- 1Graduate school, Tianjin University of Traditional Chinese Medicine, Tianjin, China
- 2Dermatology and Medical Cosmetology Department, Laishan branch of Yantai Yuhuangding Hopitali, Shandong, China
- 3Department of Dermatology, Tianjin Academy of Traditional Chinese Medicine Affiliated Hospital, Tianjin, China
Background: This study aimed to develop an autoimmune thyroid disease (AITD) risk prediction model for patients with vitiligo based on readily available characteristics.
Methods: A retrospective analysis was conducted on the clinical characteristics, demographics, skin lesions, and laboratory test results of patients with vitiligo. To develop a model to predict the risk of AITD, the Least Absolute Shrinkage and Selection Operator (LASSO) method was used to optimize feature selection, and logistic regression analysis was used to select further features. The C-index, Hosmer–Lemeshow test, and decision curve analysis were used to evaluate the calibration, discrimination ability and clinical utility of the model. Internally, the model was verified using bootstrapping; externally, two independent cohorts were used to confirm model accuracy.
Results: Sex, vitiligo type, family history of AITD, family history of other autoimmune disease, thyroid nodules or tumors, negative emotions, skin involvement exceeding 5% of body surface area, and positive immune serology (IgA, IgG, IgM, C3, and C4) were predictors of AITD in the prediction nomogram. The model showed good calibration and discrimination (C-index: 0.746; 95% confidence interval: 0.701–0.792). The accuracy of this predictive model was 74.6%.In both internal validation (a C-index of 1000 times) and external validation, the C-index outperformed (0.732, 0.869, and 0.777). The decision curve showed that the AITD nomogram had a good guiding role in clinical practice.
Conclusion: The novel AITD nomogram effectively evaluated the risk of AITD in patients with vitiligo.
1 Introduction
Vitiligo is a depigmenting skin disease that is prevalent in the population (1). The cause is currently unknown, but the most widely accepted theory is an autoimmune etiology; this has been supported by epidemiological, clinical, and experimental investigations (2–10). Epidemiologic reports have also confirmed that vitiligo is present in the autoimmune polyendocrine syndrome (11). Autoimmune thyroid disease (AITD) presents primarily as two clinical manifestations: Hashimoto’s thyroiditis and Graves’ disease (12). The prevalence of AITD in patients with vitiligo is 14.3% (13); in contrast, the prevalence of vitiligo in patients with AITD ranges from 2.7%–6.8% (14, 15). Currently, there is controversy regarding the order in which vitiligo and AITD arise, although some studies have suggested that vitiligo appears earlier (16–19). Others have reported that the probability of thyroid disease in patients with vitiligo increases with age (17). At this time, there is no clinical treatment for the source of AITD; in addition, prolonged AITD leads to serious and life-threatening complications (20). Therefore, there is a need to develop a model to identify, diagnose, and treat the damage caused by AITD at an early stage of the disease, and to predict whether a person will eventually experience AITD.
Cumulative studies have reported many factors closely related to the pathogenesis of AITD. A large proportion of women experience AITD; this may be owing to an important role of estrogen in the pathogenesis of AITD (21). In addition, the onset of AITD has often shown familial clustering and thus, common causative factors are under consideration (22). Patients with vitiligo have also been found more likely to have AITD when their immunoglobulin plus complement tests (IgA, IgG, IgM, C3, and C4) were abnormal, when they had thyroid nodules or tumors, and also when the Positive and Negative Affect Schedule (PANAS) assessment registered negative emotions (12, 23, 24). Another study has reported that different types of vitiligo have varying probability of developing AITD; this may be related to different clinical manifestations and pathogenesis (25). Patients with non-segmental vitiligo (NSV) are more likely to develop AITD.
This study aimed to provide an accurate and straightforward model to predict the presence of AITD in patients with vitiligo, using features and clinical symptoms that are simple for patients to understand. This predictive model can be helpful for the education and individualized treatment of patients with high-risk AITD; it might also reduce the burden of medical insurance and thus, ease communication and exchange of patients. In addition, this study and model might help to avoid the problem of overtreatment in the large population of vitiligo patients in China with varying socioeconomic status.
2 Materials and methods
2.1 Patients
We collected data from patients visiting the outpatient dermatology department at the Affiliated Hospital of the Tianjin Academy of Traditional Chinese Medicine between January 2019 and December 2020. Data collected from the same department between January 2021 and May 2022 were considered as external verification (Group 1). Patient data collected between January and December 2021 at the Yantai YuHuangDing Hospital were considered as external validation (Group 2). All patients had completed a detailed survey within the medical record including demographic characteristics, family history of vitiligo and AITD, history of smoking and drinking, and other basic AITD risk factor information. Follow-up data were collected at return visits and through telephone surveys. Two dermatologists made the diagnosis of vitiligo based on imaging results, such as skin confocal laser scanning microscopy, Wood’s lamp, or dermoscopy. Physicians and dermatologists diagnosed AITD based on clinical observations and laboratory tests. This study was approved by the Ethics Committee of the Affiliated Hospital of Tianjin Academy of Traditional Chinese Medicine, Tianjin, China. This was a retrospective study, approved by the Ethics Committee, and the need for informed consent was waived.
2.2 Evaluation criteria
Clinical vitiligo can be classified as segmental (SV), NSV, and mixed vitiligo (26). Typical SV displays a unilateral distribution, while NSV presents with bilaterally symmetrical white macules (24). The PANAS (27) emotional scale was used to evaluate the current emotional state of patients. Laboratory examinations assessed positive immunoglobulin and complement (IgA, IgG, IgM, C3, and C4) levels. Whether the area of vitiligo exceeded 5% of the entire body area was assessed according to the area of the palm of the hand. Unilateral palm area is approximately equal to 1%.The remaining parameters were obtained through patient interview or case review. Most indicators mentioned in this article were for the etiological determination and data retrieval required for vitiligo patients and did not add additional financial burden to the patient.
2.3 Statistical analysis
All information is reported as count (%), including demographic details, laboratory results, clinical symptoms, and vitiligo phases. SPSS (version 26.0; http://www.spss.com.cn), STATA (version 15.0; https://www.stata.com), and R (version 3.6.3; https://www.R-project.org) software were used for statistical analyses. All the above factors were included within the Least Absolute Shrinkage and Selection Operator (LASSO) method to reduce the dimensionality of the data (28). These were appropriate for choosing the top AITD risk-predicting features. In the LASSO regression model (29), we selected 11 features with non-zero coefficients, and statistical software was inputted to establish the prediction model using multivariate and univariate logistic regression analyses. We used odds ratios (ORs) and p-values with 95% confidence interval (CI). A predictive model for AITD was developed using the variables with a univariate p-value < 0.05 and a multivariate p-value in the logistic regression analysis of the optimal model. The Hosmer–Lemeshow test was used to evaluate calibration of the AITD nomogram. The C-index was calculated to measure the AITD nomogram’s discrimination performance. To measure the predictive power of the nomogram, the area under the curve (AUC) was plotted. The AITD nomograms were validated by bootstrapping (1000 bootstrapping resampling) to calculate the relative corrected C-index (30). To determine the C-index, two different queues (queues 1 and 2) were used to validate external validation. By measuring the net rate of return under various threshold probabilities using a decision curve analysis (DCA), the clinical value of the AITD nomogram was evaluated (31).
