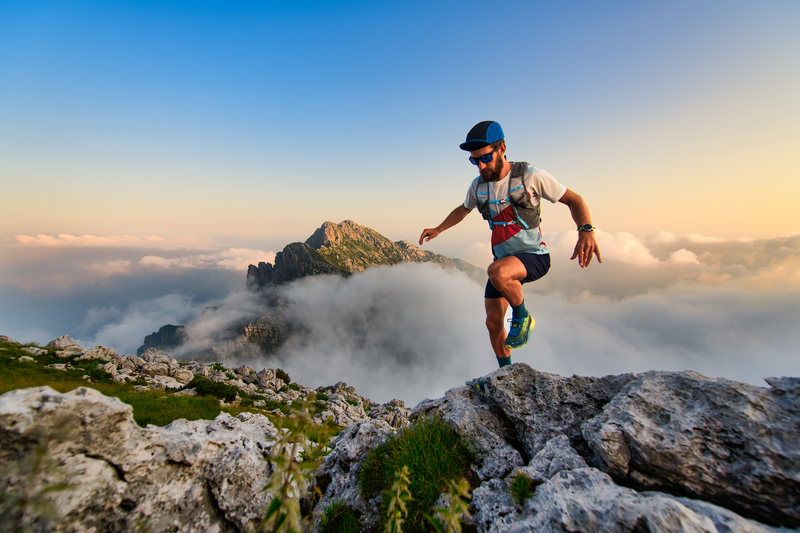
94% of researchers rate our articles as excellent or good
Learn more about the work of our research integrity team to safeguard the quality of each article we publish.
Find out more
ORIGINAL RESEARCH article
Front. Endocrinol. , 14 August 2023
Sec. Bone Research
Volume 14 - 2023 | https://doi.org/10.3389/fendo.2023.1178831
This article is part of the Research Topic The Role of the Gut Microbiota on Bone Mass in Health and Disease View all 8 articles
A commentary has been posted on this article:
Commentary: Causal effects of specific gut microbiota on bone mineral density: a two-sample Mendelian randomization study
Background: Recent studies have reported that the gut microbiota is essential for preventing and delaying the progression of osteoporosis. Nonetheless, the causal relationship between the gut microbiota and the risk of osteoporosis has not been fully revealed.
Methods: A two-sample Mendelian randomization (MR) analysis based on a large-scale genome-wide association study (GWAS) was conducted to investigate the causal relationship between the gut microbiota and bone mineral density (BMD). Instrumental variables for 211 gut microbiota taxa were obtained from the available GWAS meta-analysis (n = 18,340) conducted by the MiBioGen consortium. The summary-level data for BMD were from the Genetic Factors for Osteoporosis (GEFOS) Consortium, which involved a total of 32,735 individuals of European ancestry. The inverse variance-weighted (IVW) method was performed as a primary analysis to estimate the causal effect, and the robustness of the results was tested via sensitivity analyses by using multiple methods. Finally, a reverse MR analysis was applied to evaluate reverse causality.
Results: According to the IVW method, we found that nine, six, and eight genetically predicted gut microbiota were associated with lumbar spine (LS) BMD, forearm (FA) BMD, and femoral neck (FN) BMD, respectively. Among them, the higher genetically predicted Genus Prevotella9 level was correlated with increased LS-BMD [β = 0.125, 95% confidence interval (CI): 0.050–0.200, P = 0.001] and FA-BMD (β = 0.129, 95% CI: 0.007–0.251, P = 0.039). The higher level of genetically predicted Family Prevotellaceae was associated with increased FA-BMD (β = 0.154, 95% CI: 0.020–0.288, P = 0.025) and FN-BMD (β = 0.080, 95% CI: 0.015–0.145, P = 0.016). Consistent directional effects for all analyses were observed in both the MR-Egger and weighted median methods. Subsequently, sensitivity analyses revealed no heterogeneity, directional pleiotropy, or outliers for the causal effect of specific gut microbiota on BMD (P > 0.05). In reverse MR analysis, there was no evidence of reverse causality between LS-BMD, FA-BMD, and FN-BMD and gut microbiota (P > 0.05).
Conclusion: Genetic evidence suggested a causal relationship between the gut microbiota and BMD and identified specific bacterial taxa that regulate bone mass variation. Further exploration of the potential microbiota-related mechanisms of bone metabolism might provide new approaches for the prevention and treatment of osteoporosis.
Osteoporosis is a common metabolic osteopathy with characteristics of decreased bone mineral density (BMD), which can cause bone fragility and an increased risk of fracture (1, 2). As the population ages, approximately 200 million individuals worldwide suffer from osteoporosis. By 2050, the prevalence of osteoporosis in men and women is expected to increase by 3.1- and 2.4-fold, respectively (3, 4). Osteoporotic fracture is a serious clinical complication of osteoporosis, which often occurs in the vertebra, hip, distal forearm (FA), and pelvis. Due to its high mortality and disability rates, it has a considerable detrimental influence on patients’ quality of life (5). Moreover, the annual cost of treating osteoporosis and its related bone fractures is estimated to increase from $10 billion in 2010 to $17 billion in 2030 (6, 7). Therefore, the prevention and management of osteoporosis has been globally recognized as an important public health issue that needs to be solved urgently.
