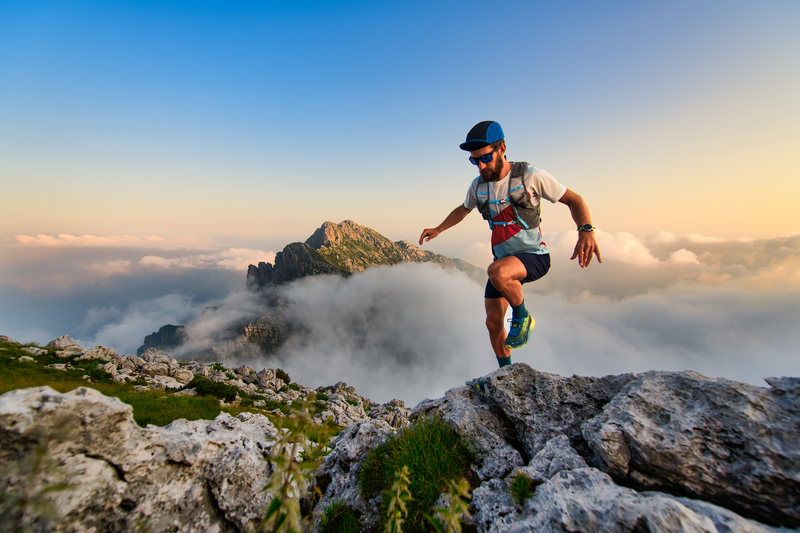
95% of researchers rate our articles as excellent or good
Learn more about the work of our research integrity team to safeguard the quality of each article we publish.
Find out more
SYSTEMATIC REVIEW article
Front. Endocrinol. , 04 May 2023
Sec. Clinical Diabetes
Volume 14 - 2023 | https://doi.org/10.3389/fendo.2023.1068254
This article is part of the Research Topic Application of Telehealth to Diabetes Care Delivery and Medical Training: Challenges and Opportunities View all 8 articles
The high disease burden of type 2 diabetes seriously affects the quality of life of patients, and with the deep integration of the Internet and healthcare, the application of electronic tools and information technology to has become a trend for disease management. The aim of this study was to evaluate the effectiveness of different forms and durations of E-health interventions in achieving glycemic control in type 2 diabetes patients. PubMed, Embase, Cochrane, and Clinical Trials.gov were searched for randomized controlled trials reporting different forms of E-health intervention for glycemic control in type 2 diabetes patients, including comprehensive measures (CM), smartphone applications (SA), phone calls (PC), short message service (SMS), websites (W), wearable devices (WD), and usual care. The inclusion criteria were as follows: (1) adults (age≥18) with type 2 diabetes mellitus; (2) intervention period ≥1 month; (3) outcome HbA1c (%); and (4) randomized control of E-health based approaches. Cochrane tools were used to assess the risk of bias. R 4.1.2 was used to conduct the Bayesian network meta-analysis. A total of 88 studies with 13,972 type 2 diabetes patients were included. Compared to the usual care group, the SMS-based intervention was superior in reducing HbA1c levels (mean difference (MD)-0.56, 95% confidence interval (CI): -0.82 to -0.31), followed by SA (MD-0.45, 95% CI: -0.61 to -0.30), CM (MD-0.41, 95% CI: -0.57 to -0.25), W (MD-0.39, 95% CI: -0.60 to -0.18) and PC (MD-0.32, 95% CI: -0.50 to -0.14) (p < 0.05). Subgroup analysis revealed that intervention durations of ≤6 months were most effective. All type of E-health based approaches can improve glycemic control in patients with type 2 diabetes. SMS is a high-frequency, low-barrier technology that achieves the best effect in lowering HbA1c, with ≤6 months being the optimal intervention duration.
Systematic review registration: https://www.crd.york.ac.uk/prospero, identifier CRD42022299896.
We compared previous randomized controlled E-health interventions for self-management behaviors in patients with type 2 diabetes to determine which forms of interventions and what durations were most effective in lowering HbA1c levels.
We found that SMS is a high frequency, low cost, low barrier technology that achieves the best effect in lowering HbA1c, with an optimal duration of ≤6 months.
Future studies should fully investigate the advantages of various forms and combinations of intervention methods to maximize the effectiveness of disease management.