3 Results
3.1 Features of vitiligo and autoimmune thyroid disease
In this retrospective study, we reviewed the data of 703 patients with vitiligo, of whom 239 had concomitant AITD. All patient data, including demographic characteristics, laboratory tests, clinical manifestations, and stages of vitiligo and other information, are shown in Table 1. Regarding external validation, the Group 1 data were from 156 patients with vitiligo, of whom 55 had concomitant AITD. The Group 2 data comprised that of 42 patients with vitiligo and 14 with concomitant AITD.
An increasing number of researchers have found that many factors associated with vitiligo are also related to the onset of AITD (32, 33); therefore, we reviewed the results of the data for these. Among vitiligo patients, 165 female patients (69%), 197 patients (83%) with NSV, 208 patients (87%) with thyroid nodules or tumors, 114 patients (48%) with negative emotions, 35 patients (15%) with > 5% of the skin affected, and 49 patients (21%) with positive immune serology displayed AITD symptoms. Patients with vitiligo combined family history of AITD were found in 28 cases (12%). Twenty-nine cases (12%) had AITD, who had patients with vitiligo with a history of autoimmune diseases except AITD (Table 1).
3.2 Predictor selection
We selected the following 11 potential predictors in the 703 patients with vitiligo (Figures 1, 2), including sex, age, vitiligo type, family history of AITD, family medical history of non-thyroid autoimmune diseases, thyroid nodules or tumors, PANAS evaluation, smoking history, alcohol history, vitiligo involvement > 5% of body area, and immune serology (immunoglobulins and complement) (Table 2).
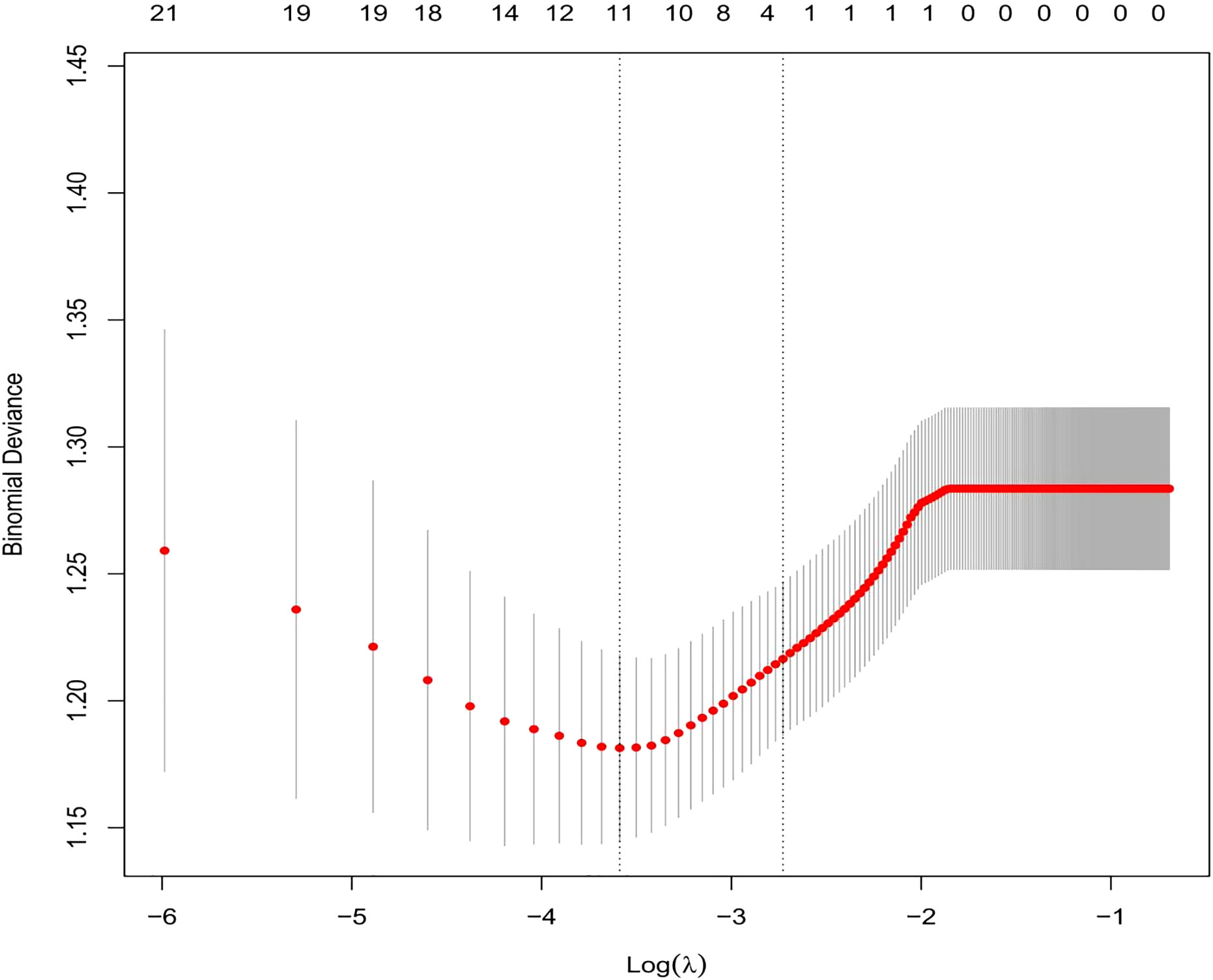
Figure 1 By using the five-weight verification method, the optimal parameter (Lambda) (29) can be selected in the LASSO model. Using partially mild deviation curves (two deviations) and paired graphs (Lambda), a virtual line is drawn vertically at the optimal value to determine the characteristic factor.
