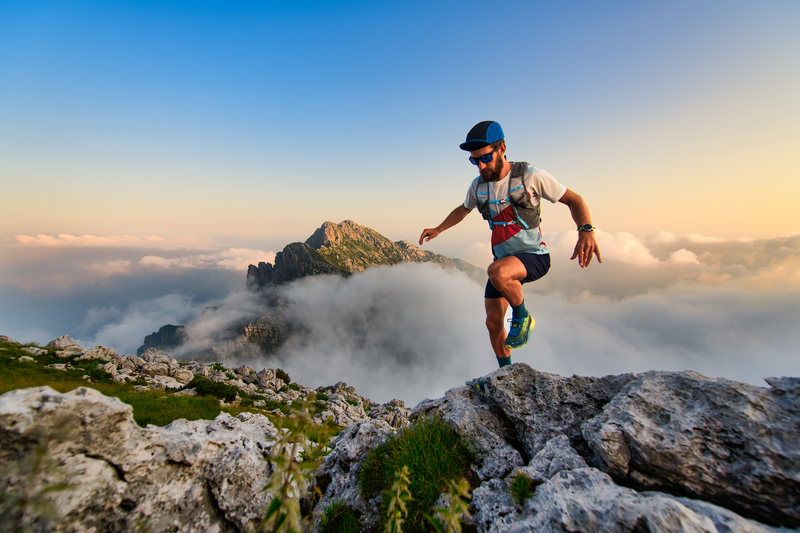
94% of researchers rate our articles as excellent or good
Learn more about the work of our research integrity team to safeguard the quality of each article we publish.
Find out more
SYSTEMATIC REVIEW article
Front. Endocrinol. , 18 September 2023
Sec. Reproduction
Volume 14 - 2023 | https://doi.org/10.3389/fendo.2023.1106625
Introduction: Polycystic Ovarian Syndrome (PCOS) is the most common endocrinopathy in women of reproductive age and remains widely underdiagnosed leading to significant morbidity. Artificial intelligence (AI) and machine learning (ML) hold promise in improving diagnostics. Thus, we performed a systematic review of literature to identify the utility of AI/ML in the diagnosis or classification of PCOS.
Methods: We applied a search strategy using the following databases MEDLINE, Embase, the Cochrane Central Register of Controlled Trials, the Web of Science, and the IEEE Xplore Digital Library using relevant keywords. Eligible studies were identified, and results were extracted for their synthesis from inception until January 1, 2022.
Results: 135 studies were screened and ultimately, 31 studies were included in this study. Data sources used by the AI/ML interventions included clinical data, electronic health records, and genetic and proteomic data. Ten studies (32%) employed standardized criteria (NIH, Rotterdam, or Revised International PCOS classification), while 17 (55%) used clinical information with/without imaging. The most common AI techniques employed were support vector machine (42% studies), K-nearest neighbor (26%), and regression models (23%) were the commonest AI/ML. Receiver operating curves (ROC) were employed to compare AI/ML with clinical diagnosis. Area under the ROC ranged from 73% to 100% (n=7 studies), diagnostic accuracy from 89% to 100% (n=4 studies), sensitivity from 41% to 100% (n=10 studies), specificity from 75% to 100% (n=10 studies), positive predictive value (PPV) from 68% to 95% (n=4 studies), and negative predictive value (NPV) from 94% to 99% (n=2 studies).
Conclusion: Artificial intelligence and machine learning provide a high diagnostic and classification performance in detecting PCOS, thereby providing an avenue for early diagnosis of this disorder. However, AI-based studies should use standardized PCOS diagnostic criteria to enhance the clinical applicability of AI/ML in PCOS and improve adherence to methodological and reporting guidelines for maximum diagnostic utility.
Systematic review registration: https://www.crd.york.ac.uk/prospero/, identifier CRD42022295287.
Polycystic Ovary Syndrome (PCOS) is the most common endocrinopathy in reproductive aged women, with an estimated prevalence ranging from 4% to 20% and affecting more than 66 million worldwide in 2019 (1–5). PCOS is associated with increased incidence of cardiovascular disease, infertility, and of endometrial cancer (6–9). Its public health burden is immense, with nearly eight billion US dollars spent in 2020 to manage PCOS-related symptoms among women in the United States alone (10).
The diagnosis of PCOS is based on clinical criteria, with the Rotterdam criteria/International PCOS criteria (11, 12) being the most widely accepted. PCOS is characterized by the presence of a combination of hyperandrogenism, ovulatory dysregulation, and polycystic ovarian morphology (PCOM) (13–15). This already heterogenous clinical phenotype is complicated further by the elaborate interplay of genetic and environmental factors, such as diet related obesity or lifestyle factors, which affect clinical presentation (16). The criteria-based diagnosis of PCOS is complicated by variations in the clinical assessment of hyperandrogenism and determination of menstrual irregularity. Furthermore, the variation in normative standards for PCOM compounds these challenges (17). Estimates suggest that diagnosis is delayed by more than two years in one third of women with PCOS; yet this is likely an underestimation (18).
