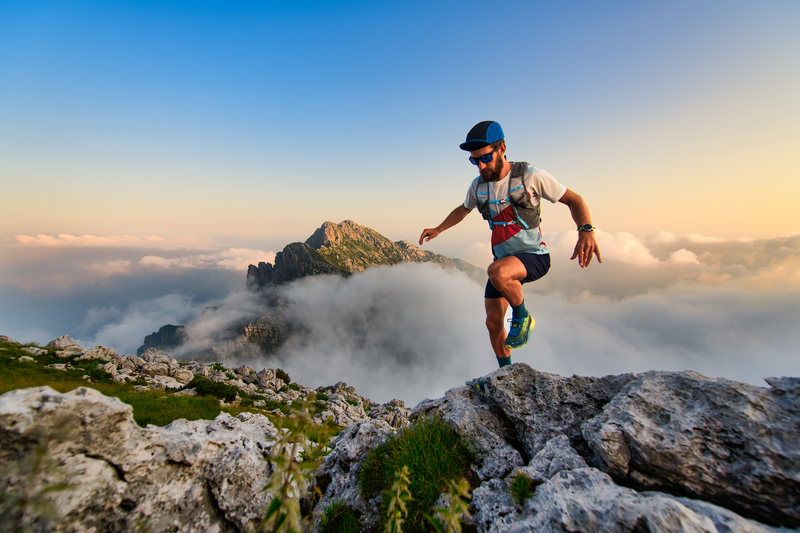
95% of researchers rate our articles as excellent or good
Learn more about the work of our research integrity team to safeguard the quality of each article we publish.
Find out more
ORIGINAL RESEARCH article
Front. Endocrinol. , 01 March 2023
Sec. Cancer Endocrinology
Volume 14 - 2023 | https://doi.org/10.3389/fendo.2023.1131767
This article is part of the Research Topic Substance and Energy Metabolism Associated with Neuroendocrine Regulation in Tumor Cells View all 41 articles
Background: It is well known that the occurrence and development of ovarian cancer are closely related to the patient’s weight and various endocrine factors in the body.
Aim: Mendelian randomization (MR) was used to analyze the bidirectional relationship between insulin related characteristics and ovarian cancer.
Methods: The data on insulin related characteristics are from up to 5567 diabetes free patients from 10 studies, mainly including fasting insulin level, insulin secretion rate, peak insulin response, etc. For ovarian cancer, UK Biobank data just updated in 2021 was selected, of which the relevant gene data was from 199741 Europeans. Mendelian randomization method was selected, with inverse variance weighting (IVW) as the main estimation, while MR Pleiotropy, MR Egger, weighted median and other methods were used to detect the heterogeneity of data and whether there was multi validity affecting conclusions.
Results: Among all insulin related indicators (fasting insulin level, insulin secretion rate, peak insulin response), the insulin secretion rate was selected to have a causal relationship with the occurrence of ovarian cancer (IVW, P < 0.05), that is, the risk of ovarian cancer increased with the decrease of insulin secretion rate. At the same time, we tested the heterogeneity and polymorphism of this indicator, and the results were non-existent, which ensured the accuracy of the analysis results. Reverse causal analysis showed that there was no causal effect between the two (P>0.05).
Conclusion: The impairment of the insulin secretion rate has a causal effect on the risk of ovarian cancer, which was confirmed by Mendel randomization. This suggests that the human glucose metabolism cycle represented by insulin secretion plays an important role in the pathogenesis of ovarian cancer, which provides a new idea for preventing the release of ovarian cancer.
Ovarian cancer is a kind of cancer that occurs in female ovarian tissue. There are many pathological subtypes, among which high-grade serous ovarian cancer (1) is the most common. In developed countries, ovarian cancer is the main cause of death among all gynecological cancers (2). Due to the lack of specific signs and symptoms at the early stages of the disease, ovarian cancer is usually found in late stage, with extensive peritoneal (Phase III) or extraperitoneal (Phase IV) spread. Tumor reduction surgery and platinum and taxane drug chemotherapy could make 75% of patients achieve clinical remission. At present, the 5-year survival rate of ovarian cancer patients is roughly less than 30% (3–5). There are many risk factors for ovarian cancer, including age, reproductive history, changeable lifestyle factors, family history and gene mutation (6).
Insulin (7) is the main regulator of glucose, lipid and protein metabolism. When oral glucose load or mixed meal is ingested, plasma glucose concentration increases, and islets of β Cells are stimulated to secrete insulin. Insulin can inhibit the production of endogenous glucose (the main target organ is the liver), stimulate the uptake and storage of glucose by muscle, liver and fat cells, and inhibit the decomposition of fat, leading to a decrease in plasma free fatty acids concentration (8), which helps to inhibit the production of glucose in the liver and increase the uptake of glucose in the muscle, and can relax muscle vessels, which helps to enhance muscle glucose disposal.
