- 1University Cologne, Faculty of Medicine and University Hospital Cologne, Department of Diagnostic and Interventional Radiology, Cologne, Germany
- 2Fraunhofer Institute of Optronics, System Technologies and Image Exploitation IOSB, Karlsruhe, Germany
Purpose: The bone marrow’s iodine uptake in dual-energy CT (DECT) is elevated in malignant disease. We aimed to investigate the physiological range of bone marrow iodine uptake after intravenous contrast application, and examine its dependence on vBMD, iodine blood pool, patient age, and sex.
Method: Retrospective analysis of oncological patients without evidence of metastatic disease. DECT examinations were performed on a spectral detector CT scanner in portal venous contrast phase. The thoracic and lumbar spine were segmented by a pre-trained neural network, obtaining volumetric iodine concentration data [mg/ml]. vBMD was assessed using a phantomless, CE-certified software [mg/cm3]. The iodine blood pool was estimated by ROI-based measurements in the great abdominal vessels. A multivariate regression model was fit with the dependent variable “median bone marrow iodine uptake”. Standardized regression coefficients (β) were calculated to assess the impact of each covariate.
Results: 678 consecutive DECT exams of 189 individuals (93 female, age 61.4 ± 16.0 years) were evaluated. AI-based segmentation provided volumetric data of 97.9% of the included vertebrae (n=11,286). The 95th percentile of bone marrow iodine uptake, as a surrogate for the upper margin of the physiological distribution, ranged between 4.7-6.4 mg/ml. vBMD (p <0.001, mean β=0.50) and portal vein iodine blood pool (p <0.001, mean β=0.43) mediated the strongest impact. Based thereon, adjusted reference values were calculated.
Conclusion: The bone marrow iodine uptake demonstrates a distinct profile depending on vBMD, iodine blood pool, patient age, and sex. This study is the first to provide the adjusted reference values.
1 Introduction
Neo-angiogenesis is a crucial molecular pathway to mediate malignant disease, since proliferating neoplastic cells require abundant supply with oxygen and nutrients (1). In dual-energy computed tomography (DECT), the elevated perfusion of a malignant tumor can be assessed by measuring the voxel-specific iodine concentration after intravenous contrast media application (2). By now, DECT has become a widely available technology and the iodine concentration is extensively obtained for the diagnosis, characterization, and treatment response monitoring of extra-skeletal malignant disease (3–10).
Despite its underrepresentation in recent literature, DECT iodine concentration imaging of the skeleton might be of particular interest, since conventional CT has only limited capability to detect malignant bone marrow disease (11, 12). Without dominating osteolytic or osteoblastic characteristics, bone marrow malignancy of the spine is regularly disguised by dense trabecular structure (12, 13). Compared to the gold standard magnetic resonance imaging (MRI) and positron emission tomography (PET), conventional CT only yielded a sensitivity of 0.77 and 0.63 to detect vertebral metastasis, or to diagnose bone marrow infiltration by Hodgkin lymphoma, respectively (11, 14). This diagnostic gap of conventional CT represents a major clinical limitation, since the spine is among the most common sites of malignant bone marrow infiltration (13–16).
To narrow this gap, several authors suggested to quantify the iodine concentration at small, non-specific lucencies or non-specific architectural distortions of the cancellous bone (17, 18). Further, the iodine concentration has been investigated to improve the diagnostic accuracy of the detection of spinal metastasis and to facilitate differential diagnosis of malignant spinal disease (6, 19–21). Despite its promising role in oncological skeletal imaging, there is a lack of reference values describing the normal iodine concentration of the spinal bone marrow in DECT. A possible explanation for this deficiency might be the expected dependence of vertebral iodine concentration assessment on the bone mineral density (BMD) and the iodine blood pool (22, 23).
The aim of this study was to provide an in-detail, adjusted database of the physiological iodine uptake of the spine’s bone marrow. Besides the dependence on the BMD and the iodine blood pool, we further aimed to investigate the influence of patient age and sex on the physiological bone marrow iodine uptake.
2 Materials and methods
All procedures performed in studies involving human participants were conducted in accordance with the ethical standards of the institutional (application number 21-1105) and national research committee and with the 1964 Helsinki declaration and its later amendments or comparable ethical standards. Informed consent was waived due to retrospective study characteristics.
Results are reported in line with the Strengthening the Reporting of Observational Studies in Epidemiology (STROBE) recommendations.
2.1 Patient enrollment
The patient population was enrolled by reviewing the institutional database for the below specified eligibility criteria.
2.1.1 Inclusion criteria were:
1) Referral by the department of dermatology between May 2016 and January 2020 after resection of a malignant skin tumor to exclude metastatic disease,
2) DECT examination of the chest and abdomen using the below specified imaging protocol,
3) Patient age >18 years.
Follow-up examinations without evidence of macroscopic metastatic disease were included until January 2021.
2.1.2 Exclusion criteria were:
1) Metal implants of the spine (n = 18 examinations of 5 patients),
2) Incomplete or corrupted DECT data (n = 26 examinations of 25 patients).
2.2 DECT imaging protocol
Patients were examined on a commercially available spectral detector DECT scanner (IQon Spectral CT, Philips Healthcare) in a head first, supine position. All scans were performed after intravenous administration of 100 ml iodine-based contrast agent with a flow rate of 3.0 ml/s (Accupaque 350, GE Healthcare). Contrast administration was followed by a saline flush of 30 ml. Bolus tracking in the descending thoracic aorta indicated portal venous phase with a delay of 50 s after surpassing a threshold of 150 HU. Tube voltage was 120 kV, the tube current was modulated by DoseRight 3D-DOM (Philips Healthcare). The collimation was 64 × 0.625 mm and the pitch 0.671.
2.3 DECT image reconstruction and post-processing
Anatomic conventional images were reconstructed using a hybrid-iterative reconstruction algorithm in “bone” preset (iDose4, convolution kernel C, Philips Healthcare). Spectral based raw data was processed to iodine concentration maps using the vendor’s proprietary software (IntelliSpace Portal 11.0, Spectral Diagnostics Suite, Philips Healthcare). All images were reconstructed in axial slices, 512 x 512 matrix, thickness 2 mm with an overlap of 1 mm. Voxel size was 0.89 x 0.89 x 2.00 mm.
2.4 Assessment of the covariate vBMD
Volumetric BMD (vBMD) was assessed phantomless by a CE-certified software in an standardized approach (IntelliSpace Portal 11.0, Bone Mineral Density, Philips Healthcare) (24). An ellipsoid volume of interest with a thickness of 9 mm was placed in the anterior portion of the vertebral body, sparing the surrounding cortical bone. Hence, vBMD measurements assessed only the cancellous part of the bone. In-body calibration to the mineral scale was achieved by analogous measurements in the paravertebral muscle and subcutaneous fatty tissue. To correct for the minor overestimation of vBMD measurements after intravenous contrast application, we applied the adjustment formula that was introduced by Abdullayev et al. and validated in consecutive research (6, 25–27). The correction formula is as follows: corrected vBMD = 0.88 * portal venous vBMD + 4.56 mg/cm3. Since the vBMD is a relatively stable biomarker (average expected change per year among women: 1.6%), we did not perform more than one measurement per patient and follow-up year (365 days) (28).
2.5 Assessment of the covariate iodine blood pool
The iodine blood pool was estimated by two region-of-interest-based, circular measurements in the portal vein and the abdominal aorta at the level of the kidney vessels, as suggested by previous studies (29, 30).
2.6 AI-based assessment of the bone marrow
The bone marrow was assessed by using artificial intelligence (AI), without requiring specific user interaction. First, the spine was segmented by a pre-trained, convolutional neural network by Payer et al. (31, 32). The neural network proved excellent performance with a dice coefficient of 0.94 and a correct vertebrae labelling rate of 0.99, based on analogous data to our study (120-kV acquisition with axial reformations, “bone” kernel favoring sharpness over noise, spatial resolution at least 1 mm) (33). After automated segmentation, the vertebrae were separated in lumbar and thoracic subsets. To exclude the bordering cortical bone, which does not contain bone marrow, the segmentation margins of each vertebra were narrowed by 3 mm using the SciPy command “scipy.ndimage.binary_erosion” (34). Consecutively, the thoracic and lumbar bone marrow segmentation masks were transferred from the conventional bone kernel image series to the iodine concentration maps. Automated assessment of the bone-marrow space is illustrated in Figure 1.