The pervasiveness of unhealthy habits, including high-calorie intake and sedentary lifestyles, has increased the prevalence and lowered the age of onset of type 2 diabetes mellitus (1, 2). Research showed that the number of people with type 2 diabetes worldwide was approximately 462 million in 2017, with a prevalence of 6,059 cases per 100,000 (3). With the long course of the disease, symptoms such as thirst, frequent urination, and blurred vision continuously plague patients, resulting in increased negative emotions and difficulties (4). Cardiovascular disease is more likely to develop in patients with diabetes, and the risk rises as glycemic control deteriorates (5). Additionally, persons with diabetes are at a 2-4 times greater risk of death than adults without diabetes (6). Moreover, a study noted that 17.0% of type 2 diabetes patients reported moderate or severe depressive symptoms, and 10.6% suffered from major depressive disorder (7).
Glycemic control is the foundation of type 2 diabetes management; it can prevent cardiovascular disease-related and microvascular complications and impact patient survival (8). A Swedish study found that patients with HbA1c ≤6.9% (age under 55) had high risk of death , approximately two-fold higher than that in general population), while patients with HbA1c levels ≥9.7% (age under 55) had approximately four times higher risk (9). However, the glycemic control of type 2 diabetes patients is typically poor, and relevant studies have shown that fewer than 50% of type 2 diabetes patients have HbA1c below 7% (10).
Among the factors affecting glycemic control in patients, in addition to drug and insulin therapy, self-management (SM) is an essential aspect. The International Diabetes Federation emphasizes the need for patients' to manage their behavior, including blood glucose monitoring, medication compliance, diet control, and exercise management (11, 12). A meta-analysis revealed that SM behaviors such as physical exercise and regular blood glucose monitoring improve the quality of life of type 2 diabetes patients (13).
However, the SM behaviors of patients are generally poor, with one survey from China revealing that only 16.4% of such patients were able to maintain appropriate exercise and blood sugar monitoring, with most not meeting these standards (14). Ji M et al. showed that only 10% of 207 type 2 diabetes patients maintained an adequate diet and were more likely to have better glycemic control than others with a lower-quality diet (15). SM in patients with type 2 diabetes is long-term in nature and requires knowledge of the disease. Patients are often confronted with multiple obstacles, such as negative emotions associated with the long disease course, misconceptions about the disease, insufficient knowledge, financial burden, and work environment, that hinder the adoption of behaviors (16). For example, low medication adherence can affect the effectiveness of disease control because some patients believe that taking medications is addictive or refuse to take them because they consider doctors to be prescribed them for their own financial benefit; sedentary behavior and irregular working hours due to the nature of the occupation can also have an impact on the patient’s lifestyle, which in turn affects their disease management and glycemic control (17, 18).
The development of the internet and information technology has promoted innovation in disease management. E-Health was proposed at the beginning of the 21st century and has been widely used in diabetes management, including smartphone applications (SA), phone calls (PC), short message service (SMS) or tele-monitoring (19–25). Hansen demonstrated that type 2 diabetes patients who used E-health for disease management were more than twice as likely to make lifestyle changes than those using traditional methods (26). Numerous studies have confirmed the effectiveness of E-health interventions for glycemic control. A meta-analysis discovered that smartphone-based interventions for SM helped in reducinged HbA1c by approximately 0.51% compared to standard care; additionally, the researchers found that the shorter the duration of type 2 diabetes, the better the control (27). Tchero et al. found that telemedicine improved the treatment cooperation of patients and helped in controlling their glycemic status, reducing HbA1c by approximately 0.48% in patients with type 2 diabetes compared to the HbA1c levels of patients in standard care group (28).
Several recent studies have investigated the feasibility of using different types of E-health interventions, including PC, SMS, or SA, in the management of type 2 diabetes, but have not comprehensively explored which form of E-health intervention has a superior effect on glycemic control. Therefore, this study aims to use network meta-analysis to compare the differences and effectiveness of various types of E-health interventions in the disease management and glycemic control of patients.
This study with network meta-analysis (NMA) was reported following the PRISMA statement (29) and the PRISMA-NMA statement extension for NMA (30). The protocol was registered in the PROSPERO International Prospective Register of Systematic Reviews (No:CRD42022299896).
The aim of this study is to evaluate the effectiveness of different forms of E-health interventions for- glycemic control in type 2 diabetes patients. The PICO related to this aim was as follows.
(1) Population: adults (age≥18) with type 2 diabetes mellitus
(2) Interventions: different forms of E-health for type 2 diabetes management, including SA, PC, SMS, websites (W), wearable devices (WD) and their combinations: comprehensive measures (CM).
(3) Comparisons: usual care (UC), including regular examinations, follow-up visits, and doctor-advised treatment options.