The incidence rate of various cancers is higher in patients with insulin secretion disorder (especially in patients with type 2 diabetes). Many studies and observations in the field of overseas studies have confirmed this view. It is reported that among patients with type 2 diabetes, the relative risk of endometrial cancer, liver cancer and pancreatic cancer is more than 2 times, while the relative risk of bladder cancer, breast cancer and colorectal cancer is as high as 1.5 times (9–12). In addition to the increase in incidence rate, the overall mortality rate of diabetes patients when diagnosed with cancer is higher (13) than that of the non-disease group. Systematic reviews (14, 15) could suggest that overweight people have a higher risk of ovarian cancer, and the risk of ovarian cancer increases with obesity. The increase and abnormality of obesity or body mass index often could lead to the disorder of endocrine system in the human body, such as insulin resistance, estrogen level change and other characteristics, which are factors that cannot be ignored in the role of obesity factors in weight related cancer.
Therefore, it is important to understand the hormone specific relationship between metabolism and cancer (16). In this paper, bidirectional mendelian randomization analysis was used to confirm the causal relationship between insulin related characteristics and ovarian cancer risk.
This study included six insulin related indicators from three studies, including Fasting blood insulin, Fasting blood insulin adjusted for BMI, Insulin secret rate, Peak insulin response, Acute insulin response and Insulin disposition index. The specific description of relevant data can be shown in Table 1.
The GWAS data (17) came from Genome wide association studies for fast glucose (FG) and fast insulin (FI), which analyzed the exon array data of 33231 non-diabetes patients of European origin. The data and SNP of fasting insulin came from this study.
The data of this indicator (18) came from a study of “Genome wide method considering body mass index determines genetic variation affecting fasting blood glucose characteristics and insulin resistance”, which includes 96496 non diabetes patients. The fasting insulin data here were adjusted by body mass index.
This study explored the genome-wide association study based on IVGTT’s first phase insulin secretion measurement, which refined the potential physiology of type 2 diabetes variation. Insulin secretion rate (ISR) is the estimated insulin secretion rate (ISR) (19) based on the measured serum C-peptide concentration at 0, 2, 4, 6, 8 and 0, 2, 3, 4, 5, 6, 7, 8, 10, 12, 14, 16 and 19 (FAMILY) minutes. ISEC software (20) is used to calculate the secretion rate according to predefined C-peptide kinetic parameters, including each person’s weight, height, age Gender and clinical status (glucose tolerance and obesity status) were determined in a population-based study (21). The ISR provides an estimate of the rate of insulin secretion before hepatic insulin clearance.
Peak insulin response was measured as peak insulin minus baseline insulin. Determine the peak insulin time point of each study according to the time point when the average insulin value of all individuals is the highest.
The acute insulin response (AIR) was measured as the incremental area under the insulin curve in the first 10 minutes. Or if the 10 minutes measurement is not available, the minimum insulin value at 0, 2, 4, 6 and 8 minutes during the IVGTT using the trapezoidal equation during the first 8 minutes. Incremental insulin was calculated by subtracting fasting insulin levels.
Insulin disposition index was calculated as the product of AIR, and insulin sensitivity index was calculated by the MINMOD (22), which took into account the level of background insulin resistance.
Through IEU Open GWAS (MR Base) (23) public database(https://gwas.mrcieu.ac.uk/)to retrieve and obtain data on ovarian cancer. The data of ovarian cancer patients are from UK Biobank. According to the data, the latest update is 2021, which includes 9822229 SNPs from 199741 Europeans. The classification of data is binary data, that is, whether ovarian cancer has occurred. The website shows that 1218 patients were included, while 198523 patients were included in the control group.
Two-sample bidirectional MR was used to test the causal relationship between insulin-related traits and tumors. In order to determine whether insulin-related traits could be a risk factor for various tumors, we first selected closely related SNPs from ovarian cancers’ GWAS results. In this process, insulin-related traits acted as exposure and ovarian cancer occurs as a result. In order to verify whether ovarian tumors cause insulin-related traits, SNPs related to various tumors are selected as the instrumental variable in the reverse MR analysis process, with ovarian tumors as the exposure, and insulin-related traits as the result.
