- 1Department of Psychiatry, and National Clinical Research Center for Mental Disorders, The Second Xiangya Hospital of Central South University, Changsha, Hunan, China
- 2Department of Psychiatry, First Hospital of Shanxi Medical University, Taiyuan, China
- 3Chinese Academy of Science (CAS) Key Laboratory of Mental Health, Institute of Psychology, Chinese Academy of Sciences, Beijing, China
- 4Department of Psychology, University of Chinese Academy of Sciences, Beijing, China
Backgrounds: Co-occurrence of thyroid dysfunction, metabolic disturbances, and worsening clinical symptoms in major depressive disorder (MDD) patients with suicidal attempts (SA) are common. However, their relationship in SA patients remains unexplored. We aimed to (1) determine the independent association of thyroid dysfunction, clinical symptoms, and metabolic disturbances with SA; and (2) identify their interactions in SA patients via the network approach.
Methods: 1718 FEDN MDD patients were recruited. Depressive, anxiety, and psychotic symptoms were assessed by the Hamilton Rating Scale for Depression (HAMD), the Hamilton Rating Scale for Anxiety (HAMA), and the Positive and Negative Syndrome Subscale positive subscale, respectively. The serum levels of thyroid hormones and other metabolic parameters were assessed. Logistic regression model was applied to determine the correlates of SA. Network analysis was applied to determine the interaction between thyroid dysfunction, clinical symptoms, and metabolic disturbances.
Results: SA patients had significant worse metabolic disturbances, thyroid dysfunction, and clinical symptoms than non-SA patients. Thyroid peroxidases antibody, thyroid stimulating hormone (TSH), HAMD scores, HAMA scores, and systolic blood pressure was independently associated with SA. Network analysis suggested that TSH was the hub of the network, exhibiting substantial associations with metabolic disturbances, anxiety, and psychotic symptoms in SA patients.
Conclusions: Our work highlights the predominant role of serum TSH levels in the pathophysiology of SA. Regular thyroid function tests might help early detect SA. Targeting increased TSH levels may help reduce metabolic disturbances and clinical symptoms in SA patients.
Introduction
Suicide is the most devastating consequence of patients with major depressive disorder (MDD). The lifetime prevalence of suicidal ideation, suicidal planning, and suicidal attempts (SA) in MDD patients is 37.7%, 15.1%, and 23.7%, respectively (1, 2). A recent meta-analysis shows that MDD patients are approximately 7 times more likely to have SA in the past year than healthy individuals (3). The high prevalence of SA highlights the strong need to identify the potential risk factors for SA, which is valuable for SA screening and intervening in MDD patients.
Although numerous studies have identified demographic and clinical risk factors for SA in MDD patients (4, 5), the biological correlates of SA remain largely unexplored (5). Several studies have suggested that thyroid hormones and metabolic parameters can be potential biomarkers for SA (6–9). However, the reported results are inconsistent. For example, one meta-analysis published in 2020 demonstrated that SA was associated with low serum levels of total cholesterol (TC) and low-density lipoprotein cholesterol (LDL-C) in 7068 patients with MDD (7). However, this view has been challenged by two recent large-scale studies (N=1279 and N=580) (10, 11), which found higher concentrations of TC and LDL-C in MDD patients with SA. Similarly, the relationship between thyroid dysfunction and SA in patients with MDD was also in debate. Several studies indicated that elevated TSH increased the risk of suicide (11), whilst some found an inverse association (12) or no association (13). The inconsistency in the previous studies may be due to the different study samples. Studies have shown that disease duration, comorbidities, and medication may have a substantial impact on thyroid function and metabolism (14–16), which may obscure their association with SA. Therefore, assessing the relationship between thyroid dysfunction, metabolic disturbances, and SA in first-episode drug-naïve (FEND) MDD patients may provide more solid evidence.
Studies have confirmed that the co-occurrence of thyroid dysfunction and metabolic disturbances is very common (17–20), especially in patients with MDD (21). For example, Kim et al. found that subclinical hypothyroidism increased the risk of metabolic syndrome by 7 times among individuals with depression (21). Two recent studies also found that MDD patients with SA exhibited more severe clinical symptoms, metabolic disturbances, and thyroid dysfunction than those without (11, 22). However, no prior study directly evaluated their relationship in patients with SA. Clarifying whether and how thyroid dysfunction, metabolic disorders, and clinical symptoms are interconnected in patients with SA may provide new insights into the pathophysiology of SA.
Network analysis, as an emerging tool, has advantages over traditional methods such as regression models in visualizing and describing independent associations between variables (23, 24). In a network model, a variable is visualized as a “node”. After sufficient adjustment for other variables within the network, the unique association between two variables is visualized as an “edge”. In addition to identifying correlations between variables, network analysis identifies the most influential variables that are most closely linked to the other variables in the network (i.e., central variables). The central variable is considered to play an important role in triggering and maintaining the network (25). Hence, the central variable may be a promising target for clinical interventions to reduce thyroid dysfunction, metabolic disturbances, and clinical symptoms in MDD patients with SA.
To date, emerging studies have applied network analysis to assess associations between variables in clinical medicine (26, 27). For example, Jia et al. have assessed the association of lipid markers with cognition performance and depression through a network approach (27). A recent study also determined the networks of lipid metabolism, inflammation, and depressive symptoms (26). However, there are no previous studies evaluating the network of clinical symptoms, thyroid dysfunction, and metabolic disturbance in MDD patients with SA, which gave us the motivation to conduct the present study. We recruited a large sample of FEDN MDD patients and evaluated SA, metabolic parameters, thyroid hormones, and clinical symptoms. We have two main aims (1): to determine the association of SA with clinical symptoms, metabolic disturbances, and thyroid dysfunction in first-episode drug-naïve patients with MDD; and (2) to determine the inter-relationship between metabolic disturbances, thyroid dysfunction, and clinical symptoms in patients with SA via the network approach.
Methods
Study procedure and participants
Participants were recruited at the psychiatric outpatient department of the First Hospital of Shanxi Medical University from 2015 to 2017. Inclusion criteria were as follows: (1) fulfilling DSM-IV criteria for MDD, diagnosed by two trained psychiatrists using the Structured Clinical Interview for DSM-IV Disorders (SCID); (2) 17-item Hamilton Depression Scale (HAMD) score of more than 23; (3) age 18-60 years old, Han nationality; (4) no prior medication, including antidepressant, antipsychotic drugs, thyroid hormone therapy, hypoglycemic agents, antihypertensive and lipid-lowering drugs; and (5) depression symptoms were first-episode and the disease duration of no more than 24 months. Exclusion criteria included: (1) pregnant or breastfeeding women; (2) concurrent DSM-IV axis I disorder including bipolar disorder, schizophrenia, and schizoaffective or severe medical conditions; (3) substance use disorder except for tobacco; and (4) unwillingness to provide informed consent.