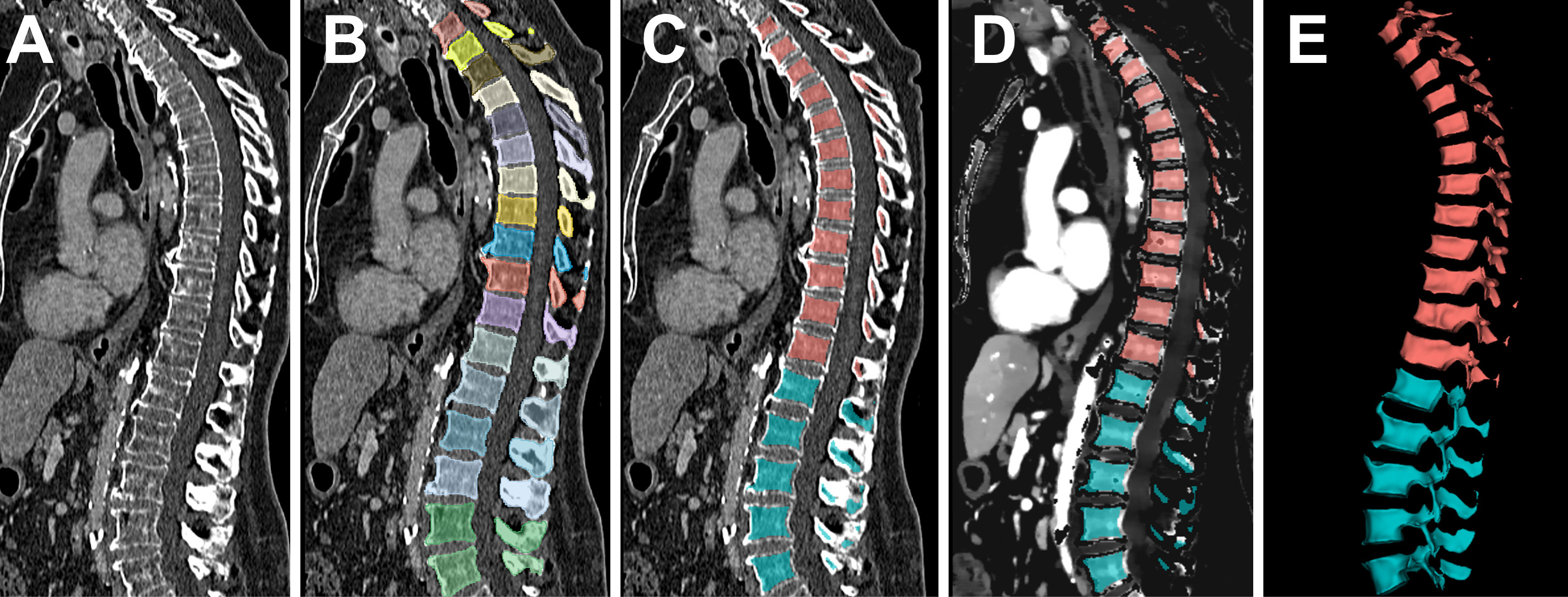
Figure 1 Automated assessment of the bone marrow iodine uptake. Conventional CT images served as the input to the pre-trained convolutional neural network by Payer et al. (A). After automated, vertebra-by-vertebra segmentation of the spine (B), the bottom-most five vertebrae were adopted as the lumbar segment, followed by the consecutive 12 vertebrae as the thoracic segment (C). The segmentation was narrowed at each margin by 3 mm to exclude the bordering cortical bone, since it does not contain bone marrow. Consecutively, the bone marrow segmentation mask was transferred to the iodine concentration maps (D) and extracted as volumetric iodine concentration data for the lumbar and thoracic spine (E).
Evaluation of the iodine concentration of the bone marrow space
Voxel-wise iodine concentration was extracted from both the thoracic and lumbar bone marrow volumes as histograms ranging from -0.05 mg/ml to 19.95 mg/ml, with a bin size of 0.05 mg/ml.
2.7 Statistical data assessment
Statistical analysis was performed in R language for statistical computing, R Foundation, Vienna, Austria, version 4.0.0 (35). To test the data for normal distribution, we performed Shapiro-Wilk’s test using the R library dplyr (36).
To evaluate the dependence of the bone marrow iodine uptake on patient sex, age, vBMD, and iodine blood pool in the aorta as well as portal vein, a multivariate regression model was fit by lm(y~x1+x2+x3+x4+x5), including the five above listed independent variables and the dependent variable “median bone marrow iodine concentration”. Both lumbar and thoracic iodine uptake were assessed in an individual model each. Multicollinearity was assessed by the variance inflation factor (VIF), calculated for each independent variable using the R library cars (a VIF <2.5 suggests no significant collinearity) (37, 38). In case of significant collinearity of one or more variables, those variables were excluded one at a time, starting with the highest VIF. After exclusion of multicollinearity, the remaining independent variables were ordered by their impact on the dependent variable, using the standardized regression coefficient β. Each independent variable’s β was calculated using the R library QuantPsyc (39). Finally, reference values of bone marrow iodine uptake were reported grouped by the independent variables, which mediated the strongest impact in the multivariate regression model (highest β). The power level of the final lumbar and thoracic regression models was calculated post hoc using the software G*Power for a sample size of 678 and a significance level of 0.05 (40).
Visualization was achieved using the R library ggplot2 (41). Statistical significance was defined as p ≤ 0.05.
3 Results
In total, 678 DECT scans of 189 patients (93 female, patient age at examination 61.4 ± 16.0 years, range 24.2 – 90.8 years) were evaluated. The median number of included examinations per patient was 3 [2-5]. The most common type of resected malignancy was malignant melanoma (184/189, 97.3%), followed by Merkel cell carcinoma (3/189, 1.6%), and squamous cell carcinoma (2/189, 1.1%). Automated spine segmentation successfully yielded volumetric bone marrow data of 3390/3390 (100.0%) of included lumbar and 7896/8136 (97.1%) of included thoracic vertebrae, respectively. Mean number of included voxels was 95,987 ± 46,269 and 79,533 ± 50,127 per segmented lumbar and thoracic bone marrow space, respectively.
3.1 Assessment of the covariate vBMD
We performed vBMD measurements on 334/678 (49.3%) of the included DECT scans. For the other 344 examinations, which were not subject to vBMD quantification, the vBMD of the most approximate previous- or follow-up-scan was adopted for further analysis. In those cases, the mean time to the most approximate vBMD measurement was 192 ± 68 days. Mean vBMD was 85.6 ± 28.1 mg/cm3 (Figure 2).