Three different MR methods, including inverse variance weighted random effects (IVW), MR Egger and weighted median, were used to evaluate heterogeneity and test multiple effects. SNPs and outliers of insulin related traits identified by MR-PRESSO were removed. In the face of Mendelian randomization, IVW was used as the main analysis method, which was widely accepted. The threshold for screening exposure variables was 10^-6. MR Egger (24) was often a test that allows all genetic variations to have pleiotropic effects, but requires pleiotropic effects to be independent of the exposure association between variations. For Mendelian randomization pleiotropy test, MR Egger intercept test and retention analysis were used to further evaluate the level pleiotropy. Cochran’s Q test was implemented in each MR analysis to detect data heterogeneity between exposure and outcome, which was an important indicator affecting the reliability of final results. In the final visualization part, the funnel chart was used to evaluate the possible directional pleiotropy, similar to the evaluation of publication bias in meta-analysis, and also to observe the data distribution. The forest map is used to show the results of each SNP and the final MR, which was a convenient and intuitive method for visualizing the results.
All bidirectional mendelian randomization statistical analysis and data visualization used “TwoSampleMR” (https://github.com/MRCIEU/TwoSampleMR) in R software version 4.1.1. RStudio (https://posit.co/products/open-source/rstudio/) was used as a platform tool for opening and analysis, which was an integrated development environment for R and Python. It included a console, syntax highlighting editor that supports direct code execution, and tools for drawing, history, debugging, and workspace management. Bilateral P value less than 0.05 was considered statistically significant.
Six insulin related indicators, including Fasting blood insulin, Fasting blood insulin adjusted for BMI, Insulin secret rate, Peak insulin response, Acute insulin response and Insulin disposition index, went Two-sample bidirectional MR with ovarian cancer. We conducted a total of 12 statistical tests in 6 groups.
As shown in Table 2, we summarize all positive MR results into this table. The insulin secretion rate was statistically significant (IVW, p<0.05).
On the contrary, the other five insulin related indicators (Fasting blood insulin, Fasting blood insulin adjusted for BMI, Peak insulin response, Acute insulin response and Insulin disposition index) did not show any correlation with the risk of ovarian cancer (Supplementary Figures S1-S5).
When taking insulin secretion rate as the exposure factor, we found that impaired insulin secretion was associated with an increased risk of ovarian cancer (OR 0.9991305 (0.9984108, 0.9998507), p=0.017968, Figure 1), which was confirmed in the positive MR analysis (Figure 2). There were 9 SNPs related to the above results (rs10830963, rs10983538, rs11135317, rs138478706, rs1779638, rs58858201, rs7756992, rs9425530, rs9479886), and the details were shown in S-Table 1 of Supplementary Materials. For the pleiotropy test of MR analysis, no obvious pleiotropy was found (p>0.05, Table 2). The retention analysis of the above results shows that all SNPs are generally stable (Figure 3), and the funnel plot did not show significant heterogeneity (Supplementary Figure S6).
Figure 1 Mendelian randomization results of the association of the insulin secretion rate on ovarian cancer (Forward).
However, we still got statistically significant results (IVW, p<0.05) when we performed reverse MR analysis on the insulin secret rate. That was to say, with ovarian cancer as the exposure factor and the insulin secret rate as the outcome variable, we still got the causal relationship of the above two parties (OR 3.092427e-13 (3.816945e-23, 2.505434e-03), p=0.013, Figure 4), which was confirmed in the inversive MR analysis (Figure 5). The above results indicate that ovarian cancer had a causal relationship with human insulin secret rate. In order to test the reliability of the above results, we conducted Cochran’s Q test (p=0.4528141) and pleiotropy test (p=0.6568936). However, these test results indicate that the above results do not have the pleiotropy and heterogeneity of imaging conclusions. There were 10 SNPs related to the above results (rs114858887, rs1358253, rs1687403, rs2143612, rs28678815, rs35486093, rs4443540, rs76264086, rs78231145, rs79693379), and the details were shown in S-Table 2 of Supplementary Materials. The retention analysis of the above results showed that all SNPs were generally stable (Figure 6), and the funnel plot did not show significant heterogeneity (Supplementary Figure S7).
Figure 4 Mendelian randomization results of the association of ovarian cancer on the insulin secretion rate (Reverse).
This study is a Bidirectional Mendelian Randomization Study, which used MR to analyze the two-way causal relationship between insulin related traits and the risk of ovarian cancer. We found that the insulin secretion rate has a two-way causal relationship with ovarian cancer, which is rarely reported.