All participants provided written informed consent. This study was approved by the Institutional Review Board (IRB) of the First Hospital of Shanxi Medical University (No. 2016-Y27).
Interview and clinical assessments
We collected basic information, including age, gender, education, onset, and duration of MDD, and married status through a self-designed questionnaire. All participants were independently interviewed face-to-face by two trained psychiatrists via the SCID. Two psychiatrists independently assessed each participant’s depression, anxiety, and psychotic symptoms by the HAMD, Hamilton Anxiety Scale (HAMA), and the positive subscale of Positive and Negative Syndrome Subscale (PANSS), respectively. HAMD score ranges from 0-52, with a cutoff point of 24 being used to determine severe depression (28). HAMA consists of 14 items, measuring psychological and somatic anxiety symptoms (29). It applied the 5-Likert scale, with a total score ranging from 0-56. The PANSS positive subscale assesses seven positive symptoms (30). The PANSS-positive subscale score ranges from 7-49. Higher scores on the HAMA, HAMD, and PANSS indicate more severe symptoms. These three scales have been validated and widely used in the Chinese population (31–33). According to previous studies (34, 35), HAMA score >20 and PANSS positive subscale score >14 indicate significant anxiety and psychotic symptoms, respectively. The correlation coefficients between the two psychiatrists’ scores on all three scales were higher than 0.8.
We assessed SA through face-face interviews. All participants were asked the question: “In your lifetime, did you ever try to kill yourself?”. This single item has been validated and used widely in previous epidemiological studies for the detection of SA (36, 37). Those who answered “yes” were considered to have lifetime SA. We further asked them about the timing and frequency of SA. We contacted the family members of the participants for the details of SA when patients were unable to provide definitive information.
Biochemical indicators
Blood samples were collected in the morning after an overnight fast before participants received any medical treatment. Serum levels of free triiodothyronine (FT3), free thyroxine (FT4), thyroid stimulating hormone (TSH), antithyroglobulin (TgAb), thyroid peroxidase antibody (TPOAb), TC, TG, high-density lipoprotein (HDL-C), low-density lipoprotein (LDL-C), and glucose were assessed. Lipid markers (TC, TG, HDL-C, LDL-C) and glucose were measured on a Cobas E610 (Roche, Basel, Switzerland). Thyroid hormones were assayed on a Roche C6000 Electrochemiluminescence Immunoassay Analyzer (Roche Diagnostics, Indianapolis, IN, USA). Measurements were conducted in the laboratory of the First Hospital, Shanxi Medical University. The nurses measured the patients’ weight, height, and blood pressure. We calculated body mass index (BMI) according to the following formula: BMI = Weight (kg)/Height (m) 2.
According to previous studies in the Chinese population (38, 39), metabolic disturbances and thyroid dysfunction were defined as follows: (1) overweight or obesity: BMI≥24; (2) hyperglycemia: glucose≥6.1mmol/L; (3) hypertension: SBP≥140 mmHg and/or DBP≥90mmHg; (4) hypertriglyceridemia: TG≥2.3 mmol/L; (5) low HDL: HDL-C ≤ 1.0 mmol/L; (6) hypercholesterolemia: TC≥6.2 mmol/L or LDL-C≥4.1 mmol/L; (7)abnormal TgAb: TgAb≥115 IU/L; (8) abnormal TPOAb: TPOAb ≥34 IU/L; (9) subclinical hypothyroidism (SCH): TSH >4.2 mIU/L with normal fT4 concentration (10–23 pmol/L); (10) hyperthyroidism: TSH<0.27 mIU/L and FT4 >23 pmol/L, and (11) hypothyroidism: TSH >4.2 mIU/L with low FT4 concentration (<10 pmol/L).
Statistical analysis
Data processing
According to the Shapiro-Wilk test, the continuous data in our study were not normally distributed. Therefore, we expressed the continuous data as the median and interquartile range (IRQ; 25-75%) and the categorical data as frequencies and percentages. All statistical analyses were conducted on R (ver. 4.20). We adopt two-tailed tests with p<0.05 indicating statistical significance.
Univariate and multiple analyses
We assessed differences in metabolic disturbances, clinical symptoms, and thyroid dysfunction between MDD patients with and without SA by chi-square test, Fisher’s exact test, and Whitney U test, as appropriate. Bonferroni correction was employed for multiple testing (p’=0.05/40 = 0.00125). A multiple logistic regression model was conducted to identify independent correlates of SA. Variables with P < 0.05 in univariate tests were included in the multiple logistic regression analysis using the stepwise method.
Network analysis
Clinical symptoms (HAMA, HAMD, and PANSS scores), metabolic parameters (TC, TG, LDL-C, HDL-C, SBP, DBP, and BMI), and thyroid hormones (TSH, TPOAb, TgAb, FT3, and FT4) were included in the network. Following a previous study (40), we performed nonparanormal transformations using Rpackage “huge” because the data were not normally distributed. We estimated and visualized the network using Rpackage “qgraph” and “bootnet” (41). We estimated the network using the default of the EBICglasso model, which was widely used in psychological network models (42).γ was set to 0.5, which made the network more sparse and strikes a balance between sensitivity and specificity in preserving true edges. The network consisted of “nodes” (i.e., metabolic parameters, thyroid function, and clinical symptoms) and “edges” (i.e., pairwise correlations between two nodes after controlling for other variables within the network). Thicker edges implied a greater association (43). Red edges indicated negative associations, while blue edges indicated positive associations. We calculated the centrality index “strength” to quantify the importance of the nodes. Nodes with higher strength were considered to exhibit strong associations and impacts on other nodes within the network. We also calculated the predictability of the nodes by Rpackage “MGM” (44). Similar to the R2 in the regression model, predictability referred to the extent to which the variance of a node can be explained by other nodes in the network (45).
Finally, we evaluated the stability and accuracy of our network by Rpackage “bootnet”. Bootstrap procedures were performed with 1000 bootstrap samples to determine the accuracy of the estimated edges. We conducted a case-dropping procedure to evaluate the stability of the network. The correlation stability coefficient (CS-C) was calculated, and a CS-C above 0.5 implied reasonable stability.
Results
Sample characteristics
We recruited 1718 FEDN MDD patients (Table 1). The majority of the participants were female (1130, 66%), married (1216, 71%), and had a degree below college (1173, 68%). One-fifth of the participants (346, 20%) had lifetime SA. 235 (14%) had SA in the past two weeks.