(4) Outcome: HbA1c, which has been widely used in many studies to reflect glycemic control level and intervention effectiveness (31, 32).
We searched three core databases (PubMed, Embase and Cochrane) and a clinical trial registration database (Clinical Trials.gov) from database inception until May 2022. Our search query is shown in Supplementary Table 1; the search were performed in July 2022. All studies included in the search were in English.
The inclusion criteria were as follows: (1) adults (age≥18) with type 2 diabetes mellitus; (2) minimum follow-up duration of 1 month; (3) HbA1c (%) outcomes; and (4) randomized controlled trials (RCTs) of E-heath interventions.
Studies were excluded if (1) patients had serious complications or mental disorders; (2) patients were considered to have gestational diabetes or type 1 diabetes mellitus; (3) outcome values in the study were insufficient to perform NMA; (4) non-RCT studies, such as protocols, conference abstracts, or systematic reviews; and (5) the results of the study could not be found.
All the identified studies were exported to Endnote X9. Two researchers, with Master’s in Public Health and well-trained for NMA before the study, reviewed study titles and abstracts after removing duplicates and independently marked them for inclusion or exclusion. They downloaded and read the full text of all remaining articles for full-text screening and data collection. In this step, data included year and country of publication, authors, description of intervention and control, HbA1c, sociodemographic characteristics (gender, age, and race), sample size, intervention period, and follow-up duration.
In addition, to summarize key points about different forms of interventions, researchers collected and assessed details of interventions, including intervention strategies (behavior reminder, information feedback, health education course, blood glucose data monitoring, SM guidance and encouragement and emotional support), frequency of intervention (high, middle, low or unclear), health provider involvement (yes or no), interactivity (yes or no), and personalization (yes or no). Their criteria were as follows.
Intervention strategies: We analsis guiding and training methods to improve the behavior of patients through E-health interventions and define intervention strategies as behavior reminder, information feedback, health education, blood glucose monitoring, SM guidance and encouragement and emotional support. Behavior reminder—the reminders such as patient medication reminders and urging patients to exercise and maintain a healthy diet were considered in; information feedback—whether a patient could get feedback related to their own situation during the intervention, for example, receiving the blood glucose curve from the monitoring instruments, apps, or a message; health education—researchers used health education course, including video and live classes, to intervene in patient's behavior; blood glucose monitoring—patients can apply E-health devices to record their blood glucose data and can view their blood glucose values and changes; SM guidance—guidance regarding a reasonable diet, proper exercise, timely medication, and blood glucose monitoring; encouragement and emotional support—addressing the psychological obstacles faced by patients through companionship, talking to people, or using psychotherapeutic strategies to alleviate their negative emotions and enhance disease management.
Frequency: High frequency was defined as intervention more frequent than once a week, middle frequency - once a week to once a month, and low frequency – less than once a month.
Health provider involvement (Yes): If health care personnel (including doctors, nurses, nutritionists, etc.) participated in the intervention process. Whether an article showed health provider involvement was determined by our judgement.
Interactivity (Yes): Whether bidirectional informative feedback exists is the primary principle for judging interactivity. If the researchers and patients gave information in the form of E-health platforms and communicated with each other during the intervention, it can be defined as interactivity. The offline face-to-face instructions regarding the study, including instructions on device use before the start of the study or instructions from the physician during the routine treatment of patient’s, cannot be counted as interactivity, and we define interactivity primarily as the part conducted using E-health platforms.
Personalization (Yes): If the research team could provide individualized interventions or adjust the intervention strategies according to the patient’s conditions, we judged that the intervention described in the article was personalized.
With reference to the definition of E-health (19, 20) and previous studies that divided E-health interventions into five forms (33), we summarized the forms of E-health intervention into the following six categories: SA, PC, SMS, W, WD, and their combinations coded as comprehensive measures (CM). Their definitions in this study are as follows.
● W: interventions based on websites, which are widely used in computer-based interventions.
● PCs: interventions based on phone calls.
● SMSs: interventions based on short messages, which are basic functions of smartphones.
● WDs: interventions based on wearable devices that measure and upload biological data, including mobile blood glucose detectors, heart rate monitors, and pedometers.
● SA: interventions based on smartphone applications, including those involved in making voice or video calls, sending short messages or feedback, and collecting biological data.
● Interventions including two or more forms of E-health platforms were defined as CM.