Insulin is an important hormone in mammalian homeostasis regulation, which regulates metabolism together with glucagon antagonism. The insulin secretion rate provides an estimate of the insulin secretion rate before hepatic insulin clearance (25). The main physiological stimulation of insulin secretion is the increase of circulating glucose concentration in the postprandial state. Impaired insulin secretion is often associated with high body mass index, and a large number of statistics have proved the association between overweight and ovarian cancer (15, 26). As mentioned in the introduction of this article, impaired insulin status is associated with the risk or survival of many cancers.
By analyzing the MR results in this paper, we could easily find that impaired insulin secretion was associated with an increased incidence of ovarian cancer. We will analyze the influence of insulin secretion on ovarian cancer from the following aspects. First, from the perspective of insulin and tumor cell energetics, compared with healthy cells, ovarian cancer tumor cells have a huge energy demand to support the abnormal proliferation and metastasis of tumors. Compared with normal human cells, tumor cells tend to change their metabolic mode, such as the transformation of primary glucose utilization pathway from oxidative phosphorylation to glycolysis, namely Warburg effect (27). Insulin also controls systemic and intracellular metabolism through substrate (glucose) distribution (28). However, tumors have changes in PI3K mTOR signaling pathway, and mTOR also changes the availability of glucose in tumor cells by regulating glucose uptake and glycogen decomposition (29). At the same time, anti-tumor drugs targeting systemic glucose homeostasis and tumor growth regulation have also entered the clinical trial stage (30). Second, impaired insulin secretion is associated with glucagon. Hyperinsulinemia is associated with the increased risk of breast cancer (31), endometrial cancer (32), ovarian cancer (33) and prostate cancer (34), and is closely related to the increased mortality of pancreatic cancer and breast cancer (35, 36), and some studies indicate that glucagon increases the overall mortality of cancer (37). However, it should be noted that many studies have pointed out that the postmenopausal serum insulin level is not related or is very weak to ovarian cancer after adjustment and correction (33, 38), which is consistent with the negative results of this study. However, we found that the significant results are insulin secretion rather than simple serum levels. At the same time, there is no denying that insulin and glucagon, which are hormones, are closely related to lipid peroxidation and metabolism (39), fibroblast growth factor receptor-1 (40), and inflammatory cytokines (41), and these factors undoubtedly play a key role in the progress of cancer. Third, the repeated mention of obesity or overweight is undoubtedly related to impaired insulin secretion. Ovarian cancer cells use fat cells as a source of energy for growth and migration (42). At the same time, as metabolic disorders, their internal metabolism affects each other. Because of changes in lifestyle factors, the prevalence of metabolic disorders is increasing year by year worldwide, just as obesity, type II diabetes and metabolic syndrome are all associated with ovarian cancer (43–46). A recent meta-analysis (47) showed that the risk of diabetes and OC was weak but still related, and many studies had many bias or confounding factors. It has also been pointed out that despite normal BMI, people with unhealthy metabolism or central obesity have a higher risk of cancer (48). Fourth, insulin is also related to immunity. Insulin (49) is related to regulating different immune phenotypes and responses, and the expression of insulin receptors on T cells, B cells and macrophages proves this view (50).
At the same time, another study showed that the existence of ovarian cancer was related to insulin secretion. The mechanism involved in this is very complex, because the metabolism of tumor variant fish is very complex. We speculate that the anaerobic glycolysis of tumors occupies the main form of metabolism, and pentose phosphate shunt pathway and its nucleotide products (51) play a certain role in the regulation of insulin secretion. Among them, glucose-6-phosphate dehydrogenase (G6PDH) (52) can explain the impairment of insulin secretion by islet cells through the impairment of NADPH production, and the 6-phosphogluconate dehydrogenase (6PGDH) (53) negative impact is attributed to the accumulation of intermediate metabolites of this pathway, leading to the activation of extracellular regulated kinase (ERK). Currently, it is known that ERK (53) can promote insulin transcription in response to acute signals, but its continuous activation may lead to β Cell dysfunction and apoptosis.
These studies have many defects and deficiencies, as follows: (1) Avoiding the pleiotropy of SNPs selected as instrumental variables is an important principle to ensure the accuracy of MR analysis. Usually, MR Egger intercept and MR-PRESSO methods are used to detect horizontal pleiotropy to reduce bias, but the method is not absolute for detection of pleiotropy. The MR analysis results of this study did not find heterogeneity and level pleiotropy, which proved the robustness of the results, but still could not completely rule out the interference of potential pleiotropy. This limitation is due to the existing analysis methods, and there are also some works (54) exploring other multiple validity testing methods. (2) The Insulin related Trains included in this study may lack some indicators. When selecting indicators, we selected open and common indicators. For the selection of databases, we also selected databases based on the same species, recent time and large number of people. However, this may not fully represent the function and release of insulin. We try to avoid these limitations, but it is undeniable that they may still exist. (3) There is also the race problem in the database. In order to control the same race, we try to select European samples, which will undoubtedly affect the conclusion to be extended to other colored people. (4) The sample size of some indicators may not be enough to avoid bias, which is also caused by database restrictions.
