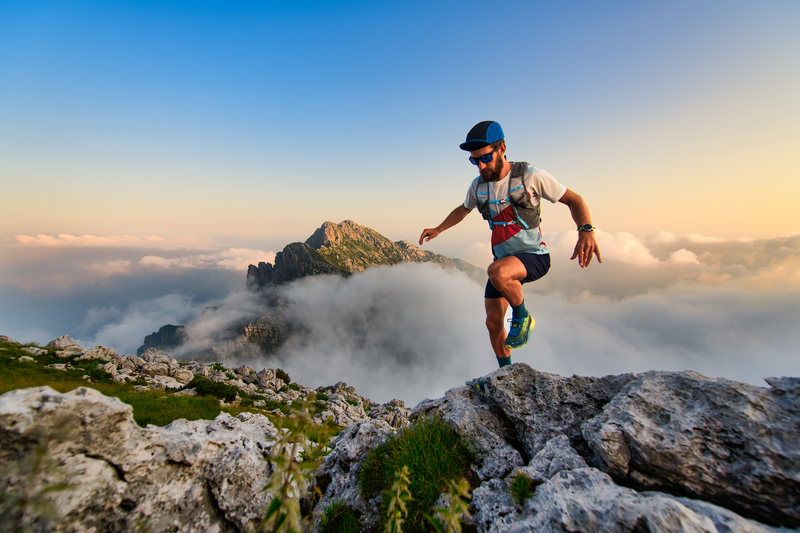
95% of researchers rate our articles as excellent or good
Learn more about the work of our research integrity team to safeguard the quality of each article we publish.
Find out more
ORIGINAL RESEARCH article
Front. Endocrinol. , 21 July 2023
Sec. Reproduction
Volume 14 - 2023 | https://doi.org/10.3389/fendo.2023.1170957
This article is part of the Research Topic Epigenetics of Polycystic Ovary Syndrome View all 7 articles
Background: Polycystic ovary syndrome (PCOS) is a complex, multifactor disorder in women of reproductive age worldwide. Although RNA editing may contribute to a variety of diseases, its role in PCOS remains unclear.
Methods: A discovery RNA-Seq dataset was obtained from the NCBI Gene Expression Omnibus database of granulosa cells from women with PCOS and women without PCOS (controls). A validation RNA-Seq dataset downloaded from the European Nucleotide Archive Databank was used to validate differential editing. Transcriptome-wide investigation was conducted to analyze adenosine-to-inosine (A-to-I) RNA editing in PCOS and control samples.
Results: A total of 17,395 high-confidence A-to-I RNA editing sites were identified in 3,644 genes in all GC samples. As for differential RNA editing, there were 545 differential RNA editing (DRE) sites in 259 genes with Nucleoporin 43 (NUP43), Retinoblastoma Binding Protein 4 (RBBP4), and leckstrin homology-like domain family A member 1 (PHLDA) showing the most significant three 3′-untranslated region (3′UTR) editing. Furthermore, we identified 20 DRE sites that demonstrated a significant correlation between editing levels and gene expression levels. Notably, MIR193b-365a Host Gene (MIR193BHG) and Hook Microtubule Tethering Protein 3 (HOOK3) exhibited significant differential expression between PCOS and controls. Functional enrichment analysis showed that these 259 differentially edited genes were mainly related to apoptosis and necroptosis pathways. RNA binding protein (RBP) analysis revealed that RNA Binding Motif Protein 45 (RBM45) was predicted as the most frequent RBP binding with RNA editing sites. Additionally, we observed a correlation between editing levels of differential editing sites and the expression level of the RNA editing enzyme Adenosine Deaminase RNA Specific B1 (ADARB1). Moreover, the existence of 55 common differentially edited genes and nine differential editing sites were confirmed in the validation dataset.
Conclusion: Our current study highlighted the potential role of RNA editing in the pathophysiology of PCOS as an epigenetic process. These findings could provide valuable insights into the development of more targeted and effective treatment options for PCOS.
RNA editing is an epigenetic alteration of the RNA nucleotide sequence with nucleotide insertions, deletions, or substitutions (1). In mammals, canonical RNA editing includes adenosine-to-inosine (A-to-I) editing and cytidine-to-uridine (C-to-U) editing (2). Altered A-to-I editing has been implicated in various diseases, including autoimmune disorders, cardiovascular diseases, neurological diseases, and cancers, suggesting its involvement in the molecular mechanisms of these pathological processes (3–6).
Polycystic ovary syndrome (PCOS) is a complex multigenic disorder characterized by excessive androgen levels and ovarian dysfunction (7). It is the most common endocrine-metabolic disorder in women of reproductive age in the world (8). Granulosa cells (GCs) play a crucial role in the pathogenesis of PCOS. Studies have demonstrated that atrial natriuretic peptides can inhibit GC apoptosis to modulate ovarian function in PCOS (9). Furthermore, emerging studies show epigenetic modifications and altered gene expression patterns in GCs from PCOS women and mouse models, suggesting a potential contribution of epigenetic mechanisms to PCOS development (10, 11). However, understanding A-to-I RNA editing in the context of PCOS remains limited. Further investigation is needed to elucidate the role of A-to-I RNA editing in PCOS development and progression.
To identify A-to-I RNA editing associated with PCOS, we performed a transcriptome-wide analysis of RNA-Seq data from ovarian GCs and validated the findings using a cross-cohort approach. Our findings revealed dramatic A-to-I RNA editing alterations in PCOS compared to controls and underlined their substantial role in the epigenetic regulation of PCOS.
We searched the OmicsDI database and found two RNA-Seq datasets of GCs from PCOS and control women. The dataset GSE138518 retrieved from the NCBI Gene Expression Omnibus (GEO, http://www.ncbi.nlm.nih.gov/geo) database was used for RNA editing event discovery and consisted of GCs from adult women, including five with PCOS and six controls without PCOS undergoing in vitro fertilization treatments (IVF) (12). In addition, the validation dataset PRJNA762274 was retrieved from the European Nucleotide Archive Databank (https://www.ebi.ac.uk/), which contained GCs from four PCOS patients and four controls undergoing IVF or intracytoplasmic sperm injection (13). The processes of RNA extraction, library construction, and RNA sequencing were described in the original studies.
