- 1Max Planck Institute for Metabolism Research, Cologne, Germany
- 2Department for Psychiatry, Psychosomatic Medicine and Psychotherapy, University Hospital Frankfurt, Goethe University, Frankfurt am Main, Germany
- 3Faculty of Medicine and University Hospital Cologne, Policlinic for Endocrinology, Diabetes and Preventive Medicine (PEPD), University of Cologne, Cologne, Germany
- 4Cluster of Excellence in Cellular Stress Responses in Aging-associated Diseases (CECAD), Cologne, Germany
Objective: While variations in the first intron of the fat mass and obesity-associated gene (FTO, rs9939609 T/A variant) have long been identified as a major contributor to polygenic obesity, the mechanisms underlying weight gain in risk allele carriers still remain elusive. On a behavioral level, FTO variants have been robustly linked to trait impulsivity. The regulation of dopaminergic signaling in the meso-striatal neurocircuitry by these FTO variants might represent one mechanism for this behavioral alteration. Notably, recent evidence indicates that variants of FTO also modulate several genes involved in cell proliferation and neuronal development. Hence, FTO polymorphisms might establish a predisposition to heightened trait impulsivity during neurodevelopment by altering structural meso-striatal connectivity. We here explored whether the greater impulsivity of FTO variant carriers was mediated by structural differences in the connectivity between the dopaminergic midbrain and the ventral striatum.
Methods: Eighty-seven healthy normal-weight volunteers participated in the study; 42 FTO risk allele carriers (rs9939609 T/A variant, FTO+ group: AT, AA) and 39 non-carriers (FTO− group: TT) were matched for age, sex and body mass index (BMI). Trait impulsivity was assessed via the Barratt Impulsiveness Scale (BIS-11) and structural connectivity between the ventral tegmental area/substantia nigra (VTA/SN) and the nucleus accumbens (NAc) was measured via diffusion weighted MRI and probabilistic tractography.
Results: We found that FTO risk allele carriers compared to non-carriers, demonstrated greater motor impulsivity (p = 0.04) and increased structural connectivity between VTA/SN and the NAc (p< 0.05). Increased connectivity partially mediated the effect of FTO genetic status on motor impulsivity.
Conclusion: We report altered structural connectivity as one mechanism by which FTO variants contribute to increased impulsivity, indicating that FTO variants may exert their effect on obesity-promoting behavioral traits at least partially through neuroplastic alterations in humans.
Highlights
- Human healthy-weight carriers of the fat mass and obesity-associated (FTO) risk allele variant rs9939609 show enhanced trait impulsivity
- FTO risk allele carriers also demonstrate increased structural meso-striatal connectivity
- The greater impulsivity related to variants of FTO is partially mediated by the increased structural connectivity between dopaminergic midbrain and nucleus accumbens suggesting a mechanism by which genetic predisposition might affect this behavioral trait in humans
Introduction
Obesity results from an interplay between obesogenic environmental factors and individual (genetic) susceptibility (1). In 2007, variations in the first intron of the fat mass and obesity-associated gene (FTO) were identified to be associated with increased body mass (2). This association has since been replicated in several populations of children and adults across both genders, providing now-established evidence that variations in the FTO gene contribute to polygenic obesity (3–6). However, the underlying molecular mechanisms are entwined affecting multiple organs and processes from thermogenesis in brown adipose tissue and lipid storage (7) to encoding of adaptive behavior in the brain (8). Furthermore, the direct and indirect molecular mechanisms of FTO are particularly complicated to disentangle, as FTO polymorphisms are not only of functional relevance for behavioral encoding in the adult brain but also affect a multitude of signaling pathways during early development causing structural brain changes and hence potentially the basis for hard-wired predispositions for behavioral alterations.
The (neuro)developmental effects of FTO are based on its role as a demethylase. Specifically, FTO modulates the expression of several genes involved in cell proliferation, migration, and neuronal development (9–14). Loss of FTO leads to the altered expression of several key components of the brain-derived neurotrophic factor pathway [BDNF, 13], which has been repeatedly linked to polygenic and mono-genetic obesity forms via several molecular mechanisms (15–18). Loss of FTO also reduces the pool of adult neural stem cells and leads to decreased brain size, deficient neurogenesis (10, 13, 19)
and hypomyelination (20). Additionally, evidence from animal studies also indicates an indirect role of FTO polymorphism in neuronal plasticity promoting obesity by regulating genes or enhancers involved in neurogenesis relevant for body weight homeostasis (21–26) without affecting FTO expression (26). Hence, FTO polymorphism might - directly and indirectly - affect obesity development via neurodevelopmental pathways.
In addition to its effects on neuronal plasticity, FTO polymorphisms also affect the neural encoding of behavior in adulthood. Due to the regulatory role of FTO polymorphisms in FTO function as demethylase, intronic variants affect the modification of specific mRNAs of critical components of D2/3R-signaling (8) and consequently D2/D3 receptor-mediated auto-inhibition. Thus, FTO regulates the activity of DA neurons in the basic reward circuit of meso-striatal regions altering reward sensitivity (8). Evidence from human studies links risk-variants to alterations in multiple reward-related behaviors such as increased preference for high-caloric food (27, 28), heightened food craving (29), differences in food-cue reactivity (30), impaired reward learning (31) and alterations in impulse control (32).
Among the behavioral traits affected by FTO and encoded by the DA reward circuitry, impulsivity has been consistently associated with FTO polymorphism (32–34) and increased risk for future weight gain and overeating (35–38). According to the conceptualization of trait impulsivity by the Barett Impulsiveness Scale (BIS), particularly attentional (impaired ability to focus or concentrate) and motor (acting without thinking) impulsivity interact to promote weight gain (39). As the regulation of motor impulsivity predominantly depends on intact DA functioning in the ventral striatum (40) and given the regulatory role of FTO in D2-receptors (D2R) signaling in striatal regions, FTO might affect obesity development by regulating dopaminergic encoding of motor impulsivity in the midbrain. Indeed, this role is supported by our previous finding that FTO modulates increased functional coupling in meso-striatal regions —specifically ventral tegmental area/substantia nigra (VTA/SN) and the nucleus accumbens (NAc) as well as further downstream targets such as the medial prefrontal cortex (31). It is, however, unclear if FTO polymorphisms contribute to these alterations in the dopaminergic encoding of reward-related behavior via their neurodevelopmental effect on structural connectivity in the meso-striatal system. We, therefore, studied the structural connectivity of projections from the midbrain (VTA/SN) to the ventral striatum (NAc) and its contribution to the impulsive phenotype of FTO risk allele carriers.
Methods
Participants and study design
A cohort of 589 healthy, young Caucasians enrolled in the University of Cologne were recruited and genotyped for the rs9939609 T/A variant of the FTO gene (hereafter: FTO– group (TT) and FTO+ group (AT, AA)) as previously described (30, 31).
Participants were invited for further partially overlapping sub-studies. Eighty-seven participants were included in this sub-study, in which all participants underwent an impulsivity assessment using the Barrattt Impulsivity Scale-11 among other questionnaires (Becks Depression Inventory (BDI-2), Sensitivity to Punishment and Reward Questionnaire (SPSRQ), Alcohol Use Disorders Identification Test (AUDIT)), a buccal swab for DNA isolation and genotyping, a blood draw to assess the metabolic status and MRI imaging. The assessment of the Barrattt Impulsivity Scale and DNA isolation from the buccal swabs were performed as part of a previously published study (31). Inclusion criteria for the analysis performed in this study comprised: fully answered BIS-11 questionnaire and complete MRI imaging, no known diseases, fasting glucose and insulin within normal range, BDI-2 within normal range. 6 participants had to be excluded from further analyses due to unanswered BIS-11 questionnaire (5) and suspected diabetes mellitus (1) as indicated by a Homeostasis Model Assessment of Insulin Resistance (HOMA-IR, (41)) >5. In total, eighty-one participants were included in the final analysis (Table 1), 39 non-carriers (FTO–; 24.8 ± 3.5 years, 22.6 ± 2.6 kg/m2) and 42 risk allele carriers (FTO+; 25.7 ± 4.4 years; 23.2 ± 3.2 kg/m2).
All participants gave written informed consent to participate in the experiment, which had been approved by the local ethics committee of the Medical Faculty of the University of Cologne (Cologne, Germany, 10-226).