Through the Bidirectional Mendelian Randomization analysis, we obtained the two-way causal relationship between the insulin secret rate and ovarian cancer, that is, the reduction of the insulin secret rate is related to the risk of ovarian cancer, and the occurrence of ovarian cancer also has an impact on the insulin secret rate. When this research needs large sample data research in the real world, we hope to have research to further verify this conclusion.
The original contributions presented in the study are included in the article/Supplementary Material. Further inquiries can be directed to the corresponding authors.
Ethical review and approval was not required for the study on human participants in accordance with the local legislation and institutional requirements. Written informed consent for participation was not required for this study in accordance with the national legislation and the institutional requirements.
XW wrote the main manuscript. JL and LC developed the model, WL and JS collected and analyzed the data. ZY reviewed and revised the manuscript. ZW and HL designed the study. Other personnel participate in discussion and article revision. All authors contributed to the article and approved the submitted version.
This work was supported by Grant 61931013 (ZW), 62171297 (HL) 82202258 (WL) from the National Natural Science Foundation of China, Beijing Hospitals Authority Clinical Medicine Development of Special Funding Support NO:ZYLX202101, the Beijing Municipal Science and Technology Commission [Grant Number Z201100005620009].
The authors declare that the research was conducted in the absence of any commercial or financial relationships that could be construed as a potential conflict of interest.
All claims expressed in this article are solely those of the authors and do not necessarily represent those of their affiliated organizations, or those of the publisher, the editors and the reviewers. Any product that may be evaluated in this article, or claim that may be made by its manufacturer, is not guaranteed or endorsed by the publisher.
The Supplementary Material for this article can be found online at: https://www.frontiersin.org/articles/10.3389/fendo.2023.1131767/full#supplementary-material
1. Kohn EC, Ivy SP. Whence high-grade serous ovarian cancer. Am Soc Clin Oncol Educ book. (2017) 37:443–8. doi: 10.1200/EDBK_174718
2. Vergote I, González-Martín A, Ray-Coquard I, Harter P, Colombo N, Pujol P, et al. European Experts consensus: BRCA/homologous recombination deficiency testing in first-line ovarian cancer. Ann oncol: Off J Eur Soc Med Oncol (2022) 33:276–87. doi: 10.1016/j.annonc.2021.11.013
3. Reid BM, Permuth JB, Sellers TA. Epidemiology of ovarian cancer: A review. Cancer Biol Med (2017) 14:9–32. doi: 10.20892/j.issn.2095-3941.2016.0084
4. Miller KD, Nogueira L, Devasia T, Mariotto AB, Yabroff KR, Jemal A, et al. Cancer treatment and survivorship statistics, 2022. CA: Cancer J Clin (2022) 72:409–36. doi: 10.3322/caac.21731
5. Wang X, Xu C, Grzegorzek M, Sun H. Habitat radiomics analysis of pet/ct imaging in high-grade serous ovarian cancer: Application to ki-67 status and progression-free survival. Front Physiol (2022) 13:948767. doi: 10.3389/fphys.2022.948767
