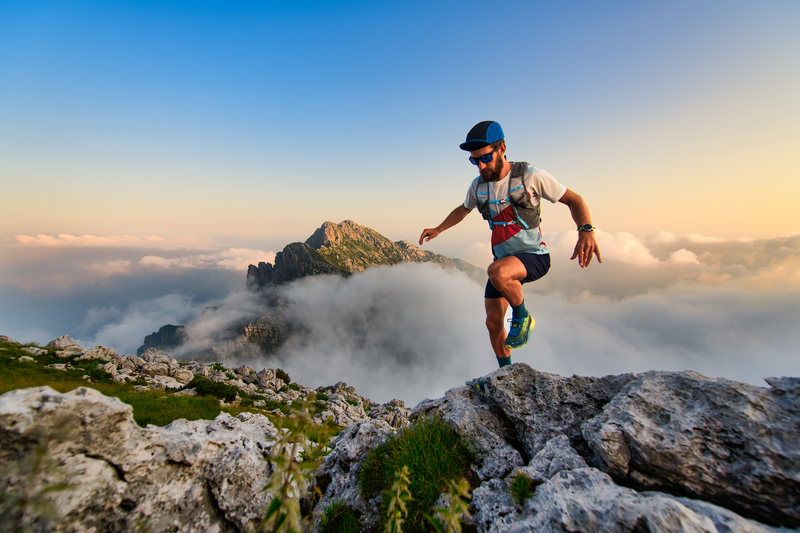
95% of researchers rate our articles as excellent or good
Learn more about the work of our research integrity team to safeguard the quality of each article we publish.
Find out more
STUDY PROTOCOL article
Front. Endocrinol. , 07 June 2023
Sec. Obesity
Volume 14 - 2023 | https://doi.org/10.3389/fendo.2023.1168688
This article is part of the Research Topic Obesity, Hyperglycemia, and Pregnancy: From Pathophysiology to Clinical Practice - Volume II View all 5 articles
Background: Gestational diabetes mellitus (GDM) is a common complication of pregnancy associated with serious adverse outcomes for mothers and their offspring. Achieving glycaemic targets is the mainstream in the treatment of GDM in order to improve pregnancy outcomes. As GDM is usually diagnosed in the third trimester of pregnancy, the time frame for the intervention is very narrow. Women need to get new knowledge and change their diet very quickly. Usually, these patients require additional frequent visits to healthcare professionals. Recommender systems based on artificial intelligence could partially substitute healthcare professionals in the process of educating and controlling women with GDM, thus reducing the burden on the women and healthcare systems. We have developed a mobile-based personalized recommendation system DiaCompanion I with data-driven real time personal recommendations focused primarily on postprandial glycaemic response prediction. The study aims to clarify the effect of using DiaCompanion I on glycaemic levels and pregnancy outcomes in women with GDM.
Methods: Women with GDM are randomized to 2 treatment groups: utilizing and not utilizing DiaCompanion I. The app provides women in the intervention group the resulting data-driven prognosis of 1-hour postprandial glucose level every time they input their meal data. Based on the predicted glucose level, they can adjust their current meal so that the predicted glucose level falls within the recommended range below 7 mmol/L. The app also provides reminders and recommendations on diet and lifestyle to the participants of the intervention group. All the participants are required to perform 6 blood glucose measurements a day. Capillary glucose values are retrieved from the glucose meter and if not available, from the woman’s diary. Additionally, data on glycaemic levels during the study and consumption of major macro- and micronutrients will be collected using the mobile app with electronic report forms in the intervention group. Women from the control group receive standard care without the mobile app. All participants are prescribed with insulin therapy if needed and modifications in their lifestyle. A total of 216 women will be recruited. The primary outcome is the percentage of postprandial capillary glucose values above target (>7.0 mmol/L). Secondary outcomes include the percentage of patients requiring insulin therapy during pregnancy, maternal and neonatal outcomes, glycaemic control using glycated hemoglobin (HbA1c), continuous glucose monitoring data and other blood glucose metrics, the number of patient visits to endocrinologists and acceptance/satisfaction of the two strategies assessed using a questionnaire.
Discussion: We believe that the approach including DiaCompanion I will be more effective in patients with GDM for improving glycaemic levels and pregnancy outcomes. We also expect that the use of the app will help reduce the number of clinic visits.