Artificial intelligence (AI) refers to simulation of human intelligence by computer based systems (19). On the other hand, machine learning (ML) is a subdivision of AI focused on learning from previous events and applying this knowledge to future decision making (20). ML techniques can be sub-classified as either supervised or unsupervised (21). The revolutionary advances in AI and ML over the last decade promise to rapidly advance our ability to diagnose and manage PCOS. This is in part due to the ability of AI to process massive amounts of disparate data, making it an ideal aid in the diagnosis of heterogeneous disorders like PCOS.
Several studies have investigated the ability of ML models to synthesize such disparate data as family genetic history, biomarkers, and demographic information into a unified algorithm for the diagnosis of PCOS, and make diagnostic predictions (22). Some pitfalls of these studies are their small size (22), lack of relevant comparators (23), use of varied diagnostic criteria (24, 25), and heterogeneity in reporting structures. Thus, the real gaps in knowledge and the full scope of AI/ML in the diagnosis of PCOS remain unclear. To better understand and summarize the body of evidence related to the application of AI/ML in PCOS, we conducted a systematic review of all relevant studies published up to January 1, 2022.
This manuscript employed the Preferred Reporting Items for Systematic Reviews and Meta-analysis (PRISMA) guidelines, and was submitted to PROSPERO (record number PROSPERO 2022 CRD42022295287) (26). We included English language, peer-reviewed original studies that evaluated the use of AI/ML in diagnosing, classifying, stratifying, or predicting PCOS. We subdivided studies into those that ‘diagnosed’ and those that ‘classified’ PCOS subjects. Studies were considered to diagnose PCOS if they employed standard diagnostic criteria such as NIH, Rotterdam, androgen excess-PCOS and international PCOS criteria. In contrast, those studies that partially used standard criteria or only used some measures to determine PCOS were considered to ‘classify’ subjects as having PCOS.
We applied a search strategy developed in collaboration with an experienced librarian to find potentially eligible studies. Databases searched were MEDLINE, Embase, the Cochrane Central Register of Controlled Trials, the Web of Science, and the IEEE Xplore Digital Library. The search included all articles from the time of inception of the dataset to May 2019. Conference abstracts were included if they fulfilled the eligibility criteria provided the manuscript wasn’t published. The full search strategy is included in Supplementary Material 3.
We uploaded all references to Covidence and performed two rounds of screening, title-and-abstract screening, and full-text screening. Each article was assessed for eligibility by two independent reviewers in both rounds of screening using standardized instructions. Pilot phases were conducted before each screening round to ensure a baseline understanding of the eligibility criteria and resolve misunderstandings between reviewers. Inter-rater reliability assessed through Cohen’s Kappa statistic was high (κ>0.80) in both rounds of screening.
In the first screening round, disagreements were included in the second round. In the second round, disagreements were resolved by consensus between reviewers or by arbitration of a third trained reviewer.
Five reviewers working independently and in duplicate extracted data from studies using a standardized extraction form. Two pilot phases were performed to ensure proficiency in the data extraction procedure. Further disagreements were discussed and resolved by consensus, and the database was cleaned by two reviewers. The extracted variables were: 1) study characteristics (authors’ information, publication year, country and setting, study design, aim and type of machine learning used, and type of data entered into the models); 2) artificial intelligence/machine learning characteristics (type of dataset used, dataset independence, type of results reported [sensitivity, specificity, area under the curve, diagnostic accuracy, precision]); 3) PCOS characteristics (definition of the disease, sample size); and 4) risk of bias.
Each study was assessed for risk of bias by two independent reviewers and disagreements were resolved by two separate reviewers. We used a modified version of the Quality Assessment of Diagnostic Accuracy Studies (QUADAS-2) tool, which includes four domains: patient selection, index test, reference standard, and flow and timing. As this tool is not designed for systematic reviews of diagnostic accuracy studies using AI/ML interventions, we summarized and complemented it with input from the authors to ensure that critical questions for AI/ML interventions were included in addition to the relevant pre-existing QUADAS-2 questions. Details of the modified QUADAS-2 tool are provided in Supplementary Material 2. The tailored QUADAS-2 tool was piloted on five studies by all reviewers and differences resolved with consensus. If a study had at least two domains at unclear risk of bias without any domain deemed at high risk of bias, it was judged to be at unclear risk of bias. Finally, studies with domains classified as low risk of bias without any domain of unclear or high risk of bias were considered low risk of bias.
A total of 31 studies met our inclusion criteria (Figure 1). All studies were observational and used retrospective data samples to assess the performance of the AI/ML process on the diagnosis or classification of patients. Seven of 31 studies (23%) were multi-center studies and many were conducted either in India (29%) or in China (16%). Eleven studies (36%) included subjects who did not have PCOS as controls. Sample size ranged from 9 to 2,000 patients with PCOS and the median age of participants included in studies was 29 years. The rest of the general characteristics can be found in Table 1.
Figure 1 Selection process of the studies. Article selection flow chart for studies related to AI/ML and PCOS according to Preferred Reporting Items for Systematic Reviews and Meta-Analyses (PRISMA) guidelines.
Nearly half of all studies (48%) used ultrasound images to implement the AI/ML intervention. Twelve studies (39%) used clinical data such as anthropometric features (10%), signs and symptoms (16%), biomarkers (19%), genetics (13%) and metabolomics or proteomics (10%).