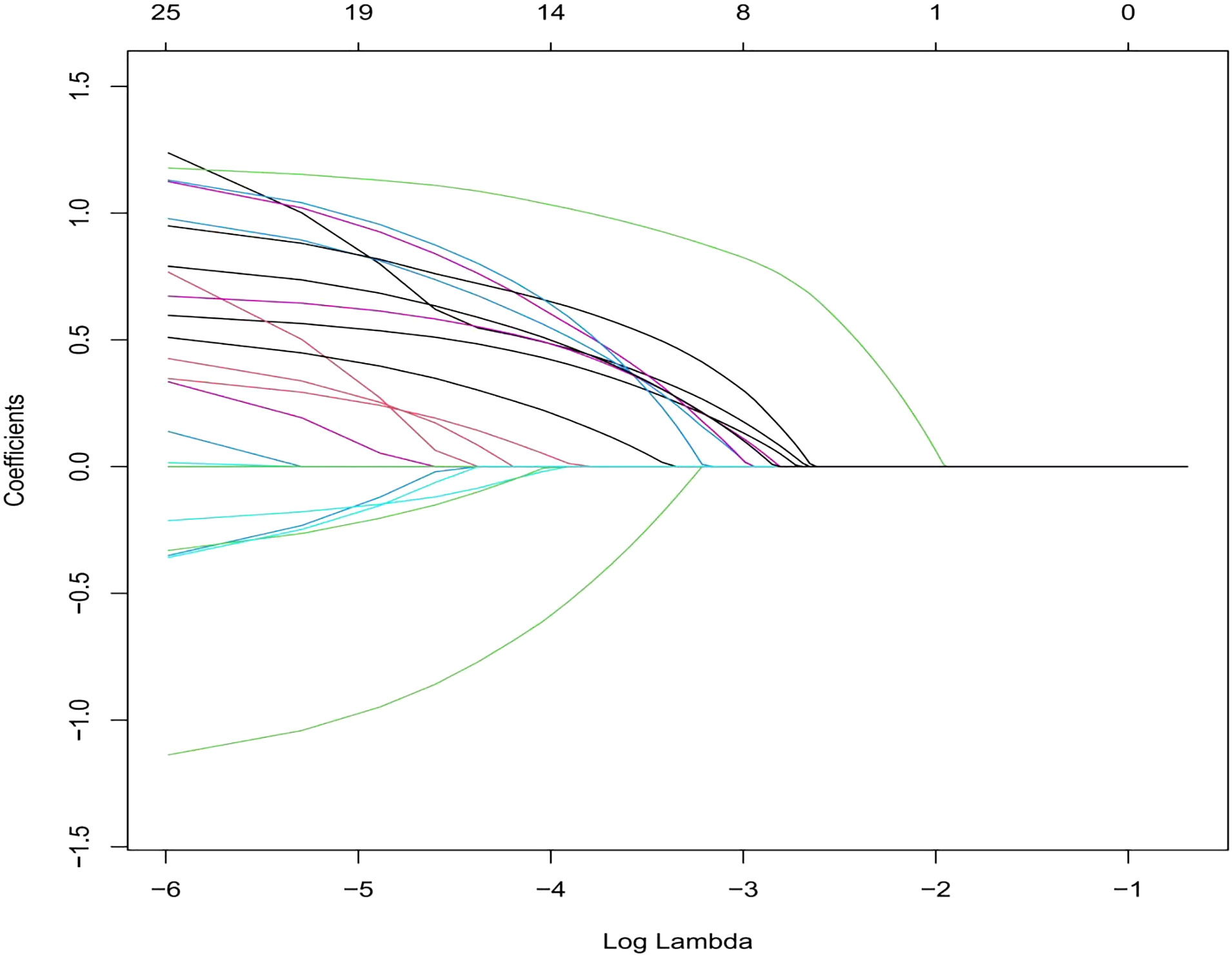
Figure 2 This section consists of 11 characteristics based on the sequence of the number (Lambda) sequence. A vertical line is drawn at the selected value using five weights cross-verification, and the coefficient of five elements with non-zero values is selected.
3.3 Construction of the personalized prediction model
Table 2 displays the findings of the logistic regression analysis. Patient sex, vitiligo type, family history of AITD, family history of autoimmune diseases other than AITD, thyroid nodules or tumors, emotion type, vitiligo involvement > 5% of body area, and abnormal immune serology (immunoglobulin plus complement) entered the nomogram as model predictors. The prediction equation used for the nomogram was as follows: LogitP = -3.053 + (0.5676 * female sex) + (0.96 * family history of autoimmune diseases except AITD) + (0.850 * family history of AITD) + (0.178 * combination of thyroid nodules or tumors) + (1.164 * negative emotion) + (0.720 * vitiligo involvement > 5% of body area) + (0.769 * abnormal immune serology) + (1.440 * segmental vitiligo). This predictive model is suitable for predicting the risk of AITD in patients with vitiligo based on current clinical characteristics and laboratory tests. The nomogram creates segments of various lengths to predict the disease based on how significant its predictors are in the overall variables (34). Figure 3 shows how to use it. The prediction process is statistically consistent, while the images are concise and aesthetically pleasing; we believe this will help with effective communication between doctors and patients.
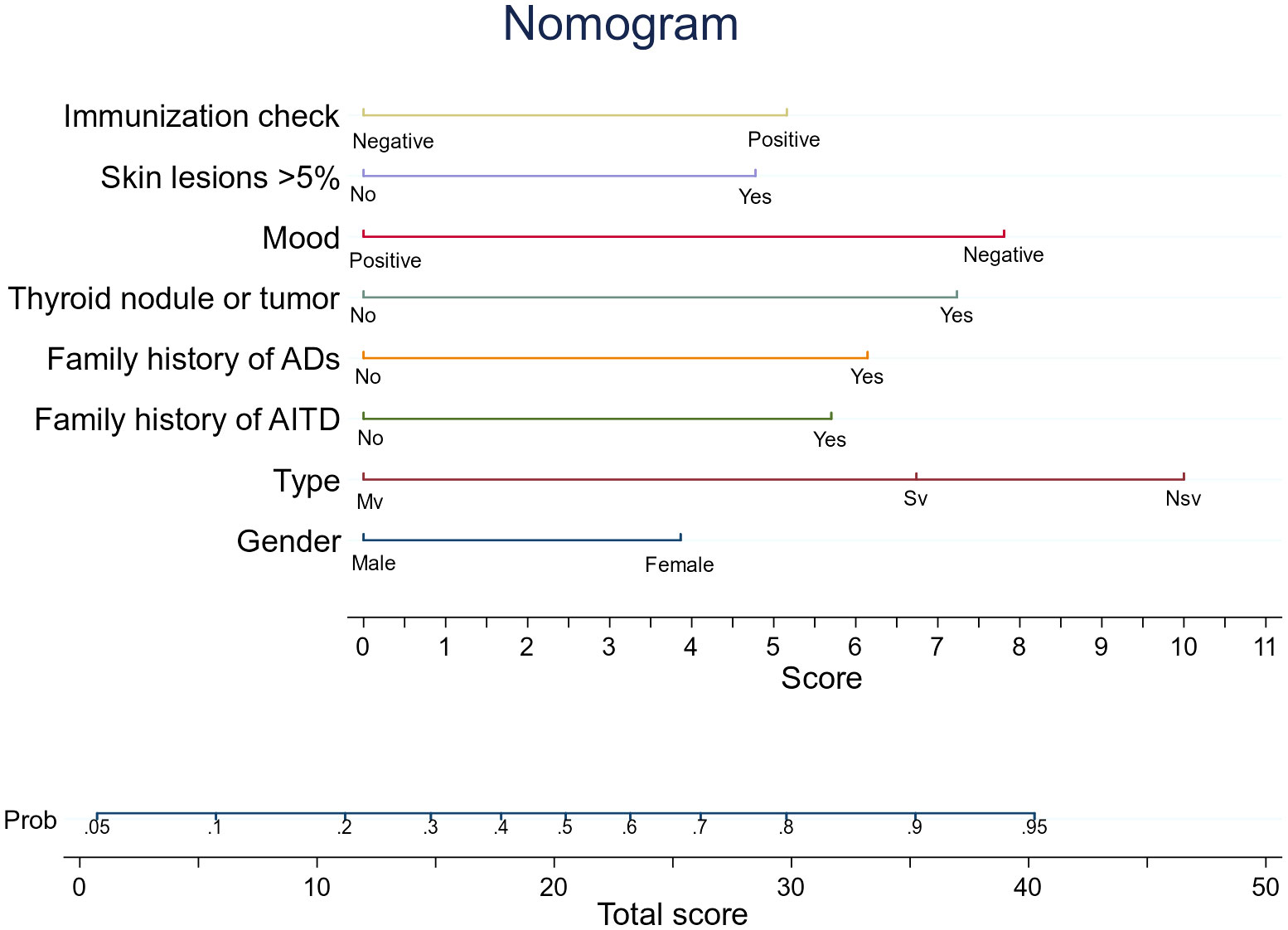
Figure 3 An AITD prediction line diagram may be developed in the queue based on a number of factors, including sex, vitiligo type, AITD family history, except for AITD autoimmune disease family history, thyroid nodules or tumors, and negative emotions. Involved skin area exceeds 5% of body surface area, and immunoglobulin plus tonic is positive. To calculate the probability that patients with vitiligo will develop AITD, draw a straight line on the point axis (for example, patients with non-segmental vitiligo will get 10 points). For each variable, repeat this process. Find the sum of the axis corresponding to each