Assessment of impulsivity (BIS-11)
Impulsivity in participants was assessed by the German version of the BIS-11 questionnaire (42). The BIS-11 is the most often used self-report questionnaire to assess trait impulsivity (43). It consists of 30 items and is divided into 6 first-order (Attention, Motor, Self-control, Perseverance, Cognitive Instability, Cognitive Complexity) and 3 second-order factors (Attentional impulsiveness, Motor impulsiveness, and Non-planning impulsiveness). Each item is measured on a 4-point ‘Likert Scale’ ranging from “rarely/never” to “almost always/always”. The total score is calculated by summing all the item ratings, of which 8 cover attentional impulsiveness, 11 motor impulsiveness, and 11 non-planning impulsiveness, with a possible total range from 30 to 120.
DNA isolation and SNP-genotyping
Isolation of DNA from buccal swabs was performed using the QIAamp DNA Blood Mini Kit (# 51106, Qiagen GmbH, Hilden, Germany) according to the manufacturer’s instructions. The concentration and quality of the DNA were determined with a ND-1000 UV/Vis-Spectrophotometer (Peqlab GmbH, Erlangen, Germany). SNP genotyping for rs9939609 (FTO) was performed with 20 ng of DNA in triplicates using allelic discrimination assays (TaqMan SNP Genotyping Assays, Applied Biosystems by Life Technology GmbH, Darmstadt, Germany). The genotyping PCR was carried out on a 7900HT Fast Real-Time PCR System (Applied Biosystems) and the resulting fluorescence data were analyzed with Sequence Detection Software (SDS) 2.3 (Applied Biosystems) as already previously described for this cohort of patients.
Blood draw
Blood was drawn after an overnight fasting period of at least 8 hours to determine insulin, glucose, cholesterol and triglyceride values to compare the metabolic state of the participants. Insulin resistance was calculated according to the homeostatic model of insulin resistance (HOMA-IR) (41).
MRI acquisition
Imaging was performed on a 3 Tesla MRI scanner (Siemens TIM Trio, Erlangen, Germany). First, for each subject structural MRI data sets were acquired in a 12-channel array head coil and a maximum gradient strength 40 mT/m with a whole-brain field of view (T1-weighted: MDEFT3D; TR = 1930 ms, TE = 5.8 ms, resolution 1.0 × 1.0 × 1.25 mm, flip angle 18°, sagittal; T2-weighted: RARE; TR = 3200 ms, TE = 458 ms, 176 sagittal slices, resolution 1.0 × 1.0 × 1.0 mm3). Subsequently diffusion-weighted magnetic resonance imaging (dMRI) was conducted using spin echo planar imaging [SE-EPI; TR = 12,000 ms, TE = 100 ms, resolution 1.7 × 1.7 × 1.7 mm3, flip angle: 90°, Field of View (FoV): 220 × 220 × 122 mm3, bandwidth: 1345 Hz/pixel, orientation: axial, data matrix: 128 × 128 × 72, PAT factor 2, partial Fourier 6/8] with double-spin echo preparation (44). Diffusion-weighting was isotropically distributed along 60 diffusion-weighted directions (b value = 1000 s/mm2). Additionally, in each subject, seven data sets with no diffusion weighting (B0) were acquired initially and interleaved after each block of 10 diffusion-weighted images as anatomical reference for motion correction. To optimize the signal-to-noise ratio, when available scans from three assessments were averaged.
Masks
To create seed masks informing the tractography approach, we used anatomical constraints to outline the regions of VTA/SN, NAc, and caudate nucleus. Specifically, the mask location comprising the midbrain (VTA/SN) was chosen upon comparison across standard anatomical atlases (45, 46) as detailed in Pelzer et al. (47). For the midbrain mask, the following structures marked the border: ventromedially the red nucleus, latero-dorsally the subthalamic nucleus, and anteriorly the cerebral peduncles. The SN/VTA mask was labeled on an average T2-image in standard space in axial plane, and subsequently checked in coronal and sagittal plane. The masks for NAc and the caudate were retrieved from the Harvard-Oxford subcortical atlas (48, 49). Initially labeled in MNI-152-1mm space, the masks were afterwards transformed to individual diffusion space using the FSL’s applywarp function (50) and visually checked for each participant (Figure 1).
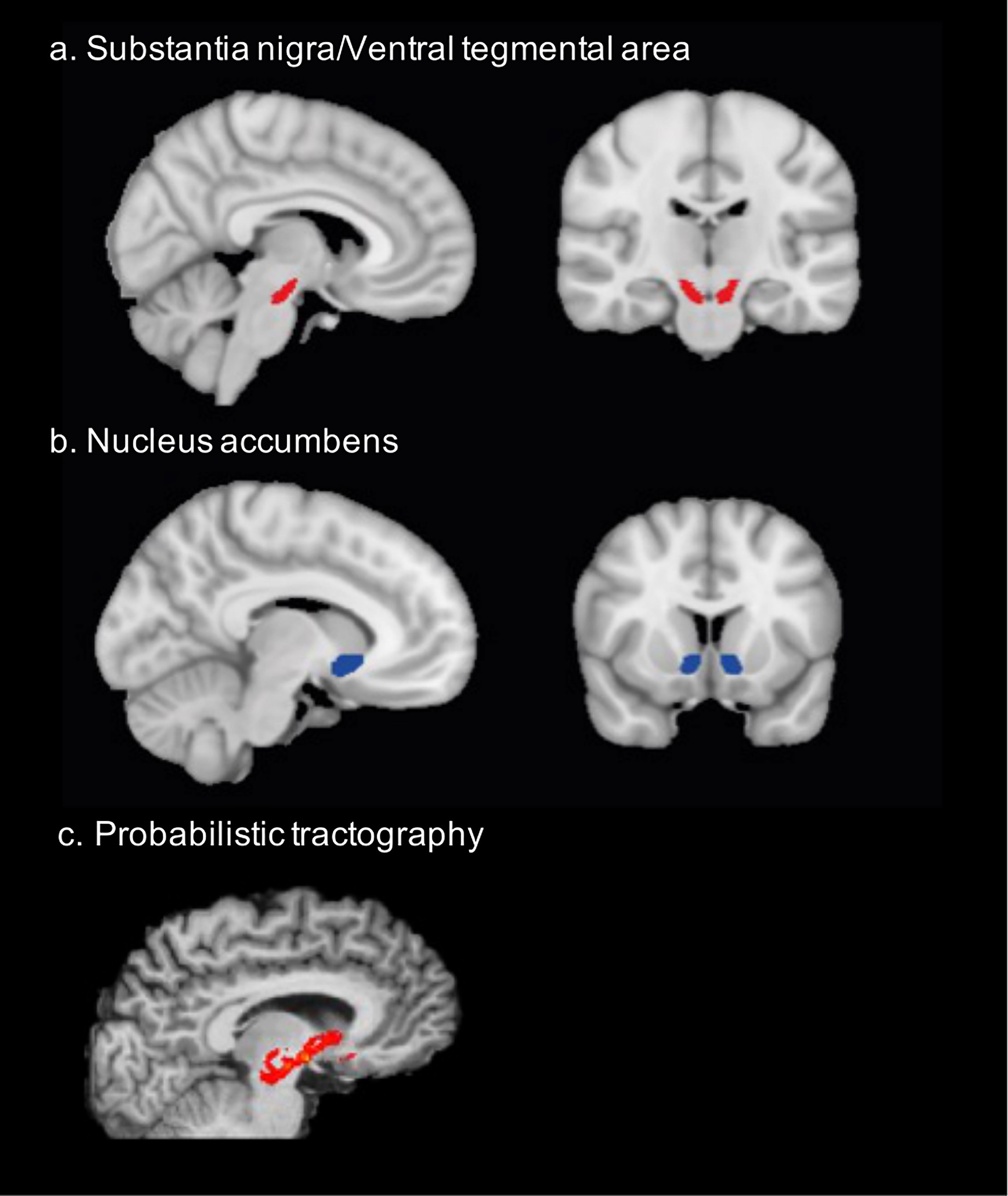
Figure 1 Structural connectivity was assessed between (A) the ventral tegmental area/substantia nigra (x=96, y= 106, z=53) and (B) the nucleus accumbens (x=99, y=138, z=62). The mask location comprising the midbrain (VTA/SN) was chosen upon comparison across standard anatomical atlases as detailed in Pelzer et al. (47). The NAc mask was retrieved from the Harvard-Oxford subcortical atlas. (C) probabilistic tractography was performed between these masks to assess structural connectivity. This is the example of one participant.