After the raw sequencing data were retrieved, FASTQC was first used to analyze the raw data for quality control. Adaptor and low-quality sequences were removed using FASTP Version 0.23.4 (14). RNA STAR (Version 2.7.0e) was used to map sequencing reads to the human reference genome (UCSC hg38) and generate alignment files in Binary Alignment Map (BAM) format (15). The BAM files were processed using SamTools (Version 1.9) to remove optic duplications and retain only reads uniquely mapped to the human reference genome (16). GATK (Version 4.1.3) was used to recalibrate the base quality scores of the BAM files by following the instructions provided in the GATK best practice guidance (17).
Variant calling was then conducted to identify candidates for RNA editing events. Single-nucleotide variation (SNV) was called from the BAM files by using VarScan (Version 2.4.3) as described in our previous study (18). The variant calling criteria were defined as follows: a minimum base quality of 25, a total sequencing depth of at least 10, an alternative allele depth of 2 or more, and an alternative allele frequency (AAF) of 1% or higher. To eliminate potential false-positive SNVs, VarScan was employed with its default parameters to filter and remove them. The SNVs were then annotated using the Ensembl Variant Effect Predictor (VEP) (19). SNVs were further filtered and removed according to the criteria as follows unless annotated as RNA editing sites in the REDIportal V2.0 database: (1) located in homopolymer runs ≥ five nucleotides (nt), simple repeats, or the mitochondria, (2) within six nt from splice junctions, one nt from insertions or deletions, or 4% to the ends of reads; (3) annotated in the dbSNP database Build 142; (4) more than 90% of the samples had an AAF equal to 100% or between 40% and 60% (20–22). The remaining high-confidence A-to-I (recognized as A-to-G transition in the RNA-Seq reads) RNA editing events were kept if the editing levels were observed ≥ 1% in at least two samples (20, 21).
To quantify the gene expression levels, FeatureCounts Version 2.0.1 was used to calculate the pseudo-counts of gene expression from the BAM files, and EdgeR (Version 3.7) was then used to calculate the value of transcripts read per thousand bases per million mappings (TPM) for each gene (23, 24).
To evaluate how RNA editing could contribute to the difference between PCOS and controls, the principal component analysis (PCA) of A-to-I RNA editing events was performed using the function prcomp of R (Version 3.6.3) and visualized using the ggplot2 (Version 2.2.1) package.
To understand the possible functional relevance of RNA editing, gene ontology (GO) and Kyoto Encyclopedia of Genes and Genomes (KEGG) pathways of edited genes were analyzed using Enrichr (25). Items with enrichment p < 0.05 were considered significant and visualized using the online tools provided at the website (http://www.bioinformatics.com.cn/).
To evaluate the potential functional impact of RNA editing, the RNAfold web server (http://rna.tbi.univie.ac.at/cgi-bin/RNAWebSuite/RNAfold.cgi) was used to predict secondary structures of single-stranded RNA sequences surrounding RNA editing sites (26, 27). To further understand the potential functional impact of RNA editing, RBPmap (http://rbpmap.technion.ac.il) was used to predict the binding of RNA binding protein (RBP) to differential RNA editing sites (28). The results of RBP binding prediction were visualized using the wordcloud package (Version 2.6).
To identify differential RNA editing, the intergroup RNA editing levels were compared using the generalized linear model (GLM), and the likelihood ratio test (LRT) was used. The t-test was used to compare the gene expression intergroup levels. p < 0.05 was used as the significance cutoff. The correlation between RNA editing and gene expression was analyzed using the Spearman’s correlation method to calculate the correlation coefficient (r) and p-value.
Our analysis identified 17,395 high-confidence A-to-I RNA editing events in 3,644 genes in GCs (Figure 1A). The SNV density across various chromosomes is shown in Supplementary Figure S1. Regarding the genomic distribution of the identified editing sites, the majority (56.5%) were annotated as intronic variants, while 24.5% were located in the 3′-untranslated regions (3′UTR). The remaining variants were distributed across other regions (Figure 1B). Notably, 65.5% of all editing sites were located in Alu repetitive elements (Figure 1C). Sorting intolerant from tolerant (SIFT) algorithm was utilized to predict the functional impact of missense variants, revealing that 50.9% of the missense variants were predicted to be deleterious (including both deleterious and low confidence deleterious variants) and might potentially affect the encoded protein (Figure 1D; Supplementary Table S1).
Figure 1 A-to-I RNA editing events in GC. (A) Circos plot of gene expression and A-to-I RNA editing sites in PCOS and controls. Outer circle: average level of gene expression. Inner circle: A-to-I RNA editing sites. (B) A-to-I RNA editing functional categories. (C) repetitive elements overlapped with RNA editing sites. (D) SIFT prediction of that missense variants. P, PCOS; C, controls.
We then conducted a motif analysis of the sequence between 6 bp upstream and downstream of the editing sites. Our findings indicated that in most categories, G was suppressed 1 bp upstream of the editing sites (Supplementary Figure S2).
By comparing the RNA editing between PCOS and control GCs, a lower number of editing sites and genes unique to PCOS was observed. In total, 428 genes were uniquely edited in controls, whereas 76 genes were uniquely edited in PCOS. Likewise, 7,464 editing sites were unique to controls, whereas 312 were unique to PCOS (Figures 2A, B).