The gut microbiota is a massive complex community of microbial species inhabiting the human gastrointestinal tract, which is closely related to nutrition, immunity, inflammation, and various diseases (8, 9). On the one hand, growing evidence suggests that the gut microbiota could regulate the T regulatory cell (Treg)/T helper cell (Th)17 cell balance or relevant cytokines through the immune system, affecting the intestinal and systemic immune states, thereby establishing a dynamic balance between osteoblasts (OBs) and osteoclasts (OCs) (10). On the other hand, microbial metabolites such as saturated fatty acids (SFAs), secondary bile acid (SBA), and indole derivatives participate in the reconstruction of bone resorption, metabolism, and fracture healing by providing energy to gut epithelium cells and promoting calcium and phosphorus absorption (11). According to a study by Xu et al. (12), the gut microbiota compositions of patients with osteoporosis were significantly different from those of healthy controls, especially the enriched Dialister and Faecalibacterium genera. In addition, Ma et al. (13) also showed that transplanting intestinal flora from young rats into old rats can improve intestinal homeostasis at the phylum and family levels and increase trabecular number, trabecular thickness, and bone volume fraction, demonstrating that the gut microbiota could directly affect bone metabolism (13). However, these studies are mainly based on observational and cross-sectional analyses, and it is still not clear whether there is a causal relationship between the gut microbiome and osteoporosis.
Mendelian randomization (MR) is a groundbreaking analytical method that provides an unbiased estimation of the causal link between phenotypes (14). Compared with traditional epidemiological studies, the MR study uses genetic variation as instrumental variables (IVs) to avoid the influence of traditional confounding factors, which provides robust evidence on the mechanisms of the pathogenesis of disease and the efficacy of treatments (15, 16). Therefore, we conduct a bidirectional two-sample MR analysis to investigate the causality of specific gut microbiota and BMD and to identify specific causal bacterial taxa based on genome-wide association study (GWAS) data.
Summary-level data from published GWASs and the Genetic Factors for Osteoporosis (GEFOS) Consortium website were used in our study. Based on the bidirectional two-sample MR analysis, we evaluated the causal relationship between gut microbial genera and BMD. Our first step was to determine whether the gut microbiome contributes to the prevention or promotion of BMD by selecting the gut microbiome as the exposure and BMD as the outcome. Furthermore, we examined changes in the gut microbiota following the change in BMD. In the MR analysis, the following three assumptions should be met: 1) The instruments of genetic variations should be robustly associated with the gut microbiota; 2) The genetic variations should not be associated with any confounders of the gut microbiota and BMD nor with osteoporosis; 3) The genetic variations should affect BMD solely through the gut microbiota, not via other pathways (17).
The summary data of the gut microbiota were derived from a large-scale multi-ethnic GWAS meta-analysis, which included 18,340 European individuals and individuals from 24 cohorts (MiBioGen Consortium). Three different variable regions of the 16S rRNA gene were targeted in order to profile the microbial composition. Only the taxa found in >10% of the samples were included in the quantitative microbiome trait loci (mbQTL) mapping study for each cohort. Furthermore, after adjustment for age, sex, technical covariates, and genetic principal components, Spearman’s correlation analysis was conducted to identify genetic loci that affected the covariate-adjusted abundance of bacterial taxa (18).
The GEFOS Consortium is a large international collaboration made up of various research organizations. BMD is a highly heritable trait and is an essential index of bone strength, in which genetic determinants may explain nearly 83%, 73%, and 75% of the variance in BMD at the sites of the lumbar spine (LS), forearm (FA), and femoral neck (FN), respectively (19). Therefore, we collected the published data on BMD from the GEFOS, which identified novel loci for BMD (g/cm2) derived from Dual-energy x-ray absorptiometry (DXA) at the FN (n = 32,735), LS (n = 28,498), and FA (n = 8,143) of 53,236 European participants (20). Detailed information on the demographic characteristics of selected summary-level GWASs applied in the MR study was shown in Table 1.
1) The IVs selected for analysis are highly related to the corresponding exposures [We chose significant single nucleotide polymorphisms (SNPs) based on a loose cutoff of P < 1 × 10-5 to ensure sufficient IVs for screening]. 2) The IVs are mutually independent and avoid the offset caused by linkage disequilibrium (LD) between the SNPs (r2 < 0.001, LD distance >10,000 kb). 3) We eliminated IVs with an F-statistic <10 to minimize potential weak instrument bias F = R2 (n-k-1)/k (1-R2) (n is the sample size, k is the number of included IVs, and R2 is the exposure variance explained by the selected SNPs).