Ten (32%) studies used a validated diagnostic criterion to select the population, such as exclusively the Rotterdam criteria (23%), the NIH Criteria (6%), with one study using a combination of NIH and Rotterdam criteria (3%) (11, 12, 57) (Table 1). Another study (3%) used the Adams criteria, an imaging-based criteria which has not been clinically validated (58). The remaining 20 studies (65%) used clinical information to make the diagnosis, with or without complementary imaging (55%), with one study using ICD codes. Two (7%) studies reported that they used age-matched participants without the diagnosis of PCOS as controls, while other studies reported scarce information about controls; including definitions such as “normal ovaries through imaging”, or “normal ovulation cycles”. Five (16%) studies provided no definition for controls.
Among the ten (32%) studies that used standardized diagnostic criteria, the area under the receiver operator curve ranged from 80% to 100% (n=3 studies), diagnostic accuracy from 89% to 100% (n=4 studies), sensitivity from 87% to 100% (n=3 studies), specificity from 90% to 100% (n=3 studies), and positive predictive value from 68% to 81% (n=2 studies), and negative predictive value (NPV) from 94% to 99% (n=2 studies). Performance measures for all the included studies are shown in Table 2. The studies that used standardized PCOS criteria are summarized in Figure 2 by outcome type.
Figure 2 Unpooled results of studies with well-defined PCOS patient population. Outcomes and interventions are denoted in shorthand as Area Under the Curve (AUC), Partial Least-Squares Discriminant Analysis (PLS-DA), Support Vector Machine (SVM), and K-Nearest Neighbor (K-NN). A parameter threshold of 80% (0.8) indicated by the dotted line was considered a benchmark to evaluate studies assuming a 20% performance error.
The majority (71%) of studies we investigated used supervised methods. The most common were support vector machine (SVM) (42%), K-nearest neighbor (26%), regression models (23%), and Random Forest (23%). Unsupervised methods were used in nine (29%) studies and included neural networks (13%), Otsu’s thresholding and Watershed + object growing algorithm (6%), clustering analysis (6%), and self-organizing maps (3%) (Table 2). Various AI/ML models are described in Table 3.
Only six (19%) studies performed all major steps of training, testing, and validation in their AI/ML interventions. About three-quarters of studies (74%) performed at least one of these steps. Specifically, ten (32%) studies performed only training and testing, four (13%) only training and validation, and three (10%) completed only one of them. Among those studies that used at least two steps, all used an independent data set for each step by using a proportion of their sample for each step or cross-validation models (where data is trained and tested on different observations).
Nineteen (61%) studies compared the effectiveness of two or more AI/ML interventions on the same sample, while only three (10%) compared AI/ML interventions against a non-machine learning classifier (board-certified physician or ICD-9 codes). Of these three, two studies described the criteria used by the clinician or the codes used to make the diagnosis.
Overall, the risk of bias was judged to be high across all studies (Supplementary Material 1). Six (19%) studies described using a consecutive or random sample of the enrolled patients. Moreover, five (16%) studies used validated criteria to select their population, which affected risk of bias due to misclassification bias but also applicability bias due to an unclearly defined patient population in the studies. About half of all (52%) studies used an independent dataset to validate the AI/ML intervention. Finally, nine (29%) studies had hospital affiliations or a physician as a co-author of the study.
We performed a systematic review of AI/ML interventions in PCOS. All included studies were observational and retrospective. A small number used standard inclusion criteria such as the NIH, Rotterdam, or International PCOS criteria for diagnosis. Most studies achieved a high ability to diagnose PCOS or ‘classify’ patients as having PCOS using AI informed by clinical, radiological, electronic health records or biochemical data. Among the ten studies that used standardized criteria, the area under the receiver operator curve ranged from 80% to 100%, diagnostic accuracy from 89% to 100%, sensitivity from 87% to 100%, specificity from 90% to 100%, and positive predictive value from 68% to 81%. The most common AI/ML methods were SVM in 13 (42%) studies, K-nearest neighbor in eight (26%) studies, and regression models in seven (23%) studies. Importantly, a large number of the studies analyzed in the current review were able to achieve a high degree of diagnostic accuracy relative to standardized criteria. For instance, Deshpande et al. (2014) attained a 95% diagnostic accuracy against the Rotterdam criteria using an SVM algorithm using ultrasound imaging, clinical, and biochemical data (44). Similarly, Bharti et al. (2020) employed multiple ML algorithms to a dataset of 364 women with and without PCOS using clinical and imaging data and reported a > 90% diagnostic accuracy for the best SVM model (28).
AI/ML-based screening techniques for diabetic retinopathy and colorectal cancer have previously been found to be highly cost-effective (59, 60). In the case of colorectal cancer, cost savings of 400 million USD have been estimated when comparing next generation sequencing approaches to AI-based screening techniques (61). The potential use of AI/ML in the diagnosis and management of endocrine disorders has sparked intense research activity. A recent review reported that among the 611 ML-based endocrinology studies published between 2015 and 2020, 52% focused on diabetes, 14% on retinopathy, 14% on thyroid dysfunction, 8% on endocrine-related carcinoma, 7% on osteoporosis, and 5% on other disease states (62). Despite a growth in such studies, FDA-approved applications of AI for diagnostic or therapeutic purposes have lagged and approved devices employing AI/ML are concentrated in the management of diabetes and related conditions (63, 64).