MRI data analysis
Preprocessing and data analysis were performed using tools from the FMRIB software library (FSL, version 5.0.4, https://www.fmrib.ox.ac.uk/fsl): First, we applied FSL’s brain extraction tool (BET) to T1-weighted (T1w) images and applied the resulting brain mask to T2-weighted (T2w) images, that had been aligned to T1-weighted images using rigid body alignment. Diffusion-weighted images were corrected for head motion using affine registration [FLIRT; 51] while using the first acquired B0 as a reference volume. Based on this transformation, the original b-vectors were corrected for the rotational components. To further enhance the reliability of the upcoming tractography algorithm, the preprocessed diffusion-weighted data were interpolated to 1mm (51, 52). T1-, T2- weighted images were then linearly co-registered using FSL’s FLIRT tool (53) to the MNI-152 1mm isotropic brain (12 DOF-affine registration). Diffusion-weighted images were co-registered to standard space using non-linear registration (FNIRT). The anatomical masks were nonlinearly transformed from standard space to diffusion space by applying the transformation matrices acquired with FSL’s FNIRT function (54). FSL’s BedpostX program was applied to model diffusion parameters for crossing fibers in native space. Afterwards, probabilistic tractography was performed in diffusion space using FSL’s Probtrackx2 (53, 55, 56). Probabilistic tractography is leveraged to reconstruct white matter structural connectivity in diffusion data. Starting from a voxel in the seed mask the tracking is performed in a stepwise manner from a distribution of possible directions. The distributions of principal diffusion directions calculated through BedpostX were used at each voxel to compute a streamline through these local samples and generate a probabilistic streamline. By taking many such samples, the FMRIB’s diffusion toolbox (FDT) is able to build upon the posterior distribution on the streamline location or the connectivity distribution (55). For each subject and seed region, the following parameters were chosen: The number of samples was 5000 for each seed voxel with a curvature threshold of 0.2, a maximum number of steps of 2000 with a step length of 0.5 mm. A path distribution function was added in order to correct for differences in distance between seed and target regions. The tractography was performed twice between seed and target in either direction. The ventricles were included as exclusion masks.
Connectivity measure
Structural connectivity between the SN/VTA and the NAc was analyzed. In order to test for construct validity, we also tested for differences in the structural connectivity between the DA midbrain and caudate, which is more implicated in attentional impulsivity than motor impulsivity and hence served as a validator for the specificity of the expected alterations in VTA/SN-NAc connectivity. The connectivity measure was defined as described in Stephan et al. (57). From the seed region (S) 5000 samples were initiated, and the proportion of the samples (KS) that intersected with target (T) defined the connectivity between these areas. Hence, this quantity reflects a relative connection density yielding numbers between 0 and 1 and does not inform about the absolute number of connections between these areas (58). It is important to note that this measure differs depending on whether tractography is sourced from S or T. Therefore, we performed the tractography in both directions and normalized by the size of the source region (N). Hence the relative connectivity density (φST) was defined by the following formula:
For statistical analyses this connectivity measure was considered to assess connectivity between the VTA/SN and NAc. This connectivity measure was also used to assess connectivity between the VTA/SN and the caudate in order to demonstrate the specificity of the effect of FTO polymorphism on structural connectivity between VTA/SN and NAc. For both VTA/SN-NAc and VTA/SN-caudate, connectivity measures were analyzed averaged across hemispheres.
Statistical analysis
Data was analyzed using RStudio [version 1.4.1717; (59)] and R [version 4.0.0; (60)] using the functions of R’s ‘Stats’ package (version 3.6.2) if not stated otherwise. Frequency distribution was tested with the Shapiro-Wilk-Test. For the connectivity data the Shapiro-Wilk-Test resulted in a deviation (p< 0.001) from the normal distribution. Due to this deviation from the normal distribution, we performed a logarithmic transformation of the connectivity values before further parametric testing. For further statistical assessment, linear regression models were performed using the “lm”-function in R. First, a linear regression model was used to assess differences in the total score of the BIS-11 scale between both groups (FTO+ vs FTO–, Table S1). To test for differences in the second-order factors of the BIS-11 (attentional impulsiveness, motor impulsiveness and non-planning impulsiveness) between the FTO+ and the FTO– group we also applied linear regression models (Table S2). We controlled for multiple comparisons using Bonferroni correction. Then we analyzed differences in (log) connectivity between the two groups (Table S3) and tested for the effect of FTO risk allele carrier status and (log) connectivity on motor impulsiveness in a combined model as required for the following mediation analysis (Table S4) using linear regression models respectively. Last, a mediation analysis was performed to test if (log) connectivity mediates the effect of risk allele carrier status on motor impulsiveness using R’s ‘Mediation’ package [version 4.5.0; (61); Table S5]. Effect sizes were calculated using R’s package ‘Effectsize’ [version 0.4.5; (62)]. Statistical significance was reported at a level of p< 0.05 if not otherwise stated.
For all analyses, we report the formula of the model using the Wilkinson notation in addition to the degrees of freedom, t- or F-values, significance of the respective regressors and their effect size in the Supplementary Material. A post hoc power analysis with an alpha-error of 0.05 was performed for each t-test and linear regression using the respective effect sizes and number of predictors (see Supplemental Tables 1–4). The power analysis was performed in G*Power [version 3.1; (63)].
Results
Based on the role of FTO in DA signaling, its association with trait impulsivity (which is determined by DA function in midbrain neurocircuitry), and strong evidence for FTO affecting cell proliferation and neurogenesis suggesting a role for FTO in neurodevelopment, we tested whether hardwired, structural connectivity changes between FTO risk allele carriers and non-carriers in meso-striatal circuitry contribute to an impulsive phenotype. We specifically considered the projections from the DA midbrain (VTA/SN) to the ventral striatum and tested whether the impulsive phenotype observed in risk allele carriers was mediated by such structural changes. Additionally, we also measured structural connectivity between VTA/SN and the caudate, which is implicated in attentional impulsivity (64) but not primarily in motor impulsiveness, to verify the specificity of the expected effect of gene variant on connectivity between the midbrain and the nucleus accumbens. We also tested if the observed differences have an underlying sex dimorphism.
Similar metabolic status and self-reported food intake behavior in risk allele carriers and non-carriers
In total, eighty-one participants were included in the final analysis (Table 1), 39 non-carriers (FTO–; 24.8 ± 3.5 years, 22.6 ± 2.6 kg/m2) and 42 risk allele carriers (FTO+; 25.7 ± 4.4 years; 23.2 ± 3.2 kg/m2), who were matched for age, BMI and sex. All participants self-reported to be healthy without any known diagnoses. Accordingly, participants in both groups did not differ in their metabolic status as measured by fasting insulin, glucose, cholesterol, and triglyceride values (all p > 0.4; Table 1). Neither risk allele carriers nor non-carriers reported depressive symptoms according to the BDI-2 (65) nor alcohol use disorder according to the alcohol use disorder identification test (66). Sensitivity to punishment and reward as well as eating behavior as assessed using the German version of the three-factor eating questionnaire were comparable across groups (all p > 0.05, Table 1).
Greater motor impulsivity in FTO risk allele carriers than in non-carriers
We first tested, whether FTO risk allele carriers differ in their impulsive phenotype in comparison to the non-carriers. Trait impulsivity in general, as measured by the BIS-11 total score, did not differ between the FTO+ and the FTO– group (F = 2.08, p = 0.156, Supplemental Table S1). As motor and attentional impulsivity have been particularly associated with weight gain, with motor impulsiveness being strongly linked to DA functioning in the ventral striatum and attentional impulsiveness in the caudate (64), we next assessed if motor impulsiveness differed between the FTO+ and FTO– groups. Compared to controls, FTO risk allele carriers demonstrated increased motor impulsiveness (F = 6.63, p = 0.030 after adjustment for multiple comparisons using Bonferroni correction), while no significant differences between FTO risk allele carriers and non-carriers could be observed for the other second-order factors of the BIS-11 including attentional impulsiveness (Supplemental Table S2, Figure 2A). Neither motor impulsivity nor the effect of FTO risk allele status on motor impulsivity differed between sexes (Supplemental Tables S1, S2).