The inverse variance-weighted (IVW) method was employed as the main analysis to obtain an unbiased estimate of the causal association between the gut microbiota and BMD. Furthermore, the weighted median, MR-Egger, simple mode, and weighted mode methods were applied as additional methods to estimate causal effects under different conditions. The weighted median could combine data from multiple genetic variants into a single causal estimate, providing a consistent estimate when at least 50% of weights are from valid IVs (21). The MR-Egger method, which allows all SNPs with horizontal pleiotropic effects to be unbalanced or directed, was used to estimate the causal effect of exposure on the outcome (22). The intercept of MR-Egger regression was calculated to assess horizontal pleiotropy, and P > 0.05 indicated that the possibility of a pleiotropy effect in causal analysis is weak. Cochran’s Q test was derived from IVW estimation and used to detect heterogeneity among IVs. In addition, we applied the Mendelian randomization pleiotropy residual sum and outlier (MR-PRESSO) method to determine horizontal pleiotropy and correct potential outliers (23). The leave-one-out method was used for the sensitivity analysis, which sequentially removed one of the SNPs and used the remaining SNPs as IVs for two-sample MR analysis to judge the degree of influence of the causal association effect by a single SNP. Finally, we also performed a reverse MR analysis to determine whether there was a reverse direction causal relationship. The “TwoSampleMR” package and the “MRPRESSO” package in R software (version 4.1.3) were used for all MR analyses.
To begin with, 14,587 (locus-wide significance level, P < 1 × 10-5) and 456 (genome-wide statistical significance threshold, P < 5 × 10-8) SNPs associated with the gut microbiota were identified as IVs from the MiBioGen Consortium. After a series of quality control steps, 2,929 (P < 1 × 10-5) and 18 (P < 5 × 10-8) SNPs were finally included in the analysis. In addition, as presented in Supplementary Table S1, the F-statistics of all IVs were >10, indicating no evidence of a weak instrument bias. The results of the associations between 211 bacterial traits and LS-BMD, FA-BMD, and FN-BMD were presented in Supplementary Tables S2–S4, respectively.
According to the results of the IVW method, genetically predicted Class Erysipelotrichia [β = 0.111, 95% confidence interval (CI): 0.002–0.225, P = 0.046], Family Actinomycetaceae (β = 0.128, 95% CI: 0.032–0.225, P = 0.009), Order Actinomycetales (β = 0.129, 95% CI: 0.032–0.225, P = 0.009), Genus Barnesiella (β = 0.083, 95% CI: 0.001–0.166, P = 0.043), Genus Prevotella9 (β = 0.125, 95% CI: 0.050–0.200, P = 0.001), and Genus Sellimonas (β = 0.048, 95% CI: 0.001–0.094, P = 0.045) were positively associated with LS-BMD (Table 2), and Family Peptococcaceae (β = -0.111, 95% CI: -0.217 to -0.004, P = 0.041), Genus Eubacteriumventriosumgroup (β = -0.113, 95% CI: -0.199 to -0.027, P = 0.010), and Genus RuminococcaceaeUCG003 (β = -0.107, 95% CI: -0.198 to -0.017, P = 0.020) were negatively associated with LS-BMD (Figure 1). The MR estimates of the weighted median indicated that elevated levels of Genus Prevotella9 (β = 0.127, 95% CI: 0.022–0.232, P = 0.018) and Family Peptococcaceae (β = -0.165, 95% CI: -0.277 to -0.053, P = 0.004) were related to increased and decreased LS-BMD, respectively. Based on the Cochran’s Q test, there was no evidence of heterogeneity for the effect of specific gut microbiota on LS-BMD (P > 0.05) (Table 3). All P values of the MR-Egger intercept tests were >0.05, which indicated no horizontal pleiotropy. Furthermore, we also did not discover any outliers through the MR-PRESSO global test (Table 3). Detailed scatter plots for each MR method analysis were shown in Supplementary Figure S1. Results from the leave-one-out analysis demonstrated that no SNP was an influential outlier (Supplementary Figure S4).
Figure 1 Associations between the gut microbiota and lumbar spine bone mineral density (BMD). The forest plot contains the effects, 95% CI, and P-values of all of the examined associations in analyses. Effect = the combined effect of the exposure on the BMD; P-value = P-value of the estimate.
Table 2 MR estimates for the association between the gut microbiota and lumbar spine bone mineral density.
Table 3 Sensitivity analysis of the MR analysis results of the gut microbiota and lumbar spine bone mineral density.
The estimates of the IVW test indicated that genetically predicted Family Prevotellaceae (β = 0.154, 95% CI: 0.020–0.288, P = 0.025), Genus Eubacteriumbrachygroup (β = 0.108, 95% CI: 0.009–0.207, P = 0.033), and Genus LachnospiraceaeUCG001 (β = 0.133, 95% CI: 0.002–0.265, P = 0.046) were positively associated with FA-BMD (Table 4), and Family Rikenellaceae (β = -0.204, 95% CI: -0.335 to -0.072, P = 0.002), Genus Coprococcus3 (β = -0.208, 95% CI: -0.395 to -0.021, P = 0.029), and Genus Prevotella9 (β = 0.129, 95% CI: 0.007–0.251, P = 0.039) were negatively associated with FA-BMD (Figure 2). Futhermore, the estimates of weighted median suggested that genetically predicted Family Prevotellaceae, Family Rikenellaceae, and Genus Eubacteriumbrachygroup were causally related to FA-BMD (P < 0.05). The results of Cochran’s Q test indicated no significant heterogeneity (P > 0.05). The horizontal pleiotropy between specific gut microbiota and FA-BMD was evaluated by MR-Egger regression, showing no evidence of horizontal pleiotropy (Table 5). No outliers were discovered in the analysis of Family Prevotellaceae (P = 0.462), Genus Eubacteriumbrachygroup (P = 0.969), Genus LachnospiraceaeUCG001 (P = 0.723), Family Rikenellaceae (P = 0.546), Genus Coprococcus3 (P = 0.708), and Genus Prevotella9 (P = 0.511) by MR-PRESSO (Table 5). Detailed scatter plots of the causal relationships between the gut microbiota and FA-BMD were presented in Supplementary Figure S2. Results from the leave-one-out analysis demonstrated that no SNP was an influential outlier (Supplementary Figure S5).