Figure 2 Differential A-to-I RNA editing sites in PCOS and controls. (A, B) Venn plots of A-to-I RNA editing events in PCOS and controls. (C) Manhattan plot of differential RNA editing sites across the chromosomes. (D) Types of mRNA variants resulted from 545 differential A-to-I RNA editing events. (E–G) boxplots of the top 3 3′UTR editing sites (NUP43:chr6:149725375, RBBP4:chr1:32680141, and PHLDA1:chr12:76027474). (H–J) Boxplots of the top three intronic editing sites (NR5A2:chr1:200074554, CELF1:chr11:47481265, and FLNB:chr3:58166381). (K, L) Boxplots of synonymous editing sites (TUBA1B:chr12:49128795 and MX1:chr21:41452734). Blue and red represent the groups of PCOS and control, respectively. P, PCOS; C, controls.
In the GLM and LRT results, 545 sites in 259 genes exhibited differential A-to-I RNA editing between PCOS and control GCs (Figure 2C; Supplementary Table S2). These differential RNA editing (DRE) sites included various consequence types, with the majority (66.24%) located in the 3′UTR and a significant portion (24.95%) in intronic regions (Figure 2D). The most significant 3′UTR variants were found in Nucleoporin 43 (NUP43), Retinoblastoma Binding Protein 4 (RBBP4), and leckstrin homology-like domain family A member 1 (PHLDA), including NUP43:chr6:149725375, RBBP4:chr1:32680141, and PHLDA1:chr12:76027474 (Figures 2E–G). The top three intronic variants were found in Nuclear Receptor Subfamily 5 Group A Member 2 (NR5A2), CUGBP Elav-Like Family Member 1 (CELF), and Filamin B, (FLNB), including NR5A2:chr1:200074554, CELF1:chr11:47481265, and FLNB:chr3:58166381 (Figures 2H–J). In addition, we also identified two synonymous variant sites in Tubulin Alpha 1b (TUBA1B), and MX Dynamin Like GTPase 1 (MX1), including TUBA1B:chr12:49128795 and MX1:chr21:41452734 (Figures 2K, L).
Additionally, we performed PCA using these differential RNA editing sites. The results showed that PC1 and PC2 accounted for 73.28% and 13.03% of the total variance, respectively (Supplementary Figure S3).
We then conducted the correlation to explore the relationship between RNA editing and gene expression levels. Our findings revealed significant correlations between editing sites and gene expression levels for 20 DRE sites (p < 0.05). Among these sites, eight showed a positive correlation, while the remaining 12 exhibited a negative correlation. Noteworthy, the top three editing sites with positive correlations were found in Hook Microtubule Tethering Protein 3 (HOOK3), Rhomboid Domain Containing 2 (RHBDD2), and Transmembrane Protein 16 (TMEM165), including HOOK3:chr8:43021004 (r = 0.79), RHBDD2:chr7:75883398 (r = 0.8), and TMEM165:chr4:55412273 (r = 0.84) (Figures 3A–C).
Figure 3 Differential editing sites with cis-regulatory effects on gene expression. (A–F) Scatter plots showing their RNA editing and gene expression levels in PCOS and controls. (G, H) The RNA editing and expression levels of MIR193BHG and HOOK3. (I, J) The RNA structure and stability parameters before and after RNA editing for HOOK3:chr8:43021004 and (K, L) MIR193BHG: chr16:14330019. P, PCOS; C, controls; MFE, minimum free energy; FE, free energy of the thermodynamic ensemble; Freq MFE, frequency of the MFE structure in the ensemble; ED, ensemble diversity.
On the other hand, sites in FLNB, Hyccin PI4KA Lipid Kinase Complex Subunit 2 (HYCC2, also called FAM126B), and MIR193BHG, including, FLNB:chr3:58166381 (r = −0.89), FAM126B:chr2:200977846 (r = −0.84), and MIR193BHG:chr16:14330019 (r = −0.78) showed the top three significant negative correlations with gene expression (Figures 3D–F). The editing levels of these 20 sites are shown in Supplementary Figure S4.
Furthermore, we examined the expression levels of genes containing the identified editing sites and observed significantly lower expression levels of MIR193BHG and HOOK3 in PCOS compared to those in controls (Figures 3G, H). We then performed prediction analysis to investigate whether RNA editing changed the stability of the mRNA secondary structures of HOOK3 and MIR193BHG before and after editing. The detailed information of the two editing sites (HOOK3:chr8:43021004 and MIR193BHG:chr16:14330019) including the minimum free energy (MFE), the free energy of the thermodynamic ensemble (FE), the frequency of the MFE structure in the ensemble, and ensemble diversity is presented in Figures 3I–L.
We further assessed the expression of editing enzymes, including Adenosine Deaminase RNA Specific (ADAR) and Adenosine Deaminase RNA Specific B1 (ADARB1). We observed a significant decrease in ADARB1 expression in PCOS compared to controls (Figure 4A). Therefore, we further looked into the correlation between ADARB1 expression and PCOS-associated RNA editing and identified six ADARB1-related sites in Scavenger Receptor Class B Member 2 (SCARB2), Charged Multivesicular Body Protein 3 (CHMP3), Ubiquitin Specific Peptidase 22 (USP22), FAM126B, Solute Carrier Family 47 Member 1 (SLC47A1), and Coiled-Coil Domain Containing 69 (CCDC69), including SCARB2:chr4:76159678, CHMP3:chr2:86504167, USP22:chr17:21000918, FAM126B:chr2:200978748, FAM126B:chr2:200978747, SLC47A1:chr17:19578434, and CCDC69:chr5:151181355, all with significantly downregulated RNA editing correlated to the decreased ADARB1 expression (Figures 4B–I).
Figure 4 The correlation between RNA editing enzyme expression and differential editing sites. (A) The correlation between ADARB1 expression and differential A-to-I RNA editing sites. (B) The expression level of ADARB1. (C–I) The editing level of ADARB1-related DRE sites. P, PCOS; C, controls.
We performed enrichment analysis to gain insights into the biological functions of PCOS-associated A-to-I RNA editing in GCs. Our results revealed that the differentially edited genes were primarily enriched in biological processes related to the regulation of the mitotic cell cycle and transmembrane transporter processes (Figure 5A). Moreover, the most significantly enriched KEGG pathways of the differentially edited genes included apoptosis, necroptosis, and coronavirus disease (Figure 5B), implicating a potential link between PCOS and coronavirus disease.