Trial registration number: ClinicalTrials.gov, Identifier NCT05179798.
Gestational diabetes mellitus (GDM) is a common endocrine disorder complicating up to 9-26% of pregnancies, if diagnosed according to IADPSG consensus panel-recommended criteria (1). GDM is associated with adverse pregnancy outcomes, including but not limited to increased rate of cesarean section, macrosomic infants, shoulder dystocia and birth trauma (2). In long term perspective, GDM is associated with an increased risk of obesity, type 2 diabetes mellitus and cardiovascular diseases in mothers and their offspring (3).
Maintaining normal glycaemic levels in pregnancy is essential to prevent adverse pregnancy outcomes and to break the vicious cycle of intergenerational transmission of predisposition to metabolic diseases (4, 5).
Diet is the mainstay of the treatment of GDM. As GDM is usually diagnosed in the third trimester of pregnancy, the time frame for the intervention is very narrow. Women need to get new knowledge and to change their diet very quickly. Thus, the development of effective methods of selecting optimal meal composition to prevent elevated postprandial glycaemic response (PPGR) is important for the treatment of patients with GDM.
Usually, patients with GDM require additional frequent visits to healthcare professionals. Recommender systems based on artificial intelligence could partially substitute healthcare professionals in the process of educating and controlling women with GDM, thus reducing the burden for the women and healthcare systems.
We have developed a mobile-based personalized recommendation system DiaCompanion I with data-driven real time personal recommendations focused primarily on PPGR prediction. The process of developing algorithms for predicting parameters of PPGR [1-hour postprandial blood glucose level (BG60), incremental area under the glycaemic curve 2 hours after meal (iAUC120)] using regression models constructed from anthropometry and laboratory examination data, medical history, questionnaires and food diaries of pregnant women with GDM is described elsewhere as well as the prototype of the recommendation system DiaCompanion (6–8).
Using the developed models, adequate accuracy in predicting PPGRs for women with GDM was obtained (7). The model for predicting peak blood glucose levels after meals, with a mean absolute error of 0.48 mmol/l, formed the basis of the recommendation block of the DiaCompanion I mobile application developed by the authors.
The interactive mobile app, DiaCompanion I, provides real-time prediction of GDM and recommends changes in food intake to prevent hyperglycaemia. The mobile app includes a recommendation block based on recommendations and guidelines for the management of patients with GDM.
A model built into the app predicts what blood glucose levels a woman with GDM will have after a particular meal and, if high blood glucose levels are predicted, estimates the reasons for the increase and shows dietary recommendations to prevent hyperglycaemia.
Currently there is growing interest in digital health solutions for the management of women with GDM. A recent meta-analysis has shown benefits of using m-health solutions to improve glycaemic control and pregnancy outcomes in GDM (9). However, comparison is complicated by the differences among the developed technologies and by little agreement on the key components of interventions. Only a few digital health applications have been integrated in clinical practice (10). To the best of our knowledge, no one of the published studies used PPGR prediction in their digital health solutions for women with GDM.
We aim to clarify the effect of using mobile-based personalized recommendation system DiaCompanion I on glycaemic levels and pregnancy outcomes in women with GDM.
This study is an interventional open-labeled randomized controlled trial. A total of 216 women with GDM will take part in this trial performed in the Almazov National Medical Research Centre. Patients are randomized 1:1 to the intervention or control group. The intervention group receives DiaCompanion I app and standard antenatal care with the reduced number of visits to endocrinologists. The control group receives standard antenatal care. Patients participate in the study from recruitment (between 12 and 32 completed weeks of pregnancy) until delivery and will be invited to the follow-up visit at 3-6 months postpartum. The study protocol follows the Standard Protocol Items: Recommendations for Interventional Trials (SPIRIT) (11). Study design and data collection are shown in Figure 1. Please find the detailed information on the intervention and data collection in the sections below.