6. Lele S ed. Ovarian cancer. Brisbane (AU: Exon Publications Copyright© 2022 Exon Publications. (2022).
7. Norton L, Shannon C, Gastaldelli A, DeFronzo RA. Insulin: The master regulator of glucose metabolism. Metab: Clin Exp (2022) 129:155142. doi: 10.1016/j.metabol.2022.155142
8. Patti AM, Giglio RV, Papanas N, Serban D, Stoian AP, Pafili K, et al. Experimental and emerging free fatty acid receptor agonists for the treatment of type 2 diabetes. Medi (Kaunas Lithuania) (2022) 58(1): 109. doi: 10.3390/medicina58010109
9. Catsburg C, Gunter MJ, Chen C, Cote ML, Kabat GC, Nassir R, et al. Insulin, estrogen, inflammatory markers, and risk of benign proliferative breast disease. Cancer Res (2014) 74:3248–58. doi: 10.1158/0008-5472.CAN-13-3514
10. Gurney J, Stanley J, Teng A, Krebs J, Koea J, Lao C, et al. Cancer and diabetes co-occurrence: A national study with 44 million person-years of follow-up. PloS One (2022) 17:e0276913. doi: 10.1371/journal.pone.0276913
11. Barone BB, Yeh HC, Snyder CF, Peairs KS, Stein KB, Derr RL, et al. Long-term all-cause mortality in cancer patients with preexisting diabetes mellitus: A systematic review and meta-analysis. Jama (2008) 300:2754–64. doi: 10.1001/jama.2008.824
12. García-Jiménez C, Gutiérrez-Salmerón M, Chocarro-Calvo A, García-Martinez JM, Castaño A, de la Vieja A. From obesity to diabetes and cancer: Epidemiological links and role of therapies. Br J Cancer (2016) 114:716–22. doi: 10.1038/bjc.2016.37
13. Currie CJ, Poole CD, Jenkins-Jones S, Gale EA, Johnson JA, Morgan CL. Mortality after incident cancer in people with and without type 2 diabetes: Impact of metformin on survival. Diabetes Care (2012) 35:299–304. doi: 10.2337/dc11-1313
14. Ding N, Zhan J, Shi Y, Qiao T, Li P, Zhang T. Obesity in children and adolescents and the risk of ovarian cancer: A systematic review and dose-response meta-analysis. PloS One (2022) 17:e0278050. doi: 10.1371/journal.pone.0278050
15. Foong KW, Bolton H. Obesity and ovarian cancer risk: A systematic review. Post Reprod Health (2017) 23:183–98. doi: 10.1177/2053369117709225
16. Leitner BP, Siebel S, Akingbesote ND, Zhang X, Perry RJ. Insulin and cancer: A tangled web. Biochem J (2022) 479:583–607. doi: 10.1042/BCJ20210134
17. Mahajan A, Sim X, Ng HJ, Manning A, Rivas MA, Highland HM, et al. Identification and functional characterization of G6PC2 coding variants influencing glycemic traits define an effector transcript at the G6PC2-ABCB11 locus. PloS Genet (2015) 11:e1004876. doi: 10.1371/journal.pgen.1004876
18. Manning AK, Hivert MF, Scott RA, Grimsby JL, Bouatia-Naji N, Chen H, et al. A genome-wide approach accounting for body mass index identifies genetic variants influencing fasting glycemic traits and insulin resistance. Nat Genet (2012) 44:659–69. doi: 10.1038/ng.2274
19. Wood AR, Jonsson A, Jackson AU, Wang N, van Leewen N, Palmer ND, et al. A genome-wide association study of IVGTT-based measures of first-phase insulin secretion refines the underlying physiology of type 2 diabetes variants. Diabetes (2017) 66:2296–309. doi: 10.2337/db16-1452
20. Hovorka R, Soons PA, Young MA. ISEC: A program to calculate insulin secretion. Comput Methods prog biomed (1996) 50:253–64. doi: 10.1016/0169-2607(96)01755-5
21. Hovorka R, Koukkou E, Southerden D, Powrie JK, Young MA. Measuring pre-hepatic insulin secretion using a population model of c-peptide kinetics: accuracy and required sampling schedule. Diabetologia (1998) 41:548–54. doi: 10.1007/s001250050945
22. Pacini G, Bergman RN. MINMOD: A computer program to calculate insulin sensitivity and pancreatic responsivity from the frequently sampled intravenous glucose tolerance test. Comput Methods prog biomed (1986) 23:113–22. doi: 10.1016/0169-2607(86)90106-9
23. Hemani G, Zheng J, Elsworth B, Wade KH, Haberland V, Baird D, et al. The MR-base platform supports systematic causal inference across the human phenome. eLife (2018) 7. doi: 10.7554/eLife.34408
24. Bowden J, Davey Smith G, Burgess S. Mendelian randomization with invalid instruments: effect estimation and bias detection through egger regression. Int J Epidemiol (2015) 44:512–25. doi: 10.1093/ije/dyv080
25. Van Cauter E, Mestrez F, Sturis J, Polonsky KS. Estimation of insulin secretion rates from c-peptide levels. comparison of individual and standard kinetic parameters for c-peptide clearance. Diabetes (1992) 41:368–77. doi: 10.2337/diab.41.3.368
26. Nagle CM, Dixon SC, Jensen A, Kjaer SK, Modugno F, deFazio A, et al. Obesity and survival among women with ovarian cancer: Results from the ovarian cancer association consortium. Br J Cancer (2015) 113:817–26. doi: 10.1038/bjc.2015.245
27. Vander Heiden MG, Cantley LC, Thompson CB. Understanding the warburg effect: the metabolic requirements of cell proliferation. Sci (New York N.Y.) (2009) 324:1029–33.
28. Magkos F, Wang X, Mittendorfer B. Metabolic actions of insulin in men and women. Nutr (Burbank Los Angeles County Calif.) (2010) 26:686–93.