Figure 5 GO (A) and KEGG pathways (B) enriched by genes with differential A-to-I RNA editing between PCOS and controls.
To gain insights into the potential mechanism of PCOS-associated RNA editing, we employed the RBPsmap website to predict the RBP binding of the identified RNA editing sites (Supplementary Figure S5). Figure 6 illustrates the top 10 RBPs with the highest binding frequency of differential RNA editing sites. Notably, RNA binding motif protein 45 (RBM45), splicing factor proline and glutamine rich (SFPQ), and heterogeneous nuclear ribonucleoprotein L (HNRNPL) were found to most frequently bind to the PCOS-associated RNA editing sites.
Figure 6 RNA-binding protein (RBPs) prediction showing the top 10 frequent RBPs binding to DRE sites.
To validate the common characteristics of RNA editing, we conducted a comparative analysis using another PCOS and control GC sample dataset (PRJNA762274) from an independent study (13). By using the same analysis procedure, we identified the differential editing sites shown in Supplementary Table S3. To assess their functional relevance, we then performed enrichment analysis on the common set of differentially edited genes shared between the discovery dataset GSE138518 and the validation dataset PRJNA762274. The Venn plots (Figures 7A, B) show the overlap between the discovery and validation datasets, revealing 55 differentially edited genes and nine differential editing sites shared by both datasets. Furthermore, the enrichment analysis found that these 55 edited genes were mainly involved in apoptosis, the HIF-1 signaling pathway, cellular response to thyroid hormone stimulus, and arylsulfatase activity (Figure 7C). Among the nine differential editing sites, six in Scavenger Receptor Class B Member 1 (SCARB1), Thioredoxin Domain Containing 15 (TXNDC15), RNA Polymerase I Subunit E (POLR1E), DEAD-Box Helicase 19A (DDX19A), and FKBP Prolyl Isomerase 11 (FKBP11) showed consistent changes between the two datasets, namely SCARB1:chr12:124783357, TXNDC15:chr5:134900418, POLR1E:chr9:37503395, DDX19A:chr16:70372975, AC013394.1:chr15:92889425, and FKBP11:chr12:48923107 (Figures 7D, E).
Figure 7 Validation of RNA editing events in dataset PRJNA762274. (A) Venn plot of differential edited genes between datasets GSE138518 and PRJNA762274. (B) Venn plot of differential editing sites between datasets GSE138518 and PRJNA762274. (C) The top 5 functional analysis terms of KEGG and GO for the 55 common differentially edited genes shared by the two datasets. (D) Six common differential RNA editing sites with the same differential editing trend in dataset GSE138518. (E) Six common differential RNA editing sites with the same differential editing trend in dataset PRJNA762274. P, PCOS; C, controls.
Understanding the molecular mechanism of PCOS could help develop new stratiges for diagnosis and treatment of PCOS (29, 30). Several biomarkers associated with PCOS have been identified and might serve as potential therapeutic targets. RNA editing has been reported to play a role in cancer, aging, neurological, and autoimmune diseases, yet how it is involved in PCOS remains poorly understood (31). In this study, we systematically investigated and validated A-to-I RNA editing associated with PCOS as biomarkers at the transcriptome level, providing valuable insights into the underlying mechanisms of PCOS.
RNA editing dysfunction, particularly A-to-I RNA editing events, has been implicated in various diseases, including immune signaling pathways and certain cancers (32–35). Recent studies have shown that synonymous variants can also affect mRNA splicing, mRNA stability, and protein function (36–38). We identified two synonymous variants, TUBA1B:chr12:49128795 and MX1:chr21:41452734, while the role of the two in PCOS is unclear. However, TUBA1B, a critical gene in postmenopausal osteoporosis, has also been linked to BMI in children’s muscles and the overall survival of colorectal cancer patients (39–41). The expression level of MX1 in cumulus-oophorus cells was related to the immunological defense processes (42). Regarding the 3′UTR RNA editing, NUP43:chr6:149725375, PHLDA1:chr12:76027474, and RBBP4:chr1:32680141 were the top three significant editing sites. PHLDA1 has been reported to possibly contribute to PCOS phenotypes and regulate proinflammatory cytokine production by interacting with Tollip (43, 44). RBBP4 is involved in apoptosis in early mouse embryonic development, and NUP43 plays a crucial role in various cancers, including breast cancer and gastric cancer (45–47). Further study is necessary to determine the roles of these genes and their RNA editing in PCOS.
Our cis-Regulation analysis showed that 20 DRE sites were significantly related to the gene expression level. Notably, the expression levels of MIR193BHG and HOOK3 showed significant differences between the two groups. Previous studies have identified MIR193BHG as a prognostic marker in pancreatic, ovarian, and head and neck squamous cell carcinoma (48–50). Additionally, MIR193BHG might play a pivotal role in preeclampsia related to blood pressure and urine protein and could be a potential prognostic biomarker in early-onset preeclampsia (51, 52). The phosphorylation of HOOK3 has been shown to regulate Golgi stability during mitosis (53). High HOOK3 expression could predict a poor prognosis for prostate cancer (54). Future research should focus on elucidating the underlying molecular mechanisms and conducting functional studies to validate the clinical significance of such cis-regulatory editing.
Functional enrichment analysis showed that apoptosis and necroptosis mainly enriched PCOS-associated RNA editing. In previous studies, apoptosis and necroptosis have been reported to be linked with PCOS. For instance, SH2B adaptor protein 3 (SH2B3, also called LNK) could promote GC apoptosis through the AKT-FOXO3 pathway (55). Apoptosis could also be regulated by the toll-like receptor TLR8 (56). In addition, apoptosis and necroptosis were involved in the modulation of hyperandrogenism (57).