Pregnant women with GDM are invited to participate if they are not more than 4 weeks after confirmation of GDM diagnosis (after a clinical visit when patient is informed of the diagnosis and prescribed with diet). GDM is diagnosed according to IADPSG (International Association of the Diabetes and Pregnancy Study Groups) criteria, i.e. fasting plasma glucose between 5.1 mmol/L and 6.9 mmol/L after the 6th week of gestation and/or 1-hour plasma glucose value after 75 g oral glucose tolerance test (OGTT) ≥ 10.0 mmol/L and/or 2-hour plasma glucose value between 8.5 mmol/L and 11.0 mmol/L at the 24-31st week of gestation. According to Russian national guidelines, OGTT is performed between 24th-28th week of gestation and in exceptional cases can be performed up to the 32nd gestational week. The inclusion and exclusion criteria are listed in Table 1.
Participants are recruited from the outpatient department of the Perinatal Center of the Almazov National Medical Research Center and from antenatal clinics of Saint Petersburg. Local obstetricians and endocrinologists inform about the possibility to participate in the DiaCompanion I trial and share flyers with pregnant women during their first visit after GDM confirmation. Pregnant women with GDM willing to participate are screened by the team members (endocrinologists of the Almazov Centre) for eligibility. Those women that meet the inclusion/exclusion criteria listed in Table 1 and sign the consent form are included into the study. The consent form is signed by the woman and a qualified health-care professional with delegated authority in two copies, one for the woman and one for the study records. We started the recruitment in January 2022 and we plan to continue until November 2024.
Women are randomized individually in an equal ratio of standard care (control) to DiaCompanion I app use (intervention). Randomization is performed with a randomized computer-generated number table generated by the statistician using the randomizeR package in R (12) prior to the first participant’s baseline visit. The randomization list is available only to research officers. А research member in the clinic will call research officers to obtain group allocation during the baseline visit after obtaining the consent form.
Intervention group will use the mobile app DiaCompanion I with personalized dietary recommendations. DiaCompanion I was developed in 2021. In the previous study, we developed and tested in clinical practice the mobile application DiaCompanion for data collection and exchange (8, 13, 14). It allows tracking of food intakes, physical activities, insulin doses, urine ketones and blood glucose measurements performed by the participants. The data accumulated during the study on the postprandial glycaemic response (PPGR) of GDM patients to certain meals in various situations allowed us to develop predictive models for assessing the risk of a high PPGR after a meal based on data on the current meal, meals during the last day, and individual characteristics of the patient (7).
In addition to the functions of DiaCompanion, the DiaCompanion I app contains a recommendation block which predicts the PPGR based on the personal parameters, current planned meal content and information from the diary for the last few days (Figure 2). Then, depending on the predicted postprandial BG level, the application generates one of the pop-up messages with recommendations. In the case of predicting a BG above target (> 7,0 mmol/L), pop-up messages contain recommendations on how to prevent high postprandial glucose after the planned meal. The messages include recommendations to exclude or reduce the number of foods with a high glycaemic index (GI), reduce the number of carbohydrates in the index meal or during snacks, and make changes to the meal. As participants receive predicted BG level and corresponding recommendations at the time of planning a food intake, they can change the content of the planned meal in the app till the predicted BG value falls within the target range. In the case of predicting a PPGR within the normal range, a message appears about a successfully added record. The app also analyzes potential reasons for a high predicted BG level. If the reason is beyond the high glycaemic load of the planned meal, recommendations on additional physical activity, reduction of carbohydrates in snacks, increasing the consumption of fibers and/or seeking advice from endocrinologist may appear.
Figure 2 The recommendation system DiaCompanion I with personalized dietary recommendations for women with GDM.
The application implements a system for visualizing the quantitative effect on the PPGR of individual products and dishes, taking into account their GI: green - dishes with a GI of less than 20, yellow - from 20 to 69, red - 70 and higher. Usually, a GI below 55 is considered to be «low» (15). However, foods with a GI slightly below 55 can also induce a high PPGR if consumed in substantial amounts. For the purpose of our study, we marked by green color the foods which are likely not to increase the level of glycemia in any amounts consumed.
The application contains teaching materials including general information about GDM, nutrition principles for patients with GDM, information about the GI of foods, dietary fibers and their importance in the diet, and recommendations on physical activity.
The application also contains an alert system in the form of pop-up messages on a woman’s device with a reminder of the insufficient number of diary records entered for the previous days, a reminder to send a diary to the doctor, and generates reports for the doctor.