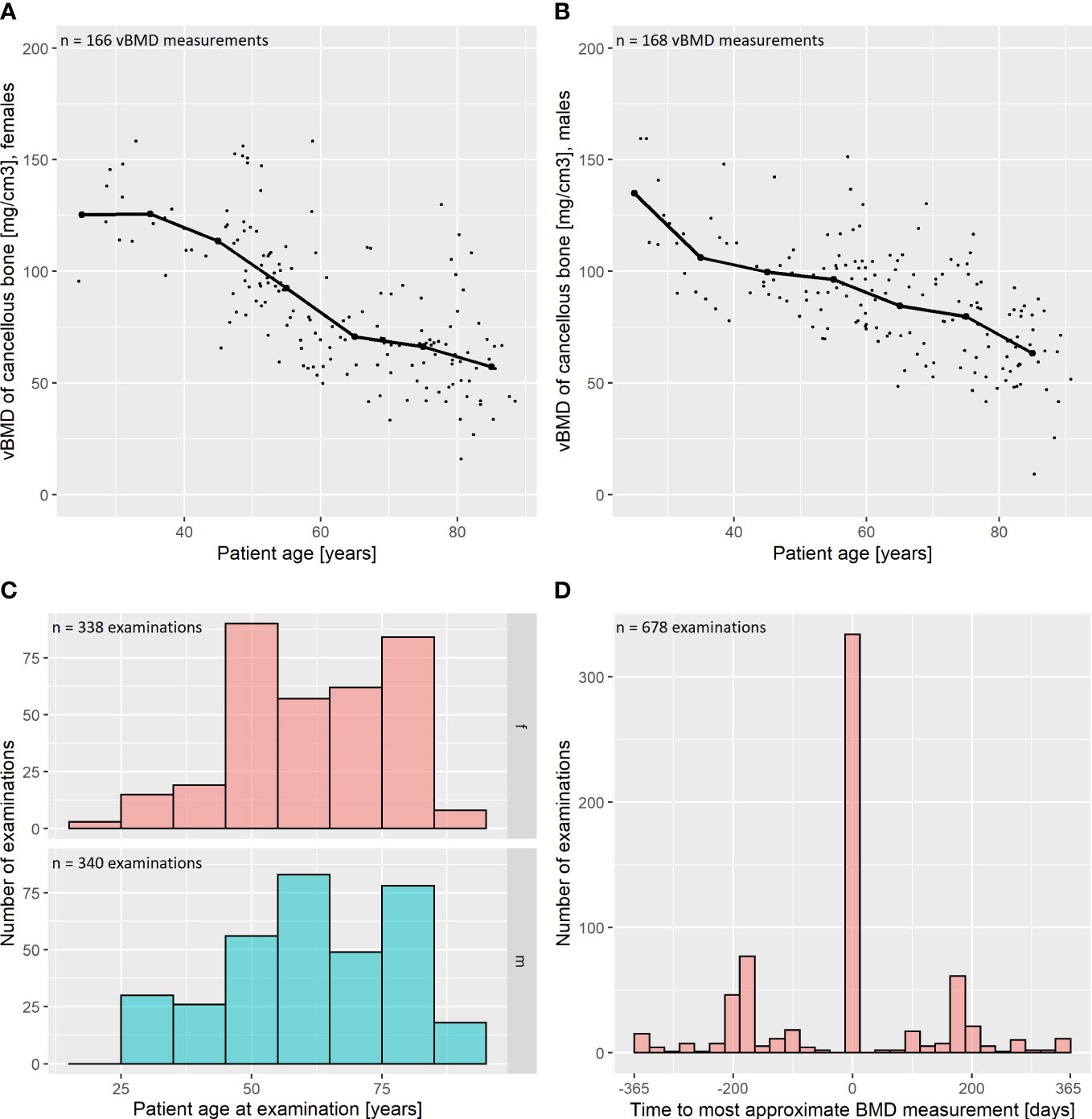
Figure 2 Descriptive statistics: bone mineral density and patient age. (A, B) Volumetric bone mineral density (vBMD) was assessed phantomless by an in-body calibrated, CE-certified software. The vBMD was corrected for intravenous contrast administration as suggested by Abdullayev et al., 2018 (25). Results are reported for female (A) and male (B) patients. The vBMD declines with increasing age of the assessed patients. Median values for intervals of 10 years are marked as thick scatter points with a connecting line. (C) Distribution of patient age in our study population, grouped by sex (f = female; m = male). (D) vBMD measurements were performed on 334 out of 678 included CT examinations (49.3%). For the remaining 344 examinations, the measurement of the most approximate CT was adopted for further analysis. In those cases, the mean time to the most approximate vBMD measurement was 192 ± 68 days, which is plotted as a histogram.
3.2 Assessment of the covariate iodine blood pool
ROI-based measurements of the abdominal aorta and portal vein yielded a mean iodine concentration of 4.7 ± 1.1 mg/ml and 5.2 ± 1.2 mg/ml, respectively. We observed a strong, linear relationship of the median iodine concentrations in the aorta and portal vein, which was modelled by a linear regression with the formula iodineaorta = 0.79 * iodineportal vein + 0.59 mg/ml (r² = 0.78, Figure 3), indicating collinearity.
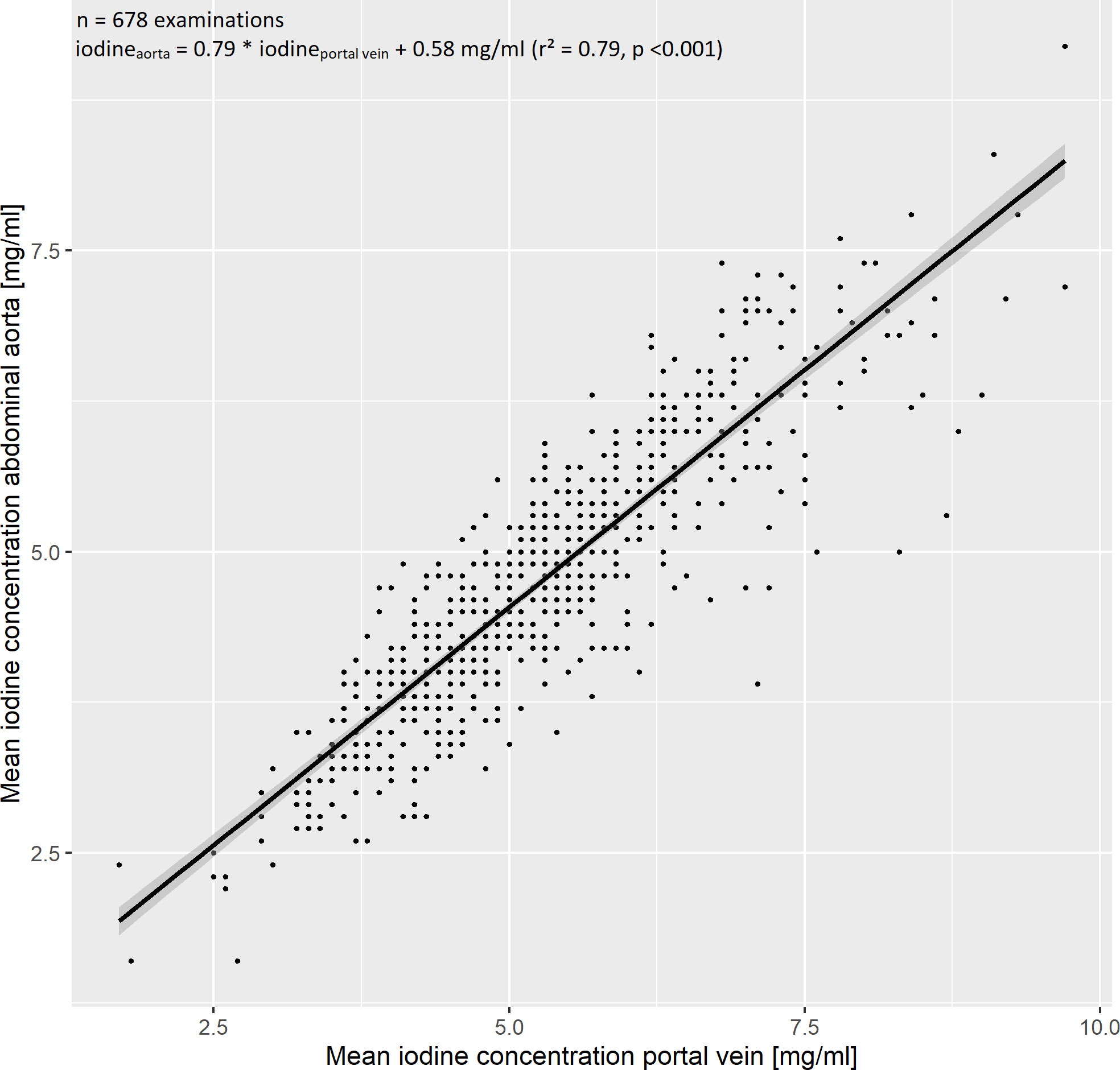
Figure 3 Evaluation of the iodine blood pool. The iodine blood pool was estimated by region of interest-based iodine concentration measurements in the abdominal aorta and portal vein. We observed a strong linear relationship, which was modelled by the regression equation iodineaorta = 0.79 * iodineportal vein + 0.58 mg/ml (r² = 0.79). The 95% confidence interval is marked by a grey band.
3.3 Fitting the multivariate regression analysis
To investigate the influence of patient age, sex, iodine blood pool (aorta as well as portal vein), and vBMD on the bone marrow’s iodine uptake, we fit two multivariate regression models with the five above enumerated independent variables and the dependent variable “median bone marrow iodine concentration of the lumbar spine” and “…of the thoracic spine”, respectively. The preliminary models including all five independent variables suggested collinearity of the iodine blood pool in the aorta and portal vein (VIFaorta = 4.95, VIFportal vein = 4.70). After exclusion of the abdominal aorta iodine blood pool, significant multicollinearity was ruled out (VIFs < 2.5). See Figure 4 for the exemplary plot of the final thoracic model.