Figure 2 Associations between the gut microbiota and forearm bone mineral density (BMD). The forest plot contains the effects, 95% CI, and P-values of all of the examined associations in analyses. Effect = the combined effect of the exposure on the BMD; P-value = P-value of the estimate.
Table 4 MR estimates for the association between the gut microbiota and forearm bone mineral density.
Table 5 Sensitivity analysis of the MR analysis results of the gut microbiota and forearm bone mineral density.
According to the IVW method, higher genetically predicted Class Lentisphaeria (β = 0.060, 95% CI: 0.002–0.117, P = 0.042), Family Prevotellaceae (β = 0.080, 95% CI: 0.015–0.145, P = 0.016), Order Victivallales (β = 0.060, 95% CI: 0.002–0.117, P = 0.042), and Phylum Lentisphaerae (β = 0.064, 95% CI: 0.010–0.118, P = 0.020) were linked to an increase in FN-BMD (Table 6). While Family Acidaminococcaceae (β = -0.124, 95% CI: -0.224 to -0.025, P = 0.014), Family FamilyXIII (β = -0.091, 95% CI: -0.182 to -0.001, P = 0.047), Genus Ruminococcusgauvreauiigroup (β = -0.109, 95% CI: -0.186 to -0.033, P = 0.005), and Genus Olsenella (β = -0.043, 95% CI: -0.086 to -0.000, P = 0.048) were associated with a decrease in FN-BMD (Figure 3). In addition, the Cochran’s Q test revealed no heterogeneity for the causal effect of specific gut microbiota on FN-BMD (P > 0.05). Based on the global test of MR-PRESSO and the intercept of MR-Egger, we excluded potential heterogeneity and horizontal pleiotropy in causal associations (Table 7). Detailed scatter plots of the causal correlations between the gut microbiota and FN-BMD were shown in Supplementary Figure S3. In addition, results from the leave-one-out analysis revealed that genetically predicted gut microbiota and FN-BMD remained substantially consistent after omitting one single SNP at a time (Supplementary Figure S6).
Figure 3 Associations between the gut microbiota and femoral neck bone mineral density (BMD). The forest plot contains the effects, 95% CI, and P-values of all of the examined associations in analyses. Effect = the combined effect of the exposure on the BMD; P-value = P-value of the estimate.
Table 6 MR estimates for the association between the gut microbiota and femoral neck bone mineral density.
Table 7 Sensitivity analysis of the MR analysis results of the gut microbiota and femoral neck bone mineral density.
When MR analysis was conducted with gut microbiome as a whole, IVW results indicated that higher genetically predicted gut microbiome was positively linked with LS-BMD (β = 0.087, 95% CI: 0.018–0.156, P = 0.013), FA-BMD (β = 0.100, 95% CI: 0.002–0.199, P = 0.045), and FN-BMD (β = 0.048, 95% CI: 0.001–0.095, P = 0.047) (Table 8). There was a tendency toward a protective impact of increasing genetically predicted total gut microbiome on FN-BMD in the MR-Egger and weighted median methods. Additionally, the results of Cochran’s Q statistics indicated no significant heterogeneity (P > 0.05), and MR-Egger regression demonstrated no horizontal pleiotropy between the total gut microbiome and BMD. Finally, we also did not discover any outliers through the MR-PRESSO global test (Table 9).
Table 8 MR estimates for the association between the total gut microbiota and LS-BMD, FA-BMD, and FN-BMD.
Table 9 Sensitivity analysis of the MR analysis results of the total gut microbiota and LS-BMD, FA-BMD, and FN-BMD.
The results of reverse MR analysis were shown in Supplementary Table S6. Considering cross-validation, we did not observe any reverse causal relationships between LS-BMD, FA-BMD, and FN-BMD and the gut microbiota (P > 0.05). In addition, sensitivity analyses revealed no heterogeneity, directional pleiotropy, or outliers for the causal effect of BMD on specific gut microbiota (P > 0.05).
To our knowledge, this was the first comprehensive and in-depth investigation of causal associations between the gut microbiota and BMD based on publicly available GWAS data. According to the findings of our study, a total of 21 gut microbiota were potentially causally associated with BMD and the progression of osteoporosis. A higher genetically predicted gut microbiome was positively correlated with BMD at different skeletal sites (LS-BMD, FA-BMD, and FN-BMD). These findings might provide new ideas for osteoporosis management and treatment by targeting specific gut microbiota in the future.