The difference in metabolic disturbances, thyroid function, and clinical symptoms in FEDN MDD patients with and without SA
SA patients tended to be older, had a longer duration of disease, and had a later onset (Table 1). Compared with non-SA patients, SA patients had significantly more severe metabolic disturbances, thyroid dysfunction, and psychological distress than non-SA patients, showing higher scores on HAMD, HAMA, and PANSS positive subscale. The prevalence rates of SCH, abnormal TgAb, abnormal TPOAb, hyperglycemia, abnormal TC, abnormal LDL-C, low HDL, hypertension, and hypercholesterolemia were significantly higher in SA patients than in non-SA patients. Their associations remained significant after the Bonferroni correction. In addition, SA patients were also more likely to have hypertriglyceridemia. However, the association between hypertriglyceridemia and SA was no longer significant after multiple testing.
Independent correlates of SA in FEDN MDD patients
We conducted a multiple logistic regression model in variables showing p<0.05 in univariate analysis (i.e., age, duration, and the onset of MDD, HAMD, HAMA, PANSS, TSH, TPOAb, TgAb, TC, TG, HDL-C, LDL-C, glucose, SBP, and DBP). Table 2 summarizes the results of the logistic regression model. HAMD (Odds ratio, OR, 1.081, 95% confidence intervals, 95% CI, 1.016-1.151, p=0.014), HAMA (OR, 1.251, 95%CI, 1.189-1.316, p<0.001), TSH (OR, 1.115, 95%CI, 1.047-1.187, p=0.001), TPOAb (OR, 1.002, 95%CI, 1.001-1.003, p<0.001), and SBP (OR, 1.023, 95%CI, 1.008-1.038, p=0.002) were independently associated with SA in FEDN MDD patients.
Network of thyroid dysfunction, metabolic disturbances, and clinical symptoms in MDD patients with SA
Figure 1 illustrates the network of thyroid dysfunction, metabolic disturbances, and clinical profiles in MDD patients with SA. The network was composed of 16 nodes and 32 edges. Visually, TSH was in the center of the network. It exhibited a strong positive association with metabolic parameters including SBP, TC, and glucose. TSH was also positively correlated with PANSS and HAMA. In contrast, BMI, FT3, and FT4 were at the margin of the network, exhibiting a very weak association with clinical symptoms. We also observed a strong association between PANSS, HAMA, and HAMD. The correlation matrix between the nodes is presented in Table S1.
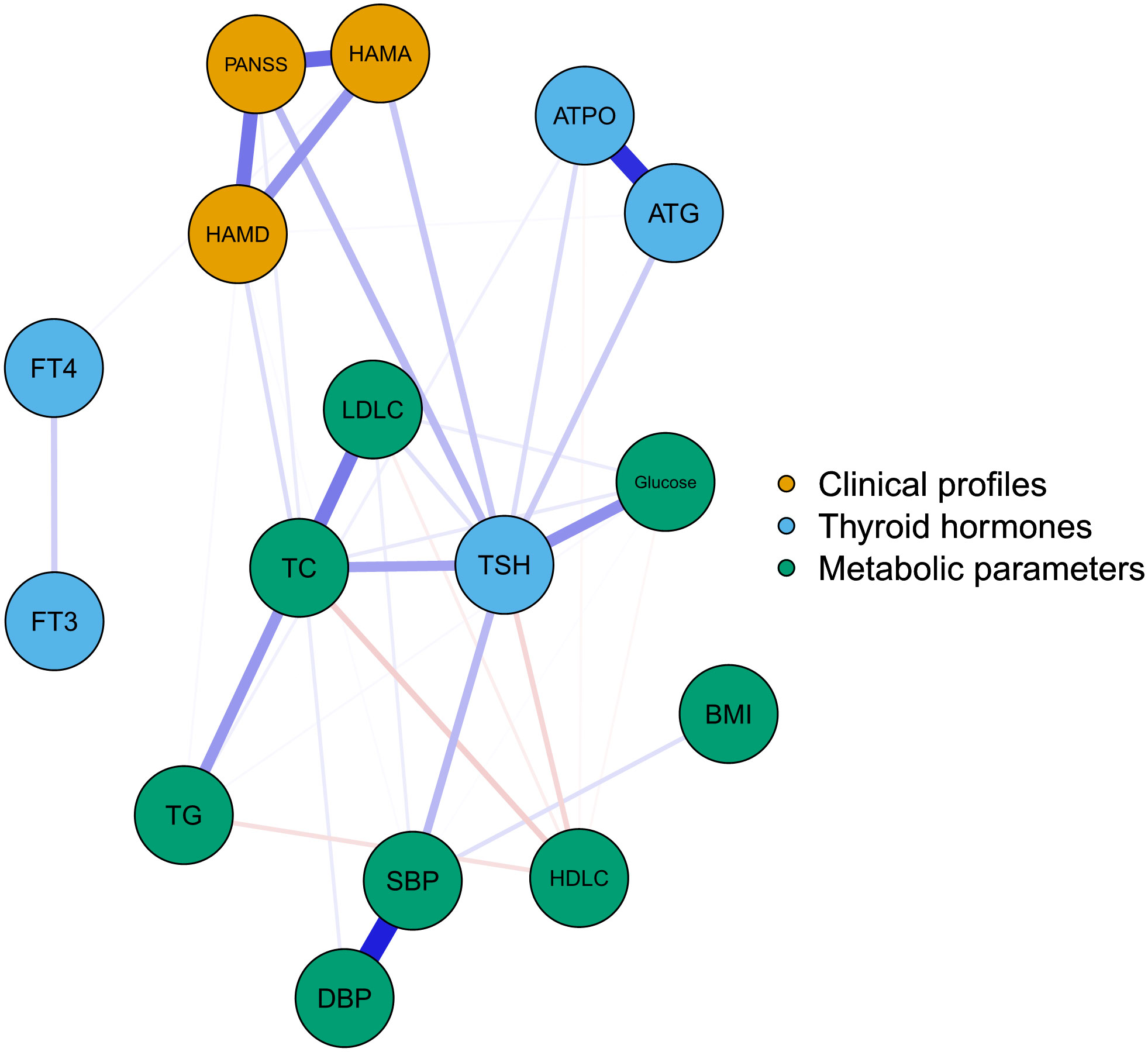
Figure 1 The network of thyroid-dysfunction, metabolic disturbances, and clinical symptoms in FEDN MDD patients with suicidal attempts. Blue, orange, and green nodes represented thyroid hormones, clinical symptoms, and metabolic parameters, respectively. Blue and red edges indicated positive and negative associations, respectively. Thicker edges suggested stronger associations. HAMD = Hamilton Depression Rating Scale, HAMA = Hamilton Anxiety Rating Scale, PANSS = the Positive and Negative Syndrome Scale, TSH = thyroid-stimulating hormone, FT3 = free triiodothyronine, FT4 = free thyroxine, TgAb = antithyroglobulin, TPOAb = thyroid peroxidases antibody, TC = total cholesterol, HDL-C = high-density lipoprotein, LDL-C = low-density lipoprotein, TG = total triglycerides, BMI = body mass index.