In comparison, polycystic ovarian syndrome represents an ideal setting for future AI-based tools, given its high prevalence, significant healthcare burden, delayed detection, and complex diagnostic criteria spanning clinical, biochemical, and radiological domains. The diagnostic delay of greater than two years in a third of women reporting PCOS symptoms is a potent target for AI/ML-based approaches (18). Furthermore, geographical heterogeneity in clinical features of PCOS suggests an additional role of environmental influences, which may be overcome through adoption of AI/ML (65). Together, high costs and diagnostic delays in PCOS present a major unmet need which could be filled by the adoption of AI technology, as effectively demonstrated in other diseases. AI holds especially high potential for the diagnosis of PCOS because of its heterogeneous nature, with clinical, biochemical and radiological features each being incorporated into its diagnostic criteria (12). The use of AI on electronic health record (EHR) systems holds the potential to integrate these features while reducing diagnostic delays in PCOS.
The current body of research on AI in PCOS has revealed high rates of sensitivity and accuracy of PCOS detection. This implies that a well-designed AI/ML based program has the potential to significantly enhance our capability to diagnose PCOS early, with associated cost savings and a reduced burden of PCOS on patients and on the health system. However, several gaps remain in the domain of AI/ML based detection of PCOS. First, we noted that only a third of studies (32%) used standardized criteria such as the Rotterdam, NIH and International PCOS criteria as reference standards when evaluating AI in PCOS. This presents a high possibility misclassification of disease and biased detection estimates. Second, there was considerable heterogeneity in assessed AI-based studies, with some relying exclusively on a single parameter of PCOS diagnosis such as radiological, biochemical, or clinical features, despite Rotterdam criteria recommending diagnosis based on more than one of these elements. Third, a large number of assessed studies did not exhaustively report methodology/algorithms for AI based diagnosis, presenting concerns about the reproducibility of their findings. Most studies also relied on observational/retrospective data without use of prospective studies or validation datasets, limiting their applicability (66). A fourth major gap was the inadequate utilization of electronic health records, one of the most promising avenues for AI integration due to their potential for synthesizing clinical, biochemical, radiological, and genetic information and reducing lead time to the diagnosis in PCOS. This warrants further investigation in future studies. Finally, we noted that a vast number of AI/ML based studies were conducted in non-healthcare settings (71%) with non-healthcare investigators (97%). This raises the possibility of reduced applicability and relevance of studies in the clinical management of PCOS since such studies, while being technically robust, may not account for clinically important variables and outcomes. It is therefore important for physicians to become more aware of the advantages of AI/ML based methodologies and for physicians and computational scientists interested in AI/ML to work together to optimize the power of these new tools. Moreover, future AI/ML studies with applications for PCOS or other conditions, should make greater efforts to increase the methodological quality to increase the validity of the results. For this, we recommend the following five measures to improve the applicability of AI/ML for diagnosing PCOS and improving its care.
1. Increase collaboration between clinicians, researchers, and computational biologists.
2. Set up combined registries of data that include defined clinical, radiological (including images), and laboratory data (with reference values) of PCOS patients.
3. Use standardized criteria to train machine learning models as the standard reference and perform robust training and validation studies in PCOS patients.
3. Since some of the data used to develop the model may have some variation by time, it is important that future studies also test for performance (accuracy measures) consistency across time.
4. Enhance integration of population-based studies [e.g. All of Us, NHANES (67, 68)] with electronic health datasets to identify risk factors and risk enhancers for PCOS.
5. Include commonly used biochemical tests such as AMH, gonadal hormones, markers of insulin resistance and others in AI/ML to identify reliable biomarkers that can aid the diagnosis of PCOS.
To our knowledge, this is the first systematic review of AI/ML in the diagnosis of PCOS, spanning all published studies to date. We followed the methodological standards for systematic reviews proscribed by PRISMA guidelines. Despite the absence of a methodological assessment tool for evaluation of AI/ML based studies at the time of execution of this review, we performed a thorough evaluation of the quality by adapting the QUADAS-2 tool and adding relevant questions for the AI/ML interventions evaluated. Although not a weakness of our methods, confidence in our results is limited by the relatively small number of studies conducted on this subject, the heterogeneity of available data, and the risk of bias in primary studies. Broadly, poor dataset sourcing using non-standardized criteria, inconsistent use of best-practice machine learning methods, and limited clinical affiliations among authorship all undermined confidence in our selected studies.
In conclusion, our findings suggest that there is a high potential of AI/ML based programs in the diagnosis and care of PCOS, but that future studies should focus on enhancing methodological robustness and incorporating variables and outcomes of clinical importance.
The original contributions presented in the study are included in the article/Supplementary Material. Further inquiries can be directed to the corresponding author.
FB and SS designed the study. FB and EB participated in all phases of the conduction of the study. FB, EB, SS, AR, JO, HP, and EL participated in screening, data extraction, and manuscript writing. JH, RR-G and SS reviewed the final version of the manuscript. All authors contributed to the article and approved the submitted version.
Intramural Research Program (ZIDES102465 and ZID ES103323) of the National Institute of Environmental Health Sciences, National Institutes of Health, United States.