DiaCompanion I is represented by two mobile versions developed for Android and iOS devices, as well as a cross-platform web version. The implementation features of the Android and iOS applications also allow them to be used on computers with preset Java Runtime Machine. The experience gained in developing the web version influenced the choice of tools for creating a server to exchange information between different user devices within the same account.
The app contains plenty of screens with a wide range of functions, including the main menu, user input forms, record management and information, user settings, data export form, and help. Some of these screens are presented in Figure 3.
Figure 3 Screenshots of main views in the DiaCompanion I app. (A) a view of food intake data before entering planned food items and current blood glucose level. (B) an example of a meal with a high level of predicted postprandial blood glucose. (C) an example of a meal with a level of predicted postprandial blood glucose within targets.
The built-in food database, collected from open sources (including the Scientific Research Institute of Nutrition of the Russian Academy of Medical Sciences and the USDA food databases) enables tracking 27 food parameters (macronutrients and micronutrients). Additionally, glycaemic indexes were assigned to all food items in the food database using a standardized protocol (6).
Patients can add their own dishes to the app by composing them from the ingredients presented in the built-in database. The automatic algorithm calculates their nutrient profile.
The app allows the users to export data in Excel spreadsheets and store them on their devices as well as send them remotely to physicians. An example of a standardized report exported from the app is shown in Figure 4.
Figure 4 Examples of standardized reports exported from the app. (A) = a spreadsheet with glucose levels measured by a glucometer. (B) = an example of a spreadsheet with food intake records, including food items (dishes), their weights, macronutrient contents, GI and glycaemic loads.
To synchronize data between patient devices, implement authorization and automatically save user data, a web application for the server based on the Django framework was developed.
Patient data in this system is stored on the servers of the Almazov National Medical Research Centre and is automatically updated every week by downloading data from the patient’s devices. This approach protects patient data by TLS encryption and by avoiding weakly protected communication channels like email. Moreover, the system protects data from unintentional deletion of record history.
Participants in the intervention group are required to enter all food intakes into the app. They are also required to enter blood glucose measurements before main food intakes (breakfast, dinner and lunch) to enable the prediction of postprandial BG level. In case of a high predicted BG they are encouraged to change the amount and/or content of the planned meal in a manner that will lead to a predicted BG within target range.
Women in the intervention group are recommended to send their electronic diaries (standardized reports) to their endocrinologists every week in order to get feedback. The doctors check the food records and glucose levels and send back recommendations on dietary corrections if necessary. In case of revealing indications for insulin therapy, the women are appointed to an office visit within a few days.
The participants of the intervention group have only two scheduled visits to endocrinologists during pregnancy (at baseline and on the 35-37th gestational week (GW)) if they are included after the 20th GW. Those included before the 20th GW are also invited at the 24th GW. Additional visits are appointed in case of the appearance of indications to insulin treatment (repeated fasting capillary glucose of ≥5.1mmol/L or a 1-hour postprandial value of ≥7.0 mmol/L during 1-2 weeks). Two or more abnormal values during 1-2 weeks of monitoring are required to commence insulin treatment according to Russian national guidelines (16).
Women in the control group will have a standard of care without a mobile app. According to Russian national guidelines, women diagnosed with GDM receive guidance on diet, physical activity and self-monitoring of BG with capillary glucose meters. They are required to self-record their fasting, pre-prandial and 1-hour postprandial BG values and they are also advised to self-record their food intakes in a paper diary at home. They are attending the maternity clinic every 2-3 weeks where an endocrinologist reviews their readings and diary. In case of repeated fasting capillary glucose of ≥5.1mmol/L or a 1-hour postprandial value of ≥7.0 mmol/L during 1-2 weeks or one reading above 9.0 mmol/L at any time of day, participants are instructed to call the clinic and schedule an additional visit to reconsider indications for insulin treatment.
Primary Outcome is the percentage of postprandial capillary glucose values above target (>7.0 mmol/L) in the time frame from randomization up to delivery. The women will be asked to perform 6 measures a day. Capillary glucose values will be retrieved from the glucose meter, and if not available, from the woman’s diary.
Secondary outcome measures are:
1. Rate of patients requiring insulin therapy during pregnancy
⚬ Rate of patients requiring insulin therapy (either basal or prandial)
⚬ Rate of patients requiring prandial insulin therapy
2. Capillary glucose values in the following time frames: within 2 weeks after randomization , at gestational weeks 35-37, and in the period from randomization up to delivery.