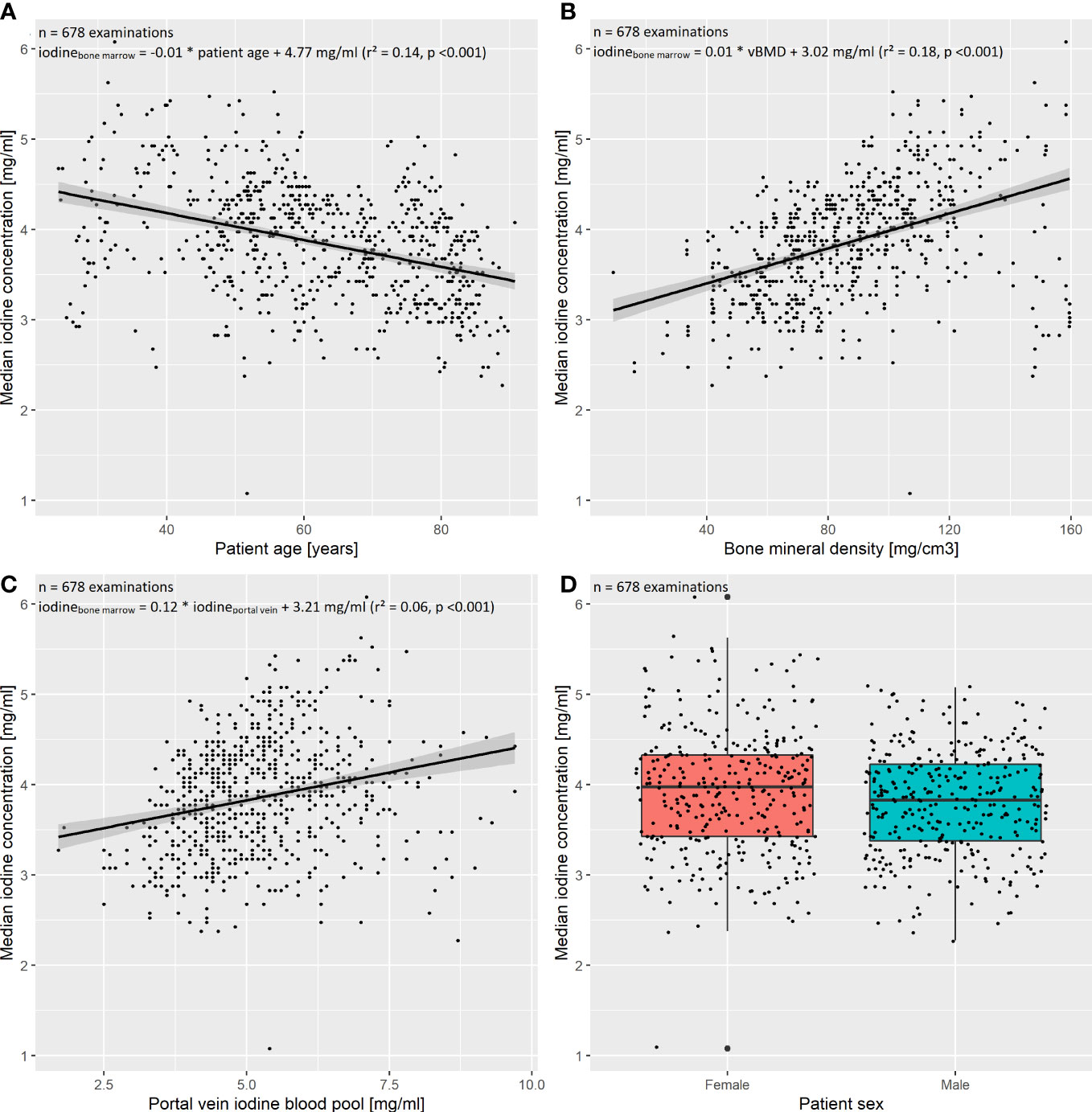
Figure 4 Multivariate regression of the thoracic spine’s bone marrow iodine uptake. The final multivariate regression model was fit including the independent variables (A) patient age, (B) bone mineral density (BMD), (C) portal vein iodine blood pool, as well as (D) patient sex, and the dependent variable “median bone marrow iodine concentration of the thoracic spine”. After exclusion of multicollinearity, the four independent variables remained significant regressors (p < 0.05). The multivariate model proved significant (F(4,673) = 89.53, p<0.001), with a multiple R²=0.34. The standardized regression coefficients β were calculated to order the independent variables by their impact on the dependent variable. The portal vein iodine blood pool had the largest impact on the thoracic bone marrow iodine uptake (β = 0.44), followed by the BMD, patient age, and sex (β = 0.35, β = -0.27, and β = 0.08, respectively).
The final models are reported in-detail in Table 1.
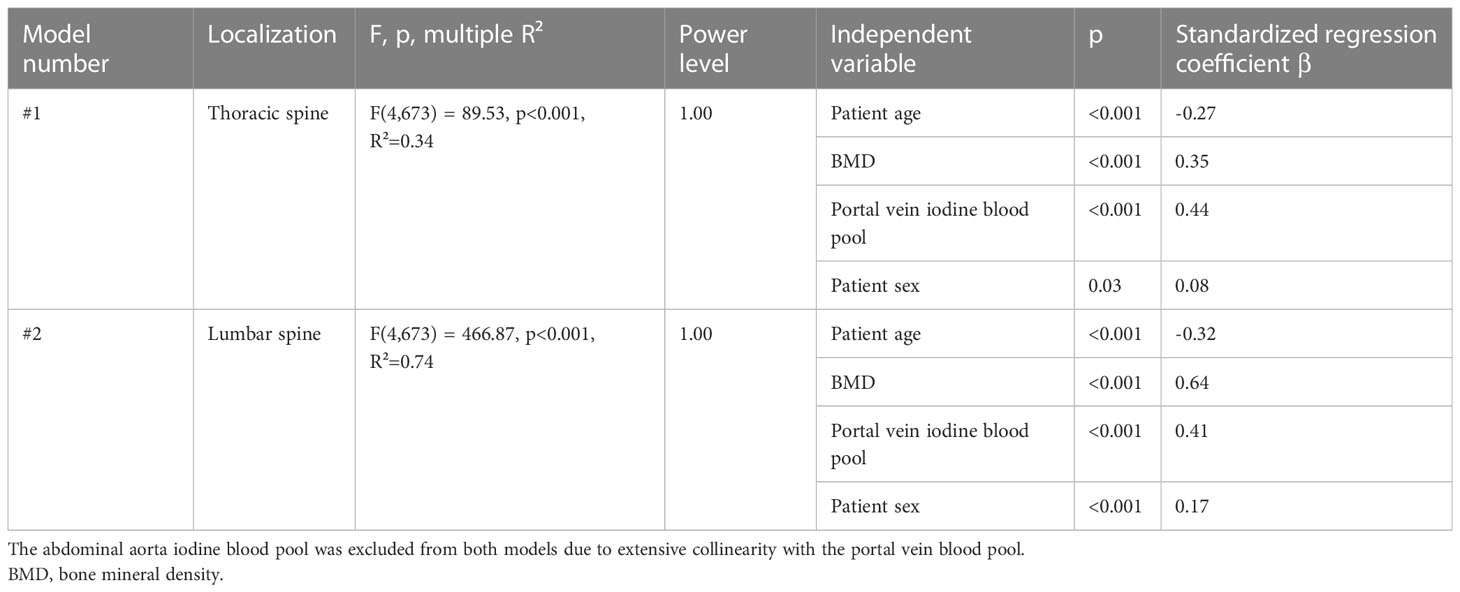
Table 1 Final regression models to evaluate the relationships between the bone marrow iodine uptake and the included independent variables.
Within both final regression models, patient age, sex, vBMD, and the portal vein iodine blood pool were significant regressors. Mean standardized regression coefficients β were -0.30, 0.50, 0.43, and 0.13 for patient age, vBMD, iodine blood pool, and patient sex, respectively. I.e., assuming one standardized magnitude of change in iodine blood pool, a corresponding change of the vBMD had the 1.16-fold effect (0.50/0.43) on the bone marrow iodine uptake.
3.4 Quantitative features of the bone marrow’s iodine uptake
Since the vBMD and the iodine blood pool mediated the strongest influence on the bone marrow’s iodine uptake, we report the quantitative iodine reference values grouped by those variables. The cutoff values to define the groups were determined by dividing the dataset into three equal parts, ordered by vBMD and iodine blood pool. Figure 5 illustrates the results as histograms, while Table 2 presents the in-detail descriptive statistics, including median, interquartile range, 95th percentiles, and histogram maximum for each group. The equivalent data, yet grouped by patient age and sex, are documented in Supplementary Material 1.