The authors declare that the research was conducted in the absence of any commercial or financial relationships that could be construed as a potential conflict of interest.
All claims expressed in this article are solely those of the authors and do not necessarily represent those of their affiliated organizations, or those of the publisher, the editors and the reviewers. Any product that may be evaluated in this article, or claim that may be made by its manufacturer, is not guaranteed or endorsed by the publisher.
The Supplementary Material for this article can be found online at: https://www.frontiersin.org/articles/10.3389/fendo.2023.1106625/full#supplementary-material
1. Carmina E, Lobo RA. Polycystic ovary syndrome (PCOS): arguably the most common endocrinopathy is associated with significant morbidity in women. J Clin Endocrinol Metab (1999) 84(6):1897–9. doi: 10.1210/jcem.84.6.5803
2. Knochenhauer ES, Key TJ, Kahsar-Miller M, Waggoner W, Boots LR, Azziz R. Prevalence of the polycystic ovary syndrome in unselected black and white women of the southeastern United States: a prospective study. J Clin Endocrinol Metab (1998) 83(9):3078–82. doi: 10.1210/jc.83.9.3078
3. Yildiz BO, Bozdag G, Yapici Z, Esinler I, Yarali H. Prevalence, phenotype and cardiometabolic risk of polycystic ovary syndrome under different diagnostic criteria. Hum Reprod (2012) 27(10):3067–73. doi: 10.1093/humrep/des232
4. Azziz R, Woods KS, Reyna R, Key TJ, Knochenhauer ES, Yildiz BO. The prevalence and features of the polycystic ovary syndrome in an unselected population. J Clin Endocrinol Metab (2004) 89(6):2745–9. doi: 10.1210/jc.2003-032046
5. IHME. Polycystic ovarian syndrome. GBD Results, 2019 (2022). Available at: https://www.healthdata.org/results/gbd_summaries/2019/polycystic-ovarian-syndrome-level-4-cause.
6. Dumesic DA, Lobo RA. Cancer risk and PCOS. Steroids (2013) 78(8):782–5. doi: 10.1016/j.steroids.2013.04.004
7. Dahlgren E, Janson PO, Johansson S, Lapidus L, Odén A. Polycystic ovary syndrome and risk for myocardial infarction. Evaluated from a risk factor model based on a prospective population study of women. Acta Obstet Gynecol Scand (1992) 71(8):599–604. doi: 10.3109/00016349209006227
8. Hart R, Norman R. Polycystic ovarian syndrome–prognosis and outcomes. Best Pract Res Clin Obstet Gynaecol (2006) 20(5):751–78. doi: 10.1016/j.bpobgyn.2006.04.006
9. Balen AH, Conway GS, Kaltsas G, Techatrasak K, Manning PJ, West C, et al. Polycystic ovary syndrome: the spectrum of the disorder in 1741 patients. Hum Reprod (1995) 10(8):2107–11. doi: 10.1093/oxfordjournals.humrep.a136243
10. Riestenberg C, Jagasia A, Markovic D, Buyalos RP, Azziz R. Health care-related economic burden of polycystic ovary syndrome in the United States: pregnancy-related and long-term health consequences. J Clin Endocrinol Metab (2022) 107(2):575–85. doi: 10.1210/clinem/dgab613
11. Teede HJ, Misso ML, Costello MF, Dokras A, Laven J, Moran L, et al. Recommendations from the international evidence-based guideline for the assessment and management of polycystic ovary syndrome. Hum Reprod (2018) 33(9):1602–18. doi: 10.1093/humrep/dey256
12. Rotterdam ESHRE/ASRM-Sponsored PCOS consensus workshop group. Revised 2003 consensus on diagnostic criteria and long-term health risks related to polycystic ovary syndrome (PCOS). Hum Reprod (2004) 19(1):41–7. doi: 10.1093/humrep/deh098
13. Escobar-Morreale HF. Polycystic ovary syndrome: definition, aetiology, diagnosis and treatment. Nat Rev Endocrinol (2018) 14(5):270–84. doi: 10.1038/nrendo.2018.24
14. Bulsara J, Patel P, Soni A, Acharya S. A review: Brief insight into Polycystic Ovarian syndrome. Endocr Metab Sci (2021) 3:100085. doi: 10.1016/j.endmts.2021.100085
15. El Hayek S, Bitar L, Hamdar LH, Mirza FG, Daoud G. Poly cystic ovarian syndrome: an updated overview. Front Physiol (2016) 7:124–4. doi: 10.3389/fphys.2016.00124
16. Escobar-Morreale HF, Luque-Ramírez M, San Millán JL. The molecular-genetic basis of functional hyperandrogenism and the polycystic ovary syndrome. Endocr Rev (2005) 26(2):251–82. doi: 10.1210/er.2004-0004
17. Legro RS, Arslanian SA, Ehrmann DA, Hoeger KM, Murad MH, Pasquali R, et al. Diagnosis and treatment of polycystic ovary syndrome: an endocrine society clinical practice guideline. J Clin Endocrinol Metab (2013) 98(12):4565–92. doi: 10.1210/jc.2013-2350
18. Gibson-Helm M, Teede H, Dunaif A, Dokras A. Delayed diagnosis and a lack of information associated with dissatisfaction in women with polycystic ovary syndrome. J Clin Endocrinol Metab (2017) 102(2):604–12. doi: 10.1210/jc.2016-2963