The centrality plot (Figure 2) confirmed that TSH was the central node of the network, followed by TC and PANSS scores. Table S2 displays the predictability of the nodes in the network. The predictability of TSH was the highest (0.57). The predictability of clinical symptoms was 0.53 for PANSS, 0.50 for HAMA, and 0.45 for HAMD. These results indicated that half of the variance of clinical symptoms could be explained by the nodes in the network. The lowest predictability was found for FT3, FT4, and BMI.
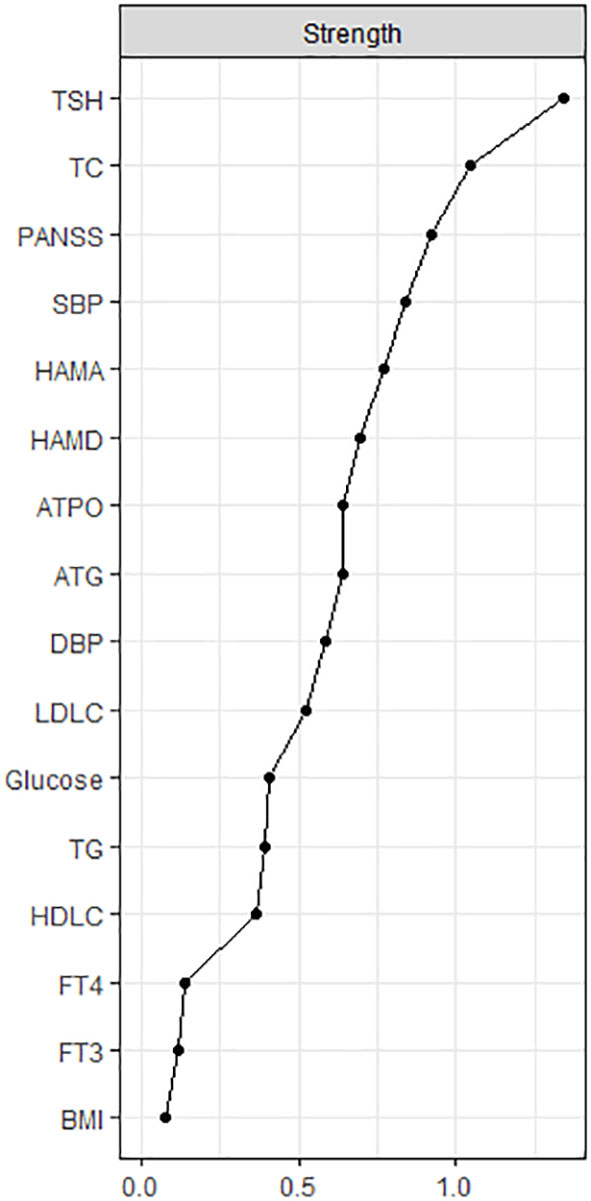
Figure 2 The centrality plot of the network. The X-rays represented the strength of each node. Nodes with higher strength have stronger impact in other nodes within the network. HAMD = Hamilton Depression Rating Scale, HAMA = Hamilton Anxiety Rating Scale, PANSS = the Positive and Negative Syndrome Scale, TSH = thyroid-stimulating hormone, FT3 = free triiodothyronine, FT4 = free thyroxine, TgAb = antithyroglobulin, TPOAb = thyroid peroxidases antibody, TC = total cholesterol, HDL-C = high-density lipoprotein, LDL-C = low-density lipoprotein, TG = total triglycerides, BMI = body mass index.
The network had reasonable stability with a value of 0.671 for CS-C (Figure S1), indicating that after omitting 67% of the raw data, the network remained highly correlated with the original network (r=0.7). The bootstrap procedure also demonstrated high accuracy of the estimated edges within the network (Figure S2).
Discussion
To our knowledge, this is the first study to explore the relationship between thyroid dysfunction, metabolic disturbances, and clinical symptoms in SA patients through a network approach. Our main findings included (1): SA MDD patients exhibited more severe metabolic disturbances, thyroid dysfunction, and clinical symptoms compared to non-SA MDD patients; (2) the severity of anxiety and depression symptoms, SBP, TSH, and TPOAb were independently associated with SA in FEDN MDD patients; and (3) TSH played an important role in the network of thyroid dysfunction, metabolic disturbances, and clinical symptoms in SA patients. Taken together, our work highlights the predominant role of serum TSH levels in the pathophysiology of SA. In addition to being a potential biomarker for SA in MDD patients, the serum TSH level is closely associated with SA-related metabolic disturbances and clinical symptoms. Hence, regular thyroid function tests might help early detect SA. Targeting increased TSH levels may help to reduce metabolic disturbances and clinical symptoms in MDD patients with SA.
Consistent with previous studies (46–48), our study demonstrated a very high metabolic burden and thyroid dysfunction in patients with SA, which called for regular metabolic and thyroid function tests in this population. There are a few explanations for the biological changes in SA patients. First, SA patients have more severe depressive symptoms, which may lead to an unhealthy lifestyle, such as irregular sleep and diet, resulting in metabolic disturbances and thyroid dysfunction (49). Second, inflammation may act as a bridge between SA and metabolic disorders. Emerging studies have found that inflammation plays an important role in MDD and its associated SA (50–54). Metabolic disorders were found to be associated with a chronic inflammatory state (55) and therefore may contribute to SA. Third, thyroid dysfunction was tightly associated with abnormal neurotransmitters (e.g., 5-hydroxytryptamine and norepinephrine), which played an important role in SA (56). Fourth, the high level of TPOAb might indicate the autoimmune status of MDD patients with SA. The disturbances in the kynurenine pathway and hypothalamic-pituitary-adrenal axis in autoimmune status might contribute to the SA (57).