29. Emami Nejad A, Najafgholian S, Rostami A, Sistani A, Shojaeifar S, Esparvarinha M, et al. The role of hypoxia in the tumor microenvironment and development of cancer stem cell: A novel approach to developing treatment. Cancer Cell Int (2021) 21:62. doi: 10.1186/s12935-020-01719-5
30. Blow T, Hyde PN, Falcone JN, Neinstein A, Vasan N, Chitkara R, et al. Treating alpelisib-induced hyperglycemia with very low carbohydrate diets and sodium-glucose Co-transporter 2 inhibitors: A case series. Integr Cancer therapies (2021) 20:15347354211032283. doi: 10.1177/15347354211032283
31. Lee J, Chang Y, Kim Y, Park B, Ryu S. Insulin resistance and the development of breast cancer in premenopausal women: The kangbuk Samsung health study. Breast Cancer Res Treat (2022) 192:401–9. doi: 10.1007/s10549-022-06513-7
32. Gunter MJ, Hoover DR, Yu H, Wassertheil-Smoller S, Manson JE, Li J, et al. A prospective evaluation of insulin and insulin-like growth factor-I as risk factors for endometrial cancer. Cancer epidemiol Biomarkers prevention: Publ Am Assoc Cancer Research cospons by Am Soc Prev Oncol (2008) 17:921–9. doi: 10.1158/1055-9965.EPI-07-2686
33. Kabat GC, Kim MY, Lane DS, Zaslavsky O, Ho GYF, Luo J, et al. Serum glucose and insulin and risk of cancers of the breast, endometrium, and ovary in postmenopausal women. Eur J Cancer prevention: Off J Eur Cancer Prev Organisat (ECP) (2018) 27:261–8. doi: 10.1097/CEJ.0000000000000435
34. Pandeya DR, Mittal A, Sathian B, Bhatta B. Role of hyperinsulinemia in increased risk of prostate cancer: A case control study from Kathmandu valley. Asian Pacific J Cancer prevention: APJCP (2014) 15:1031–3. doi: 10.7314/APJCP.2014.15.2.1031
35. Kim NH, Chang Y, Lee SR, Ryu S, Kim HJ. Glycemic status, insulin resistance, and risk of pancreatic cancer mortality in individuals with and without diabetes. Am J Gastroenterol (2020) 115:1840–8. doi: 10.14309/ajg.0000000000000956
36. Pan K, Chlebowski RT, Mortimer JE, Gunter MJ, Rohan T, Vitolins MZ, et al. Insulin resistance and breast cancer incidence and mortality in postmenopausal women in the women’s health initiative. Cancer (2020) 126:3638–47. doi: 10.1002/cncr.33002
37. Wargny M, Balkau B, Lange C, Charles MA, Giral P, Simon D. Association of fasting serum insulin and cancer mortality in a healthy population - 28-year follow-up of the French TELECOM study. Diabetes Metab (2018) 44:30–7. doi: 10.1016/j.diabet.2017.03.006
38. Michels KA, McNeel TS, Trabert B. Metabolic syndrome and risk of ovarian and fallopian tube cancer in the United States: An analysis of linked SEER-Medicare data. Gynecol Oncol (2019) 155:294–300. doi: 10.1016/j.ygyno.2019.08.032
39. Marino N, German R, Rao X, Simpson E, Liu S, Wan J, et al. Upregulation of lipid metabolism genes in the breast prior to cancer diagnosis. NPJ Breast Cancer (2020) 6:50. doi: 10.1038/s41523-020-00191-8
40. Wellberg EA, Kabos P, Gillen AE, Jacobsen BM, Brechbuhl HM, Johnson SJ, et al. FGFR1 underlies obesity-associated progression of estrogen receptor-positive breast cancer after estrogen deprivation. JCI Insight (2018) 3. doi: 10.1172/jci.insight.120594
41. Padmanabhan S, Gaire B, Zou Y, Uddin MM, Vancurova I. IFNγ-induced PD-L1 expression in ovarian cancer cells is regulated by JAK1, STAT1 and IRF1 signaling. Cell signal (2022) 97:110400. doi: 10.1016/j.cellsig.2022.110400
42. Nieman KM, Kenny HA, Penicka CV, Ladanyi A, Buell-Gutbrod R, Zillhardt MR, et al. Adipocytes promote ovarian cancer metastasis and provide energy for rapid tumor growth. Nat Med (2011) 17:1498–503. doi: 10.1038/nm.2492
43. Siegel RL, Miller KD, Jemal A. Cancer statistics, 2018. CA: Cancer J Clin (2018) 68:7–30. doi: 10.3322/caac.21442
44. Lauby-Secretan B, Scoccianti C, Loomis D, Grosse Y, Bianchini F, Straif K. Body fatness and cancer–viewpoint of the IARC working group. New Engl J Med (2016) 375:794–8. doi: 10.1056/NEJMsr1606602