The gut microbiota consists of trillions of bacteria in the gastrointestinal tract, which has functions such as improving intestinal permeability, attenuating the inflammatory response, and participating in immune regulation of the skeletal system (24). In recent years, the “gut–bone” axis has been receiving increasing attention in the field of bone health and orthopedic diseases, and multiple studies indicated that the composition of the gut microbiota regulates bone metabolism through multiple pathways (25). An experimental animal study demonstrated that Erysipelotrichia, Enterobacteriales, Actinomycetales, and Ruminococcus were significantly associated with serum biomarkers related to bone metabolism, such as serum bone Gla-protein (BGP), osteoprotegerin (OPG), and tartrate-resistant acid phosphatase (TRACP), which have beneficial effects on improving bone microarchitecture (26). In contrast, Huang et al. (27) observed that the relative abundance of Ruminococcus was higher in osteoporosis patients compared with that in healthy patients. Similarly, another animal study found that Ruminococcus in ovariectomized (OVX) rats was significantly increased compared with that in the sham group and was positively associated with bone loss (28). Based on the findings reviewed above, we speculated that the different effects of Ruminococcus on osteoporosis may be species- and strain-specific, which warrants further investigation.
In addition, Nogal et al. (29) reported a close correlation between acetate and Barnesiella, and that acetate was a health-promoting molecule with bone and gut protective effects that enhances immunity and inhibits intestinal inflammation and OC differentiation, thereby regulating bone metabolism. According to some literature, LachnospiraceaeUCG001 belonged to the Lachnospiraceae family of bacteria, and it might play an anti-inflammatory role by controlling metabolite (such as short-chain fatty acids, SBA, indole derivatives, and polyamines) production (30). Moreover, we also found that some innovative gut bacteria, including Lentisphaeria, Victivallales, and Lentisphaerae, were associated with the levels of BMD. However, the exact mechanism of their effect on osteoporosis needs further study.
Our MR analysis also found that the abundance of Prevotella9 and Prevotellaceae was associated with high BMD at different sites. Consistent directional effects for all analyses were observed in both MR-Egger and weighted median methods, which suggests that Prevotella might be a promising target for osteoporosis prevention. Prevotella was a commensal bacterium that widely exists in the human gut, oral, and reproductive tracts (31). In a study by Wang et al. (32), altered richness and a decreased proportion of Prevotella in the postmenopausal osteoporosis (PMO) group relative to the normal bone mass group and transplantation of Prevotella into OVX mice were effective at slowing bone loss. Furthermore, several mechanism studies have indicated that Prevotella reduces the intestinal permeability of estrogen-deficient mice by upregulating the expression of tight junction proteins including zonula occludens-1 (ZO-1) and occludin, which further prevents the release of inflammatory cytokines. Together, these effects inhibited osteoclastic bone resorption and prevented bone loss through the “gut–bone” axis (33).
Our study based on genetic prediction found that there were causal relationships between several gut microbial taxa and the decline of BMD, some of which have been confirmed in previous observational studies. Wei et al. (34) demonstrated that the absolute and relative abundances of Clostridium_XLVa, Coprococcus, and Lactobacillus were higher and the abundance of Veillonella genus was lower in osteoporosis patients compared with those in the controls. According to the results of another study, the abundance of Eubacterium ventriosum group, Ruminococcus_1, Family_XIII, and Coprococcus was negatively linked with the risk of rheumatoid arthritis, suggesting that these specific gut microbiota can increase matrix metallo-proteinase (MMP)-1, MMP-3, and MMP-13 expression, as well as OC activity to aggravate cartilage and bone damage (35). According to a case-control study, the level of Family Rikenellaceae was higher in the low BMD group and Rikenellaceae might have an adverse impact on bone resorption and bone density (36). Similarly, compared with the OVX group, the anti-osteoporosis treatment group increased the abundances of Lactobacillus_reuteri, Muribaculaceae, and Clostridia that were reported to increase bone mass and inhibited the relative abundance of Rikenellaceae (37).
Interestingly, when MR analysis was performed with the gut microbiome as a whole, the results of IVW indicated that a higher genetically predicted gut microbiome was positively linked with LS-BMD, FA-BMD, and FN-BMD. The gut microbiota was usually divided into beneficial conditional pathogen and pathogenic bacteria, and the balance between beneficial and pathogenic flora was critical for homeostasis and preventing bone metabolic diseases (38). He et al. (39) tested the gut microbiota of 106 postmenopausal women and found that the richness of the bacterial community was significantly lower in the osteoporosis group than that in the normal bone mass and osteopenia groups. Among them, Enterobacter, Citrobacter, Pseudomonas, and Desulfovibrio were enriched in postmenopausal osteopenia, while the osteoporosis group was more abundant in Parabacteroides, Lactobacillus, and Actinomycetales. Similarly, we found that the gut microbiota causally associated with BMD were mostly beneficial flora, suggesting that regulating the balance of the overall gut microbiota composition may be beneficial for improving bone health and reducing the risk of osteoporosis. In recent years, multiple studies have been published indicating that probiotics can be involved in the regulation of bone metabolism by mediating the production of immune inflammatory factors, improving intestinal barrier permeability, and regulating the metabolism of short-chain fatty acids (40–42). Li et al. (43) confirmed through animal experiments that supplementation of exogenous probiotics could reduce the serum concentration of tumor necrosis factor-á (TNF-á), receptor activator of NF-kB ligand (RANKL), interleukin 17A (IL-17A) in estrogen-deficient mice, attenuating systemic inflammatory responses, maintaining intestinal and whole body immune system balance, and slowing bone loss. In addition, a randomized double-blind controlled trial reported that multispecies probiotic supplementation for 6 months significantly reduced BMD loss caused by estrogen deficiency and improved bone turnover in postmenopausal women with osteopenia (44).