19. Poole D, Alan M, Goebel R. Computational Intelligence: A Logical Approach. New York: Oxford University Press (1998).
20. Choi RY, Coyner AS, Kalpathy-Cramer J, Chiang MF, Campbell JP. Introduction to machine learning, neural networks, and deep learning. Trans Vision Sci Technol (2020) 9(2):14. doi: 10.1167/tvst.9.2.14
21. Rashidi HH, Tran N, Albahra S, Dang LT. Machine learning in health care and laboratory medicine: General overview of supervised learning and Auto-ML. Int J Lab Hematol (2021) 43(S1):15–22. doi: 10.1111/ijlh.13537
22. Silva IS, Ferreira CN, Costa LBX, Sóter MO, Carvalho LML, de Albuquerque C J, et al. Polycystic ovary syndrome: clinical and laboratory variables related to new phenotypes using machine-learning models. J Endocrinol Invest (2022) 45(3):497–505. doi: 10.1007/s40618-021-01672-8
23. Mehrotra P, Chatterjee J, Chakraborty C, Ghoshdastidar B, Ghoshdastidar S. Automated screening of Polycystic Ovary Syndrome using machine learning techniques, In: 2011 Annual IEEE India Conference (2011).
24. Kumar HP, Srinivasan S. Segmentation of polycystic ovary in ultrasound images, In: Second International Conference on Current Trends In Engineering and Technology - ICCTET, Vol. 2014 (2014).
25. Sitheswaran R, Malarkhodi S. (2014)., In: 2014 International Conference on Electronics and Communication Systems (ICECS).
26. Shekhar S, Barrera FJ, Brown E, Rojo A, Obeso J, Plata H, et al. Artificial intelligence and machine learning in the detection of polycystic ovarian syndrome: A systematic review and meta-analysis. Endocr Pract (2022) 29(5):S88–9. doi: 10.1016/j.eprac.2023.03.198
27. Nazarudin AA, Zulkarnain N, Mokri SS, Zaki WMDW, Hussain A. An implementation of Otsu thresholding and the Chan–Vese method on the PCO segmentation of ultrasound images, In: 2020 2nd International Conference on Electrical, Control and Instrumentation Engineering (ICECIE) (2020). pp. 1–9.
28. Bharati S, Podder P, Hossain Mondal MR. Diagnosis of polycystic ovary syndrome using machine learning algorithms, In: 2020 IEEE Region 10 Symposium (TENSYMP) (2020). pp. 1486–9.
29. Cahyono B, Adiwijaya , Mubarok MS, Wisesty UN. An implementation of convolutional neural network on PCO classification based on ultrasound image, In: 2017 5th International Conference on Information and Communication Technology (ICoIC7) (2017).
30. Castro V, Shen Y, Yu S, Finan S, Pau CT, Gainer V, et al. Identification of subjects with polycystic ovary syndrome using electronic health records. Reprod Biol Endocrinol (2015) 13:116. doi: 10.1186/s12958-015-0115-z
31. RoyChoudhury S, Mishra BP, Khan T, Chattopadhayay R, Lodh I, Datta Ray C, et al. Serum metabolomics of Indian women with polycystic ovary syndrome using (1)H NMR coupled with a pattern recognition approach. Mol Biosyst (2016) 12(11):3407–16. doi: 10.1039/C6MB00420B
32. Rodriguez EM, Thomas D, Druet A, Vlajic-Wheeler M, Lane KJ, Mahalingaiah S. Identifying women at risk for polycystic ovary syndrome using a mobile health app: virtual tool functionality assessment. JMIR Form Res (2020) 4(5):e15094. doi: 10.2196/15094
33. Purnama B, Adiwijaya Wisesti UN, Nhita F, Gayatri A, Mutiah T. A classification of polycystic Ovary Syndrome based on follicle detection of ultrasound images, In: 2015 3rd International Conference on Information and Communication Technology (ICoICT), Nusa Dua, Bali, Indonesia (2015). pp. 396–401. doi: 10.1109/ICoICT.2015.7231458
34. Prapty AS, Shitu TT. An efficient decision tree establishment and performance analysis with different machine learning approaches on polycystic ovary syndrome, In: 2020 23rd International Conference on Computer and Information Technology (ICCIT) (2020). pp. 1–5.