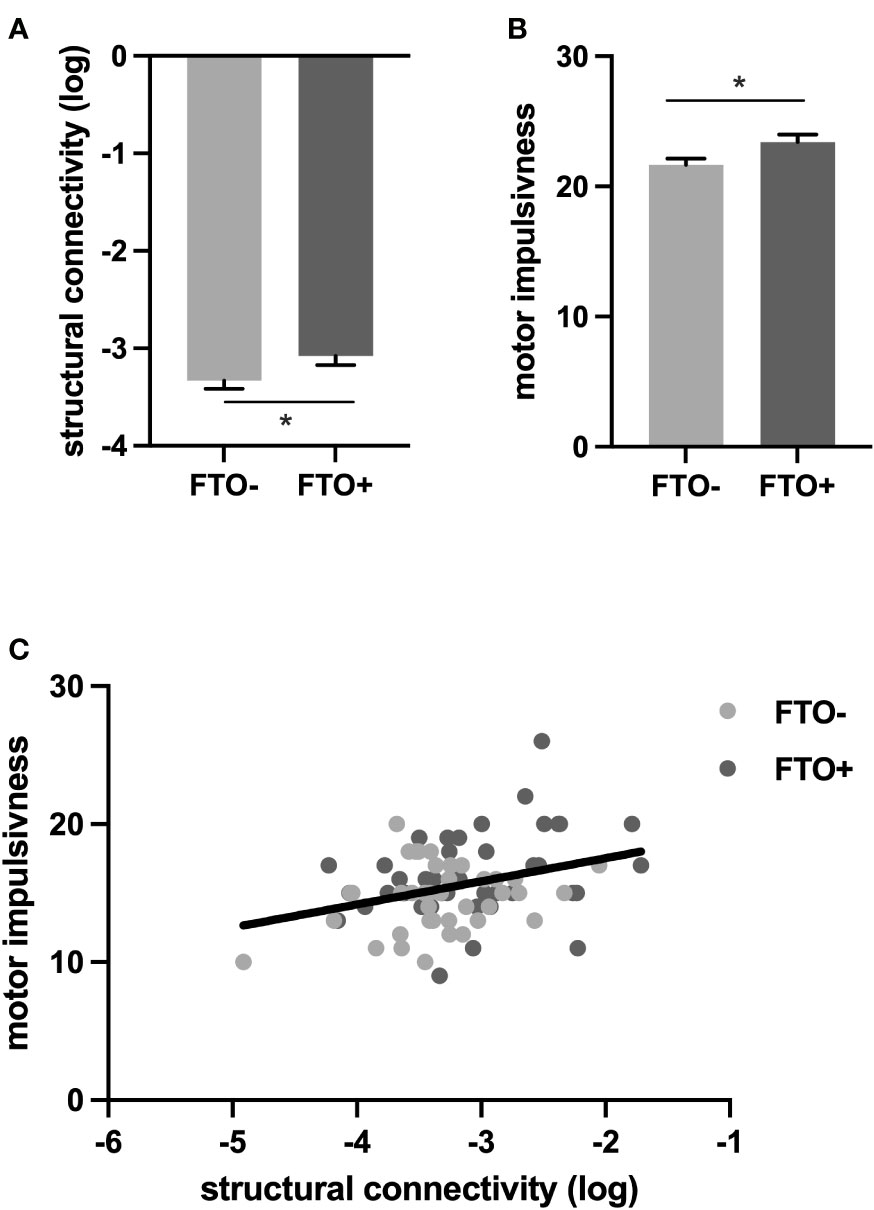
Figure 2 FTO allele status affects impulsivity and structural connectivity. (A). Log structural connectivity is higher in the FTO+ group compared to the FTO–group. (B) Motor impulsiveness is higher in the FTO+ than in the FTO– group (C) Increased (log) structural connectivity is associated with increased motor impulsivity. *p < 0.05; rs9939609 T/A variant, FTO− group: TT; FTO+ group: AT, AA.
Increased structural connectivity between the midbrain and the nucleus accumbens in FTO risk allele carriers but not between the midbrain and the caudate nucleus in the same participants
We next analyzed, if structural connectivity is changed in FTO risk allele carriers compared to non-carriers. We particularly considered connectional changes between VTA/SN and NAc due to their relevance in encoding motor impulsiveness (40) and the link of FTO to DA functioning. Additionally, we also measured structural connectivity between VTA/SN and the caudate nucleus, which is implicated in attentional impulsivity (64) but not primarily in motor impulsiveness, to verify the specificity of the expected effect of gene variant on connectivity between the midbrain and the NAc.
We found that connectivity between the VTA/SN and the NAc was increased in FTO risk allele carriers compared to non-carriers (F = 4.06, p = 0.044, Supplemental Table S3, Figure 2B) while we did not find a significant difference in structural connectivity between VTA/SN and the caudate (FTO+ vs FTO- group; F = 1.17, p = 0.283, Supplemental Table S3). Neither VTA/SN – NAc nor VTA/SN-caudate connectivity nor the effect of FTO risk allele carrier status on either connectivity measurement differed between sexes (Supplemental Table S3).
Increased structural connectivity between the midbrain and the nucleus accumbens mediates the effect of FTO risk allele carrier status on heightened motor impulsiveness
To assess, whether increased connectivity in meso-striatal pathways in FTO risk allele carriers contributes to the greater motor impulsivity also observed in the FTO+ group, we applied a mediation analysis. We demonstrate that increased structural connectivity between VTA/SN and NAc mediates the effect of allele status on motor impulsivity. Specifically, 21% of the FTO effect on motor impulsivity was mediated by the structural connectivity between the VTA/SN and NAc (Supplemental Tables S4, S5, Figure 2C), indicating a hardwired predisposition, that may well relate to neurodevelopmental alterations in FTO risk allele carriers and contribute to their impulsive phenotype.
Discussion
The obesity-promoting allele of FTO is a common polymorphism with a minor allele frequency of 40–45% in European ancestry populations and has a relatively large effect on BMI [0.35 kg/m2 per allele; equivalent to 1 kg for a person who is 1.7 m tall; 2]. However, the mechanisms by which FTO risk allele carrier status causes weight gain and weight-gain-promoting behavior are not fully unraveled yet. We reveal, that increased impulsivity of FTO risk allele carriers is partially mediated by increased structural connectivity between the encoding brain regions —the DA midbrain and the ventral striatum - indicating that structural alterations in FTO risk allele carriers might contribute to their obesity-promoting behavioral phenotype.
Our result demonstrating greater impulsivity in FTO risk allele carriers is in line with previous studies reporting alterations in impulsivity for FTO polymorphism (32–34). Impulsivity has been conceptualized as both a trait being an enduring facet of the personality as well as a transient state relating to a reaction to specific conditions in an environment (67). Both of these aspects of impulsive behavior have been shown to relate to obesity (68–70). From the structural perspective, we focused on trait impulsivity, which is the main readout of the Barrattt Impulsivity Scale. In our data, FTO risk allele carrier status was specifically associated with greater motor impulsivity —the tendency to act without thinking. Motor impulsivity has been shown to interactively drive the risk of weight gain by increasing binge eating frequency and the preference for sweet snacks (71–73). In other words, if FTO risk allele status is associated with greater motor impulsivity, FTO risk allele carriers have a higher risk to engage in behavior that promotes body weight gain without immediately considering the health-adverse consequences.
Even though a number of studies have shown a clear link between FTO risk allele status and impulsivity, the direction of this effect is inconsistent with some reporting greater impulsivity, while others report reduced impulsivity in association with FTO variants (33, 74). In this context it is important to consider, that impulsivity is not a rigid construct. Hence the methodological constraints of quantifying such a complex concept limit comparability between studies (if different measures are used). In addition, the association of carrier status and impulsivity seems to depend on the developmental state: FTO risk allele carrier status was associated with lower impulsivity in childhood (74) and young adulthood (33), but the reduction of impulsivity associated with aging is lower in FTO variant carriers compared to non-carriers (32) indicating an ongoing effect of FTO on development possibly obscured by compensatory mechanisms during aging.