Network analysis suggested that thyroid dysfunction, metabolic disturbance, and clinical symptoms were highly correlated among SA patients. High TSH levels were found to be the central variables within the network, which were tightly associated with both metabolic disturbances (impaired glucose metabolism, lipid metabolism, and hypertension) and clinical symptoms (psychotic and anxiety symptoms) in SA patients. The strong association of TSH with metabolic disturbances replicates findings in the general population (17), which can be explained by the following points. First, serum TSH levels can regulate lipid metabolism in various ways (58). High TSH levels can regulate cholesterol metabolism by binding to TSH receptors on the surface of hepatocytes (59). It can accelerate cholesterol synthesis and reduce cholesterol clearance (58), which can lead to dyslipidemia and obesity. Second, TSH levels may also play a role in insulin resistance and glucose tolerance (60). Studies have shown that high TSH levels are associated with the impairment of glucose transport (61).
Emerging studies suggested that thyroid dysfunction could predict several negative consequences in patients with MDD, including long-term readmission, conversion to bipolar disorder, and anxiety (62–64). However, most of these association was observed in the context of overt hypothyroidism. The relationship between SCH and clinical symptoms in patients with MDD remained controversial. Meta-analysis suggested that SCH exhibited a rather weak association with depressive symptoms (65). One population-based study demonstrated a negative association of TSH levels with anxiety (66). Interestingly, Liu et al. reported the same results as ours (11), finding higher serum TSH levels were associated with anxiety and psychosis among 1279 patients with MDD. The different results may be due to differences in sample characteristics (MDD patients versus community samples). Unfortunately, the relationship between SCH and clinical symptoms in patients with MDD was mostly studied in the cross-sectional study. The biological mechanism remained largely unexplored. Further studies are needed to validate our findings and to assess the possible mechanisms.
Our study has several important clinical implications. First, our study showed a high prevalence of metabolic disorders, thyroid dysfunction, anxiety, and psychotic symptoms in MDD patients with SA. Therefore, screening for these problems is crucial in this particular population. Second, our study suggested the severity of anxiety and depression, TSH level, TPOAb level, and SBP were independently associated with SA. Regular monitoring of these clinical variables might help early detect and prevent SA. Third, our study highlighted the predominant role of TSH in the pathophysiology of SA. Targeting TSH may be valuable in reducing metabolic disorders, clinical symptoms, and thyroid dysfunction associated with SA. To date, a few studies have shown that thyroid hormone therapy is effective in improving lipid metabolism in patients with SCH (67). Some studies have also documented its effectiveness in the treatment of MDD and bipolar depression (68, 69), but the results are inconsistent (70, 71). Therefore, more studies are in need to test our hypothesis.
Our study has several limitations. First, we used a cross-sectional study design, which prevented us from drawing causal relationships. Second, this study is monocentric and includes only the Han Chinese population. It remains unknown whether our findings can be generalized to other populations. Third, we did not collect several important sociocultural risk factors for SA, such as stressful life events and economic hardship (5). In addition, we did not collect lifestyle factors, such as smoking and exercise, as well as diet, which are strongly associated with metabolic disturbances and thyroid dysfunction, and this should be remedied in future studies. Fourth, our study is mainly descriptive and the underlying biological mechanisms are unknown. Fifth, we assessed suicide attempts by a single item only. Application of a specific suicide rating scale may better assess various aspects of suicidality (suicidal ideation, suicide planning, and SA) and their relationship with clinical symptoms, metabolic disturbances, and thyroid dysfunction. Further longitudinal studies with a more comprehensive assessment of confounding factors and suicidality are needed to validate our findings.
In conclusion, our study demonstrates that MDD patients with SA have severe thyroid dysfunction, metabolic disturbances, and clinical symptoms. Anxiety, depression, TSH, TPOAb, and SBP were independently associated with SA in FEDN MDD patients. Targeting increased TSH in MDD patients with SA may help reduce metabolic disturbances, clinical symptoms, and thyroid dysfunction in SA patients.
Data availability statement
The raw data supporting the conclusions of this article will be made available by the authors, without undue reservation.
Ethics statement
All participants provided written informed consent. This study was approved by the Institutional Review Board (IRB) of the First Hospital of Shanxi Medical University (No. 2016-Y27). The patients/participants provided their written informed consent to participate in this study.
Author contributions
PP, formal analysis, writing - original draft. QW and XL, writing – review and editing. TL and X-YZ, conceptualization, writing–review and editing. All authors contributed to the article and approved the submitted version.
Funding
This study was supported by the Provincial Natural Science Foundation of Hunan (Grant No. 2020JJ4795 to TL). These sources had no further role in this study design, in the data collection and analysis, in the writing of the report, and in the decision to submit the paper for publication.
Acknowledgments
We would like to acknowledge all the participants.
Conflict of interest
The authors declare that the research was conducted in the absence of any commercial or financial relationships that could be construed as a potential conflict of interest.
Publisher’s note
All claims expressed in this article are solely those of the authors and do not necessarily represent those of their affiliated organizations, or those of the publisher, the editors and the reviewers. Any product that may be evaluated in this article, or claim that may be made by its manufacturer, is not guaranteed or endorsed by the publisher.