predicted variable. To determine the probability that patients with vitiligo will develop AITD, draw a line directly. For example, if a female patient with vitiligo has a family history of AITD, which is greater than 5%, and has a negative emotional type, then the prediction equation indicates that she has 53% chance of developing AITD. LASSO, Least Absolute Shrinkage and Selection Operator; AITD, autoimmune thyroid disease. The decision curve of the Hosmer–Lemeshow (HL) calibration curve predicts the nomogram, the area under the curve (AUC), and the risk of autoimmune thyroid disease (AITD).
3.4 Validation of AITD risk nomogram
We developed probability plots for the nomogram and evaluated nomogram calibration for its ability to identify patient outcomes or discriminate patients by comparing the predicted probability with the actual probability plots (35). The calibration curve of the nomogram used to predict AITD risk in patients with vitiligo indicated good agreement (Figure 4). The C-index measured the prediction accuracy (discriminant) of the nomogram (35). The accuracy of the predicted nomogram was 0.746 (95% CI: 0.701–0.792), with a bootstrapping validation score of 0.732. At test time, the accuracy was determined to be 0.869 and 0.777 by an external validation cohort; thus, the prediction model showed good discrimination ability. The accuracy of this predictive model was 0.7461 (Figure 5), sensitivity was 64.7%, and specificity was 73.1%. The higher the c-index, sensitivity, and specificity, the higher the accuracy of the prediction. In the external validation Group 1, the accuracy of AITD probability, sensitivity, and specificity were 0.869, 72.7%, and 87.1%, respectively. In the external validation Group 2, the accuracy of AITD probability, sensitivity, and specificity were 0.777, 92.3%, and 58.6%, respectively. These results indicated that the AITD predicting model had high predictive ability.
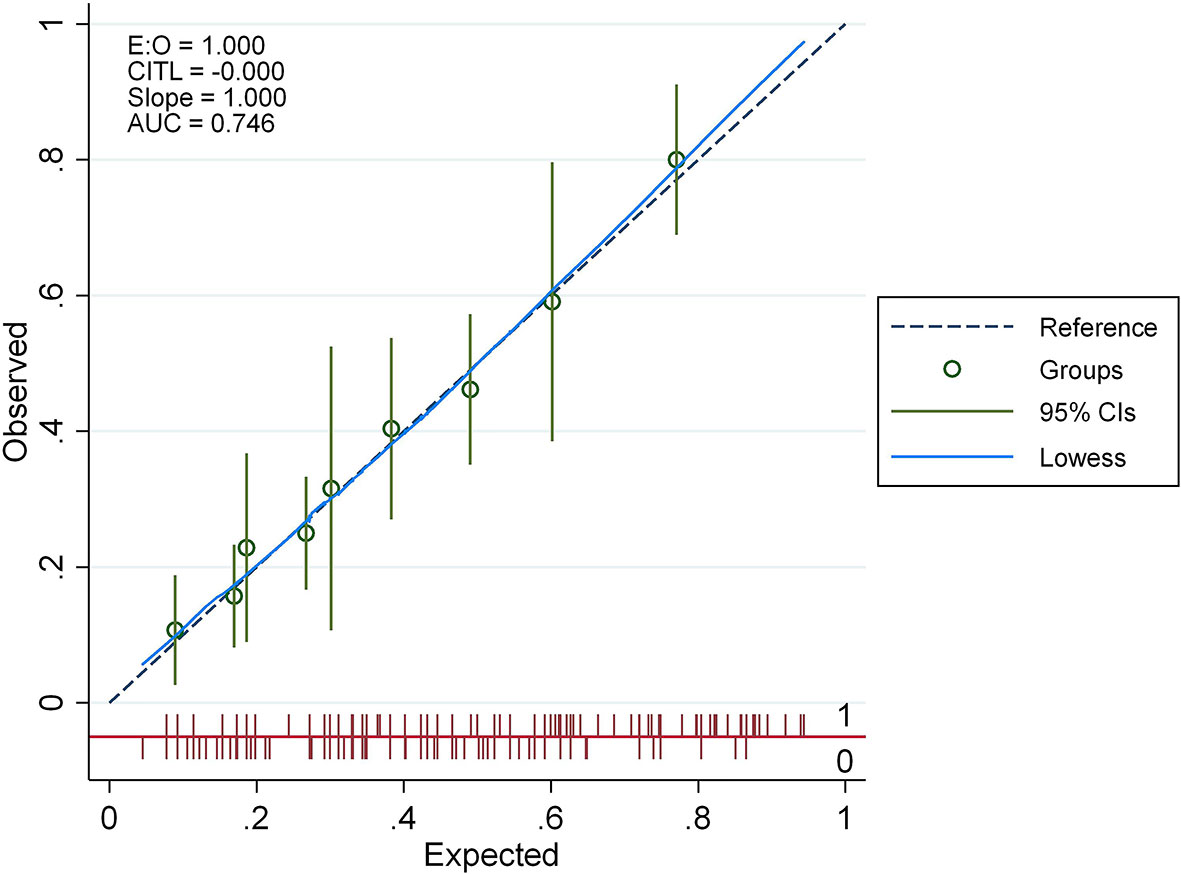
Figure 4 The calibration curve for the AITD prediction nomogram. The expected risk of developing AITD is shown on the x-axis. For the ideal model, diagonal dashed lines reflect accurate predictions. The performance is shown by the solid line, and the more closely it resembles the dashed line, the more accurate the forecasted risk.