Our findings also reveal decreased ADARB1 expression in PCOS correlated with seven downregulated DRE sites. In ovarian cancer, the downregulation of ADARB1 has been reported to have a potential role in the development of ovarian cancer (58). These edited genes could exert a related role in various diseases. For instance, CCDC69 has been associated with immune infiltration and serves as a prognostic marker in breast and colon cancers (59, 60). Polymorphisms in SLC47A1 are associated with type 2 diabetes (61, 62). Additionally, the hyperediting of FAM126B, represented by FAM126B:chr2:200978748 and FAM126B:chr2:200978747, might potentially contribute to PCOS. Taken together, such findings indicated a substantial role of ADARB1 and RNA editing mediated by it in the pathophysiological process of PCOS.
RBPs play a crucial role in regulating post-transcriptional processes, including RNA splicing, decay, and editing (63, 64). Importantly, our findings suggest that RBM45 is the RBP with the highest number of binding sites in the identified differentially edited genes. Previous studies have reported the regulation of splicing machinery by RBM45 in liver biopsies from patients with nonalcoholic fatty liver disease (65). Furthermore, RBM45 has been shown to bind to N6-methyladenosine, thereby controlling and regulating mRNA processing (66). These results highlight the possible regulatory role of RBPs in the biological functions of RNA editing in the context of PCOS.
In conclusion, our study conducted a transcriptome-wide analysis of PCOS-associated A-to-I RNA editing sites and provided new insight into understanding the role of RNA editing in the pathogenesis of PCOS.
The original contributions presented in the study are included in the article/Supplementary Material. Further inquiries can be directed to the corresponding author.
FSK and ZJL contributed equally to this article. FSK contributed to drafting the manuscript. YPM, ZJL and JHW revised and improved the writing of the manuscript. YZ performed the data analysis. YHL, RFJ, BLZ, PWH and CYR contributed to the revision of the manuscript. JHC and YPM designed the study and revised the manuscript. All authors contributed to the article and approved the submitted version.
This project was supported by the National Natural Science Foundation of China (No. 31671311); the National First-Class Discipline Program of Light Industry Technology and Engineering (LITE2018-14); the “Six-Talent Peak” Plan of Jiangsu Province (No. SWYY-127); the Innovative and Entrepreneurial Talents of Jiangsu Province, the Program for High-Level Entrepreneurial and Innovative Talents of Jiangsu Province, Taihu Lake Talent Plan, and the Fundamental Research Funds for the Central Universities (JUSRP51712B and JUSRP1901XNC); the Postgraduate Research and Practice Innovation Program of Jiangsu Province (KYCX20_1946); the Top Talent Support Program for Young and Middle-Aged People of Wuxi Health Committee (No. HB2020038); the Maternal and Child Health Research Project of Jiangsu in China (No. F202117); the Maternal and Child Health Research Project of Wuxi (No. FYKY201804); and the Excellent Program for Overseas Students of Wuxi in 2018 (No. 23).
The authors declare that the research was conducted in the absence of any commercial or financial relationships that could be construed as a potential conflict of interest.
All claims expressed in this article are solely those of the authors and do not necessarily represent those of their affiliated organizations, or those of the publisher, the editors and the reviewers. Any product that may be evaluated in this article, or claim that may be made by its manufacturer, is not guaranteed or endorsed by the publisher.
The Supplementary Material for this article can be found online at: https://www.frontiersin.org/articles/10.3389/fendo.2023.1170957/full#supplementary-material
Supplementary Figure 1 | The single-nucleotide variant density in various chromosomes.
Supplementary Figure 2 | The sequence preference of the A-to-I RNA editing sites in PCOS.
Supplementary Figure 3 | Principal Component Analysis of the samples of PCOS and control.
Supplementary Figure 4 | The editing level of Cis-Regulatory editing sites.
Supplementary Figure 5 | The word cloud of the types of RBPs targeting differential editing sites.
1. Bass BL. RNA Editing by adenosine deaminases that act on RNA. Annu Rev Biochem (2002) 71:817–46. doi: 10.1146/annurev.biochem.71.110601.135501
2. Gu T, Buaas FW, Simons AK, Ackert-Bicknell CL, Braun RE, Hibbs MA. Canonical a-to-I and c-to-U RNA editing is enriched at 3’UTRs and microRNA target sites in multiple mouse tissues. PloS One (2012) 7(3):e33720. doi: 10.1371/journal.pone.0033720
3. Rice GI, Kasher PR, Forte GMA, Mannion NM, Greenwood SM, Szynkiewicz M, et al. Mutations in ADAR1 cause aicardi-goutières syndrome associated with a type I interferon signature. Nat Genet (2012) 44(11):1243–8. doi: 10.1038/ng.2414