W are interventions based on websites, which widely used in computer-based interventions. PC are interventions based on phone calls while SMS are interventions based on short messages, which are both basic functions of the smartphone. WD are interventions based on wearable devices that measure and upload biological data, including mobile blood glucose detectors, heart rate monitors, and pedometers. SA are interventions based on smartphone applications, including those involved in making voice or video calls, sending short messages or feedback, and collecting biological data. Interventions including two or more forms of E-health were defined as CM.
Using Cochrane Collaboration’s risk of bias tool (34), two authors independently assessed all studies for bias in (1) random sequence generation, (2) allocation concealment, (3) blinding of participants and personnel, (4) blinding of outcome assessment, (5) incomplete outcome data, (6) selective reporting and (7) other. Each bias was marked as low, unclear, or high. Both authors discussed any disagreements and consulted the third author if needed. Publication bias was evaluated using Egger’s test (35) and visualized in a funnel plot.
RevMan (Version 5.4. The Cochrane Collaboration, 2020) was used for data analysis in the assessment of bias, while Stata (StataCorp. 2015. Stata Statistical Software: Release 15. College Station, TX) was used to perform Egger’s test and sensitivity analysis and draw a funnel plot.
R 4.1.2 (R Foundation for Statistical Computing, Vienna, Austria Package: gemtc) was used to conduct the Bayesian random-effects NMA, which can evaluate multiple interventions even if they were not directly compared in the studies.
We used nodes to represent different forms of interventions and edges to represent comparisons between interventions in the reticulated relationship plot (drawn by Stata 15.0 to achieve a better effect). As the primary outcome is HbA1c (%), which is a continuous variable with a normal distribution, the results are presented as mean difference (MD) and standard deviation (SD) for HbA1c with a 95% confidence interval (CI) and shown in network forest plots, where in lower values indicate better treatment. In addition, the league table of E-health intervention effects was used to summarize the direct and indirect comparison results, while the E-health intervention effect ranks were evaluated by their distribution of ranking probability and the surface under the cumulative ranking curve (SUCRA).
Based on the Bayesian Markov chain Monte Carlo algorithm, the results of the random effect model were evaluated and processed a priori (four chains were used for simulation analysis; the initial value was 2, the iteration times were adjusted by 20000, and the simulation iteration times were 50000). Study heterogeneity was evaluated using Higgins I2 values, which were <25%, 25~50%, and >50%, indicating low, moderate, and high heterogeneity, respectively. The node split model was used for the inconsistency test. If there was no statistical difference among the studies in the subgroup, the heterogeneity of the included studies was considered small, and the consistency model was used for analysis; otherwise, the inconsistency model was used for analysis.
In addition, subgroup analysis was performed according to gender, age, follow-up duration, and race to explore the causes of heterogeneity and inconsistency and differences in the effectiveness of interventions among different studies. Based on gender, age, and race, the patients were divided into two groups each, depending on whether the male proportion was above 50%, age was above 60 years, and race was white, respectively.
Overall, 1880 and 190 records were identified from electronic databases and other sources, respectively. After duplicates were removed, the 1607 remaining records were screened based on the inclusion and exclusion criteria, among these 1165 records were excluded for the following reasons: other than type 2 diabetes (117), not RCT (165), not E-health, or not focusing on lifestyle modification (883); thus, full texts were sought for the remaining 442 records. Finally, 88 records remained after full-text screening. Figure 1 shows the process of data extraction.
A total of 88 studies with 13,972 type 2 diabetes patients were included. The study characteristics included country, author and publication year, intervention description, sample size, follow-up duration, age, and gender. These RCTs were conducted across 29 countries, with 17.0% from America, 15.9% from China, 10.2% from Korea, and 5.7% from Australia. The mean (SD) age of the patients was 57.6 (5.61) years; 55.1% were male, and 56.8% were white (Table 1). These studies were published between 2000 and 2022, and their follow-up period ranged from 1 to 24 months (Supplementary Table 2).
Assessment of bias was calculated using Cochrane Collaboration’s risk of bias tool (Figure 2). Most studies had a low risk of bias in random sequence generation (76, 86.4%), incomplete outcome data (84, 95.5%), selective reporting (86, 97.7%), and other biases (66, 75.0%). Several studies did not mention the methods for blinding; 33 (37.5%) studies had an unclear risk in both blinding of participants and personnel, while another 49 (55.7%) studies had an unclear risk only in the blinding of outcome assessments. Due to the interventions, it was difficult to implement the concealment and blinding of participants and personnel, which resulted in a high risk of bias (13.6% and 42.0%, respectively). Publication bias was judged using funnel plots, which were also plotted for each group of interventions and subjected to Egger tests with p values ranging from 0.062 to 0.876 (Supplementary Tables 3-8).