Supplementary material
The Supplementary Material for this article can be found online at: https://www.frontiersin.org/articles/10.3389/fendo.2023.1136806/full#supplementary-material
References
1. Cai H, Jin Y, Liu S, Zhang Q, Zhang L, Cheung T, et al. Prevalence of suicidal ideation and planning in patients with major depressive disorder: A meta-analysis of observation studies. J Affect Disord (2021) 293:148–58. doi: 10.1016/j.jad.2021.05.115
2. Dong M, Wang S-B, Li Y, Xu D-D, Ungvari GS, Ng CH, et al. Prevalence of suicidal behaviors in patients with major depressive disorder in China: A comprehensive meta-analysis. J Affect Disord (2018) 225:32–9. doi: 10.1016/j.jad.2017.07.043
3. Cai H, Xie X-M, Zhang Q, Cui X, Lin J-X, Sim K, et al. Prevalence of suicidality in major depressive disorder: A systematic review and meta-analysis of comparative studies. Front Psychiatry (2021) 12:690130. doi: 10.3389/fpsyt.2021.690130
4. Lin J-Y, Huang Y, Su Y-A, Yu X, Lyu X-Z, Liu Q, et al. Association between perceived stressfulness of stressful life events and the suicidal risk in Chinese patients with major depressive disorder. Chin Med J (Engl) (2018) 131:912–9. doi: 10.4103/0366-6999.229898
5. Li X, Mu F, Liu D, Zhu J, Yue S, Liu M, et al. Predictors of suicidal ideation, suicide attempt and suicide death among people with major depressive disorder: A systematic review and meta-analysis of cohort studies. J Affect Disord (2022) 302:332–51. doi: 10.1016/j.jad.2022.01.103
6. Fu X-L, Li X, Ji J-M, Wu H, Chen H-L. Blood hormones and suicidal behaviour: A systematic review and meta-analysis. Neurosci Biobehav Rev (2022) 139:104725. doi: 10.1016/j.neubiorev.2022.104725
7. Li H, Zhang X, Sun Q, Zou R, Li Z, Liu S. Association between serum lipid concentrations and attempted suicide in patients with major depressive disorder: A meta-analysis. PloS One (2020) 15:e0243847. doi: 10.1371/journal.pone.0243847
8. Zhang Q, Zhao S, Liu Z, Luo B, Yang Y, Shi Y, et al. Association of thyroid-stimulating hormone and lipid levels with suicide attempts among adolescents with major depressive disorder in China. Front Psychiatry (2022) 13:1031945. doi: 10.3389/fpsyt.2022.1031945
9. Wu S, Ding Y, Wu F, Xie G, Hou J, Mao P. Serum lipid levels and suicidality: A meta-analysis of 65 epidemiological studies. J Psychiatry Neurosci (2016) 41:56–69. doi: 10.1503/jpn.150079
10. Zhou S, Zhao K, Shi X, Sun H, Du S, Miao X, et al. Serum lipid levels and suicide attempts within 2 weeks in patients with major depressive disorder: Is there a relationship? Front Psychiatry (2021) 12:676040. doi: 10.3389/fpsyt.2021.676040
11. Liu W, Wu Z, Sun M, Zhang S, Yuan J, Zhu D, et al. Association between fasting blood glucose and thyroid stimulating hormones and suicidal tendency and disease severity in patients with major depressive disorder. Bosn J Basic Med Sci (2022), 22(4):635-42. doi: 10.17305/bjbms.2021.6754
12. Peng R, Dai W, Li Y. Low serum free thyroxine level is correlated with lipid profile in depressive patients with suicide attempt. Psychiatry Res (2018) 266:111–5. doi: 10.1016/j.psychres.2018.05.059
13. Ma Y-J, Wang D-F, Yuan M, Zhang X-J, Long J, Chen S-B, et al. The prevalence, metabolic disturbances and clinical correlates of recent suicide attempts in Chinese inpatients with major depressive disorder. BMC Psychiatry (2019) 19:144. doi: 10.1186/s12888-019-2131-6
14. Bou Khalil R, Richa S. Thyroid adverse effects of psychotropic drugs: a review. Clin Neuropharmacol (2011) 34:248–55. doi: 10.1097/WNF.0b013e31823429a7
15. Gehlawat P, Gupta R, Rajput R, Gahlan D, Gehlawat VK. Diabetes with comorbid depression: role of SSRI in better glycemic control. Asian J Psychiatr (2013) 6:364–8. doi: 10.1016/j.ajp.2013.03.007
16. Köhler-Forsberg O, Otte C, Gold SM, Østergaard SD. Statins in the treatment of depression: Hype or hope? Pharmacol Ther (2020) 215:107625. doi: 10.1016/j.pharmthera.2020.107625
17. Liu F-H, Hwang J-S, Kuo C-F, Ko Y-S, Chen S-T, Lin J-D. Subclinical hypothyroidism and metabolic risk factors association: A health examination-based study in northern Taiwan. BioMed J (2018) 41:52–8. doi: 10.1016/j.bj.2018.02.002
18. Li R-C, Zhang L, Luo H, Lei Y, Zeng L, Zhu J, et al. Subclinical hypothyroidism and anxiety may contribute to metabolic syndrome in sichuan of China: A hospital-based population study. Sci Rep (2020) 10:2261. doi: 10.1038/s41598-020-58973-w
19. Ding X, Zhao Y, Zhu C-Y, Wu L-P, Wang Y, Peng Z-Y, et al. The association between subclinical hypothyroidism and metabolic syndrome: an update meta-analysis of observational studies. Endocr J (2021) 68:1043–56. doi: 10.1507/endocrj.EJ20-0796
20. Nakajima Y, Yamada M, Akuzawa M, Ishii S, Masamura Y, Satoh T, et al. Subclinical hypothyroidism and indices for metabolic syndrome in Japanese women: one-year follow-up study. J Clin Endocrinol Metab (2013) 98:3280–7. doi: 10.1210/jc.2013-1353
21. Kim M-D, Yang H-J, Kang NR, Park JH, Jung Y-E. Association between subclinical hypothyroidism and metabolic syndrome among individuals with depression. J Affect Disord (2020) 264:494–7. doi: 10.1016/j.jad.2019.11.080
22. Liu F, Huang L, Zhu R, Peng C, Deng X. The thyroid dysfunction of suicide attempts in major depression. Annales Médico-psychologiques Rev psychiatrique (2021) 179:329–33. doi: 10.1016/j.amp.2020.12.022
23. McInerney AM, Lindekilde N, Nouwen A, Schmitz N, Deschênes SS. Diabetes distress, depressive symptoms, and anxiety symptoms in people with type 2 diabetes: A network analysis approach to understanding comorbidity. Diabetes Care (2022), 45(8):1715–23. doi: 10.2337/dc21-2297
24. Borsboom D, Deserno MK, Rhemtulla M, Epskamp S, Fried EI, McNally RJ, et al. Network analysis of multivariate data in psychological science. Nat Rev Methods Primers (2021) 1:1–18. doi: 10.1038/s43586-021-00055-w
25. McNally RJ. Can network analysis transform psychopathology? Behav Res Ther (2016) 86:95–104. doi: 10.1016/j.brat.2016.06.006