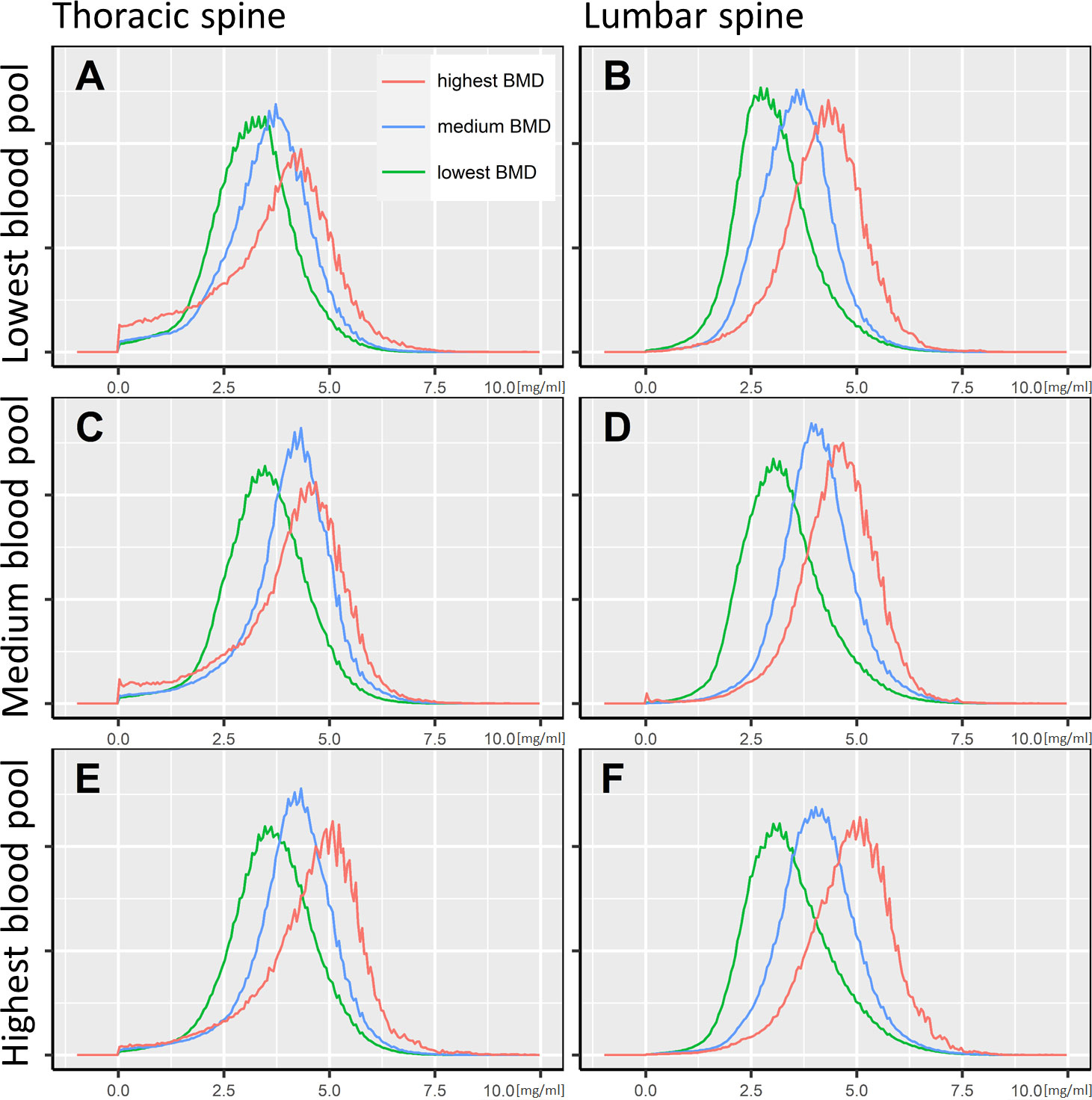
Figure 5 Bone marrow iodine uptake histograms. The iodine concentration distribution of the thoracic and lumbar spine’s bone marrow space is illustrated as histograms, standardized to a common spine volume. Accordingly, all histograms cover an identical area under the curve. The x-axis shows the voxel-wise iodine concentration in mg/ml. Six plots (A–F) are presented, grouped by the portal vein iodine blood pool and the bone mineral density. The cutoff for the lowest to highest iodine blood pool were <4.6 mg/ml, 4.6-5.6 mg/ml, and >5.6 mg/ml, respectively (A/B, C/D, and E/F). Regarding the bone mineral density, the cutoffs were <70.0 mg/cm3, 70.0-96.6 mg/cm3, and >96.6 mg/cm3, respectively (green, blue, and red lines).
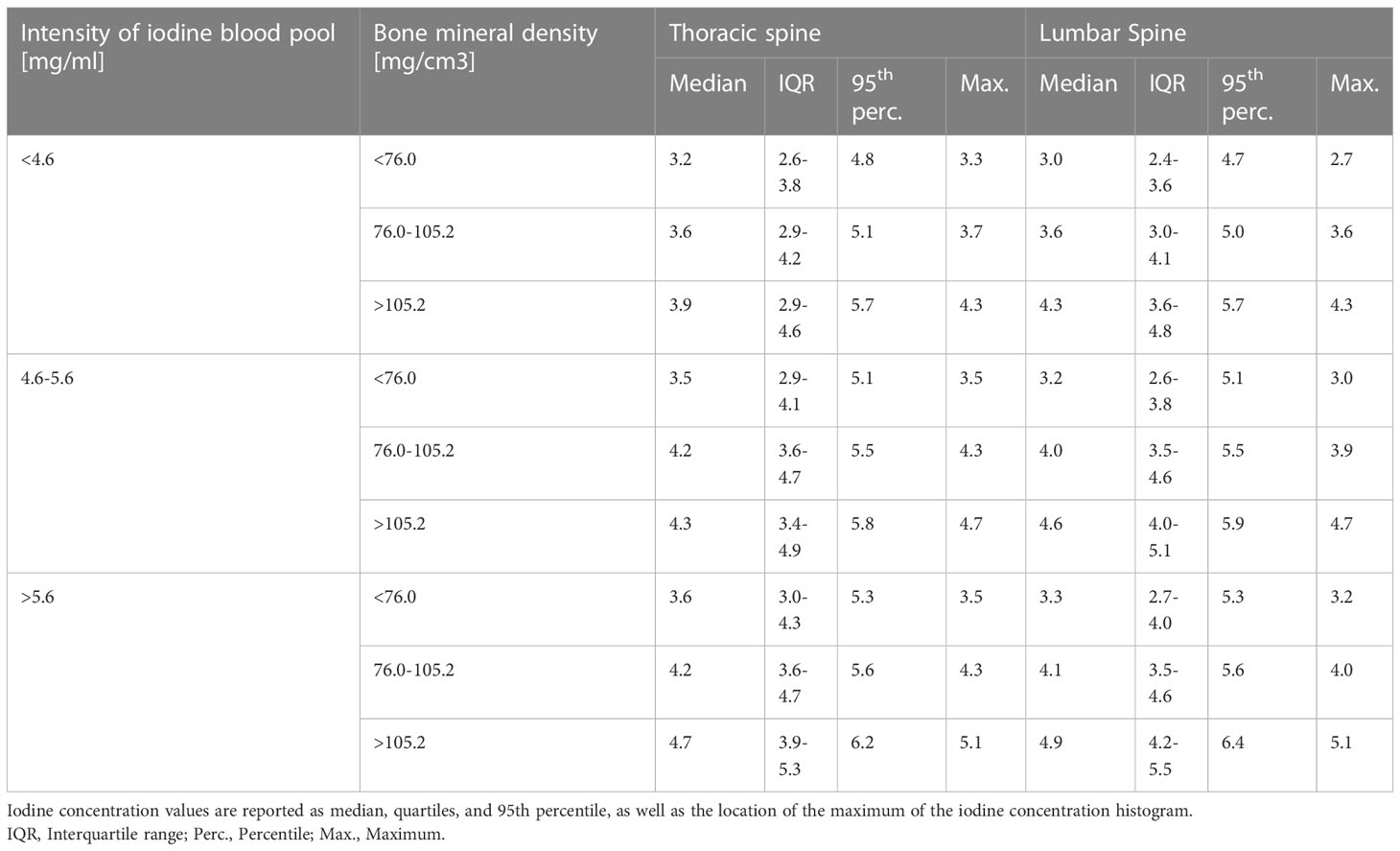
Table 2 Descriptive statistics of the bone marrow’s iodine uptake, grouped by bone mineral density and the intensity of the iodine blood pool.
4 Discussion
Contrast enhanced CT is the most frequently performed imaging procedure for staging of solid cancer. Yet, there is a considerable chance to miss metastatic disease of the spine when examining a conventional CT scan with reported sensitivity of only about 0.77 (11, 12). To narrow this clinical limitation, several authors suggested to evaluate iodine concentration images from DECT, considering that malignant bone disease might stand out by elevated perfusion (6, 17, 18, 42). However, to date there is a lack of data about the physiological iodine uptake of the bone marrow, which is crucially required to delineate pathologically elevated iodine levels. The present study investigated physiological bone marrow iodine uptake on a sample of 11,286 vertebrae in 678 DECT scans, and presents the in-detail quantitative features as its main result. Further, we examined the influence of patient age, sex, the iodine blood pool, and the vBMD on the bone marrow iodine uptake.