Our study had several strengths. First of all, we used MR analysis to infer that the correlation between the gut microbiome and BMD should be less susceptible to confounding and reverse causation than traditional observational analyses. Additionally, we analyzed the causal effect of each taxon on BMD from the genus to the phylum level, which provides guidance for the prevention and treatment of osteoporosis by targeting specific gut microbiota in clinical practice. However, the present study also had some limitations that should be noted. Firstly, due to the lack of demographic data in the original study (e.g., gender, age, and race), we were unable to perform further subgroup analyses to obtain more specific effect relationships. Secondly, the GWAS data on the gut microbiota used in this study are based on the population cohort from the largest macrogenome sequencing study to date. In the future, summary data of other gut microbiota need to be obtained for a more comprehensive assessment of the causal relationship between gut microbes and osteoporosis risk. Last but not least, this study was confined to individuals of European origin, and other populations require further MR studies, as causal relationships may vary from race to race.
In summary, we evaluated the causal relationship between the gut microbiota and BMD and identified potentially causal bacterial taxa that may be responsible for osteoporosis. However, further prospective cohort studies and mechanistic studies are needed to confirm these findings.
Ethical approval and informed consent for studies included in the analyses was provided in the original publications.
The original contributions presented in the study are included in the article/Supplementary Material. Further inquiries can be directed to the corresponding author.
SC collected data. HH and JJ organized the study and performed the statistical analysis. GZ and ZL drafted the manuscript, to which all authors contributed, and approved the final version for publication. All authors contributed to the article and approved the submitted version.
This work was supported by the Elderly Health Research Project of Jiangsu Commission of Health (LKZ2022008), the Natural Science Foundation of Nanjing University Of Chinese Medicine (XZR2021060), and the Foundation of The Second Affiliated Hospital of Nanjing University of Chinese Medicine (SEZ202003).
We are very grateful to Genetic Factors for Osteoporosis Consortium (GEFOS) and MiBioGen Consortium for their selfless public sharing of GWAS summary data, which provides us with great convenience to carry out this research.
We declare that the research was conducted in the absence of any commercial or financial relationships that could be construed as a potential conflict of interest.
All claims expressed in this article are solely those of the authors and do not necessarily represent those of their affiliated organizations, or those of the publisher, the editors and the reviewers. Any product that may be evaluated in this article, or claim that may be made by its manufacturer, is not guaranteed or endorsed by the publisher.
The Supplementary Material for this article can be found online at: https://www.frontiersin.org/articles/10.3389/fendo.2023.1178831/full#supplementary-material
1. Kanis JA, Cooper C, Rizzoli R, Reginster JY. European Guidance for the diagnosis and management of osteoporosis in postmenopausal women. Osteoporos Int (2019) 30:3–44. doi: 10.1007/s00198-018-4704-5
2. Kirk B, Zanker J, Duque G. Osteosarcopenia: epidemiology, diagnosis, and treatment-facts and numbers. J Cachexia Sarcopenia Muscle (2020) 11:609–18. doi: 10.1002/jcsm.12567
3. Johnell O, Kanis JA. An estimate of the worldwide prevalence and disability associated with osteoporotic fractures. Osteoporos Int (2006) 17:1726–33. doi: 10.1007/s00198-006-0172-4
4. Gullberg B, Johnell O, Kanis JA. World-wide projections for hip fracture. Osteoporos Int (1997) 7:407–13. doi: 10.1007/pl00004148
5. Burge R, Dawson-Hughes B, Solomon DH, Wong JB, King A, Tosteson A. Incidence and economic burden of osteoporosis-related fractures in the united states, 2005-2025. J Bone Miner Res (2007) 22:465–75. doi: 10.1359/jbmr.061113
6. Tanha K, Fahimfar N, Nematollahi S, Sajjadi-Jazi SM, Gharibzadeh S, Sanjari M, et al. Annual incidence of osteoporotic hip fractures in Iran: a systematic review and meta-analysis. BMC Geriatr (2021) 21:668. doi: 10.1186/s12877-021-02603-1
7. Williams SA, Daigle SG, Weiss R, Wang Y, Arora T, Curtis JR. Economic burden of osteoporosis-related fractures in the US Medicare population. Ann Pharmacother (2021) 55:821–29. doi: 10.1177/1060028020970518
8. Qin J, Li R, Raes J, Arumugam M, Burgdorf KS, Manichanh C, et al. A human gut microbial gene catalogue established by metagenomic sequencing. Nature (2010) 464:59–65. doi: 10.1038/nature08821