26. Zainal NH, Newman MG. Prospective network analysis of proinflammatory proteins, lipid markers, and depression components in midlife community women. Psychol Med (2022), 1–12. doi: 10.1017/S003329172200232X
27. Jia Q-F, Yang H-X, Zhuang N-N, Yin X-Y, Zhu Z-H, Yuan Y, et al. The role of lipoprotein profile in depression and cognitive performance: a network analysis. Sci Rep (2020) 10:20704. doi: 10.1038/s41598-020-77782-9
28. Zimmerman M, Martinez JH, Young D, Chelminski I, Dalrymple K. Severity classification on the Hamilton depression rating scale. J Affect Disord (2013) 150:384–8. doi: 10.1016/j.jad.2013.04.028
29. Thompson E. Hamilton Rating scale for anxiety (HAM-a). Occup Med (Lond) (2015) 65:601. doi: 10.1093/occmed/kqv054
30. Kay SR, Fiszbein A, Opler LA. The positive and negative syndrome scale (PANSS) for schizophrenia. Schizophr Bull (1987) 13:261–76. doi: 10.1093/schbul/13.2.261
31. Duan Y, Wei J, Geng W, Jiang J, Zhao X, Li T, et al. Research on cognitive function in anxious depression patients in China. J Affect Disord (2021) 280:121–6. doi: 10.1016/j.jad.2020.11.078
32. Li X, Liu H, Hou R, Baldwin DS, Li R, Cui K, et al. Prevalence, clinical correlates and IQ of suicidal ideation in drug naïve Chinese han patients with major depressive disorder. J Affect Disord (2019) 248:59–64. doi: 10.1016/j.jad.2018.12.017
33. Li Z, Xue M, Zhao L, Zhou Y, Wu X, Xie X, et al. Comorbid major depression in first-episode drug-naïve patients with schizophrenia: Analysis of the depression in schizophrenia in China (DISC) study. J Affect Disord (2021) 294:33–8. doi: 10.1016/j.jad.2021.06.075
34. Peng P, Wang Q, Ren H, Zhou Y, Hao Y, Chen S, et al. Association between thyroid hormones and comorbid psychotic symptoms in patients with first-episode and drug-naïve major depressive disorder. Psychiatry Res (2023), 320:115052. doi: 10.1016/j.psychres.2023.115052
35. Zhou Y, Wang Q, Ren H, Yang WFZ, Ma Y, Wu Q, et al. Prevalence and related factors of anxiety in first episode and drug naïve Chinese han outpatients with psychotic major depression. J Affect Disord (2022) 316:217–22. doi: 10.1016/j.jad.2022.08.018
36. Shen Y, Chan BSM, Liu J, Meng F, Yang T, He Y, et al. Estimated prevalence and associated risk factors of attention deficit hyperactivity disorder (ADHD) among medical college students in a Chinese population. J Affect Disord (2018) 241:291–6. doi: 10.1016/j.jad.2018.08.038
37. Li Y-C, Bai W, Cai H, Wu Y, Zhang L, Ding Y-H, et al. Suicidality in clinically stable bipolar disorder and schizophrenia patients during the COVID-19 pandemic. Transl Psychiatry (2022) 12:303. doi: 10.1038/s41398-022-02045-2
38. He J, Lai Y, Yang J, Yao Y, Li Y, Teng W, et al. The relationship between thyroid function and metabolic syndrome and its components: A cross-sectional study in a Chinese population. Front Endocrinol (Lausanne) (2021) 12:661160. doi: 10.3389/fendo.2021.661160
39. Liu Y, Sheng C, Feng W, Sun F, Zhang J, Chen Y, et al. A multi-center study on glucometabolic response to bariatric surgery for different subtypes of obesity. Front Endocrinol (Lausanne) (2022) 13:989202. doi: 10.3389/fendo.2022.989202
40. Skjerdingstad N, Johnson MS, Johnson SU, Hoffart A, Ebrahimi OV. Feelings of worthlessness links depressive symptoms and parental stress: A network analysis during the COVID-19 pandemic. Eur Psychiatry (2021) 64:e50. doi: 10.1192/j.eurpsy.2021.2223
41. Epskamp S, Borsboom D, Fried EI. Estimating psychological networks and their accuracy: A tutorial paper. Behav Res (2018) 50:195–212. doi: 10.3758/s13428-017-0862-1
42. Peng P, Liang M, Wang Q, Lu L, Wu Q, Chen Q. Night shifts, insomnia, anxiety, and depression among Chinese nurses during the COVID-19 pandemic remission period: A network approach. Front Public Health (2022) 10:1040298. doi: 10.3389/fpubh.2022.1040298
43. Burger J, Isvoranu A-M, Lunansky G, Haslbeck JMB, Epskamp S, Hoekstra RHA, et al. Reporting standards for psychological network analyses in cross-sectional data. psychol Methods (2022). doi: 10.1037/met0000471
44. Bai W, Xi H-T, Zhu Q, Ji M, Zhang H, Yang B-X, et al. Network analysis of anxiety and depressive symptoms among nursing students during the COVID-19 pandemic. J Affect Disord (2021) 294:753–60. doi: 10.1016/j.jad.2021.07.072
45. Ren L, Wang Y, Wu L, Wei Z, Cui L-B, Wei X, et al. Network structure of depression and anxiety symptoms in Chinese female nursing students. BMC Psychiatry (2021) 21:279. doi: 10.1186/s12888-021-03276-1
46. Zhao K, Zhou S, Shi X, Chen J, Zhang Y, Fan K, et al. Potential metabolic monitoring indicators of suicide attempts in first episode and drug naive young patients with major depressive disorder: a cross-sectional study. BMC Psychiatry (2020) 20:387. doi: 10.1186/s12888-020-02791-x
47. Chang J-C, Yen AM-F, Lee C-S, Chen SL-S, Chiu SY-H, Fann JC-Y, et al. Metabolic syndrome and the risk of suicide: a community-based integrated screening samples cohort study. Psychosom Med (2013) 75:807–14. doi: 10.1097/PSY.0000000000000014
48. Ko J-K, Han K-M, Shin C, Lee S-H, Han C, Kim Y-K, et al. Association of metabolic syndrome and its components with suicidal ideation and depression in adults: A nationally representative sample of the Korean population. J Affect Disord (2019) 249:319–26. doi: 10.1016/j.jad.2019.02.049
49. Marazziti D, Rutigliano G, Baroni S, Landi P, Dell’Osso L. Metabolic syndrome and major depression. CNS Spectr (2014) 19:293–304. doi: 10.1017/S1092852913000667
50. Beurel E, Toups M, Nemeroff CB. The bidirectional relationship of depression and inflammation: Double trouble. Neuron (2020) 107:234–56. doi: 10.1016/j.neuron.2020.06.002
51. Dolsen MR, Prather AA, Lamers F, Penninx BWJH. Suicidal ideation and suicide attempts: associations with sleep duration, insomnia, and inflammation. Psychol Med (2021), 51(12):2094–2103. doi: 10.1017/S0033291720000860