⚬ Capillary fasting glucose values, mmol/L
⚬ Capillary preprandial glucose values, mmol/L
⚬ Capillary postprandial glucose values, mmol/L
3. The proportion of women with glucose values within target in the following time frames: within 2 weeks after randomization, at gestational weeks 35-37, and in the period from randomization up to delivery.
⚬ The proportion of women with fasting glucose values within the target
⚬ The proportion of women with postprandial glucose values within the target
4. Mean incremental area under the blood glucose curve 2 hours after meals (iAUC120) according to 7-day continuous glucose monitoring 1-2 weeks after randomization and at the 35-37th GW.
5. Hypoglycaemia
⚬ Severe hypoglycaemia: requiring the assistance of another person to actively correct the level of glycaemia and neurological symptoms
⚬ Documented symptomatic hypoglycaemia: event during which typical symptoms of hypoglycaemia are accompanied by a measured capillary glucose concentration <3.9 mmol/L
⚬ Asymptomatic hypoglycaemia: event not accompanied by typical symptoms of hypoglycaemia but with a measured capillary glucose concentration <3.3 mmol/L
6. Maternal metabolic parameters at 35-37th GW (the level of HbA1c, Fasting insulin, Fasting glucose, HOMA-IR index, serum triglyceride levels).
7. Gestational weight change by the time of delivery
⚬ Gestational weight change during pregnancy, kg
⚬ Gestational weight change between inclusion and delivery, kg
8. Large (>90th percentile) for gestational age infant
9. Small for gestational age (< 10th percentile) infant
10. Birth weight, kg
11. Birth weight ≥ 4000 g; ≥ 4500 g (yes/no)
12. Neonatal hypoglycaemia defined as glucose levels <2,2 mmol/L in the first 4 hours of life or <2,5 mmol/L at 4–24 hours of age (yes/no) (17).
13. Shoulder dystocia (yes/no)
14. Birth injury, any of the following: plexus injury, clavicle, humeral, or skull fracture (yes/no)
15. Preterm delivery (yes/no)
16. Apgar score at 1 and 5 minutes from birth
17. Low Apgar score: 5-min Apgar score < 7 (yes/no)
18. Jaundice requiring phototherapy (yes/no)
19. Neonatal respiratory distress syndrome (yes/no)
20. Admission to neonatal intensive care unit during the three days following birth (yes/no)
21. Umbilical cord blood C-peptide, ng/mL
22. Cesarean delivery rate
23. Induction of labor rate
24. Need for operative vaginal delivery rate (forceps or vacuum-assisted vaginal delivery)
25. Preeclampsia (blood pressure ≥ 140/90 mmHg on two measurements four hours apart and proteinuria of at least 300 mg/24 hours or 3+ or more on dipstick testing or proteinuria/creatinuria >30 in a random urine sample)
26. Pregnancy-induced hypertension in women with no known hypertension before pregnancy (blood pressure ≥ 140/90 mmHg on two measurements four hours apart without proteinuria, and required anti-hypertensive therapy)
27. The number of in-patient visits to endocrinologists in the time frame from randomization to delivery.
28. Results of oral glucose tolerance test 3 months postpartum.
29. Acceptance/satisfaction of 2 strategies at 35-36th GW (Evaluation of the patient satisfaction with their treatment for GDM with a scale: give a score of 0 to 100: 0 not satisfied; 100 totally satisfied).
30. Patient satisfaction evaluated through a questionnaire at 35-36th GW.
We will use a 10-question questionnaire with multiple-choice questions, questions with numeric answers on a scale from 0 (fully unsatisfied) to 10 (fully satisfied), and open-ended questions.
The timetable of the planned visits and measurements is shown in Table 2.
The analyses will be performed on the intention-to-treat (ITT) basis, including all randomized patients. We will also perform a per-protocol analysis excluding women who did not use the DiaCompanion I app for at least 50% of expected meals to explore the efficacy of the system under optimal use.
Quantitative data will be assessed for normality using the Kolmogorov-Smirnov test. Log transformation could be used where appropriate. The data will be presented as mean and SD for normally distributed parameters and as median with quartiles for non-normally distributed characteristics. The distribution of binary or ordinary characteristics will be described as N (%).