45. Shah MM, Erickson BK, Matin T, McGwin G Jr., Martin JY, Daily LB, et al. 3rd, diabetes mellitus and ovarian cancer: More complex than just increasing risk. Gynecol Oncol (2014) 135:273–7. doi: 10.1016/j.ygyno.2014.09.004
46. Akhavan S, Ghahghaei-Nezamabadi A, Modaresgilani M, Mousavi AS, Sepidarkish M, Tehranian A, et al. Impact of diabetes mellitus on epithelial ovarian cancer survival. BMC Cancer (2018) 18:1246. doi: 10.1186/s12885-018-5162-3
47. Wang L, Zhong L, Xu B, Chen M, Huang H. Diabetes mellitus and the risk of ovarian cancer: A systematic review and meta-analysis of cohort and case-control studies. BMJ Open (2020) 10:e040137. doi: 10.1136/bmjopen-2020-040137
48. Park YM, White AJ, Nichols HB, O’Brien KM, Weinberg CR, Sandler DP. The association between metabolic health, obesity phenotype and the risk of breast cancer. Int J Cancer (2017) 140:2657–66. doi: 10.1002/ijc.30684
49. van Niekerk G, Christowitz C, Conradie D, Engelbrecht AM. Insulin as an immunomodulatory hormone. Cytokine Growth fact Rev (2020) 52:34–44. doi: 10.1016/j.cytogfr.2019.11.006
50. Andreone L, Gimeno ML, Perone MJ. Interactions between the neuroendocrine system and T lymphocytes in diabetes. Front Endocrinol (2018) 9:229. doi: 10.3389/fendo.2018.00229
51. Campbell JE, Newgard CB. Mechanisms controlling pancreatic islet cell function in insulin secretion. Nat Rev Mol Cell Biol (2021) 22:142–58. doi: 10.1038/s41580-020-00317-7
52. Spégel P, Sharoyko VV, Goehring I, Danielsson AP, Malmgren S, Nagorny CL, et al. Time-resolved metabolomics analysis of β-cells implicates the pentose phosphate pathway in the control of insulin release. Biochem J (2013) 450:595–605. doi: 10.1042/BJ20121349
53. Goehring I, Sauter NS, Catchpole G, Assmann A, Shu L, Zien KS, et al. Identification of an intracellular metabolic signature impairing beta cell function in the rat beta cell line INS-1E and human islets. Diabetologia (2011) 54:2584–94. doi: 10.1007/s00125-011-2249-7
Keywords: ovarian cancer, Mendelian randomization, insulin, insulin secretion rate, endocrine marker
Citation: Wang X, Sun J, Li J, Cai L, Chen Q, Wang Y, Yang Z, Liu W, Lv H and Wang Z (2023) Bidirectional Mendelian randomization study of insulin-related traits and risk of ovarian cancer. Front. Endocrinol. 14:1131767. doi: 10.3389/fendo.2023.1131767
Received: 26 December 2022; Accepted: 09 February 2023;
Published: 01 March 2023.
Edited by:
Ruiqin Han, Institute of Basic Medical Sciences, Chinese Academy of Medical Sciences, ChinaReviewed by:
Huanchun Yao, Sheng Jing Hospital Affiliated to China Medical University, ChinaCopyright © 2023 Wang, Sun, Li, Cai, Chen, Wang, Yang, Liu, Lv and Wang. This is an open-access article distributed under the terms of the Creative Commons Attribution License (CC BY). The use, distribution or reproduction in other forums is permitted, provided the original author(s) and the copyright owner(s) are credited and that the original publication in this journal is cited, in accordance with accepted academic practice. No use, distribution or reproduction is permitted which does not comply with these terms.
*Correspondence: Han Lv, Y2hyaXNsdmhhbkAxMjYuY29t; Zhenchang Wang, Y2pyLnd6aGNoQHZpcC4xNjMuY29t
Disclaimer: All claims expressed in this article are solely those of the authors and do not necessarily represent those of their affiliated organizations, or those of the publisher, the editors and the reviewers. Any product that may be evaluated in this article or claim that may be made by its manufacturer is not guaranteed or endorsed by the publisher.
Research integrity at Frontiers
Learn more about the work of our research integrity team to safeguard the quality of each article we publish.