A total of 88 randomized controlled trials of glycemic control using E-health in patients with type 2 diabetes were included in this study. These trials were mainly designed to compare the effect of different forms of E-health interventions (including CM, PC, SA, SMS, W, and WD) with those of UC (Figure 3).
Figure 3 Network plots for HbA1c (%) control in type 2 diabetes patients based on E-health interventions. Legend: The size of the circle represents the number of patients receiving the interventions or control measure, and the width of the lines represents the number of studies. (A) is a network plot of six types of E-health interventions versus controls. (B) is a subset of comprehensive measures, which are a network plot of all intervention types and control groups included in the study.
PC-based intervention had the highest levels of health provider engagement, interactivity and personalization. Among all PC-based intervention trials, 88.2% trials showed health provider involvement, 94.1% trials demonstrated personalization, and all trials showed interactivity. CM, SMS and WD-based interventions performed better in terms of intervention frequency, and their constituent ratios of “high” frequency were 72.7%, 77.8% and 88.9%, respectively (Table 2).
According to SUCRA, all E-health interventions resulted in a better control of HbA1c than UC, SMS (SUCRA 67.2%) was ranked as having the highest probability of being the best, followed by SA (SUCRA 37.6%), CM (SUCRA 29.3%), W (SUCRA 25.5%), PC (SUCRA 45.1%) and WD (SUCRA 71.6%) (Table 2). Upon fitting a consistency model, the results showed that compared to UC, CM (MD: -0.41, 95% CI: -0.57 to -0.25), PC (MD: -0.32, 95% CI: -0.50 to -0.14), SA (MD: -0.45, 95% CI: -0.61 to -0.30), SMS (MD: - 0.56, 95% CI: -0.82 to -0.31) and W-based interventions (MD: - 0.39, 95% CI: -0.60 to -0.18) helped in reducing patients’ HbA1c levels (%) to a great extent, and the differences were statistically significant (p<0.05). SA (MD: -0.28, 95% CI: -0.55 to -0.01) and SMS (MD: -0.39, 95% CI: -0.73 to -0.04) were more effective in reducing HbA1c than WD (p<0.05) (Table 3). Supplementary Table 9 shows detailed results of both indirect and pairwise comparisons of diabetes management interventions in the network meta-analyses.
Table 3 The league table of a network meta-analysis of E-health intervention effects (mean difference MD and 95% confidence intervals).
SA was found to be more effective in females (MD -0.70, 95% CI -1.03 to -0.38) than in males (MD -0.35%, 95% CI -0.52 to -0.18), while SMS was found to be more effective in males (MD -0.68, 95% CI -1.00 to -0.35) (Figures 4A, B). The results showed that SMS had a better effect on HbA1c reduction in the patients with age ≥ 60 years (MD -0.87, 95% CI -1.39 to -0.32) than the patients under the age of 60 (MD -0.44, 95% CI -0.75 to -0.13) (Figures 4C, D). It was found that all interventions, except W and WD, were more effective when the duration was 6 months or shorter (MD -0.76 to -0.37) than when it was longer than 6 months (MD -0.35 to 0.26) (Figures 4E, F).
Figure 4 Subgroup analysis of HbA1c (%) in patients with type 2 diabetes in different types of E-health interventions.* mean P<0.05. (A) is the E-health intervention effects on the male in the gender subgroup; (B) is the E-health intervention effects on the female in the gender subgroup; (C) is the E-health intervention effects in patients < 60 years old in the age subgroup; (D) is E-health intervention effects in patients ≥ 60 years old in the age subgroup; (E) is E-health intervention effects in the subgroup of follow-up time ≤ 6 months; (F) is E-health intervention effects in the subgroup of follow-up time > 6 months.
Compared to UC alone, E-health platforms for disease management were more helpful in controlling, SMS was the most effective, followed by SA and CM. In addition, regardless of the form, patients were able to reduce HbA1c levels by 0.18% to 0.56%. E-health has advantages as a tool to guide patients for better disease management, as it provides information regarding glycemic control, dietary and exercise guidance, counseling services, and necessary knowledge on self-management (36). However, compared with traditional methods, such as face-to-face education, E-health has certain prerequisites related to the knowledge level of patient’s and their ability to use technology (37).
SMS and PC are less demanding with regard to the ability of patients to use the technology, PC is more interactive, and SMS is more frequent to convey information; both guide the behavior of patients through information reminders or encouragement, thereby enhancing the efficiency of their actions and motivating individuals (38, 39). One study noted that cueing the behavior of patients using PC had the greatest effect on medication adherence, with patients’ blood glucose levels already under control (at approximately 7%) at 3 months and remaining stable at 9 months (40). Dobler et al. found that the rate of exercise in type 2 diabetes patients increased by 26% through PC and improved their mental health (41).