Our results further revealed increased structural connectivity between the midbrain and the ventral striatum in FTO risk allele carriers, for which the underlying mechanism is unclear. One potential mechanism could comprise the developmental effect of FTO on neuronal and oligodendrocyte plasticity. Structural connectivity measurements can be considered a tool for assessing the neurodevelopmental impact of genes on human phenotypes (75), as structural connectivity seems to be under stronger genetic control than functional connectivity (76). On a molecular level, this is evidenced by the central role of gene-expression gradients in guiding axons to their targets as the brain develops (77). Genetic effects are not uniformly distributed across the connectome, but the connectivity of some neural elements is under stronger genetic control than others (76). Our results indicate that FTO variants affect the neurodevelopment of the meso-striatal projections. We could not detect altered connectivity between the DA midbrain and the caudate, indicating a potential specificity of the neurodevelopmental effects of FTO polymorphisms. However, these findings need to be interpreted with care, as the indirect nature of structural connectivity estimates does not allow any insight into the underlying molecular effects of FTO variants on the development of DA projections. Recent evidence from animal work indicates that FTO polymorphisms affect the expression of axon guidance molecules of striatal projections (78) pointing toward one possible molecular mechanism. Other mechanisms might include alteration in neuroinflammation (79) stress sensitivity and expression of neurotrophic factors such as BDNF (13, 80).
We further show that the structural alterations between the midbrain and the ventral striatum partially mediate the impulsive phenotype of risk allele carriers. This finding extends previous reports of altered functional activation of the NAc during various behavioral tasks in risk allele carriers (28, 30, 34, 81) and assessments of functional coupling between VTA/SN and NAcc during reward learning (31). It denotes that FTO polymorphism exerts its effect on behavior most likely through alterations in neuroplasticity, ie. during connectional maturation causing a predisposition for certain behavioral traits in addition to altering DA functioning.
While the here investigated structural connectivity between the midbrain and ventral striatum is based on a strong molecular basis involving FTO in DA encoding of impulsivity in this circuitry (8), it needs to be kept in mind that impulsivity is a complex construct modulated and encoded by a multitude of brain circuitries, including the frontal cortex, for which a regulatory role of FTO cannot be precluded. Equally, while we neither find differences in attentional impulsivity nor in the structural connectivity of its encoding circuitry (the midbrain projections to the caudate), the genetic architecture of disease-associated FTO loci may involve extensive pleiotropy and shared allelic effects across tissues including temporally restricted effects. Indeed, the caudate has been established as one site of IRX3 expression, which is the long-range target of obesity-associated variants of FTO (26), rendering it a likely site-of-action of the obesity-associated FTO variants, for which the functional role has yet to be fully clarified and might extend to other behavioral facets than impulsivity.
In interpreting the results, it is important to consider, that we assessed structural connectivity and impulsivity in healthy-weight young adults ensuring that identified changes can be attributed to the FTO risk allele status and are not confounded by secondary effects of BMI, weight gain, or aging. This is particularly relevant because FTO expression is subject to nutritional regulation (82) indicating that environmental factors can interact with the genetic risk status and further complicate the association between allele status and related phenotype. As it is well established that obesity is a result of a Gene X environment interaction, investigating the mechanisms by which FTO polymorphisms drive body weight gain is critical to understand the pathophysiological underpinnings of overeating and establishing targeted treatment options. This is, in particular, relevant, since previous studies have demonstrated that a healthy lifestyle with a balanced diet and physical activity can attenuate the effect of FTO on obesity risk by approximately 30% (83–85). Moving forward, the use of genetic screenings can be considered to identify individuals at risk of gaining weight in an obesogenic environment and provide customized treatment options focusing on neurobehavioral adaptation and lifestyle-interventions.
Limitations
While the selection of our participants ensures the attribution of the observed effects to FTO risk allele carrier status, inviting exclusively non-obese participants might at the same time introduce a sampling bias, by including a specific group of participants, who despite carrying risk alleles, are able to avoid weight gain. Second, even though we here aimed for conclusive evidence to support our molecularly well-grounded hypothesis, as in all candidate gene studies, the sample-sized dependent achieved power (0.73, Table S4) might represent a noteworthy limitation (86). Future particularly molecular studies will validate whether the proposed theories and concepts about the neurodevelopmental role of FTO as a contributor to obesity-promoting behavioral traits hold true in various scenarios. Moreover, probabilistic tractography only provides an indirect measure of structural connectivity and is not sufficient to detect alterations on the axonal level (87). It further needs to be considered that the here investigated polymorphism rs9939609 is in strong linkage disequilibrium with two further SNPs [rs8050136 and rs9930506] in Europeans, rendering it impossible to fully attribute the observed phenotype to the rs9939609 SNP (88, 89). Intriguingly, in individuals of African descent, results linking rs9939609 to increased BMI are more heterogenous so that further studies a required to disentangle the effect of FTO polymorphisms in different ethnicities (90, 91). These limitations and possibly differential effects of the various FTO polymorphisms on neurodevelopment might contribute to the inconsistent findings so far linking FTO polymorphism with either altered structural connectivity (92, 93) or no connectivity changes (94). With increasing data availability and computational power, future (genome wide) association studies will be required to replicate the demonstrated relationship between allelic variation and brain connectivity across a more heterogenous sample including further (cortical) brain circuitries involved in encoding of impulsivity.
Overall, our data reveals that increased motor impulsiveness in FTO risk allele carriers is partially mediated by enhanced structural connectivity between the midbrain and ventral striatum indicating that FTO polymorphism affects obesity promoting behavior via structural alterations in addition to complementary mechanisms. Further studies are needed to scrutinize the underlying molecular mechanisms.
Data availability statement
The data reported in this study cannot be deposited in a public repository per GDPR and IRB data protection policies. To request access, please contact [Marc Tittgemeyer, Max Planck Institute for Metabolism Research, dGl0dGdlbWV5ZXJAc2YubXBnLmRl]. Data provision may include processed and unprocessed data and will require a data- sharing agreement.
Ethics statement
The studies involving human participants were reviewed and approved by the local ethics comittee of the medical faculty of the University of Cologne (10-228).The participants provided their written informed consent to parcipate in this study.
Author contributions
SET: Conceptualization, Software, Methodology, Formal analysis, Investigation, Writing; RH: Methodology, Software, Formal analysis, Validation, Writing; CM: Software; MT: Funding acquisition, Conceptualization, Supervision. All authors contributed to the article and approved the submitted version.
Acknowledgments
The authors wish to thank Patrick Weyer and Elke Bannemer for their outstanding support in data acquisition and Bertram Huth for his fantastic help with figure layout.
Conflict of interest
SET is supported by the Else-Kroener-Fresenius-Stiftung. MT is supported by funding from the German Center for Diabetes Research – Project-ID 82DZD00502 & 82DZD03C2G as well as by the Deutsche Forschungsgemeinschaft DFG, German Research Foundation under Germany’s Excellence Strategy – EXEC 2030 – 390661388.
The remaining authors declare that the research was conducted in the absence of any commercial or financial relationships that could be construed as a potential conflict of interest.