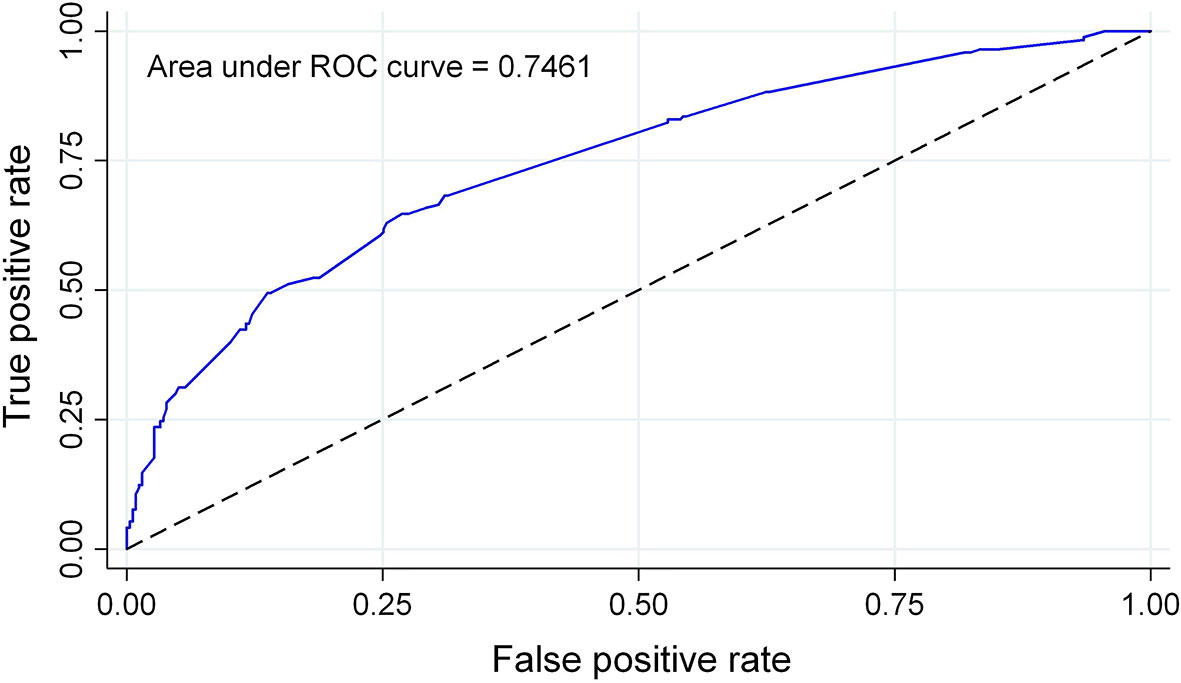
Figure 5 AUC for the AITD prediction nomogram. The probability of correctly predicting whether a patient has AITD in selected random cases is indicated by the AUC of a prediction model. The model has strong predictive power, and the overall sample AUC value is 0.7461.
3.5 Clinical application
We conducted clinical DCA on the nomogram in the training and validation sets to verify the net benefit to patients with vitiligo (36) (Figure 6). These results demonstrate that this predictive model is well suited for the prediction of AITD in patients with vitiligo, which can help guide treatment and reduce the risks of missing the AITD diagnosis in patients with vitiligo.
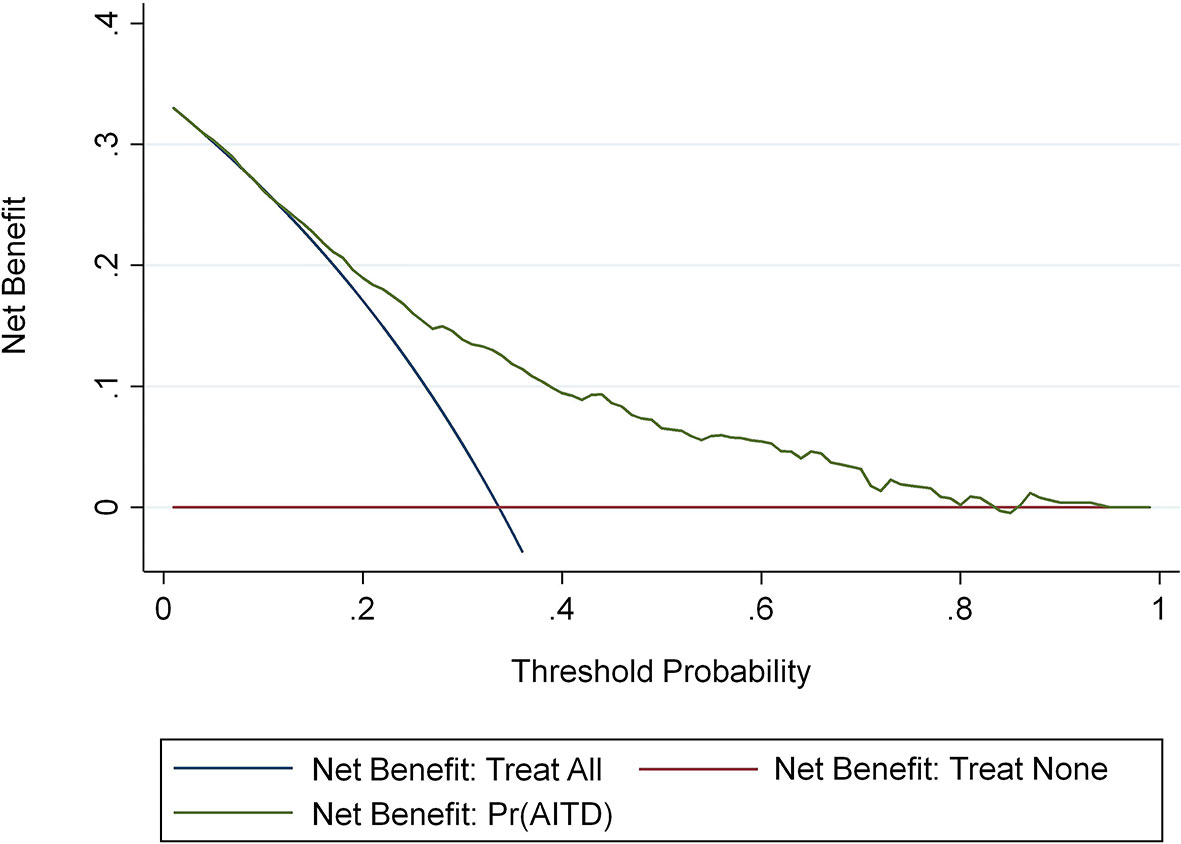
Figure 6 The AITD decision curve to assess the risk of meaning map. The y-axis represents net income. The blue line represents the risk nomogram of AITD. The thin solid line represents the hypothesis that all patients have AITD. The thick solid line represents the hypothesis that no patients have AITD.