35. Chauhan P, Patil P, Rane N, Raundale P, Kanakia H. Comparative analysis of machine learning algorithms for prediction of PCOS, In: 2021 International Conference on Communication information and Computing Technology (ICCICT), Mumbai, India (2021). pp. 1–7. doi: 10.1109/ICCICT50803.2021.9510128
36. Lawrence MJ, Eramian MG, Pierson RA, Neufeld E. Computer assisted detection of polycystic ovary morphology in ultrasound images, In: Fourth Canadian Conference on Computer and Robot Vision (CRV '07), Montreal, QC, Canada (2007). pp. 105–12. doi: 10.1109/CRV.2007.18
37. Matharoo-Ball B, Hughes C, Lancashire L, Tooth D, Ball G, Creaser C, et al. Characterization of biomarkers in polycystic ovary syndrome (PCOS) using multiple distinct proteomic platforms. J Proteome Res (2007) 6(8):3321–8. doi: 10.1021/pr070124b
38. Lehtinen JC, Forsström J, Koskinen P, Penttilä TA, Järvi T, Anttila L. Visualization of clinical data with neural networks, case study: polycystic ovary syndrome. Int J Med Inform (1997) 44(2):145–55. doi: 10.1016/S1386-5056(96)01265-8
39. Kumar HP, Srinivasan S. Classification of ovary abnorMality using the probabilistic neural network (PNN). Technol Health Care (2014) 22(6):857–65. doi: 10.3233/THC-140863
40. Madhumitha J, Kalaiyarasi M, Ram SS. Automated polycystic ovarian syndrome identification with follicle recognition, In: 2021 3rd International Conference on Signal Processing and Communication (ICPSC) (2021).
41. Ho CH, Chang CM, Li HY, Shen HY, Lieu FK, Wang PS. Dysregulated immunological and metabolic functions discovered by a polygenic integrative analysis for PCOS. Reprod BioMed Online (2020) 40(1):160–7. doi: 10.1016/j.rbmo.2019.09.011
42. Gopalakrishnan C, Iyapparaja M. Multilevel thresholding based follicle detection and classification of polycystic ovary syndrome from the ultrasound images using machine learning. Int J System Assur Eng Manage (2021). doi: 10.1007/s13198-021-01203-x
43. Dong F, Deng D, Chen H, Cheng W, Li Q, Luo R, et al. Serum metabolomics study of polycystic ovary syndrome based on UPLC-QTOF-MS coupled with a pattern recognition approach. Anal Bioanal Chem (2015) 407(16):4683–95. doi: 10.1007/s00216-015-8670-x
44. Deshpande SS, Wakankar A. Automated detection of Polycystic Ovarian Syndrome using follicle recognition, In: 2014 IEEE International Conference on Advanced Communications, Control and Computing Technologies (2014).
45. Denny A, Raj Dev A, Ashok A, Ram C, George R. i-HOPE: detection and prediction system for polycystic ovary syndrome (PCOS) using machine learning techniques, In: TENCON 2019 - 2019 IEEE Region 10 Conference, (TENCON) (2019). pp. 673–8.
46. Deng Y, Wang Y, Shen Y. An automated diagnostic system of polycystic ovary syndrome based on object growing. Artif Intell Med (2011) 51(3):199–209. doi: 10.1016/j.artmed.2010.10.002
47. Dapas M, Lin FTJ, Nadkarni GN, Sisk R, Legro RS, Urbanek M, et al. Distinct subtypes of polycystic ovary syndrome with novel genetic associations: An unsupervised, phenotypic clustering analysis. PloS Med (2020) 17(6):e1003132. doi: 10.1371/journal.pmed.1003132
48. Che Q, Liu M, Xu J, Liu Y, Cao X, Dong X, et al. Characterization of circular RNA expression profiles in cumulus cells from patients with polycystic ovary syndrome. Fertil Steril (2019) 111(6):1243–1251.e1. doi: 10.1016/j.fertnstert.2019.02.023
49. Cheng JJ, Mahalingaiah S. Data mining polycystic ovary morphology in electronic medical record ultrasound reports. Fertil Res Pract (2019) 5(1):13. doi: 10.1186/s40738-019-0067-7
50. Zhang X, Liang B, Zhang J, Hao X, Xu X, Chang HM, et al. Raman spectroscopy of follicular fluid and plasma with machine-learning algorithms for polycystic ovary syndrome screening. Mol Cell Endocrinol (2021) 523:111139. doi: 10.1016/j.mce.2020.111139
51. Xie NN, Wang FF, Zhou J, Liu C, Qu F. Establishment and analysis of a combined diagnostic model of polycystic ovary syndrome with random forest and artificial neural network. BioMed Res Int (2020) 2020:2613091. doi: 10.1155/2020/2613091
52. Vedpathak S, Thakre V. PCOcare: PCOS detection and prediction using machine learning algorithms. Biosci Biotechnol Res Commun (2020) 13:240–4. doi: 10.21786/bbrc/13.14/56
53. Vikas B, Anuhya BS, Bhargav KS, Sarangi S, Chilla M. Application of the apriori algorithm for prediction of polycystic ovarian syndrome (PCOS). In: Bhateja V, Nguyen B, Nguyen N, Satapathy S, Le DN editors. Information Systems Design and Intelligent Applications. Singapore: Springer (2018). vol 672. doi: 10.1007/978-981-10-7512-4_93
54. Setiawati E, Wirayuda A, Tjokorda AB, Widi A. A classification of polycystic ovary syndrome based on ultrasound images using supervised learning and particle swarm optimization. Advanced Sci Lett (2016) 22:1997–2001. doi: 10.1166/asl.2016.7757
55. Rihana S, Moussallem H, Skaf C, Yaacoub C. Automated algorithm for ovarian cysts detection in ultrasonogram, In: 2013 2nd International Conference on Advances in Biomedical Engineering, Lebanon (2013). pp. 219–22. doi: 10.1109/ICABME.2013.6648887
56. Deng Y, Wang Y, Chen P. Automated detection of Polycystic Ovary Syndrome from ultrasound images. Annu Int Conf IEEE Eng Med Biol Soc (2008) 2008:4772–5. doi: 10.1109/IEMBS.2008.4650280
57. Zawadski JK DA. Diagnostic criteria for polycystic ovary syndrome: Towards a rational approach. In: G.J. Dunaif A, Haseltine FP, Merriam GE, editors. Polycystic Ovary Syndrome (Current Issues in Endocrinology and Metabolism). Boston: Blackwell Scientific Inc (1992). p. 377.