Supplementary material
The Supplementary Material for this article can be found online at: https://www.frontiersin.org/articles/10.3389/fendo.2023.1130203/full#supplementary-material
References
1. Hall KD, Farooqi IS, Friedman JM, Klein S, Loos RJF, Mangelsdorf DJ, et al. The energy balance model of obesity: beyond calories in, calories out. Am J Clin Nutr (2022) 115:1243–54. doi: 10.1093/ajcn/nqac031
2. Frayling TM, Timpson NJ, Weedon MN, Zeggini E, Freathy RM, Lindgren CM, et al. A common variant in the FTO gene is associated with body mass index and predisposes to childhood and adult obesity. Science (2007) 316:889–94. doi: 10.1126/science.1141634
3. Bollepalli S, Dolan LM, Deka R, Martin LJ. Association of FTO gene variants with adiposity in African-American adolescents. Obes (Silver Spring) (2010) 18:1959–63. doi: 10.1038/oby.2010.82
4. Loos RJ, Yeo GS. The bigger picture of FTO: the first GWAS-identified obesity gene. Nat Rev Endocrinol (2014) 10:51–61. doi: 10.1038/nrendo.2013.227
5. Scuteri A, Sanna S, Chen WM, Uda M, Albai G, Strait J, et al. Genome-wide association scan shows genetic variants in the FTO gene are associated with obesity-related traits. PloS Genet (2007) 3:e115. doi: 10.1371/journal.pgen.0030115
6. Wing MR, Ziegler J, Langefeld CD, Ng MC, Haffner SM, Norris JM, et al. Analysis of FTO gene variants with measures of obesity and glucose homeostasis in the IRAS family study. Hum Genet (2009) 125:615–26. doi: 10.1007/s00439-009-0656-3
7. Claussnitzer M, Dankel SN, Kim KH, Quon G, Meuleman W, Haugen C, et al. And adipocyte browning in humans. New Engl J Med (2015) 373:895–907. doi: 10.1056/NEJMoa1502214
8. Hess ME, Hess S, Meyer KD, Verhagen LA, Koch L, Brönneke HS, et al. The fat mass and obesity associated gene (Fto) regulates activity of the dopaminergic midbrain circuitry. Nat Neurosci (2013) 16:1042–8. doi: 10.1038/nn.3449
9. Boissel S, Reish O, Proulx K, Kawagoe-Takaki H, Sedgwick B, Yeo GS, et al. Loss-of-function mutation in the dioxygenase-encoding FTO gene causes severe growth retardation and multiple malformations. Am J Hum Genet (2009) 85:106–11. doi: 10.1016/j.ajhg.2009.06.002
10. Cao Y, Zhuang Y, Chen J, Xu W, Shou Y, Huang X, et al. Dynamic effects of fto in regulating the proliferation and differentiation of adult neural stem cells of mice. Hum Mol Genet (2020) 29:727–35. doi: 10.1093/hmg/ddz274
11. Chen J, Zhang YC, Huang C, Shen H, Sun B, Cheng X, et al. m(6)A regulates neurogenesis and neuronal development by modulating histone methyltransferase Ezh2. Genomics Proteomics Bioinf (2019) 17:154–68. doi: 10.1016/j.gpb.2018.12.007
12. Gao H, Cheng X, Chen J, Ji C, Guo H, Qu W, et al. Fto-modulated lipid niche regulates adult neurogenesis through modulating adenosine metabolism. Hum Mol Genet (2020) 29:2775–87. doi: 10.1093/hmg/ddaa171
13. Li L, Zang L, Zhang F, Chen J, Shen H, Shu L, et al. Fat mass and obesity-associated (FTO) protein regulates adult neurogenesis. Hum Mol Genet (2017) 26:2398–411. doi: 10.1093/hmg/ddx128
14. Shu L, Huang X, Cheng X, Li X. Emerging roles of N6-methyladenosine modification in neurodevelopment and neurodegeneration. Cells 10 (2021) 10(10):2694. doi: 10.3390/cells10102694
15. Gray J, Yeo GS, Cox JJ, Morton J, Adlam AL, Keogh JM, et al. Hyperphagia, severe obesity, impaired cognitive function, and hyperactivity associated with functional loss of one copy of the brain-derived neurotrophic factor (BDNF) gene. Diabetes (2006) 55:3366–71. doi: 10.2337/db06-0550
16. Speliotes EK, Willer CJ, Berndt SI, Monda KL, Thorleifsson G, Jackson AU, et al. Association analyses of 249,796 individuals reveal 18 new loci associated with body mass index. Nat Genet (2010) 42:937–48. doi: 10.1038/ng.686
17. Wang P, Loh KH, Wu M, Morgan DA, Schneeberger M, Yu X, et al. A leptin-BDNF pathway regulating sympathetic innervation of adipose tissue. Nature (2020) 583:839–44. doi: 10.1038/s41586-020-2527-y
18. Yeo GS, Connie Hung CC, Rochford J, Keogh J, Gray J, Sivaramakrishnan S, et al. A de novo mutation affecting human TrkB associated with severe obesity and developmental delay. Nat Neurosci (2004) 7:1187–9. doi: 10.1038/nn1336
19. Fischer J, Koch L, Emmerling C, Vierkotten J, Peters T, Brüning JC, et al. Inactivation of the fto gene protects from obesity. Nature (2009) 458:894–8. doi: 10.1038/nature07848
20. Wu R, Li A, Sun B, Sun JG, Zhang J, Zhang T, et al. A novel m(6)A reader Prrc2a controls oligodendroglial specification and myelination. Cell Res (2019) 29:23–41. doi: 10.1038/s41422-018-0113-8
21. Stratigopoulos G, Padilla SL, LeDuc CA, Watson E, Hattersley AT, McCarthy MI, et al. Regulation of Fto/Ftm gene expression in mice and humans. Am J Physiol Regul Integr Comp Physiol (2008) 294:R1185–96. doi: 10.1152/ajpregu.00839.2007
22. Stratigopoulos G, LeDuc CA, Cremona ML, Chung WK, Leibel RL. Cut-like homeobox 1 (CUX1) regulates expression of the fat mass and obesity-associated and retinitis pigmentosa GTPase regulator-interacting protein-1-like (RPGRIP1L) genes and coordinates leptin receptor signaling. J Biol Chem (2011) 286:2155–70. doi: 10.1074/jbc.M110.188482
23. Stratigopoulos G, Martin Carli JF, O’Day DR, Wang L, Leduc CA, Lanzano P, et al. Hypomorphism for RPGRIP1L, a ciliary gene vicinal to the FTO locus, causes increased adiposity in mice. Cell Metab (2014) 19:767–79. doi: 10.1016/j.cmet.2014.04.009
24. Sobreira DR, Joslin AC, Zhang Q, Williamson I, Hansen GT, Farris KM, et al. Extensive pleiotropism and allelic heterogeneity mediate metabolic effects of IRX3 and IRX5. Science (2021) 372:1085–91. doi: 10.1126/science.abf1008
25. Chen JA, Huang YP, Mazzoni EO, Tan GC, Zavadil J, Wichterle H. Mir-17-3p controls spinal neural progenitor patterning by regulating Olig2/Irx3 cross-repressive loop. Neuron (2011) 69:721–35. doi: 10.1016/j.neuron.2011.01.014
26. Smemo S, Tena JJ, Kim KH, Gamazon ER, Sakabe NJ, Gómez-Marín C, et al. Obesity-associated variants within FTO form long-range functional connections with IRX3. Nature (2014) 507:371–5. doi: 10.1038/nature13138
27. Cecil JE, Tavendale R, Watt P, Hetherington MM, Palmer CN. An obesity-associated FTO gene variant and increased energy intake in children. New Engl J Med (2008) 359:2558–66. doi: 10.1056/NEJMoa0803839
28. Melhorn SJ, Askren MK, Chung WK, Kratz M, Bosch TA, Tyagi V, et al. FTO genotype impacts food intake and corticolimbic activation. Am J Clin Nutr (2018) 107:145–54. doi: 10.1093/ajcn/nqx029