9. Li C, Liu C, Li N. Causal associations between gut microbiota and adverse pregnancy outcomes: a two-sample mendelian randomization study. Front Microbiol (2022) 13:1059281. doi: 10.3389/fmicb.2022.1059281
10. Locantore P, Del GV, Gelli S, Paragliola RM, Pontecorvi A. The interplay between immune system and microbiota in osteoporosis. Mediators Inflammation (2020) 2020:3686749. doi: 10.1155/2020/3686749
11. Tu Y, Yang R, Xu X, Zhou X. The microbiota-gut-bone axis and bone health. J Leukoc Biol (2021) 110:525–37. doi: 10.1002/JLB.3MR0321-755R
12. Xu Z, Xie Z, Sun J, Huang S, Chen Y, Li C, et al. Gut microbiome reveals specific dysbiosis in primary osteoporosis. Front Cell Infect Microbiol (2020) 10:160. doi: 10.3389/fcimb.2020.00160
13. Ma S, Wang N, Zhang P, Wu W, Fu L. Fecal microbiota transplantation mitigates bone loss by improving gut microbiome composition and gut barrier function in aged rats. Peerj (2021) 9:e12293. doi: 10.7717/peerj.12293
14. Burgess S, Timpson NJ, Ebrahim S, Davey SG. Mendelian randomization: where are we now and where are we going? Int J Epidemiol (2015) 44:379–88. doi: 10.1093/ije/dyv108
15. Smith GD, Ebrahim S. 'Mendelian randomization': can genetic epidemiology contribute to understanding environmental determinants of disease? Int J Epidemiol (2003) 32:1–22. doi: 10.1093/ije/dyg070
16. Davey SG, Hemani G. Mendelian randomization: genetic anchors for causal inference in epidemiological studies. Hum Mol Genet (2014) 23:R89–98. doi: 10.1093/hmg/ddu328
17. Sanderson E, Davey SG, Windmeijer F, Bowden J. An examination of multivariable mendelian randomization in the single-sample and two-sample summary data settings. Int J Epidemiol (2019) 48:713–27. doi: 10.1093/ije/dyy262
18. Kurilshikov A, Medina-Gomez C, Bacigalupe R, Radjabzadeh D, Wang J, Demirkan A, et al. Large-Scale association analyses identify host factors influencing human gut microbiome composition. Nat Genet (2021) 53:156–65. doi: 10.1038/s41588-020-00763-1
19. Machiela MJ, Chanock SJ. LDlink: a web-based application for exploring population-specific haplotype structure and linking correlated alleles of possible functional variants. Bioinformatics (2015) 31:3555–57. doi: 10.1093/bioinformatics/btv402
20. Zheng HF, Forgetta V, Hsu YH, Estrada K, Rosello-Diez A, Leo PJ, et al. Whole-genome sequencing identifies EN1 as a determinant of bone density and fracture. Nature (2015) 526:112–17. doi: 10.1038/nature14878
21. Bowden J, Davey SG, Haycock PC, Burgess S. Consistent estimation in mendelian randomization with some invalid instruments using a weighted median estimator. Genet Epidemiol (2016) 40:304–14. doi: 10.1002/gepi.21965
22. Bowden J, Davey SG, Burgess S. Mendelian randomization with invalid instruments: effect estimation and bias detection through egger regression. Int J Epidemiol (2015) 44:512–25. doi: 10.1093/ije/dyv080
23. Verbanck M, Chen CY, Neale B, Do R. Detection of widespread horizontal pleiotropy in causal relationships inferred from mendelian randomization between complex traits and diseases. Nat Genet (2018) 50:693–98. doi: 10.1038/s41588-018-0099-7
24. Rizzoli R. Nutritional influence on bone: role of gut microbiota. Aging Clin Exp Res (2019) 31:743–51. doi: 10.1007/s40520-019-01131-8
25. Zhang YW, Li YJ, Lu PP, Dai GC, Chen XX, Rui YF. The modulatory effect and implication of gut microbiota on osteoporosis: from the perspective of "brain-gut-bone" axis. Food Funct (2021) 12:5703–18. doi: 10.1039/d0fo03468a
26. Wang S, Wang S, Wang X, Xu Y, Zhang X, Han Y, et al. Effects of icariin on modulating gut microbiota and regulating metabolite alterations to prevent bone loss in ovariectomized rat model. Front Endocrinol (Lausanne) (2022) 13:874849. doi: 10.3389/fendo.2022.874849
27. Huang R, Liu P, Bai Y, Huang J, Pan R, Li H, et al. Changes in the gut microbiota of osteoporosis patients based on 16S rRNA gene sequencing: a systematic review and meta-analysis. J Zhejiang Univ Sci B (2022) 23:1002–13. doi: 10.1631/jzus.B2200344
28. Ma S, Qin J, Hao Y, Shi Y, Fu L. Structural and functional changes of gut microbiota in ovariectomized rats and their correlations with altered bone mass. Aging (Albany NY) (2020) 12:10736–53. doi: 10.18632/aging.103290
29. Nogal A, Louca P, Zhang X, Wells PM, Steves CJ, Spector TD, et al. Circulating levels of the short-chain fatty acid acetate mediate the effect of the gut microbiome on visceral fat. Front Microbiol (2021) 12:711359. doi: 10.3389/fmicb.2021.711359
30. Wang N, Meng F, Ma S, Fu L. Species-level gut microbiota analysis in ovariectomized osteoporotic rats by shallow shotgun sequencing. Gene (2022) 817:146205. doi: 10.1016/j.gene.2022.146205