While PC has highly interactive features, it requires patients to receive interventions at a specific time compared with SMS, which can be sent to patients at any time, can be sent frequently, and are less costly (42). The findings of Nelson et al. are similar to ours; patients preferred SMS to PC, and sending regular SMS can greatly improve their medication adherence (43). High-frequency communication can effectively remind patients to actively adopt SM behaviors, which was confirmed by Peimani et al., who showed that regular messaging achieved better results than personalized message content (44). Another meta-analysis found that SMS interventions helped in reducing the HbA1c levels of patients by 0.38% and that content personalization greatly influenced glycemic control outcomes; however, a high degree of personalization also means a large investment in professionals and technology (45). SMS-based patient behavior management is a cost-effective option that can play a positive role through education and prompting, but the effect of SMS will vary among different behaviors. Middleton and Waller et al. found that SMS alone was insufficient to change the dietary and exercise behaviors of patients but could benefit them in other ways, for example, by improving the frequency of subsequent visits (46, 47).
CM, SA, and W provide more integrated services, including monitoring, behavioral guidance, health education, and counseling, which can meet the different needs of patients (48–50). An integrated or combined form of intervention maximizes the ability to bridge the various limitations of E-health interventions and integrate resources, which also means that patients need to acquire sufficient skills to use the technology (51, 52). In contrast, SA is mainly carried out on mobile phones, which is easier for patients to accept (49). Wang et al. showed that after the SA intervention, patients had a significant increase in knowledge and a reduction in HbA1c levels from 8.62% to 7.12%, as well as a decrease in rehospitalization and medical expenditures (53). Hilmarsdóttir et al. found the blood glucose levels and symptoms such as anxiety and depression were effectively reduced in patients, but the app usage of patients gradually decreased as their blood glucose levels stabilized (54). Bowls et al. found that the participation of patients in app usage was difficult to guarantee, with only 9.9%-17.0% of patients participating; owing to a lack of personalized guidance and forgetfulness, HbA1c levels of the intervention group were only reduced by 0.10% after 6 months (55).
The content provided by the web-based interventions is similar to the use of SA. Vaughan et al. found that the HbA1c levels of 88.57% patients in the intervention group reduced by more than 0.50% through intervention (56). However, the website presents similar problems as SA; patients are reluctant to use them or discontinue use before the end of the study due to objective barriers, such as not being familiar with the use of phones, complexity, and a lack of health literacy (57). WD, such as flash glucose monitoring, is mainly used for continuous blood glucose and blood pressure monitoring. Patients adjust their SM behavior in response to the changes in blood glucose levels, which prevents adverse consequences caused by hypoglycemia (58). In addition, this approach is subject to high technical requirements, and adverse events associated with the device, such as redness and itching, can reduce patient use and the effect of intervention on glycemic control (59).
Using subgroup analysis, we discovered that females benefitted more from glycemic control through E-health interventions in general, and younger patients (age <60) benefitted more from complex interventions such as CM and SA. Previous studies have found that women are more concerned about their health and more willing to seek health information than are men; similarly, younger patients are more receptive to new things and technology, face fewer obstacles to technology and are more likely to learn and use e-technology for disease management (60).
Furthermore, the effect was stronger at an intervention duration of under 6 months than at that longer than 6 months, which was seen in several studies (61, 62). A study using SMS to provide patient interventions showed that patients had control of HbA1c in the short term, with a reduction of -0.24%; after 6 months (63). The effectiveness of disease management depends mainly on the extent to which patients participate; after using services for a while there is a decrease in motivation and willingness, which affects the subsequent outcome (64).
This study explored the differences in the effects of different forms of E-health interventions on glycemic control by direct and indirect comparison through Bayesian network meta-analysis. Compared to the frequency-based method in parameter estimation, which has the drawback of instability due to constant iterative estimation of the maximum likelihood function, the Bayesian method can reduce the risk of biased results and is more flexible (65). This study had a large sample size, analyzed the characteristics of several types of E-health, and summarized their personalization, interactivity, content, and frequency.