52. Fernández-Sevillano J, González-Ortega I, MacDowell K, Zorrilla I, López MP, Courtet P, et al. Inflammation biomarkers in suicide attempts and their relation to abuse, global functioning and cognition. World J Biol Psychiatry (2022), 23(4):307–17. doi: 10.1080/15622975.2021.1988703
53. Cáceda R, Griffin WST, Delgado PL. A probe in the connection between inflammation, cognition and suicide. J Psychopharmacol (2018) 32:482–8. doi: 10.1177/0269881118764022
54. Kappelmann N, Arloth J, Georgakis MK, Czamara D, Rost N, Ligthart S, et al. Dissecting the association between inflammation, metabolic dysregulation, and specific depressive symptoms: A genetic correlation and 2-sample mendelian randomization study. JAMA Psychiatry (2021) 78:161–70. doi: 10.1001/jamapsychiatry.2020.3436
55. Chan KL, Cathomas F, Russo SJ. Central and peripheral inflammation link metabolic syndrome and major depressive disorder. Physiol (Bethesda) (2019) 34:123–33. doi: 10.1152/physiol.00047.2018
56. Toloza FJK, Mao Y, Menon L, George G, Borikar M, Thumma S, et al. Association of thyroid function with suicidal behavior: A systematic review and meta-analysis. Medicina (Kaunas) (2021) 57:714. doi: 10.3390/medicina57070714
57. Brundin L, Bryleva EY, Thirtamara Rajamani K. Role of inflammation in suicide: From mechanisms to treatment. Neuropsychopharmacology (2017) 42:271–83. doi: 10.1038/npp.2016.116
58. Liu H, Peng D. Update on dyslipidemia in hypothyroidism: The mechanism of dyslipidemia in hypothyroidism. Endocr Connect (2022) 11:e210002. doi: 10.1530/EC-21-0002
59. Zhang W, Tian L, Han Y, Ma H, Wang L, Guo J, et al. Presence of thyrotropin receptor in hepatocytes: not a case of illegitimate transcription. J Cell Mol Med (2009) 13:4636. doi: 10.1111/j.1582-4934.2008.00670.x
60. Chaker L, Ligthart S, Korevaar TIM, Hofman A, Franco OH, Peeters RP, et al. Thyroid function and risk of type 2 diabetes: A population-based prospective cohort study. BMC Med (2016) 14:150. doi: 10.1186/s12916-016-0693-4
61. Maratou E, Hadjidakis DJ, Kollias A, Tsegka K, Peppa M, Alevizaki M, et al. Studies of insulin resistance in patients with clinical and subclinical hypothyroidism. Eur J Endocrinol (2009) 160:785–90. doi: 10.1530/EJE-08-0797
62. Yang L, Yang X, Yang T, Wu X, Sun P, Zhu Y, et al. The effect of thyroid function on the risk of psychiatric readmission after hospitalization for major depressive disorder. Psychiatry Res (2021) 305:114205. doi: 10.1016/j.psychres.2021.114205
63. Niu Z, Wu X, Zhu Y, Yang L, Shi Y, Wang Y, et al. Early diagnosis of bipolar disorder coming soon: Application of an oxidative stress injury biomarker (BIOS) model. Neurosci Bull (2022) 38:979–91. doi: 10.1007/s12264-022-00871-4
64. Zhu Y, Wu X, Zhou R, Sie O, Niu Z, Wang F, et al. Hypothalamic-Pituitary-End-Organ axes: Hormone function in female patients with major depressive disorder. Neurosci Bull (2021) 37:1176–87. doi: 10.1007/s12264-021-00689-6
65. Bode H, Ivens B, Bschor T, Schwarzer G, Henssler J, Baethge C. Association of hypothyroidism and clinical depression: A systematic review and meta-analysis. JAMA Psychiatry (2021) 78:1375–83. doi: 10.1001/jamapsychiatry.2021.2506
66. Panicker V, Evans J, Bjøro T, Åsvold BO, Dayan CM, Bjerkeset O. A paradoxical difference in relationship between anxiety, depression and thyroid function in subjects on and not on T4: findings from the HUNT study. Clin Endocrinol (2009) 71:574–80. doi: 10.1111/j.1365-2265.2008.03521.x
67. Zhao C, Wang Y, Xiao L, Li L. Effect of levothyroxine on older patients with subclinical hypothyroidism: A systematic review and meta-analysis. Front Endocrinol (Lausanne) (2022) 13:913749. doi: 10.3389/fendo.2022.913749
68. George S, Maiti R, Mishra A, Ranjan Mishra B, Jena M. Efficacy and safety of supraphysiologic doses of levothyroxine for patients with bipolar depression in adults: A systematic review. J Psychopharmacol (2022) 36:521–30. doi: 10.1177/02698811221078758
69. Bauer M, Whybrow PC. Role of thyroid hormone therapy in depressive disorders. J Endocrinol Invest (2021) 44:2341–7. doi: 10.1007/s40618-021-01600-w
70. Zhao T, Chen BM, Zhao XM, Shan ZY. Subclinical hypothyroidism and depression: A meta-analysis. Transl Psychiatry (2018) 8:239. doi: 10.1038/s41398-018-0283-7
71. Wildisen L, Feller M, Del Giovane C, Moutzouri E, Du Puy RS, Mooijaart SP, et al. Effect of levothyroxine therapy on the development of depressive symptoms in older adults with subclinical hypothyroidism: An ancillary study of a randomized clinical trial. JAMA Netw Open (2021) 4:e2036645. doi: 10.1001/jamanetworkopen.2020.36645
Keywords: suicidal attempt, major depressive disorder, network analysis, thyroid stimulating hormone, metabolic disturbances
Citation: Peng P, Wang Q, Lang X, Liu T and Zhang X-Y (2023) Clinical symptoms, thyroid dysfunction, and metabolic disturbances in first-episode drug-naïve major depressive disorder patients with suicide attempts: A network perspective. Front. Endocrinol. 14:1136806. doi: 10.3389/fendo.2023.1136806
Received: 03 January 2023; Accepted: 16 February 2023;
Published: 24 February 2023.
Edited by:
Daniel Elías-López, Instituto Nacional de Ciencias Médicas y Nutrición Salvador Zubirán (INCMNSZ), MexicoReviewed by:
Qinghua Luo, First Affiliated Hospital of Chongqing Medical University, ChinaFengchun Wu, The Affiliated Brain Hospital of Guangzhou Medical University, China
Jiang Du, Shanghai Jiao Tong University, China
Yiru Fang, Shanghai Jiao Tong University, China
Copyright © 2023 Peng, Wang, Lang, Liu and Zhang. This is an open-access article distributed under the terms of the Creative Commons Attribution License (CC BY). The use, distribution or reproduction in other forums is permitted, provided the original author(s) and the copyright owner(s) are credited and that the original publication in this journal is cited, in accordance with accepted academic practice. No use, distribution or reproduction is permitted which does not comply with these terms.
*Correspondence: Tieqiao Liu, liutieqiao123@csu.edu.cn; Xiang-Yang Zhang, zhangxy@psych.ac.cn