3.6 External verification
We also validated two independent external cohorts with the Hosmer–Lemeshow test, receiver operator characteristic curve, and DCA. The results are shown in Supplementary Material.
4 Discussion
We have found a number of traits that make vitiligo patients more likely to develop AITD, most of which have been mentioned in previous studies. These include Sex, vitiligo type, family history of AITD, family history of other autoimmune disease, thyroid nodules or tumors, negative emotions. Our latest study finds that positive immune serology or skin involvement exceeding 5% of body surface area of the whole were more likely to develop AITD.
One of the most accepted current theories regarding the pathogenesis of vitiligo is an autoimmune origin (11). In addition, the prevalence rate of AITD in patients affected by vitiligo is 14.3% (13). Previous studies have reported that compared to male patients, female patients with vitiligo have a significantly higher probability of having AITD; this is consistent with our findings. Secretion-related (37) androgens (e.g., testosterone) have suppressive effects on immune function. The increased female prevalence may be owing to the immune-boosting effects of estrogenic sex steroids (38). The type of vitiligo is also a risk factor. According to previous studies, AITD is more common in patients with NSV because of its pathogenesis, which differs from SV (25). SV is considered nerve-related, while autoimmune factors are associated with NSV (39). In recent years, SV has also been reported as autoimmune-mediated because of melanocyte-specific T cell infiltration of the same melanin cells as that of NSV (40). At present, we have not tested this theory, and we have to further increase the amount of data to verify it. Epidemiological evidence for genetic susceptibility to AITD has also been confirmed by familial clustering of the disease (22). Approximately 33% of siblings of patients with Hashimoto’s thyroiditis or Graves’ disease also have AITD. This is consistent with the results of our study. Among family members of patients with vitiligo, patients with autoimmune diseases, such as rheumatoid arthritis, psoriasis, and pernicious anemia, were also more likely to have AITD. This may reflect the susceptibility to AITD in family members of patients with vitiligo having autoimmune disease (41). We must also consider that the occurrence of diseases within a family may not only be due to heredity, but also due to external factors such as diet and geography.
Negative emotions are a risk factor for AITD in patients with vitiligo. Although consensus is lacking regarding the pathogenesis of autoimmune diseases, previous studies have concluded that stress indirectly contributes to their course and onset. Improved epidemiological investigations have revealed that patients with Graves’ disease experienced significantly higher levels of life stress than control patients. The impact of stress on Hashimoto’s thyroiditis is still unknown (42) and is of great significance for understanding the development and prognosis of stress diseases. Through its influence on the nervous and endocrine systems, stress directly or indirectly affects the immune system. Through this immune modulation, individuals are more likely to develop autoimmunity, thereby increasing the risk of developing AITD (23).
This study also analyzed blood tests and found that patients with vitiligo combined with a positive immunoglobulin plus complement test are more likely to have AITD. Testing of immunoglobulins included serum IgG, IgM, and IgA, and of complement included C3 and C4. Studies have shown that the C3 and C4 values in patients with vitiligo were significantly lower than those in controls, and reported cases where IgA and IgG concentrations decreased (43, 44). The basic feature of immunoglobulin is that it binds to the corresponding antigen to achieve phagocytosis and dissolution. C3 and C4, as nonspecific proteins, have remarkable characteristics in humoral immunity (20). Patients with vitiligo involvement exceeding 5% of the total body surface area were more likely to develop AITD. We believe that this is related to the vitiligo severity; more severe disease increases the likelihood of an autoimmune thyroid attack. Some studies have suggested that AITD is associated with thyroid cancer; therefore, more attention should be paid to the investigation of a previous history of thyroid nodules or tumors (12).
Some studies said patients with vitiligo combined other autoimmune diseases have been reported to be more likely to develop AITD (12). This was not evident in our study data; this may be related to insufficient patient numbers. In an early model of our predictive nomogram, we considered smoking as a risk factor for AITD in patients with vitiligo (45). However, smoking was excluded when the best model was selected because of the following reasons: we had a higher number of female patients, cultural factor influences, and an inadequately sized smoking population. As a result, we were unable to evaluate this risk.
Our study has several advantages. To our knowledge, this is the first study to develop a nomogram using internal and external validation processes for predicting the risk of AITD development in patients with vitiligo. Previous nomograms created for vitiligo and AITD did not use external validation and thus, lacked credibility. However, our study used external validation. Furthermore, our study analyzed a comparatively large number of patients. This helped in obtaining a wide range of basic patient information. These data can also be used in the future for related studies about vitiligo. We examined the reasons for the occurrence of independent predictors, and added speculation and explanations for some unreported factors, which are yet to be verified and improved by other research teams. Finally, in China, with its large population, the number of patients with vitiligo is substantial with a variable patient economic level. The diagnosis of AITD belongs in the endocrinology department and AITD is a complication of vitiligo. Dermatologists need to have some knowledge of the AITD. There are also limitations to this study. This research is retrospective and is based on an insufficiently diverse population. If possible, patients from different regions and ethnicities should be studied, with external validation. Multicenter validation is also necessary.
5 Conclusion
In this study, we developed and validated a practical AITD risk prediction nomogram for patients with vitiligo, based on eight predictors that are relatively easy to obtain. The C-index, Hosmer–Lemeshow test, and DCA results indicated good performance. According to our findings, the onset of AITD in vitiligo patients is closely related with the patient’s sex, type of vitiligo, family history of AITD, family history of autoimmune diseases except AITD, negative emotions, thyroid nodules or tumors, skin patches exceeding 5% of the body surface area, and a positive immune test. Future statistical analysis with samples from random populations will further validate the prediction model, to enhance simple and effective assessment for the risk of AITD. Our study contributes to both reducing the health insurance burden of preventable costs and facilitating communication between physicians and patients.