4. Jain M, Mann TD, Stulić M, Rao SP, Kirsch A, Pullirsch D, et al. RNA Editing of filamin a pre-mRNA regulates vascular contraction and diastolic blood pressure. EMBO J (2018) 37(19):e94813. doi: 10.15252/embj.201694813
5. Eran A, Li JB, Vatalaro K, McCarthy J, Rahimov F, Collins C, et al. Comparative RNA editing in autistic and neurotypical cerebella. Mol Psychiatry (2013) 18(9):1041–8. doi: 10.1038/mp.2012.118
6. Cenci C, Barzotti R, Galeano F, Corbelli S, Rota R, Massimi L, et al. Down-regulation of RNA editing in pediatric astrocytomas: ADAR2 editing activity inhibits cell migration and proliferation. J Biol Chem (2008) 283(11):7251–60. doi: 10.1074/jbc.M708316200
7. Escobar-Morreale HF. Polycystic ovary syndrome: definition, aetiology, diagnosis and treatment. Nat Rev Endocrinol (2018) 14(5):270–84. doi: 10.1038/nrendo.2018.24
8. Rosenfield RL, Ehrmann DA. The pathogenesis of polycystic ovary syndrome (PCOS): the hypothesis of PCOS as functional ovarian hyperandrogenism revisited. Endocr Rev (2016) 37(5):467–520. doi: 10.1210/er.2015-1104
9. Zheng Q, Li Y, Zhang D, Cui X, Dai K, Yang Y, et al. ANP promotes proliferation and inhibits apoptosis of ovarian granulosa cells by NPRA/PGRMC1/EGFR complex and improves ovary functions of PCOS rats. Cell Death Dis (2017) 8(10):e3145. doi: 10.1038/cddis.2017.494
10. Qu F, Wang FF, Yin R, Ding GL, El-Prince M, Gao Q, et al. A molecular mechanism underlying ovarian dysfunction of polycystic ovary syndrome: hyperandrogenism induces epigenetic alterations in the granulosa cells. J Mol Med (Berl) (2012) 90(8):911–23. doi: 10.1007/s00109-012-0881-4
11. Cao P, Yang W, Wang P, Li X, Nashun B. Characterization of DNA methylation and screening of epigenetic markers in polycystic ovary syndrome. Front Cell Dev Biol (2021) 9:664843. doi: 10.3389/fcell.2021.664843
12. Mao Z, Li T, Zhao H, Qin Y, Wang X, Kang Y. Identification of epigenetic interactions between microRNA and DNA methylation associated with polycystic ovarian syndrome. J Hum Genet (2021) 66(2):123–37. doi: 10.1038/s10038-020-0819-6
13. Tu M, Wu Y, Wang F, Huang Y, Qian Y, Li J, et al. Effect of lncRNA MALAT1 on the granulosa cell proliferation and pregnancy outcome in patients with PCOS. Front Endocrinol (Lausanne) (2022) 13:825431. doi: 10.3389/fendo.2022.825431
14. Chen S, Zhou Y, Chen Y, Gu J. fastp: an ultra-fast all-in-one FASTQ preprocessor. Bioinformatics (2018) 34(17):i884–90. doi: 10.1093/bioinformatics/bty560
15. Dobin A, Gingeras TR. Mapping RNA-seq reads with STAR. Curr Protoc Bioinf (2015) 51:11.14.1–11.14.19. doi: 10.1002/0471250953.bi1114s51
16. Li H, Handsaker B, Wysoker A, Fennell T, Ruan J, Homer N, et al. The sequence Alignment/Map format and SAMtools. Bioinformatics (2009) 25(16):2078–9. doi: 10.1093/bioinformatics/btp352
17. Van der Auwera GA, Carneiro MO, Hartl C, Poplin R, Del Angel G, Levy-Moonshine A, et al. From FastQ data to high confidence variant calls: the genome analysis toolkit best practices pipeline. Curr Protoc Bioinf (2013) 43:11.10.1–11.10.33. doi: 10.1002/0471250953.bi1110s43
18. Koboldt DC, Zhang Q, Larson DE, Shen D, McLellan MD, Lin L, et al. VarScan 2: somatic mutation and copy number alteration discovery in cancer by exome sequencing. Genome Res (2012) 22(3):568–76. doi: 10.1101/gr.129684.111
19. McLaren W, Gil L, Hunt SE, Riat HS, Ritchie GRS, Thormann A, et al. The ensembl variant effect predictor. Genome Biol (2016) 17(1):122. doi: 10.1186/s13059-016-0974-4
20. Ru FX, Kong F, Ren CY, He YS, Xia SY, Li YN, et al. Repeated winning and losing experiences in chronic social conflicts are linked to RNA editing pattern difference. Front Psychiatry (2022) 13:896794. doi: 10.3389/fpsyt.2022.896794
21. Mansi L, Tangaro MA, Lo Giudice C, Flati T, Kopel E, Schaffer AA, et al. REDIportal: millions of novel a-to-I RNA editing events from thousands of RNAseq experiments. Nucleic Acids Res (2021) 49(D1):D1012–9. doi: 10.1093/nar/gkaa916
22. Lo Giudice C, Tangaro MA, Pesole G, Picardi E. Investigating RNA editing in deep transcriptome datasets with REDItools and REDIportal. Nat Protoc (2020) 15(3):1098–131. doi: 10.1038/s41596-019-0279-7
23. Liao Y, Smyth GK, Shi W. featureCounts: an efficient general purpose program for assigning sequence reads to genomic features. Bioinformatics (2014) 30(7):923–30. doi: 10.1093/bioinformatics/btt656
24. McCarthy DJ, Chen Y, Smyth GK. Differential expression analysis of multifactor RNA-seq experiments with respect to biological variation. Nucleic Acids Res (2012) 40(10):4288–97. doi: 10.1093/nar/gks042
25. Kuleshov MV, Jones MR, Rouillard AD, Fernandez NF, Duan Q, Wang Z, et al. Enrichr: a comprehensive gene set enrichment analysis web server 2016 update. Nucleic Acids Res (2016) 44(W1):W90–97. doi: 10.1093/nar/gkw377
26. Lorenz R, Bernhart SH, Höner Zu Siederdissen C, Tafer H, Flamm C, Stadler PF, et al. ViennaRNA package 2.0. Algorithms Mol Biol (2011) 6:26. doi: 10.1186/1748-7188-6-26
27. Gruber AR, Lorenz R, Bernhart SH, Neuböck R, Hofacker IL. The Vienna RNA websuite. Nucleic Acids Res (2008) 36(suppl_2):W70–4. doi: 10.1093/nar/gkn188