The significance of differences in scale parameters (such as the percentage of postprandial capillary glucose values above target, the number of in-patient visits, etc.) between the control and intervention groups will be assessed by the Student’s t-test for normally distributed ones and Mann-Whitney test for ordinal and non-normally distributed characteristics. To compare the distribution of binary or ordinary characteristics (ex., rate of patients requiring insulin therapy during pregnancy, LGA, SGA, etc.) the χ2 criterion or Fisher’s exact test will be used. The Spearman correlation coefficient will be used to assess the relationship between two variables. We will use ANOVA repeated or nonparametric analogue for the analysis of repeated measurements (gestational weight change). The critical level of significance of the null statistical hypothesis will be assumed to be 0.05.
The sample size for the RCT was calculated as the number of participants needed to test the statistical significance of the difference between the two means (reduction in mean percent of BG readings exceeding target glycaemic levels from 15 to 11%, SD 10%) at a significance level of 0.05 and with a power of 0.80 for d/SD values = 0.4, including 25% dropout.
Our mobile-based recommendation system DiaCompanion I differentiates from existing GDM apps by the ability to predict postprandial BG level at the time of food intake planning and by providing real-time personalized recommendations on the ways of hyperglycemia prevention.
There are published and ongoing trials of the effect of personalized diets by prediction of glycaemic responses on glycaemic control in prediabetes and type 2 diabetes mellitus. However, these analogues are not intended for pregnant women (18, 19).
The authors of a recent review investigating the awareness and use of the smartphone applications (app) with respect to management of GDM concluded that mobile apps provided personalized health care services, patient care improvement, and enhanced patient’s compliance toward blood glucose monitoring and treatment (20). A relatively large RCT by Mackillop et al. (21) reported less preterm births and fewer cesarean deliveries despite the fact that there was no significant change in BG levels between the control and the intervention groups.
In another scoping review, the authors investigated mHealth apps intended for use with GDM and concluded that there were limited mHealth apps for GDM that incorporated AI or AI-based decision support (22).
It is well known that learning how to manage the results of self- tracking and adhering to a diet requires efforts from women with GDM, as associations between lifestyle, nutrition and PPGR are complex and difficult to identify, especially during the first weeks after GDM diagnosis (23). As DiaCompanion I provides recommendations at each time when a high BG is predicted, we expect the most significant difference in glucose control parameters within the first weeks after GDM diagnosis.
Our system does not consist of an app only, as participants in the intervention group are supposed to generate diary reports from the app weekly and send it to their physicians. Using this approach, we substitute most of in-person communications with a feedback based on diary analysis by means of return messages. We believe that the combination of automated feedback from the app before each meal intake with weekly feedback from a healthcare professional will further increase the efficacy of the intervention while reducing the number of in-patients visits. Apart from reducing the burden on health care facilities, we expect a reduction in total time spent for patients communicating by endocrinologists or other health care providers dealing with BG and diet control in patients with GDM.
However, this approach also poses challenges. It is difficult to differentiate the influence from automated recommendations based on PPGR prediction and the influence of weekly medical feedback. Another limitation of our study is the lack of BG prediction and insulin doses adjustment for patients treated with insulin. Women requiring insulin will be recorded and followed until delivery but they will not be able to use the prediction function. It is supposed to work incorrectly in such patients because we have not included insulin treated women in the population used for the development of PPGR prediction models. It is a task for our future research to develop such models.
The study protocol was first posted on January 5, 2022, and enrollment has started on January 12, 2022. The estimated primary completion date is January 2025.
The studies involving human participants were reviewed and approved by Ethics committee of the Almazov National Medical Research Centre. The patients/participants provided their written informed consent to participate in this study.
Conceptualization: PP, ES. Methodology: PP, EP, TP and EG. Investigation: AT, EV, AA, KP, NK, IN, MK and OL. Resources: TP. Data curation: AT, AA and EV. Writing—original draft preparation: PP and AE. Writing—review and editing: PP, EG, and TP. Statistical analysis: PP, AI, AD, AE. Supervision: EG and ES. Project administration: PP. Funding acquisition: ES. All authors contributed to the article and approved the submitted version.