Data availability statement
The original contributions presented in the study are included in the article/Supplementary Material. Further inquiries can be directed to the corresponding author.
Ethics statement
This study was approved by the Ethics Committee of Tianjin Academy of Traditional Chinese Medicine Affiliated Hospital, Tianjin, China. This is a retrospective study, approved by the Ethics Committee, without the need to sign an informed consent form. Written informed consent from the patients/participants OR patients/participants legal guardian/next of kin was not required to participate in this study in accordance with the national legislation and the institutional requirements.
Author contributions
ZM contributed to the research design, data analysis, and manuscript writing. MC, XL, and JL performed data collection and validation. KY conducted clinical diagnosis and sample collection. JZ and TG were clinical specialists and contributed to the manuscript revision. All authors contributed to the article and approved the submitted version.
Acknowledgments
We express our sincere gratitude to our physician colleagues, investigators, and statisticians for their contributions to this study.
Conflict of interest
The authors declare that the research was conducted in the absence of any commercial or financial relationships that could be construed as a potential conflict of interest.
Publisher’s note
All claims expressed in this article are solely those of the authors and do not necessarily represent those of their affiliated organizations, or those of the publisher, the editors and the reviewers. Any product that may be evaluated in this article, or claim that may be made by its manufacturer, is not guaranteed or endorsed by the publisher.
Supplementary material
The Supplementary Material for this article can be found online at: https://www.frontiersin.org/articles/10.3389/fendo.2023.1109925/full#supplementary-material
References
1. Bergqvist C, Ezzedine K. Vitiligo: A review. Dermatology (2020) 236(6):571–92. doi: 10.1159/000506103
2. Ezzedine K, Eleftheriadou V, Whitton M, van Geel N. Vitiligo. Lancet (2015) 386(9988):74–84. doi: 10.1016/s0140-6736(14)60763-7
3. Patel S, Rauf A, Khan H, Meher BR. Hassan SSU. A holistic review on the autoimmune disease vitiligo with emphasis on the causal factors. BioMed Pharmacother (2017) 92:501–8. doi: 10.1016/j.biopha.2017.05.095
4. Colucci R, Dragoni F, Moretti S. Oxidative stress and immune system in vitiligo and thyroid diseases. Oxid Med Cell Longev (2015) 2015:631927. doi: 10.1155/2015/631927
5. Manolache L, Benea V. Stress in patients with alopecia areata and vitiligo. J Eur Acad Dermatol Venereol (2007) 21(7):921–8. doi: 10.1111/j.1468-3083.2006.02106.x
6. Rodrigues M, Ezzedine K, Hamzavi I, Pandya AG, Harris JE, Vitiligo Working G. New discoveries in the pathogenesis and classification of vitiligo. J Am Acad Dermatol (2017) 77(1):1–13. doi: 10.1016/j.jaad.2016.10.048
7. Strassner JP, Harris JE. Understanding mechanisms of autoimmunity through translational research in vitiligo. Curr Opin Immunol (2016) 43:81–8. doi: 10.1016/j.coi.2016.09.008
8. Taïeb A, Picardo M. Clinical practice. Vitiligo. New Engl J Med (2009) 360(2):160–9. doi: 10.1056/NEJMcp0804388
9. Le Poole IC, Luiten RM. Autoimmune etiology of generalized vitiligo. Curr Dir Autoimmun (2008) 10:227–43. doi: 10.1159/000131485
10. Manga P, Elbuluk N, Orlow SJ. Recent advances in understanding vitiligo. F1000Res (2016) 5. doi: 10.12688/f1000research.8976.1
11. Cutolo M. Autoimmune polyendocrine syndromes. Autoimmun Rev (2014) 13(2):85–9. doi: 10.1016/j.autrev.2013.07.006
12. Antonelli A, Ferrari SM, Corrado A, Di Domenicantonio A, Fallahi P. Autoimmune thyroid disorders. Autoimmun Rev (2015) 14(2):174–80. doi: 10.1016/j.autrev.2014.10.016
13. Vrijman C, Kroon MW, Limpens J, Leeflang MMG, Luiten RM, van der Veen JPW, et al. The prevalence of thyroid disease in patients with vitiligo: A systematic review. Br J Dermatol (2012) 167(6):1224–35. doi: 10.1111/j.1365-2133.2012.11198.x
14. Fallahi P, Ferrari SM, Ruffilli I, Elia G, Biricotti M, Vita R, et al. The association of other autoimmune diseases in patients with autoimmune thyroiditis: Review of the literature and report of a Large series of patients. Autoimmun Rev (2016) 15(12):1125–8. doi: 10.1016/j.autrev.2016.09.009
16. Yang Y, Huang G, Yan X, Qing Z. Clinical analysis of thyroglobulin antibody and thyroid peroxidase antibody and their association with vitiligo. Indian J Dermatol (2014) 59(4):357–60. doi: 10.4103/0019-5154.135485
17. Lazzeri L, Colucci R, Cammi A, Dragoni F, Moretti S. Adult onset vitiligo: Multivariate analysis suggests the need for a thyroid screening. BioMed Res Int (2016) 2016:8065765. doi: 10.1155/2016/8065765
18. Gill L, Zarbo A, Isedeh P, Jacobsen G, Lim HW, Hamzavi I. Comorbid autoimmune diseases in patients with vitiligo: A cross-sectional study. J Am Acad Dermatol (2016) 74(2):295–302. doi: 10.1016/j.jaad.2015.08.063
19. Gong Q, Li X, Gong Q, Zhu W, Song G, Lu Y. Hashimoto's thyroiditis could be secondary to vitiligo: The possibility of antigen crossover and oxidative stress between the two diseases. Arch Dermatol Res (2016) 308(4):277–81. doi: 10.1007/s00403-016-1641-z
20. Goldman LM, Schafer AI. Goldman-Cecil medicine: Goldman-Cecil medicine. (2016) P216–246:P1500–1514.
21. Iacovelli P, Sinagra JL, Vidolin AP, Marenda S, Capitanio B, Leone G, et al. Relevance of thyroiditis and of other autoimmune diseases in children with vitiligo. Dermatology (2005) 210(1):26–30. doi: 10.1159/000081479
22. Tomer Y, Davies TF. Searching for the autoimmune thyroid disease susceptibility genes: From gene mapping to gene function. Endocr Rev (2003) 24(5):694–717. doi: 10.1210/er.2002-0030
23. Mizokami T, Wu Li A, El-Kaissi S, Wall JR. Stress and thyroid autoimmunity. Thyroid (2004) 14(12):1047–55. doi: 10.1089/thy.2004.14.1047