31. Maeda Y, Kurakawa T, Umemoto E, Motooka D, Ito Y, Gotoh K, et al. Dysbiosis contributes to arthritis development via activation of autoreactive T cells in the intestine. Arthritis Rheumatol (2016) 68:2646–61. doi: 10.1002/art.39783
32. Wang Z, Chen K, Wu C, Chen J, Pan H, Liu Y, et al. An emerging role of prevotella histicola on estrogen deficiency-induced bone loss through the gut microbiota-bone axis in postmenopausal women and in ovariectomized mice. Am J Clin Nutr (2021) 114:1304–13. doi: 10.1093/ajcn/nqab194
33. Ulluwishewa D, Anderson RC, McNabb WC, Moughan PJ, Wells JM, Roy NC. Regulation of tight junction permeability by intestinal bacteria and dietary components. J Nutr (2011) 141:769–76. doi: 10.3945/jn.110.135657
34. Wei M, Li C, Dai Y, Zhou H, Cui Y, Zeng Y, et al. High-throughput absolute quantification sequencing revealed osteoporosis-related gut microbiota alterations in han Chinese elderly. Front Cell Infect Microbiol (2021) 11:630372. doi: 10.3389/fcimb.2021.630372
35. Li Y, Liu C, Luo J, Zeng Y, Meng X, Wang S, et al. Ershiwuwei lvxue pill alleviates rheumatoid arthritis by different pathways and produces changes in the gut microbiota. Phytomedicine (2022) 107:154462. doi: 10.1016/j.phymed.2022.154462
36. Ozaki D, Kubota R, Maeno T, Abdelhakim M, Hitosugi N. Association between gut microbiota, bone metabolism, and fracture risk in postmenopausal Japanese women. Osteoporos Int (2021) 32:145–56. doi: 10.1007/s00198-020-05728-y
37. Zhou J, Wang R, Zhao R, Guo X, Gou P, Bai H, et al. Intermittent parathyroid hormone alters gut microbiota in ovariectomized osteoporotic rats. Orthop Surg (2022) 14:2330–38. doi: 10.1111/os.13419
38. Shin NR, Whon TW, Bae JW. Proteobacteria: microbial signature of dysbiosis in gut microbiota. Trends Biotechnol (2015) 33:496–503. doi: 10.1016/j.tibtech.2015.06.011
39. He J, Xu S, Zhang B, Xiao C, Chen Z, Si F, et al. Gut microbiota and metabolite alterations associated with reduced bone mineral density or bone metabolic indexes in postmenopausal osteoporosis. Aging (Albany NY) (2020) 12:8583–604. doi: 10.18632/aging.103168
40. Iqbal J, Yuen T, Zaidi M. Getting warmer: following one's gut to build bone. Cell Metab (2020) 32:504–06. doi: 10.1016/j.cmet.2020.09.010
41. Collins FL, Rios-Arce ND, Schepper JD, Parameswaran N, McCabe LR. The potential of probiotics as a therapy for osteoporosis. Microbiol Spectr (2017) 5. doi: 10.1128/microbiolspec.BAD-0015-2016
42. Li J, Ho W, Liu C, Chow SK, Ip M, Yu J, et al. The role of gut microbiota in bone homeostasis. Bone Joint Res (2021) 10:51–9. doi: 10.1302/2046-3758.101.BJR-2020-0273.R1
43. Li JY, Chassaing B, Tyagi AM, Vaccaro C, Luo T, Adams J, et al. Sex steroid deficiency-associated bone loss is microbiota dependent and prevented by probiotics. J Clin Invest (2016) 126:2049–63. doi: 10.1172/JCI86062
44. Lambert M, Thybo CB, Lykkeboe S, Rasmussen LM, Frette X, Christensen LP, et al. Combined bioavailable isoflavones and probiotics improve bone status and estrogen metabolism in postmenopausal osteopenic women: a randomized controlled trial. Am J Clin Nutr (2017) 106:909–20. doi: 10.3945/ajcn.117.153353
Keywords: osteoporosis, gut microbiota, bone mineral density, Mendelian randomization, causality
Citation: Chen S, Zhou G, Han H, Jin J and Li Z (2023) Causal effects of specific gut microbiota on bone mineral density: a two-sample Mendelian randomization study. Front. Endocrinol. 14:1178831. doi: 10.3389/fendo.2023.1178831
Received: 03 March 2023; Accepted: 13 April 2023;
Published: 14 August 2023.
Edited by:
Sadiq Umar, University of Illinois Chicago, United StatesReviewed by:
Mohammad Ashafaq, Jazan University, Saudi ArabiaCopyright © 2023 Chen, Zhou, Han, Jin and Li. This is an open-access article distributed under the terms of the Creative Commons Attribution License (CC BY). The use, distribution or reproduction in other forums is permitted, provided the original author(s) and the copyright owner(s) are credited and that the original publication in this journal is cited, in accordance with accepted academic practice. No use, distribution or reproduction is permitted which does not comply with these terms.
*Correspondence: Zhiwei Li, MDM5MjE2MjM1QG5qdWNtLmVkdS5jbg==
†These authors have contributed equally to this work
Disclaimer: All claims expressed in this article are solely those of the authors and do not necessarily represent those of their affiliated organizations, or those of the publisher, the editors and the reviewers. Any product that may be evaluated in this article or claim that may be made by its manufacturer is not guaranteed or endorsed by the publisher.
Research integrity at Frontiers
Learn more about the work of our research integrity team to safeguard the quality of each article we publish.