This work was financially supported by the Ministry of Science and Higher Education of the Russian Federation (Agreement No. 075-15-2022-301).
The authors declare that the research was conducted in the absence of any commercial or financial relationships that could be construed as a potential conflict of interest.
All claims expressed in this article are solely those of the authors and do not necessarily represent those of their affiliated organizations, or those of the publisher, the editors and the reviewers. Any product that may be evaluated in this article, or claim that may be made by its manufacturer, is not guaranteed or endorsed by the publisher.
1. Sacks DA, Coustan DR. Frequency of gestational diabetes mellitus at collaborating centers based on IADPSG consensus panel–recommended criteria. Diabetes Care (2012) 35:526–8. doi: 10.2337/dc11-1641
2. HAPO Study Cooperative Research Group, Metzger BE, Lowe LP, Dyer AR. Elisabeth R Trimble. Hyperglycemia Adverse Pregnancy Outcomes. N Engl J Med (2008) 358:1991–2002. doi: 10.1056/NEJMoa0707943
3. Ben-Haroush A, Yogev Y, Hod M. Epidemiology of gestational diabetes mellitus and its association with type 2 diabetes. Diabetes Med (2004) 21:103–13. doi: 10.1046/j.1464-5491.2003.00985.x
4. Hajj NE, Schneider E, Lehnen H, Haaf T. Epigenetics and life-long consequences of an adverse nutritional and diabetic intrauterine environment. Reproduction (2014) 148(6):R111–20. doi: 10.1530/REP-14-0334
5. Popova P, Castorino K, Grineva EN, Kerr D. Gestational diabetes mellitus diagnosis and treatment goals: measurement and measures. Minerva Endocrinol (2016) 41(4):421–32.
6. Pustozerov E, Tkachuk A, Vasukova E, Dronova A, Shilova E, Anopova A, et al. The role of glycemic index and glycemic load in the development of real-time postprandial glycemic response prediction models for patients with gestational diabetes. Nutrients (2020) 12:302. doi: 10.3390/nu12020302
7. Pustozerov EA, Tkachuk AS, Vasukova EA, Anopova AD, Kokina MA, Gorelova IV, et al. Machine learning approach for postprandial blood glucose prediction in gestational diabetes mellitus. IEEE Access (2020) 8:219308–21. doi: 10.1109/ACCESS.2020.3042483
8. Pustozerov E, Popova P, Tkachuk A, Bolotko Y, Yuldashev Z, Grineva E. Development and evaluation of a mobile personalized blood glucose prediction system for patients with gestational diabetes mellitus. JMIR MHealth UHealth (2018) 6:e6. doi: 10.2196/mhealth.9236
9. Xie W, Dai P, Qin Y, Wu M, Yang B, Yu X. Effectiveness of telemedicine for pregnant women with gestational diabetes mellitus: an updated meta-analysis of 32 randomized controlled trials with trial sequential analysis. BMC Pregnancy Childbirth (2020) 20:198. doi: 10.1186/s12884-020-02892-1
10. Lu H, Ding X, Hirst JE, Yang Y, Yang J, MacKillop L, et al. Digital health and machine learning technologies for blood glucose monitoring and management of gestational diabetes. TechRxiv (2023). doi: 10.36227/techrxiv.21919254.v1
11. Chan A-W, Tetzlaff JM, Gøtzsche PC, Altman DG, Mann H, Berlin JA, et al. SPIRIT 2013 explanation and elaboration: guidance for protocols of clinical trials. BMJ (2013) 346:e7586. doi: 10.1136/bmj.e7586
12. Uschner D, Schindler D, Hilgers R-D, Heussen N. randomizeR: an r package for the assessment and implementation of randomization in clinical trials. J Stat Softw (2018) 85(8):1–22. doi: 10.18637/jss.v085.i08
13. Pustozerov EA, Yuldashev ZM, Popova PV, Bolotko YA, Tkachuk AS. Information support system for patients with gestational diabetes mellitus. BioMed Eng (2018) 51:407–10. doi: 10.1007/s10527-018-9759-2
14. Pustozerov E, Popova P. Mobile-based decision support system for gestational diabetes mellitus. In: 2018 Ural symposium on biomedical engineering, radioelectronics and information technology (USBEREIT). Yekaterinburg: IEEE (2018). p. 45–8. doi: 10.1109/USBEREIT.2018.8384546
15. International Standards Organisation. ISO/FDIS 26642:2010. food products – determination of the glycemic index (GI) and recommendation for food classification. Geneva, Switzerland: ISO (2010).