Some limitations of this study must be acknowledged. First, the RCTs included in this study were at high risk for blinding and group concealment; however, considering that communication between participants and personnel is part of many E-health interventions, bias around blinding may be inevitable compared with that in studies evaluating drug treatment effects. Second, funnel plots are not perfectly symmetrical and may exhibit publication bias. However, the trim and fill method and Egger test for each E-health group revealed that the model was robust and that this factor did not affect the results of the study. The asymmetry of funnel plots may be due to the low quality, small sample size, and heterogeneity of the included studies (66). Third, heterogeneity was found in this study (I2 = 76.0%). After meta-regression and subgroup analyses, gender (I2 = 49.7%) and race (I2 = 41.6%) could explain the source of heterogeneity. Classification of more than two interventions as CM may also be a reason for the high heterogeneity (67). Finally, although this study defined the intervention content, the frequency of intervention, health provider involvement, personalization, and interactivity were not included in the network analysis. Concurrently, because most of the included studies targeted interventions for comprehensive SM behaviors, this study did not explore the role of E-health in different behaviors. More research is needed in the future to explore the effects of different characteristics of e-health on glycemic control.
This study comprehensively evaluated the effects of six forms of E-health interventions in patients with type 2 diabetes. All forms have certain advantages in disease management; these include high service efficiency and more convenient provision of health information. However, they differ in service content, frequency, and persistence of effects. Future research should fully investigate the advantages of various forms and combinations of intervention methods to maximize the effectiveness of disease management to ensure scientific and professional health. While focusing on the extent of the impact of E-health, it is also essential to seek long-term management mechanisms that are sustainable.
The raw data supporting the conclusions of this article will be made available by the authors, without undue reservation.
XZ and LZ completed data extraction, data analysis, and thesis writing. YHL, YXL, and XCY participated in the mitigation of data extraction and analysis, helped extract specific content, and assisted in writing. WC assisted in the design of the study and assisted in the systematic review of the manuscript. YJ helped interpret the findings and reviewed and edited the manuscript. CC helped with the study design, assisted in the interpretation of the study results, and reviewed the whole process. Everyone has full access to all data in the study and is responsible for the integrity of the data and the accuracy of the data analysis. All authors contributed to the article and approved the submitted version.
This study was supported by Peking University.
We would like to thank Dr. Yuhui Shi from the School of Public Health; Peking University School provided constructive suggestions and help with the study design. Additionally, we would like to thank Editage (www.editage.cn) for English language editing.
The authors declare that the research was conducted in the absence of any commercial or financial relationships that could be construed as a potential conflict of interest.
All claims expressed in this article are solely those of the authors and do not necessarily represent those of their affiliated organizations, or those of the publisher, the editors and the reviewers. Any product that may be evaluated in this article, or claim that may be made by its manufacturer, is not guaranteed or endorsed by the publisher.
The Supplementary Material for this article can be found online at: https://www.frontiersin.org/articles/10.3389/fendo.2023.1068254/full#supplementary-material
1. Chatterjee S, Khunti K, Davies MJ. Type 2 diabetes. Lancet (2017) 389(10085):2239–51. doi: 10.1016/s0140-6736(17)30058-2
2. Yeung RO, Zhang Y, Luk A, Yang W, Sobrepena L, Yoon K-H, et al. Metabolic profiles and treatment gaps in young-onset type 2 diabetes in Asia (the JADE programme): a cross-sectional study of a prospective cohort. Lancet Diabetes Endocrinology (2014) 2(12):935–43. doi: 10.1016/s2213-8587(14)70137-8
3. Khan MAB, Hashim MJ, King JK, Govender RD, Mustafa H, Al Kaabi J. Epidemiology of type 2 diabetes - global burden of disease and forecasted trends. J Epidemiol Glob Health (2020) 10(1):107–11. doi: 10.2991/jegh.k.191028.001