29. Dang LC, Samanez-Larkin GR, Smith CT, Castrellon JJ, Perkins SF, Cowan RL, et al. FTO affects food cravings and interacts with age to influence age-related decline in food cravings. Physiol Behav (2018) 192:188–93. doi: 10.1016/j.physbeh.2017.12.013
30. Kühn AB, Feis DL, Schilbach L, Kracht L, Hess ME, Mauer J, et al. FTO gene variant modulates the neural correlates of visual food perception. Neuroimage (2016) 128:21–31. doi: 10.1016/j.neuroimage.2015.12.049
31. Sevgi M, Rigoux L, Kühn AB, Mauer J, Schilbach L, Hess ME, et al. An obesity-predisposing variant of the FTO gene regulates D2R-dependent reward learning. J Neurosci (2015) 35:12584–92. doi: 10.1523/JNEUROSCI.1589-15.2015
32. Chuang YF, Tanaka T, Beason-Held LL, An Y, Terracciano A, Sutin AR, et al. FTO genotype and aging: pleiotropic longitudinal effects on adiposity, brain function, impulsivity and diet. Mol Psychiatry (2015) 20:133–39. doi: 10.1038/mp.2014.49
33. Meyre D, Mohamed S, Gray JC, Weafer J, MacKillop J, de Wit H. Association between impulsivity traits and body mass index at the observational and genetic epidemiology level. Sci Rep (2019) 9:17583. doi: 10.1038/s41598-019-53922-8
34. Wiemerslage L, Nilsson EK, Solstrand Dahlberg L, Ence-Eriksson F, Castillo S, Larsen AL, et al. An obesity-associated risk allele within the FTO gene affects human brain activity for areas important for emotion, impulse control and reward in response to food images. Eur J Neurosci (2016) 43:1173–80. doi: 10.1111/ejn.13177
35. Bari A, Mar AC, Theobald DE, Elands SA, Oganya KC, Eagle DM, et al. Prefrontal and monoaminergic contributions to stop-signal task performance in rats. J Neurosci (2011) 31:9254–63. doi: 10.1523/JNEUROSCI.1543-11.2011
36. Bari A, Robbins TW. Noradrenergic versus dopaminergic modulation of impulsivity, attention and monitoring behaviour in rats performing the stop-signal task: possible relevance to ADHD. Psychopharmacol (Berl) (2013) 230:89–111. doi: 10.1007/s00213-013-3141-6
37. Granon S, Passetti F, Thomas KL, Dalley JW, Everitt BJ, Robbins TW. Enhanced and impaired attentional performance after infusion of D1 dopaminergic receptor agents into rat prefrontal cortex. J Neurosci (2000) 20:1208–15. doi: 10.1523/JNEUROSCI.20-03-01208.2000
38. van Gaalen MM, Brueggeman RJ, Bronius PF, Schoffelmeer AN, Vanderschuren LJ. Behavioral disinhibition requires dopamine receptor activation. Psychopharmacol (Berl) (2006) 187:73–85. doi: 10.1007/s00213-006-0396-1
39. Meule A, Blechert J. Interactive and indirect effects of trait impulsivity facets on body mass index. Appetite (2017) 118:60–5. doi: 10.1016/j.appet.2017.07.023
40. Smith CT, San Juan MD, Dang LC, Katz DT, Perkins SF, Burgess LL, et al. Ventral striatal dopamine transporter availability is associated with lower trait motor impulsivity in healthy adults. Transl Psychiatry (2018) 8:269. doi: 10.1038/s41398-018-0328-y
41. McAuley KA, Mann JI, Chase JG, Lotz TF, Shaw GM. Point: HOMA–satisfactory for the time being: HOMA: the best bet for the simple determination of insulin sensitivity, until something better comes along. Diabetes Care (2007) 30:2411–3. doi: 10.2337/dc07-1067
42. Preuss UW, Rujescu D, Giegling I, Watzke S, Koller G, Zetzsche T, et al. [Psychometric evaluation of the German version of the barratt impulsiveness scale]. Nervenarzt (2008) 79:305–19. doi: 10.1007/s00115-007-2360-7
43. Stanford MS, Mathias CW, Dougherty DM, Lake SL, Anderson NE, Patton JH. Fifty years of the barratt impulsiveness scale: an update and review. Pers Individ Dif (2009) 47:385–95. doi: 10.1016/j.paid.2009.04.008
44. Reese TG, Heid O, Weisskoff RM, Wedeen VJ. Reduction of eddy-current-induced distortion in diffusion MRI using a twice-refocused spin echo. Magn Reson Med (2003) 49:177–82. doi: 10.1002/mrm.10308
46. Naidich TP, Duvernoy HM, Delman BN, Sorensen AG, Kollias SS, Haacke EM. Duvernoy’s atlas of the human brain stem and cerebellum: high-field MRI, surface anatomy, internal structure, vascularization and 3D sectional anatomy. Wien New York: Springer (2009).
47. Pelzer EA, Melzer C, Schönberger A, Hess M, Timmermann L, Eggers C, et al. Axonal degeneration in parkinson’s disease - basal ganglia circuitry and D2 receptor availability. NeuroImage Clin (2019) 23:101906. doi: 10.1016/j.nicl.2019.101906
48. Frazier JA, Chiu S, Breeze JL, Makris N, Lange N, Kennedy DN, et al. Structural brain magnetic resonance imaging of limbic and thalamic volumes in pediatric bipolar disorder. Am J Psychiatry (2005) 162:1256–65. doi: 10.1176/appi.ajp.162.7.1256
49. Makris N, Goldstein JM, Kennedy D, Hodge SM, Caviness VS, Faraone SV, et al. Decreased volume of left and total anterior insular lobule in schizophrenia. Schizophr Res (2006) 83:155–71. doi: 10.1016/j.schres.2005.11.020
50. Andersson JLR JM, Smith S. TR07JA2: non-linear registration, aka spatial normalisation. FMRIB Anal Group Tech Rep (2007).
51. Dyrby TB, Lundell H, Burke MW, Reislev NL, Paulson OB, Ptito M, et al. Interpolation of diffusion weighted imaging datasets. Neuroimage (2014) 103:202–13. doi: 10.1016/j.neuroimage.2014.09.005
52. Smith RE, Tournier JD, Calamante F, Connelly A. Anatomically-constrained tractography: improved diffusion MRI streamlines tractography through effective use of anatomical information. Neuroimage (2012) 62:1924–38. doi: 10.1016/j.neuroimage.2012.06.005
53. Jenkinson M, Smith S. A global optimisation method for robust affine registration of brain images. Med Image Anal (2001) 5:143–56. doi: 10.1016/S1361-8415(01)00036-6
54. Andersson JLR, Skare S, Ashburner J. How to correct susceptibility distortions in spin-echo echo-planar images: application to diffusion tensor imaging. Neuroimage (2003) 20:870–88. doi: 10.1016/S1053-8119(03)00336-7
55. Behrens TE, Berg HJ, Jbabdi S, Rushworth MF, Woolrich MW. Probabilistic diffusion tractography with multiple fibre orientations: what can we gain? Neuroimage (2007) 34:144–55. doi: 10.1016/j.neuroimage.2006.09.018
56. Behrens TE, Woolrich MW, Jenkinson M, Johansen-Berg H, Nunes RG, Clare S, et al. Characterization and propagation of uncertainty in diffusion-weighted MR imaging. Magn Reson Med (2003) 50:1077–88. doi: 10.1002/mrm.10609
57. Stephan KE, Tittgemeyer M, Knösche TR, Moran RJ, Friston KJ. Tractography-based priors for dynamic causal models. Neuroimage (2009) 47:1628–38. doi: 10.1016/j.neuroimage.2009.05.096
58. Pelzer EA, Hintzen A, Goldau M, von Cramon DY, Timmermann L, Tittgemeyer M. Cerebellar networks with basal ganglia: feasibility for tracking cerebello-pallidal and subthalamo-cerebellar projections in the human brain. Eur J Neurosci (2013) 38:3106–14. doi: 10.1111/ejn.12314