16. Dedov II, Krasnopol’skiy VI, Sukhikh GT. Russian National consensus statement on gestational diabetes: diagnostics, treatment and postnatal care. Diabetes mellitus (2012) 4:4–10. doi: 10.14341/2072-0351-5531
17. Adamkin DH. Committee on fetus and newborn. postnatal glucose homeostasis in late-preterm and term infants. Pediatrics (2011) 127:e20103851. doi: 10.1542/peds.2010-3851
18. Ben-Yacov O, Godneva A, Rein M, Shilo S, Kolobkov D, Koren N, et al. Personalized postprandial glucose response-targeting diet versus Mediterranean diet for glycemic control in prediabetes. Diabetes Care (2021) 44(9):1980–91. doi: 10.2337/dc21-0162
19. Rein M, Ben-Yacov O, Godneva A, Shilo S, Zmora N, Kolobkov D, et al. Effects of personalized diets by prediction of glycemic responses on glycemic control and metabolic health in newly diagnosed T2DM: a randomized dietary intervention pilot trial. BMC Med (2022) 20:56. doi: 10.1186/s12916-022-02254-y
20. Garg N, Arunan SK, Arora S, Kaur K. Application of mobile technology for disease and treatment monitoring of gestational diabetes mellitus among pregnant women: a systematic review. J Diabetes Sci Technol (2022) 16:491–7. doi: 10.1177/1932296820965577
21. Mackillop L, Hirst JE, Bartlett KJ, Birks JS, Clifton L, Farmer AJ, et al. Comparing the efficacy of a mobile phone-based blood glucose management system with standard clinic care in women with gestational diabetes: randomized controlled trial. JMIR Mhealth Uhealth (2018) 6:e71. doi: 10.2196/mhealth.9512
22. Daley BJ, Ni’Man M, Neves MR, Bobby Huda MS, Marsh W, Fenton NE, et al. mHealth apps for gestational diabetes mellitus that provide clinical decision support or artificial intelligence: a scoping review. Diabetes Med (2022) 39:e14735. doi: 10.1111/dme.14735
Keywords: gestational diabetes mellitus, postprandial glycaemic response prediction, personalized nutrition, mHealth, trial protocol
Citation: Popova P, Anopova A, Vasukova E, Isakov A, Eriskovskaya A, Degilevich A, Pustozerov E, Tkachuk A, Pashkova K, Krasnova N, Kokina M, Nemykina I, Pervunina T, Li O, Grineva E and Shlyakhto E (2023) Trial protocol for the study of recommendation system DiaCompanion with personalized dietary recommendations for women with gestational diabetes mellitus (DiaCompanion I). Front. Endocrinol. 14:1168688. doi: 10.3389/fendo.2023.1168688
Received: 17 February 2023; Accepted: 27 April 2023;
Published: 07 June 2023.
Edited by:
Elena Succurro, University of Magna Graecia, ItalyReviewed by:
Luigi Fontana, The University of Sydney, AustraliaCopyright © 2023 Popova, Anopova, Vasukova, Isakov, Eriskovskaya, Degilevich, Pustozerov, Tkachuk, Pashkova, Krasnova, Kokina, Nemykina, Pervunina, Li, Grineva and Shlyakhto. This is an open-access article distributed under the terms of the Creative Commons Attribution License (CC BY). The use, distribution or reproduction in other forums is permitted, provided the original author(s) and the copyright owner(s) are credited and that the original publication in this journal is cited, in accordance with accepted academic practice. No use, distribution or reproduction is permitted which does not comply with these terms.
*Correspondence: Polina Popova, cHZwb3BvdmFAeWFuZGV4LnJ1
Disclaimer: All claims expressed in this article are solely those of the authors and do not necessarily represent those of their affiliated organizations, or those of the publisher, the editors and the reviewers. Any product that may be evaluated in this article or claim that may be made by its manufacturer is not guaranteed or endorsed by the publisher.
Research integrity at Frontiers
Learn more about the work of our research integrity team to safeguard the quality of each article we publish.