The interdependent relation of bone mineral with iodine measurements is a well-studied observation, and might be a reason why the bone marrow iodine uptake is underrepresented among DECT studies. It is well-known, that a certain extent of misclassification of iodine and calcium in DECT material decomposition might occur due to similar x-ray absorption characteristics (6, 22, 26, 43). A recent phantom study at the identical DECT scanner as in our investigation reported a percentage error of iodine concentration in the presence of calcium of no more than 10% (<0.3 mg/ml) (22). Increasing the tube voltage from 120kV to 140kV in dual-layer CT might further reduce calcium-iodine misclassification, which could be exploited in dedicated bone marrow iodine uptake scans (6, 22).
In line with this inherent technical limitation, Borggrefe et al. reported that the iodine density in healthy trabecular bone depends on vBMD, which is, however, insignificant after inclusion of patient age (6). Contrarily, we found that the vBMD mediated the strongest impact on the bone marrow iodine concentration (p <0.001, mean β = 0.50). This finding might be promoted by inclusion of a relatively wide-spread age range of patients to our study (24-90 years), with a larger standard deviation of patient age compared to Borggrefe et al. (16.0 years vs. 12.7 years, respectively) (6). Particularly in young individuals with dense trabecular bone, iodine concentration assessment might be impaired, attributing a significant portion of the iodine concentration variance to vBMD. Yet, we consider the methodology of our study more appropriate to assess such multivariate effects: First, we evaluated a larger sample size (678 vs. 83 DECT examinations) with in total 11,286 vertebrae. Second, we avoided any subjective effects or inter-reader bias by relying on fully automated AI-segmentation of the bone marrow space. Last, the volumetric data in our study evaluated a by-magnitudes-larger iodine data pool than singular, ROI-based measurements. I.e., the mean number of included voxels per examination in our study was 175,520; assuming an in-plane resolution of 1 mm x 1 mm, a circular vertebral ROI with an exemplary diameter of 2 cm comprises only 314 voxels, implying the risk of sampling bias.
In line with Borggrefe et al., we also found a significant decrease of the bone marrow iodine uptake in elderly patients, which remained significant after adjusting for vBMD (p <0.001, mean β = -0.30). This age-dependent loss of bone marrow perfusion is a well-studied observation in histological studies and could be reproduced by our imaging results (44–46). Further, we investigated a significant impact of the iodine blood pool to the bone marrow iodine uptake (p <0.001, mean β = 0.43). This observation agrees with previous studies concerning parenchymatous abdominal organs (23, 29, 30).
Hence, we argue that the DECT bone marrow iodine concentration involves complex interactions, which should be recognized when adopting this imaging biomarker for clinical and scientific use – yet, there was no covariate that individually dominated our results. Assuming that iodine concentration reference values should be adjusted foremost to the vBMD and iodine blood pool, we report the quantitative results of our study accordingly, and provide a summary for clinical considerations in Figure 5 and Table 2.
Recently, Borggrefe et al. examined iodine concentration thresholds to discriminate bone metastasis against healthy appearing trabecular bone on the identical dual-layer CT, using a similar scanning protocol as in our study (6). In their patient population (n=83 patients, mean age 64.6 years), the most performant cutoffs were investigated at an iodine concentration of 4.5-5.0 mg/ml, with a mean iodine concentration of bone metastasis of 5.6 ml/mg. In line with our investigation, Borggrefe et al. discuss that adjustment for vBMD and patient age might improve the performance of iodine concentration thresholds, yet they do not perform such analysis. Their investigated cutoffs locate close to the 95th percentile of bone marrow iodine uptake in the respective age-group of our investigation, while the mean iodine uptake of bone metastasis surpasses the 95th percentile (see Supplementary Material 1, respective 95th percentile at 5.3 mg/ml). The definition of the 95th percentile as an upper margin of the physiological range of a biomarker is a common approach, which enables clinical application of our results (13, 47–49). In clinical practice, an extraordinarily elevated iodine uptake above the adjusted 95th percentile might hence favor the differential diagnosis of a malignant bone lesion.
This study has several limitations. First, the introduced reference values result from examinations using one specific scanner and imaging protocol. However, iodine concentration measurements have been proven robust between different DECT scanners of the latest generation, which supports generalizability of our findings (50–52). Concerning the warranted multi-vendor validation of our study, we assume our fully automated methodology excellently reproducible. The absence of specific user interaction allows for analysis of big data, which supports the warranted validation study. Although we evaluated a large-scale dataset of 678 examinations, patients at both extremes of the age spectrum are underrepresented. In particular the lack of younger patients is a frequent limitation of oncological research, since the age distribution of cancer prevalence is skewed towards the elderly (53, 54). Similar issues have been reported concerning the equity of racial and ethnic minorities in cancer research (55, 56). Hence, the ideal validation study of our data would comprise big data of multi-vendor, multi-center, multi-indication DECT with heterogenous age, sex, and ethnic distribution. To comply with the ethical standards, we did not perform DECT on healthy individuals without any medical history, but evaluated an oncological population. Albeit metastatic or recurrent disease was excluded, rare cases of undiagnosed malignancy cannot be ruled out. The same applies to possible systemic changes of physiology in oncological patients, e.g., blood pressure or kidney function, that might bias the bone marrow iodine uptake. Last, we did not include dual-energy X-ray absorptiometry (DEXA) as the gold standard to estimate the BMD. The vBMD was quantified in-body calibrated using a clinically approved vBMD tool, which is common practice in similar imaging studies and prevents for differences in BMD measurements between, e.g., the femur or radius at DEXA, compared to the spine in DECT (6, 24, 27, 57).
5 Conclusions
In conclusion, we present the first reference values of bone marrow iodine uptake in DECT, based on a large-scale cohort of 11,286 vertebrae in 678 examinations. Our multivariate analysis demonstrated that particularly the vBMD and the iodine blood pool affect the bone marrow iodine uptake, and hence should be adjusted for when adopting our results in clinical decision making. To achieve definite clinical evidence, a multi-center big-data study seems to be warranted by using the hereby provided AI-based workflow.
Data availability statement
The raw data supporting the conclusions of this article will be made available by the authors, without undue reservation.
Ethics statement
The studies involving human participants were reviewed and approved by Medizinische Fakultät der Universität zu Köln, Geschäftsstelle der Ethikkommission, Kerpener Str. 62, 50937 Köln. Written informed consent for participation was not required for this study in accordance with the national legislation and the institutional requirements.
Author contributions
PF: Conceptualization, data curation, formal analysis, investigation, methodology, validation, visualization, writing – original draft, writing – review and editing. FF: Conceptualization, data curation, investigation, methodology, software, writing – review and editing. MR: Formal analysis, resources, visualization, writing – review and editing. MW: Formal analysis, resources, writing – review and editing. JK: Formal analysis, Resources, writing – original draft, writing – review and editing. RR: Data curation, investigation, writing – review and editing. DZ: Data curation, investigation, visualization, writing – review and editing. EC: Formal analysis, investigation, writing – review and editing. DM: Formal analysis, project administration, supervision, validation, writing – review and editing. NG-H: Conceptualization, project administration, supervision, validation, writing – review and editing. TP: Conceptualization, funding acquisition, project administration, supervision, validation, writing – original draft, writing – review and editing. All authors contributed to the article and approved the submitted version.
Funding
This project was partially funded by the German Federal Ministry of Education and Research (BMBF) as part of the National Research Network of University Medicine (NUM), project: RACOON – COVID-19 under BMBF grant number 01KX2021, as well as part of the European Union’s response (REACT-EU) to the COVID-19 pandemic (#reacteu) project number RA-1-1-014. We acknowledge support for the Article Processing Charge from the DFG (German Research Foundation, 491454339). The funding did not influence the study’s design, data collection, analysis, interpretation of data, writing of the manuscript, or the decision to submit it to publication.
Conflict of interest
DZ and NG-H received research support from Philips Healthcare unrelated to this project. DM and NG-H are on the speakers bureau of Philips Healthcare. NH-H is a consultant for Bristol Myers Squibb. The other authors have no conflict of interest to declare.
The remaining authors declare that the research was conducted in the absence of any commercial or financial relationships that could be construed as a potential conflict of interest.
Publisher’s note
All claims expressed in this article are solely those of the authors and do not necessarily represent those of their affiliated organizations, or those of the publisher, the editors and the reviewers. Any product that may be evaluated in this article, or claim that may be made by its manufacturer, is not guaranteed or endorsed by the publisher.