60. R Core Team. R: a language and environment for statistical computing. R Foundation for Statistical Computing (2017).
61. Tingley D, Yamamoto T, Hirose K, Keele L, Imai K. {mediation}: {R} package for causal mediation analysis. J Stat Softw (2014) 59:1–38. doi: 10.18637/jss.v059.i05
62. Ben-Shachar MS, Lüdecke D, Makowski D. Estimation of effect size indices and standardized parameters. J Open Source Softw (2020) 5:2815. doi: 10.21105/joss.02815
63. Faul F, Erdfelder E, Lang A-G, Buchner A. G*Power 3: a flexible statistical power analysis program for the social, behavioral, and biomedical sciences. Behav Res Methods (2007) 39:175–91. doi: 10.3758/BF03193146
64. Dang LC, Samanez-Larkin GR, Young JS, Cowan RL, Kessler RM, Zald DH. Caudate asymmetry is related to attentional impulsivity and an objective measure of ADHD-like attentional problems in healthy adults. Brain Struct Funct (2016) 221:277–86. doi: 10.1007/s00429-014-0906-6
65. Kühner C, Bürger C, Keller F, Hautzinger M. [Reliability and validity of the revised beck depression inventory (BDI-II). Results German samples]. Nervenarzt (2007) 78:651–6. doi: 10.1007/s00115-006-2098-7
66. Saunders JB, Aasland OG, Babor TF, de la Fuente JR, Grant M. Development of the alcohol use disorders identification test (AUDIT): WHO collaborative project on early detection of persons with harmful alcohol consumption–II. Addiction (1993) 88:791–804. doi: 10.1111/j.1360-0443.1993.tb02093.x
67. Ruddock HK, Field M, Jones A, Hardman CA. State and trait influences on attentional bias to food-cues: the role of hunger, expectancy, and self-perceived food addiction. Appetite (2018) 131:139–47. doi: 10.1016/j.appet.2018.08.038
68. Benard M, Camilleri GM, Etile F, Mejean C, Bellisle F, Reach G, et al. Association between impulsivity and weight status in a general population. Nutrients (2017) 9(3):217. doi: 10.3390/nu9030217
69. Testa G, Mora-Maltas B, Camacho-Barcia L, Granero R, Lucas I, Agüera Z, et al. Transdiagnostic perspective of impulsivity and compulsivity in obesity: from cognitive profile to self-reported dimensions in clinical samples with and without diabetes. Nutrients (2021) 13(12):4426. doi: 10.3390/nu13124426
70. VanderBroek-Stice L, Stojek MK, Beach SRH, vanDellen MR, MacKillop J. Multidimensional assessment of impulsivity in relation to obesity and food addiction. Appetite (2017) 112:59–68. doi: 10.1016/j.appet.2017.01.009
71. Kakoschke N, Kemps E, Tiggemann M. External eating mediates the relationship between impulsivity and unhealthy food intake. Physiol Behav (2015) 147:117–21. doi: 10.1016/j.physbeh.2015.04.030
72. Meule A, Hofmann J, Weghuber D, Blechert J. Impulsivity, perceived self-regulatory success in dieting, and body mass in children and adolescents: a moderated mediation model. Appetite (2016) 107:15–20. doi: 10.1016/j.appet.2016.07.022
73. Meule A, Platte P. Facets of impulsivity interactively predict body fat and binge eating in young women. Appetite (2015) 87:352–7. doi: 10.1016/j.appet.2015.01.003
74. Velders FP, De Wit JE, Jansen PW, Jaddoe VW, Hofman A, Verhulst FC, et al. FTO at rs9939609, food responsiveness, emotional control and symptoms of ADHD in preschool children. PloS One (2012) 7:e49131. doi: 10.1371/journal.pone.0049131
75. Hirschhorn JN, Daly MJ. Genome-wide association studies for common diseases and complex traits. Nat Rev Genet (2005) 6:95–108. doi: 10.1038/nrg1521
76. Arnatkeviciute A, Fulcher BD, Bellgrove MA, Fornito A. Where the genome meets the connectome: understanding how genes shape human brain connectivity. Neuroimage (2021) 244:118570. doi: 10.1016/j.neuroimage.2021.118570
77. Dickson BJ. Molecular mechanisms of axon guidance. Science (2002) 298:1959–64. doi: 10.1126/science.1072165
78. Qi Z, Wang S, Li J, Wen Y, Cui R, Zhang K, et al. Protective role of mRNA demethylase FTO on axon guidance molecules of nigro-striatal projection system in manganese-induced parkinsonism. J Hazard Mater (2022) 426:128099. doi: 10.1016/j.jhazmat.2021.128099
79. Rasheed M, Liang J, Wang C, Deng Y, Chen Z. Epigenetic regulation of neuroinflammation in parkinson’s disease. Int J Mol Sci (2021) 22(9):4956. doi: 10.3390/ijms22094956
80. Spychala A, Rüther U. FTO affects hippocampal function by regulation of BDNF processing. PloS One (2019) 14:e0211937. doi: 10.1371/journal.pone.0211937
81. Rapuano KM, Zieselman AL, Kelley WM, Sargent JD, Heatherton TF, Gilbert-Diamond D. Genetic risk for obesity predicts nucleus accumbens size and responsivity to real-world food cues. Proc Natl Acad Sci U.S.A. (2017) 114:160–5. doi: 10.1073/pnas.1605548113
82. Cheung MK, Gulati P, O’Rahilly S, Yeo GS. FTO expression is regulated by availability of essential amino acids. Int J Obes (Lond) (2013) 37:744–7. doi: 10.1038/ijo.2012.77
83. Vimaleswaran KS, Li S, Zhao JH, Luan J, Bingham SA, Khaw KT, et al. Physical activity attenuates the body mass index-increasing influence of genetic variation in the FTO gene. Am J Clin Nutr (2009) 90:425–8. doi: 10.3945/ajcn.2009.27652
84. Kilpeläinen TO, Qi L, Brage S, Sharp SJ, Sonestedt E, Demerath E, et al. Physical activity attenuates the influence of FTO variants on obesity risk: a meta-analysis of 218,166 adults and 19,268 children. PloS Med (2011) 8:e1001116. doi: 10.1371/journal.pmed.1001116
85. Scott RA, Bailey ME, Moran CN, Wilson RH, Fuku N, Tanaka M, et al. FTO genotype and adiposity in children: physical activity levels influence the effect of the risk genotype in adolescent males. Eur J Hum Genet (2010) 18:1339–43. doi: 10.1038/ejhg.2010.131
86. Costa KM, Schoenbaum G. Replication efforts have limited epistemic value. Nature (2021) 599:201. doi: 10.1038/d41586-021-03068-3
87. Tittgemeyer M, Rigoux L, Knösche TR. Cortical parcellation based on structural connectivity: a case for generative models. Neuroimage (2018) 173:592–603. doi: 10.1016/j.neuroimage.2018.01.077
88. Elouej S, Belfki-Benali H, Nagara M, Lasram K, Attaoua R, Sallem OK, et al. Association of rs9939609 polymorphism with metabolic parameters and FTO risk haplotype among Tunisian metabolic syndrome. Metab Syndr Relat Disord (2016) 14:121–8. doi: 10.1089/met.2015.0090
89. Kalantari N, Keshavarz Mohammadi N, Izadi P, Gholamalizadeh M, Doaei S, Eini-Zinab H, et al. A complete linkage disequilibrium in a haplotype of three SNPs in fat mass and obesity associated (FTO) gene was strongly associated with anthropometric indices after controlling for calorie intake and physical activity. BMC Med Genet (2018) 19:146. doi: 10.1186/s12881-018-0664-z
90. Hennig BJ, Fulford AJ, Sirugo G, Rayco-Solon P, Hattersley AT, Frayling TM, et al. FTO gene variation and measures of body mass in an African population. BMC Med Genet (2009) 10:21. doi: 10.1186/1471-2350-10-21
91. Liu G, Zhu H, Lagou V, Gutin B, Stallmann-Jorgensen IS, Treiber FA, et al. FTO variant rs9939609 is associated with body mass index and waist circumference, but not with energy intake or physical activity in European- and African-American youth. BMC Med Genet (2010) 11:57. doi: 10.1186/1471-2350-11-57
92. Lugo-Candelas C, Pang Y, Lee S, Cha J, Hong S, Ranzenhofer L, et al. Differences in brain structure and function in children with the FTO obesity-risk allele. Obes Sci Pract (2020) 6:409–24. doi: 10.1002/osp4.417
93. Olivo G, Latini F, Wiemerslage L, Larsson EM, Schiöth HB. Disruption of accumbens and thalamic white matter connectivity revealed by diffusion tensor tractography in young men with genetic risk for obesity. Front Hum Neurosci (2018) 12:75. doi: 10.3389/fnhum.2018.00075
Keywords: FTO, dopamine, connectivity, impulsivity, structure, nucleus accumbens
Citation: Edwin Thanarajah S, Hanssen R, Melzer C and Tittgemeyer M (2023) Increased meso-striatal connectivity mediates trait impulsivity in FTO variant carriers. Front. Endocrinol. 14:1130203. doi: 10.3389/fendo.2023.1130203
Received: 22 December 2022; Accepted: 31 March 2023;
Published: 08 May 2023.
Edited by:
Reina Villareal, Baylor College of Medicine, United StatesReviewed by:
Fnu Deepika, Baylor College of Medicine, United StatesLaura Dearden, University of Cambridge, United Kingdom
Copyright © 2023 Edwin Thanarajah, Hanssen, Melzer and Tittgemeyer. This is an open-access article distributed under the terms of the Creative Commons Attribution License (CC BY). The use, distribution or reproduction in other forums is permitted, provided the original author(s) and the copyright owner(s) are credited and that the original publication in this journal is cited, in accordance with accepted academic practice. No use, distribution or reproduction is permitted which does not comply with these terms.
*Correspondence: Sharmili Edwin Thanarajah, U2hhcm1pbGkuZWR3aW50aGFuYXJhamFoQGtndS5kZQ==
†These authors have contributed equally to this work