58. Adams J, Franks S, Polson DW, Mason HD, Abdulwahid N, Tucker M, et al. Multifollicular ovaries: clinical and endocrine features and response to pulsatile gonadotropin releasing hormone. Lancet (1985) 2(8469-70):1375–9. doi: 10.1016/S0140-6736(85)92552-8
59. Morrison SL, Dukhovny D, Chan RVP, Chiang MF, Campbell JP. Cost-effectiveness of artificial intelligence-based retinopathy of prematurity screening. JAMA Ophthalmol (2022) 140(4):401–9. doi: 10.1001/jamaophthalmol.2022.0223
60. Areia M, Mori Y, Correale L, Repici A, Bretthauer M, Sharma P, et al. Cost-effectiveness of artificial intelligence for screening colonoscopy: a modelling study. Lancet Digit Health (2022) 4(6):e436–44. doi: 10.1016/S2589-7500(22)00042-5
61. Kacew AJ, Strohbehn GW, Saulsberry L, Laiteerapong N, Cipriani NA, Kather JN, et al. Artificial intelligence can cut costs while maintaining accuracy in colorectal cancer genotyping. Front Oncol (2021) 11:630953. doi: 10.3389/fonc.2021.630953
62. Hong N, Park H, Rhee Y. Machine learning applications in endocrinology and metabolism research: an overview. Endocrinol Metab (Seoul) (2020) 35(1):71–84. doi: 10.3803/EnM.2020.35.1.71
63. Benjamens S, Dhunnoo P, Meskó B. The state of artificial intelligence-based FDA-approved medical devices and algorithms: an online database. NPJ Digital Med (2020) 3(1):118. doi: 10.1038/s41746-020-00324-0
64. Padhy SK, Takkar B, Chawla R, Kumar A. Artificial intelligence in diabetic retinopathy: A natural step to the future. Indian J Ophthalmol (2019) 67(7):1004–9. doi: 10.4103/ijo.IJO_1989_18
65. VanHise K, Chan JL, Wertheimer S, Handelsman RG, Clark E, Buttle R, et al. Regional variation in hormonal and metabolic parameters of white and black women with PCOS in the United States. J Clin Endocrinol Metab (2023) 108(3):706–12. doi: 10.1210/clinem/dgac515
66. Maleki F, Muthukrishnan N, Ovens K, Reinhold C, Forghani R. Machine learning algorithm validation: from essentials to advanced applications and implications for regulatory certification and deployment. Neuroimaging Clini N Am (2020) 30(4):433–45. doi: 10.1016/j.nic.2020.08.004
67. Health, N.I.o. All of Us Research Program (2021). Available at: https://allofus.nih.gov/.
68. Statistics, N.C.f.H. National Health and Nutrition Examination Survey (2023). Available at: https://www.cdc.gov/nchs/nhanes/index.htm.
Keywords: artificial intelligence, machine learning, polycystic ovarian syndrome (PCOS), diagnosis, classification, Stein-Leventhal syndrome
Citation: Barrera FJ, Brown EDL, Rojo A, Obeso J, Plata H, Lincango EP, Terry N, Rodríguez-Gutiérrez R, Hall JE and Shekhar S (2023) Application of machine learning and artificial intelligence in the diagnosis and classification of polycystic ovarian syndrome: a systematic review. Front. Endocrinol. 14:1106625. doi: 10.3389/fendo.2023.1106625
Received: 24 November 2022; Accepted: 04 August 2023;
Published: 18 September 2023.
Edited by:
Masoud Afnan, Qingdao United Family Hospital, ChinaReviewed by:
Noraishikin Zulkarnain, Universiti Kebangsaan Malaysia, MalaysiaCopyright © 2023 Barrera, Brown, Rojo, Obeso, Plata, Lincango, Terry, Rodríguez-Gutiérrez, Hall and Shekhar. This is an open-access article distributed under the terms of the Creative Commons Attribution License (CC BY). The use, distribution or reproduction in other forums is permitted, provided the original author(s) and the copyright owner(s) are credited and that the original publication in this journal is cited, in accordance with accepted academic practice. No use, distribution or reproduction is permitted which does not comply with these terms.
*Correspondence: Skand Shekhar, c2thbmQuc2hla2hhckBuaWguZ292
Disclaimer: All claims expressed in this article are solely those of the authors and do not necessarily represent those of their affiliated organizations, or those of the publisher, the editors and the reviewers. Any product that may be evaluated in this article or claim that may be made by its manufacturer is not guaranteed or endorsed by the publisher.
Research integrity at Frontiers
Learn more about the work of our research integrity team to safeguard the quality of each article we publish.