24. Behl PN, Bhatia RK. 400 cases of vitiligo. a clinico-therapeutic analysis. Indian J Dermatol (1972) 17(2):51–6.
25. Hann SK, Lee HJ. Segmental vitiligo: Clinical findings in 208 patients. J Am Acad Dermatol (1996) 35(5 Pt 1):671–4. doi: 10.1016/s0190-9622(96)90718-5
26. Ezzedine K, Lim HW, Suzuki T, Katayama I, Hamzavi I, Lan CC, et al. Revised Classification/Nomenclature of vitiligo and related issues: The vitiligo global issues consensus conference. Pigment Cell Melanoma Res (2012) 25(3):E1–13. doi: 10.1111/j.1755-148X.2012.00997.x
27. Diaz-Garcia A, Gonzalez-Robles A, Mor S, Mira A, Quero S, Garcia-Palacios A, et al. Positive and negative affect schedule (Panas): Psychometric properties of the online Spanish version in a clinical sample with emotional disorders. BMC Psychiatry (2020) 20(1):56. doi: 10.1186/s12888-020-2472-1
28. Sauerbrei W, Royston P, Binder H. Selection of important variables and determination of functional form for continuous predictors in multivariable model building. Stat Med (2007) 26(30):5512–28. doi: 10.1002/sim.3148
29. Lee S, Seo MH, Shin Y. The lasso for high dimensional regression with a possible change point. J R Stat Soc Ser B Stat Method (2016) 78(1):193–210. doi: 10.1111/rssb.12108
30. Pencina MJ, D'Agostino RB. Overall c as a measure of discrimination in survival analysis: Model specific population value and confidence interval estimation. Statist Med (2004) 23(13):2109–23. doi: 10.1002/sim.1802
31. Huang Y-Q, Liang C-H, He L, Tian J, Liang C-S, Chen X, et al. Development and validation of a radiomics nomogram for preoperative prediction of lymph node metastasis in colorectal cancer. J Clin Oncol (2016) 34(18):2157–64. doi: 10.1200/jco.2015.65.9128
32. Kroon MW, Vrijman C, Chandeck C, Wind BS, Wolkerstorfer A, Luiten RM, et al. High prevalence of autoimmune thyroiditis in children and adolescents with vitiligo. Horm Res Paediatr (2013) 79:137–44. doi: 10.1159/000348388
33. Uncu S, Yaylı S, Bahadır S, Okten A, Alpay K. Relevance of autoimmune thyroiditis in children and adolescents with vitiligo. Int J Dermatol (2011) 50(2):175–9. doi: 10.1111/j.1365-4632.2010.04665.x
34. Zhang Z, Shao Y, Lotsch B, Hu Y-S, Li H, Janek J, et al. New horizons for inorganic solid state ion conductors. Energy Environ Sci (2018) 11(8):1945–76. doi: 10.1039/C8EE01053F
35. Iasonos A, Schrag D, Raj GV, Panageas KS. How to build and interpret a nomogram for cancer prognosis. J Clin Oncol (2008) 26(8):1364–70. doi: 10.1200/jco.2007.12.9791
36. Hijazi Z, Oldgren J, Lindbäck J, Alexander JH, Connolly SJ, Eikelboom JW, et al. The novel biomarker-based abc (Age, biomarkers, clinical history)-bleeding risk score for patients with atrial fibrillation: A derivation and validation study. Lancet (London England) (2016) 387(10035):2302–11. doi: 10.1016/s0140-6736(16)00741-8
37. Iacovelli P, Sinagra JLM, Vidolin AP, Marenda S, Capitanio B, Leone G, et al. Relevance of thyroiditis and of other autoimmune diseases in children with vitiligo. Dermatology (2004) 210:26–30.
38. Grossman CJ, Roselle GA, Mendenhall CL. Sex steroid regulation of autoimmunity. J Steroid Biochem Mol Biol (1991) 40(4-6):649–59. doi: 10.1016/0960-0760(91)90287-f
39. Namazi MR. Neurogenic dysregulation, oxidative stress, autoimmunity, and melanocytorrhagy in vitiligo: Can they be interconnected? Pigment Cell Res (2007) 20(5):360–3. doi: 10.1111/j.1600-0749.2007.00408.x
40. van Geel NA, Mollet IG, De Schepper S, Tjin EP, Vermaelen K, Clark RA, et al. First histopathological and immunophenotypic analysis of early dynamic events in a patient with segmental vitiligo associated with halo nevi. Pigment Cell Melanoma Res (2010) 23(3):375–84. doi: 10.1111/j.1755-148X.2010.00703.x
41. Laberge G, Mailloux CM, Gowan K, Holland P, Bennett DC, Fain PR, et al. Early disease onset and increased risk of other autoimmune diseases in familial generalized vitiligo. Pigment Cell Res (2005) 18(4):300–5. doi: 10.1111/j.1600-0749.2005.00242.x
42. Winsa B, Adami HO, Bergström R, Gamstedt A, Dahlberg PA, Adamson U, et al. Stressful life events and graves' disease. Lancet (London England) (1991) 338(8781):1475–9. doi: 10.1016/0140-6736(91)92298-g
43. Farrokhi S, Hojjat-Farsangi M, Noohpisheh MK, Tahmasbi R, Rezaei N. Assessment of the immune system in 55 Iranian patients with vitiligo. J Eur Acad Dermatol Venereol (2005) 19(6):706–11. doi: 10.1111/j.1468-3083.2005.01295.x
44. Ali R, Ahsan MS, Azad MAK, Ullah MA, Bari W, Islam SN, et al. Immunoglobulin levels of vitiligo patients. Pak J Pharm Sci (2010) 23(1):97–102.
Keywords: vitiligo, autoimmune thyroid disease, nomogram, predictive model, lasso
Citation: Ma Z, Cai M, Yang K, Liu J, Guo T, Liu X and Zhang J (2023) Predicting the risk of autoimmune thyroid disease in patients with vitiligo: Development and assessment of a new predictive nomogram. Front. Endocrinol. 14:1109925. doi: 10.3389/fendo.2023.1109925
Received: 28 November 2022; Accepted: 10 January 2023;
Published: 31 January 2023.
Edited by:
Silvia Martina Ferrari, University of Pisa, ItalyReviewed by:
Anette S. B. Wolff, Haukeland University Hospital, NorwayCristiane Jeyce Gomes-Lima, MedStar Health Research Institute (MHRI), United States
Copyright © 2023 Ma, Cai, Yang, Liu, Guo, Liu and Zhang. This is an open-access article distributed under the terms of the Creative Commons Attribution License (CC BY). The use, distribution or reproduction in other forums is permitted, provided the original author(s) and the copyright owner(s) are credited and that the original publication in this journal is cited, in accordance with accepted academic practice. No use, distribution or reproduction is permitted which does not comply with these terms.
*Correspondence: Junling Zhang, emhhbmdqdW5saW5ndGpAMTI2LmNvbQ==