Supplementary material
The Supplementary Material for this article can be found online at: https://www.frontiersin.org/articles/10.3389/fendo.2023.1098898/full#supplementary-material
References
1. Lugano R, Ramachandran M, Dimberg A. Tumor angiogenesis: causes, consequences, challenges and opportunities. Cell Mol Life Sci (2020) 77:1745. doi: 10.1007/S00018-019-03351-7
2. Zhang LJ, Wu S, Wang M, Lu L, Chen B, Jin L, et al. Quantitative dual energy CT measurements in rabbit VX2 liver tumors: comparison to perfusion CT measurements and histopathological findings. Eur J Radiol (2012) 81:1766–75. doi: 10.1016/J.EJRAD.2011.06.057
3. Pfeiffer D, Parakh A, Patino M, Kambadakone A, Rummeny EJ, Sahani DV. Iodine material density images in dual-energy CT: quantification of contrast uptake and washout in HCC. Abdom Radiol (New York) (2018) 43:3317–23. doi: 10.1007/S00261-018-1636-7
4. Ge X, Yu J, Wang Z, Xu Y, Pan C, Jiang L, et al. Comparative study of dual energy CT iodine imaging and standardized concentrations before and after chemoradiotherapy for esophageal cancer. BMC Cancer (2018) 18:1–7. doi: 10.1186/S12885-018-5058-2/FIGURES/1
5. Lennartz S, Le Blanc M, Zopfs D, Große Hokamp N, Abdullayev N, Laukamp KR, et al. Dual-energy CT-derived iodine maps: use in assessing pleural carcinomatosis. Radiology (2019) 290:796–804. doi: 10.1148/RADIOL.2018181567/ASSET/IMAGES/LARGE/RADIOL.2018181567.FIG6.JPEG
6. Borggrefe J, Neuhaus VF, Le Blanc M, Grosse Hokamp N, Maus V, Mpotsaris A, et al. Accuracy of iodine density thresholds for the separation of vertebral bone metastases from healthy-appearing trabecular bone in spectral detector computed tomography. Eur Radiol (2019) 29:3253–61. doi: 10.1007/S00330-018-5843-Y/FIGURES/6
7. Xu JJ, Taudorf M, Ulriksen PS, Achiam MP, Resch TA, Nielsen MB, et al. Gastrointestinal applications of iodine quantification using dual-energy CT: a systematic review. Diagnostics (2020) 10. doi: 10.3390/DIAGNOSTICS10100814
8. Wang X, Liu D, Zeng X, Jiang S, Li L, Yu T, et al. Dual-energy CT quantitative parameters for evaluating immunohistochemical biomarkers of invasive breast cancer. Cancer Imaging (2021) 21:1–10. doi: 10.1186/S40644-020-00370-7/TABLES/4
9. Yue X, Jiang Q, Hu X, Cen C, Song S, Qian K, et al. Quantitative dual-energy CT for evaluating hepatocellular carcinoma after transarterial chemoembolization. Sci Rep (2021) 11:1–10. doi: 10.1038/s41598-021-90508-9
10. Yan WQ, Xin YK, Jing Y, Li GF, Wang SM, Rong WC, et al. Iodine quantification using dual-energy computed tomography for differentiating thymic tumors. J Comput Assist Tomogr (2018) 42:873–80. doi: 10.1097/RCT.0000000000000800
11. Yang HL, Liu T, Wang XM, Xu Y, Deng SM. Diagnosis of bone metastases: a meta-analysis comparing 18FDG PET, CT, MRI and bone scintigraphy. Eur Radiol (2011) 21:2604–17. doi: 10.1007/S00330-011-2221-4/TABLES/5
12. Heindel W, Gübitz R, Vieth V, Weckesser M, Schober O, Schäfers M. The diagnostic imaging of bone metastases. Dtsch Arztebl Int (2014) 111:741–7. doi: 10.3238/ARZTEBL.2014.0741
13. Fervers P, Fervers F, Weisthoff M, Rinneburger M, Zopfs D, Reimer RP, et al. Virtual non-calcium bone marrow imaging of the spine: an AI-assisted, volumetric evaluation of a reference cohort with 500 CT scans. Diagnostics (2022) 12:671. doi: 10.3390/DIAGNOSTICS12030671
14. Gaudio F, Pedote P, Niccoli Asabella A, Ingravallo G, Sindaco P, Alberotanza V, et al. Bone involvement in hodgkin’s lymphoma: clinical features and outcome. Acta Haematol (2018) 140:178–82. doi: 10.1159/000490489
15. Soeharno H, Povegliano L, Choong PF. Multimodal treatment of bone metastasis - a surgical perspective. Front Endocrinol (Lausanne) (2018) 9:518/BIBTEX. doi: 10.3389/FENDO.2018.00518/BIBTEX
16. Kakhki VRD, Anvari K, Sadeghi R, Mahmoudian AS, Torabian-Kakhki M. Pattern and distribution of bone metastases in common malignant tumors. Nucl Med Rev Cent East Eur (2013) 16:66–9. doi: 10.5603/NMR.2013.0037
17. Mallinson PI, Coupal TM, McLaughlin PD, Nicolaou S, Munk PL, Ouellette HA. Dual-energy CT for the musculoskeletal system. Radiology (2016) 281:690–707. doi: 10.1148/RADIOL.2016151109/ASSET/IMAGES/LARGE/RADIOL.2016151109.FIG11B.JPEG
18. Rajiah P, Sundaram M, Subhas N. Dual-energy CT in musculoskeletal imaging: what is the role beyond gout? AJR Am J Roentgenol (2019) 213:493–505. doi: 10.2214/AJR.19.21095
19. Yuan Y, Lang N, Yuan H. Rapid-kilovoltage-switching dual-energy computed tomography (CT) for differentiating spinal osteolytic metastases from spinal infections. Quant Imaging Med Surg (2021) 11:620. doi: 10.21037/QIMS-20-334
20. Applications of dual energy CT in patients with osseous metastases from castrate-resistant prostate cancer - full text view - ClinicalTrials.gov. Available at: https://clinicaltrials.gov/ct2/show/NCT03111914 (Accessed April 7, 2022).
21. Lee YH, Kim S, Lim D, Suh JS, Song HT. Spectral parametric segmentation of contrast-enhanced dual-energy CT to detect bone metastasis: feasibility sensitivity study using whole-body bone scintigraphy. Acta Radiol (2015) 56:458–64. doi: 10.1177/0284185114530105
22. Hua C-HH, Shapira N, Merchant TE, Klahr P, Yagil Y. Accuracy of electron density, effective atomic number, and iodine concentration determination with a dual-layer dual-energy computed tomography system. Med Phys (2018) 45:2486–97. doi: 10.1002/mp.12903
23. Corrias G, Sawan P, Mahmood U, Zheng J, Capanu M, Salvatore M, et al. Dual energy computed tomography analysis in cancer patients: what factors affect iodine concentration in contrast enhanced studies? Eur J Radiol (2019) 120:108698. doi: 10.1016/J.EJRAD.2019.108698
24. Mueller DK, Kutscherenko A, Bartel H, Vlassenbroek A, Ourednicek P, Erckenbrecht J. Phantom-less QCT BMD system as screening tool for osteoporosis without additional radiation. Eur J Radiol (2011) 79:375–81. doi: 10.1016/j.ejrad.2010.02.008
25. Abdullayev N, Neuhaus VF, Bratke G, Voss S, Große Hokamp N, Hellmich M, et al. Effects of contrast enhancement on in-body calibrated phantomless bone mineral density measurements in computed tomography. J Clin Densitom: the Official Journal of the International Society for Clinical Densitometry (2018) 21:360–6. doi: 10.1016/J.JOCD.2017.10.001
26. Zopfs D, Lennartz S, Zaeske C, Merkt M, Laukamp KR, Reimer RP, et al. Phantomless assessment of volumetric bone mineral density using virtual non-contrast images from spectral detector computed tomography. (2020) (Accessed January 21, 2022).
27. Neuhaus V, Abdullayev N, Hellmich M, Krämer S, Maintz D, Krug B, et al. Association of quality and quantity of bone metastases and computed tomography volumetric bone mineral density with prevalence of vertebral fractures in breast cancer patients. Clin Breast Cancer (2016) 16:402–9. doi: 10.1016/j.clbc.2016.05.010
28. Manisali M, Özaksoy D, Kabakçi N. Quantitative computed tomography BMD reference values in women of izmir, Turkey. Clin Orthop Relat Res (2006) 443:109–12. doi: 10.1097/01.BLO.0000200240.07061.9D
29. Lennartz S, Abdullayev N, Zopfs D, Borggrefe J, Neuhaus VF, Persigehl T, et al. Intra-individual consistency of spectral detector CT-enabled iodine quantification of the vascular and renal blood pool. Eur Radiol (2019) 29:6581–90. doi: 10.1007/S00330-019-06266-W/FIGURES/6
30. Zopfs D, Reimer RP, Sonnabend K, Rinneburger M, Hentschke CM, Persigehl T, et al. Intraindividual consistency of iodine concentration in dual-energy computed tomography of the chest and abdomen. Invest Radiol (2021) 56:181–7. doi: 10.1097/RLI.0000000000000724
31. Sekuboyina A, Bayat A, Husseini ME, Löffler M, Li H, Tetteh G, et al. VerSe: a vertebrae labelling and segmentation benchmark for multi-detector CT images (2020). Elsevier. Available at: https://opus4.kobv.de/opus4-zib/frontdoor/index/index/docId/8090 (Accessed January 12, 2021).