28. Paz I, Kosti I, Ares M, Cline M, Mandel-Gutfreund Y. RBPmap: a web server for mapping binding sites of RNA-binding proteins. Nucleic Acids Res (2014) 42(Web Server issue):W361–367. doi: 10.1093/nar/gku406
29. Zeng Z, Lin X, Xia T, Liu W, Tian X, Li M. Identification of crucial lncRNAs, miRNAs, mRNAs, and potential therapeutic compounds for polycystic ovary syndrome by bioinformatics analysis. BioMed Res Int (2020) 2020:1817094. doi: 10.1155/2020/1817094
30. Zou J, Li Y, Liao N, Liu J, Zhang Q, Luo M, et al. Identification of key genes associated with polycystic ovary syndrome (PCOS) and ovarian cancer using an integrated bioinformatics analysis. J Ovarian Res (2022) 15(1):30. doi: 10.1186/s13048-022-00962-w
31. Gatsiou A, Vlachogiannis N, Lunella FF, Sachse M, Stellos K. Adenosine-to-Inosine RNA editing in health and disease. Antioxid Redox Signal (2018) 29(9):846–63. doi: 10.1089/ars.2017.7295
32. Qi L, Chan THM, Tenen DG, Chen L. RNA Editome imbalance in hepatocellular carcinoma. Cancer Res (2014) 74(5):1301–6. doi: 10.1158/0008-5472.CAN-13-3485
33. Costa Cruz PH, Kawahara Y. RNA Editing in neurological and neurodegenerative disorders. Methods Mol Biol (2021) 2181:309–30. doi: 10.1007/978-1-0716-0787-9_18
34. Ahmad S, Mu X, Hur S. The role of RNA editing in the immune response. Methods Mol Biol (2021) 2181:287–307. doi: 10.1007/978-1-0716-0787-9_17
35. Fritzell K, Xu LD, Lagergren J, Öhman M. ADARs and editing: the role of a-to-I RNA modification in cancer progression. Semin Cell Dev Biol (2018) 79:123–30. doi: 10.1016/j.semcdb.2017.11.018
36. Chamary JV, Parmley JL, Hurst LD. Hearing silence: non-neutral evolution at synonymous sites in mammals. Nat Rev Genet (2006) 7(2):98–108. doi: 10.1038/nrg1770
37. Gu W, Zhou T, Wilke CO. A universal trend of reduced mRNA stability near the translation-initiation site in prokaryotes and eukaryotes. PloS Comput Biol (2010) 6(2):e1000664. doi: 10.1371/journal.pcbi.1000664
38. Kimchi-Sarfaty C, Oh JM, Kim IW, Sauna ZE, Calcagno AM, Ambudkar SV, et al. A ‘silent’ polymorphism in the MDR1 gene changes substrate specificity. Science (2007) 315(5811):525–8. doi: 10.1126/science.1135308
39. Ma M, Chen X, Lu L, Yuan F, Zeng W, Luo S, et al. Identification of crucial genes related to postmenopausal osteoporosis using gene expression profiling. Aging Clin Exp Res (2016) 28(6):1067–74. doi: 10.1007/s40520-015-0509-y
40. Yao S, Wu H, Ding JM, Wang ZX, Ullah T, Dong SS, et al. Transcriptome-wide association study identifies multiple genes associated with childhood body mass index. Int J Obes (Lond) (2021) 45(5):1105–13. doi: 10.1038/s41366-021-00780-y
41. Hu J, Han C, Zhong J, Liu H, Liu R, Luo W, et al. Dynamic network biomarker of pre-exhausted CD8+ T cells contributed to T cell exhaustion in colorectal cancer. Front Immunol (2021) 12:691142. doi: 10.3389/fimmu.2021.691142
42. Chermuła B, Hutchings G, Kranc W, Józkowiak M, Jopek K, Stelmach B, et al. Expression profile of new gene markers and signaling pathways involved in immunological processes in human cumulus-oophorus cells. Genes (Basel) (2021) 12(9):1369. doi: 10.3390/genes12091369
43. Pau CT, Mosbruger T, Saxena R, Welt CK. Phenotype and tissue expression as a function of genetic risk in polycystic ovary syndrome. PloS One (2017) 12(1):e0168870. doi: 10.1371/journal.pone.0168870
44. Peng H, Wang J, Song X, Huang J, Hua H, Wang F, et al. PHLDA1 suppresses TLR4-triggered proinflammatory cytokine production by interaction with tollip. Front Immunol (2022) 13:731500. doi: 10.3389/fimmu.2022.731500
45. Miao X, Sun T, Barletta H, Mager J, Cui W. Loss of RBBP4 results in defective inner cell mass, severe apoptosis, hyperacetylated histones and preimplantation lethality in mice†. Biol Reprod (2020) 103(1):13–23. doi: 10.1093/biolre/ioaa046
46. Tian C, Zhou S, Yi C. High NUP43 expression might independently predict poor overall survival in luminal a and in HER2+ breast cancer. Future Oncol (2018) 14(15):1431–42. doi: 10.2217/fon-2017-0690
47. Li W, Duan J, Shi W, Lei L, Lv P. Long non-coding RNA NCK1-AS1 serves an oncogenic role in gastric cancer by regulating miR-137/NUP43 axis. Onco Targets Ther (2020) 13:9929–39. doi: 10.2147/OTT.S259336
48. Li J, Zhang J, Tao S, Hong J, Zhang Y, Chen W. Prognostication of pancreatic cancer using the cancer genome atlas based ferroptosis-related long non-coding RNAs. Front Genet (2022) 13:838021. doi: 10.3389/fgene.2022.838021
49. Meng C, Zhou JQ, Liao YS. Autophagy-related long non-coding RNA signature for ovarian cancer. J Int Med Res (2020) 48(11):300060520970761. doi: 10.1177/0300060520970761
50. Zheng D, Luo S, Wang S, Huang J, Zhou Y, Su L, et al. Construction of a competing endogenous RNA network in head and neck squamous cell carcinoma by pan-cancer analysis. Transl Cancer Res (2022) 11(9):3050–63. doi: 10.21037/tcr-22-632
51. Dong N, Li D, Cai H, Shi L, Huang L. Expression of lncRNA MIR193BHG in serum of preeclampsia patients and its clinical significance. J Gynecol Obstet Hum Reprod (2022) 51(5):102357. doi: 10.1016/j.jogoh.2022.102357