32. Payer C, Štern D, Bischof H, Urschler M. Coarse to fine vertebrae localization and segmentation with SpatialConfiguration-net and U-net, in: Proceedings of the 15th International Joint Conference on Computer Vision, Imaging and Computer Graphics Theory and Applications (SCITEPRESS - Science and Technology Publications), Berlin: Springer (2020) pp. 124–33. doi: 10.5220/0008975201240133
33. Leaderboard - grand challenge. Available at: https://verse2020.grand-challenge.org/evaluation/challenge/leaderboard/ (Accessed January 11, 2022).
34. Virtanen P, Gommers R, Oliphant TE, Haberland M, Reddy T, Cournapeau D, et al. SciPy 1.0: fundamental algorithms for scientific computing in Python. Nat Methods (2020) 17:261–72. doi: 10.1038/s41592-019-0686-2
35. R Core Team. R: a language and environment for statistical computing. Vienna: R Found Stat Comput (2021).
36. A grammar of data manipulation [R package dplyr version 1.0.6] (2021). Available at: https://cran.r-project.org/package=dplyr (Accessed June 16, 2021).
37. John F, Sanford W. An R companion to applied regression, 2011. Los Angeles: SAGE Publications (2019).
38. Midi H, Sarkar SK, Rana S. Collinearity diagnostics of binary logistic regression model. J Interdiscip Math (2010) 13:253–67. doi: 10.1080/09720502.2010.10700699
39. QuantPsyc-package: quantitative psychology tools in QuantPsyc: quantitative psychology tools. Available at: https://rdrr.io/cran/QuantPsyc/man/QuantPsyc-package.html (Accessed June 10, 2022).
40. Faul F, Erdfelder E, Lang AG, Buchner A. G*Power 3: a flexible statistical power analysis program for the social, behavioral, and biomedical sciences. Behav Res Methods. (2007) 39:175–91. doi: 10.3758/BF03193146
41. Wickham H. ggplot2: elegant graphics for data analysis. Berlin: Springer (2016). doi: 10.1007/978-3-319-24277-4
42. Tan MT, Lloyd TB. Utility of dual energy computed tomography in the evaluation of infiltrative skeletal lesions and metastasis: a literature review. Skeletal Radiol (2022) 51:1731–41. doi: 10.1007/s00256-022-04032-6
43. Li B, Pomerleau M, Gupta A, Soto JA, Anderson SW. Accuracy of dual-energy CT virtual unenhanced and material-specific images: a phantom study. AJR Am J Roentgenol (2020) 215:1146–54. doi: 10.2214/AJR.19.22372
44. Stucker S, Chen J, Watt FE, Kusumbe AP. Bone angiogenesis and vascular niche remodeling in stress, aging, and diseases. Front Cell Dev Biol (2020) 8:602269/BIBTEX. doi: 10.3389/FCELL.2020.602269/BIBTEX
45. Kusumbe AP, Ramasamy SK, Itkin T, Mäe MA, Langen UH, Betsholtz C, et al. Age-dependent modulation of vascular niches for haematopoietic stemcells. Nature (2016) 532:380. doi: 10.1038/NATURE17638
46. Prisby RD, Ramsey MW, Behnke BJ, Dominguez JM, Donato AJ, Allen MR, et al. Aging reduces skeletal blood flow, endothelium-dependent vasodilation, and NO bioavailability in rats. J Bone Miner Res (2007) 22:1280–8. doi: 10.1359/JBMR.070415
47. Taveras EM, Rifas-Shiman SL, Sherry B, Oken E, Haines J, Kleinman K, et al. Crossing growth percentiles in infancy and risk of obesity in childhood. Arch Pediatr Adolesc Med (2011) 165:993–8. doi: 10.1001/ARCHPEDIATRICS.2011.167
48. Keller K, Sinning C, Schulz A, Jünger C, Schmitt VH, Hahad O, et al. Right atrium size in the general population. Sci Rep (2021) 11:1–11. doi: 10.1038/s41598-021-01968-y
49. Harrington DM, Staiano AE, Broyles ST, Gupta AK, Katzmarzyk PT. BMI percentiles for the identification of abdominal obesity and metabolic risk in children and adolescents: evidence in support of the CDC 95th percentile. Eur J Clin Nutr (2012) 67:218–22. doi: 10.1038/ejcn.2012.203
50. Pelgrim GJ, van Hamersvelt RW, Willemink MJ, Schmidt BT, Flohr T, Schilham A, et al. Accuracy of iodine quantification using dual energy CT in latest generation dual source and dual layer CT. Eur Radiol (2017) 27:3904–12. doi: 10.1007/s00330-017-4752-9
51. Jacobsen MC, Schellingerhout D, Wood CA, Tamm EP, Godoy MC, Sun J, et al. Intermanufacturer comparison of dual-energy CT iodine quantification and monochromatic attenuation: a phantom study. Radiology (2018) 287:224–34. doi: 10.1148/RADIOL.2017170896
52. Sauter AP, Kopp FK, Münzel D, Dangelmaier J, Renz M, Renger B, et al. Accuracy of iodine quantification in dual-layer spectral CT: influence of iterative reconstruction, patient habitus and tube parameters. Eur J Radiol (2018) 102:83–8. doi: 10.1016/J.EJRAD.2018.03.009
53. Adolescents and minority groups underrepresented in cancer clinical trials | ASH clinical news | American society of hematology. Available at: wwashpublications.org/ashclinicalnews/news/2663/Adolescents-and-Minority-Groups-Underrepresented (Accessed June 27, 2022).
54. Subbiah IM, Tang C, Rao A, Subbiah V, Hong DS. Expanding enrollment of underrepresented populations on early phase clinical trials: an analysis of participation among adolescent and young adults (AYA) with advanced cancers on phase I clinical trials. J Clin Oncol (2018) 36:. doi: 10.1200/JCO.2018.36.15_SUPPL.E18714
55. Behring M, Hale K, Ozaydin B, Grizzle WE, Sodeke SO, Manne U. Inclusiveness and ethical considerations for observational, translational, and clinical cancer health disparity research. Cancer (2019) 125:4452. doi: 10.1002/CNCR.32495
56. Brady DC, Weeraratna AT. The race toward equity: increasing racial diversity in cancer research and cancer care. Cancer Discov (2020) 10:1451–4. doi: 10.1158/2159-8290.CD-20-1193/333559/AM/THE-RACE-TOWARD-EQUITY-INCREASING-RACIAL-DIVERSITY
Keywords: bone marrow, iodine, reference values, tomography, X-Ray computed, artificial Intelligence
Citation: Fervers P, Fervers F, Rinneburger M, Weisthoff M, Kottlors J, Reimer R, Zopfs D, Celik E, Maintz D, Große-Hokamp N and Persigehl T (2023) Physiological iodine uptake of the spine’s bone marrow in dual-energy computed tomography – using artificial intelligence to define reference values based on 678 CT examinations of 189 individuals. Front. Endocrinol. 14:1098898. doi: 10.3389/fendo.2023.1098898
Received: 15 November 2022; Accepted: 09 May 2023;
Published: 19 May 2023.
Edited by:
Phil Salmon, Bruker microCT, BelgiumReviewed by:
Ali Ghasem-Zadeh, University of Melbourne, AustraliaChristopher Bangard, Klinikum Hanau GmbH, Germany
Copyright © 2023 Fervers, Fervers, Rinneburger, Weisthoff, Kottlors, Reimer, Zopfs, Celik, Maintz, Große-Hokamp and Persigehl. This is an open-access article distributed under the terms of the Creative Commons Attribution License (CC BY). The use, distribution or reproduction in other forums is permitted, provided the original author(s) and the copyright owner(s) are credited and that the original publication in this journal is cited, in accordance with accepted academic practice. No use, distribution or reproduction is permitted which does not comply with these terms.
*Correspondence: Philipp Fervers, Philipp.Fervers@uk-koeln.de