52. Zhang Z, Wang P, Zhang L, Huang C, Gao J, Li Y, et al. Identification of key genes and long noncoding RNA-associated competing endogenous RNA (ceRNA) networks in early-onset preeclampsia. BioMed Res Int (2020) 2020:1673486. doi: 10.1155/2020/1673486
53. Wortzel I, Maik-Rachline G, Yadav SS, Hanoch T, Seger R. Mitotic HOOK3 phosphorylation by ERK1c drives microtubule-dependent golgi destabilization and fragmentation. iScience (2021) 24(6):102670. doi: 10.1016/j.isci.2021.102670
54. Melling N, Harutyunyan L, Hube-Magg C, Kluth M, Simon R, Lebok P, et al. High-level HOOK3 expression is an independent predictor of poor prognosis associated with genomic instability in prostate cancer. PloS One (2015) 10(7):e0134614. doi: 10.1371/journal.pone.0134614
55. Tan M, Cheng Y, Zhong X, Yang D, Jiang S, Ye Y, et al. LNK promotes granulosa cell apoptosis in PCOS via negatively regulating insulin-stimulated AKT-FOXO3 pathway. Aging (Albany NY) (2021) 13(3):4617–33. doi: 10.18632/aging.202421
56. Yu Y, Li G, He X, Lin Y, Chen Z, Lin X, et al. MicroRNA-21 regulate the cell apoptosis and cell proliferation of polycystic ovary syndrome (PCOS) granulosa cells through target toll like receptor TLR8. Bioengineered (2021) 12(1):5789–96. doi: 10.1080/21655979.2021.1969193
57. Zhang Y, Hu M, Jia W, Liu G, Zhang J, Wang B, et al. Hyperandrogenism and insulin resistance modulate gravid uterine and placental ferroptosis in PCOS-like rats. J Endocrinol (2020) 246(3):247–63. doi: 10.1530/JOE-20-0155
58. Zhu W, Xu Z, Huang M, Wang X, Ren X, Cai Y, et al. Downregulated ADARB1 facilitates cell proliferation, invasion and has effect on the immune regulation in ovarian cancer. Front Bioeng Biotechnol (2021) 9:792911. doi: 10.3389/fbioe.2021.792911
59. Yi Y, Xu T, Tan Y, Lv W, Zhao C, Wu M, et al. CCDC69 is a prognostic marker of breast cancer and correlates with tumor immune cell infiltration. Front Surg (2022) 9:879921. doi: 10.3389/fsurg.2022.879921
60. Wang X, Duanmu J, Fu X, Li T, Jiang Q. Analyzing and validating the prognostic value and mechanism of colon cancer immune microenvironment. J Transl Med (2020) 18(1):324. doi: 10.1186/s12967-020-02491-w
61. Chen P, Cao Y, Chen S, Liu Z, Chen S, Guo Y. Association of SLC22A1, SLC22A2, SLC47A1, and SLC47A2 polymorphisms with metformin efficacy in type 2 diabetic patients. Biomedicines (2022) 10(10):2546. doi: 10.3390/biomedicines10102546
62. Kim H, Bae S, Yoon HY, Yee J, Gwak HS. Association of the SLC47A1 gene variant with responses to metformin monotherapy in drug-naive patients with type 2 diabetes. J Clin Endocrinol Metab (2022) 107(9):2684–90. doi: 10.1210/clinem/dgac333
63. Hu X, Zou Q, Yao L, Yang X. Survey of the binding preferences of RNA-binding proteins to RNA editing events. Genome Biol (2022) 23(1):169. doi: 10.1186/s13059-022-02741-8
64. Xu W, Biswas J, Singer RH, Rosbash M. Targeted RNA editing: novel tools to study post-transcriptional regulation. Mol Cell (2022) 82(2):389–403. doi: 10.1016/j.molcel.2021.10.010
65. Del Río-Moreno M, Alors-Pérez E, González-Rubio S, Ferrín G, Reyes O, Rodríguez-Perálvarez M, et al. Dysregulation of the splicing machinery is associated to the development of nonalcoholic fatty liver disease. J Clin Endocrinol Metab (2019) 104(8):3389–402. doi: 10.1210/jc.2019-00021
Keywords: PCOS, epigenetics, cis-regulatory analysis, A-to-I RNA editing, apoptosis
Citation: Kong F-S, Lu Z, Zhou Y, Lu Y, Ren C-Y, Jia R, Zeng B, Huang P, Wang J, Ma Y and Chen J-H (2023) Transcriptome analysis identification of A-to-I RNA editing in granulosa cells associated with PCOS. Front. Endocrinol. 14:1170957. doi: 10.3389/fendo.2023.1170957
Received: 21 February 2023; Accepted: 06 June 2023;
Published: 21 July 2023.
Edited by:
Michał Kunicki, Medical University of Warsaw, PolandReviewed by:
Raviprasad Aduri, Birla Institute of Technology and Science, IndiaCopyright © 2023 Kong, Lu, Zhou, Lu, Ren, Jia, Zeng, Huang, Wang, Ma and Chen. This is an open-access article distributed under the terms of the Creative Commons Attribution License (CC BY). The use, distribution or reproduction in other forums is permitted, provided the original author(s) and the copyright owner(s) are credited and that the original publication in this journal is cited, in accordance with accepted academic practice. No use, distribution or reproduction is permitted which does not comply with these terms.
*Correspondence: Jian-Huan Chen, Y2poX2Jpb0Bob3RtYWlsLmNvbQ==; Yaping Ma, bXlwMTEyQDE2My5jb20=
†These authors have contributed equally to this work
Disclaimer: All claims expressed in this article are solely those of the authors and do not necessarily represent those of their affiliated organizations, or those of the publisher, the editors and the reviewers. Any product that may be evaluated in this article or claim that may be made by its manufacturer is not guaranteed or endorsed by the publisher.
Research integrity at Frontiers
Learn more about the work of our research integrity team to safeguard the quality of each article we publish.