4. Nanayakkara N, Pease A, Ranasinha S, Wischer N, Andrikopoulos S, Speight J, et al. Depression and diabetes distress in adults with type 2 diabetes: results from the Australian national diabetes audit (ANDA) 2016. Sci Rep (2018) 8(1):7846. doi: 10.1038/s41598-018-26138-5
5. C Emerging Risk Factors, Sarwar N, Gao P, Seshasai Sr, Gobin R, Kaptoge S, Angelantonio Di E, et al. Diabetes mellitus, fasting blood glucose concentration, and risk of vascular disease: a collaborative meta-analysis of 102 prospective studies. Lancet (2010) 375(9733):2215–22. doi: 10.1016/S0140-6736(10)60484-9
6. Dal Canto E, Ceriello A, Ryden L, Ferrini M, Hansen TB, Schnell O, et al. Diabetes as a cardiovascular risk factor: An overview of global trends of macro and micro vascular complications[J]. Eur J Prev Cardiol. doi: 10.1177/2047487319878371
7. Lloyd CE, Nouwen A, Sartorius N, Ahmed HU, Alvarez A, Bahendeka S, et al. Prevalence and correlates of depressive disorders in people with type 2 diabetes: results from the international prevalence and treatment of diabetes and depression (INTERPRET-DD) study, a collaborative study carried out in 14 countries. Diabetes Med (2018) 35(6):760–9. doi: 10.1111/dme.13611
8. Zheng Y, Ley SH, Hu FB. Global aetiology and epidemiology of type 2 diabetes mellitus and its complications. Nat Rev Endocrinol (2018) 14(2):88–98. doi: 10.1038/nrendo.2017.151
9. Tancredi M, Rosengren A, Svensson AM, Kosiborod M, Pivodic A, Gudbjornsdottir S, et al. Excess mortality among persons with type 2 diabetes. N Engl J Med (2015) 373(18):1720–32. doi: 10.1056/NEJMoa1504347
10. Davies MJ, D'Alessio DA, Fradkin J, Kernan WN, Mathieu C, Mingrone G, et al. Management of hyperglycemia in type 2 diabetes, 2018. a consensus report by the American diabetes association (ADA) and the European association for the study of diabetes (EASD). Diabetes Care (2018) 41(12):2669–701. doi: 10.2337/dci18-0033
11. Aschner P, Gagliardino JJ, Ilkova H, Lavalle F, Ramachandran A, Mbanya JC, et al. Persistent poor glycaemic control in individuals with type 2 diabetes in developing countries: 12 years of real-world evidence of the international diabetes management practices study (IDMPS). Diabetologia (2020) 63(4):711–21. doi: 10.1007/s00125-019-05078-3
12. Shrivastava SR, Shrivastava PS, Ramasamy J. Role of self-care in management of diabetes mellitus. J Diabetes Metab Disord (2013) 12(1):14–4. doi: 10.1186/2251-6581-12-14
13. Jing X, Chen J, Dong Y, Han D, Zhao H, Wang X, et al. Related factors of quality of life of type 2 diabetes patients: a systematic review and meta-analysis. Health Qual Life Outcomes (2018) 16(1):189. doi: 10.1186/s12955-018-1021-9
14. Ji M, Ren D, Gary-Webb TL, Dunbar-Jacob J, Erlen JA. Characterizing a sample of Chinese patients with type 2 diabetes and selected health outcomes. Diabetes Educ (2019) 45(1):105–15. doi: 10.1177/0145721718811561
15. Antonio JP, Sarmento RA, de Almeida JC. Diet quality and glycemic control in patients with type 2 diabetes. J Acad Nutr Diet (2019) 119(4):652–8. doi: 10.1016/j.jand.2018.11.006
16. Adu MD, Malabu UH, Malau-Aduli AEO, Malau-Aduli BS. Enablers and barriers to effective diabetes self-management: A multi-national investigation. PloS One (2019) 14(6):e0217771. doi: 10.1371/journal.pone.0217771
17. de Lusignan S, McGovern A, Hinton W, Whyte M, Munro N, Williams ED, et al. Barriers and facilitators to the initiation of injectable therapies for type 2 diabetes mellitus: A mixed methods study. Diabetes Ther (2022) 13(10):1789–809. doi: 10.1007/s13300-022-01306-z
18. Carlsson S, Andersson T, Talback M, Feychting M. Incidence and prevalence of type 2 diabetes by occupation: results from all Swedish employees[J]. Diabetologia (2020) 63(1):95–103. doi: 10.1007/s00125-019-04997-5
20. Oh H, Rizo C, Enkin M, Jadad A. What is eHealth (3): a systematic review of published definitions. J Med Internet Res (2005) 7(1):e1. doi: 10.2196/jmir.7.1.e1
21. Agarwal P, Mukerji G, Desveaux L, Ivers NM, Bhattacharyya O, Hensel JM, et al. Mobile app for improved self-management of type 2 diabetes: Multicenter pragmatic randomized controlled trial. JMIR Mhealth Uhealth (2019) 7(1):e10321. doi: 10.2196/10321
22. Shan R, Sarkar S, Martin SS. Digital health technology and mobile devices for the management of diabetes mellitus: state of the art. Diabetologia (2019) 62(6):877–87. doi: 10.1007/s00125-019-4864-7
23. Presley C, Agne A, Shelton T, Oster R, Cherrington A. Mobile-enhanced peer support for African americans with type 2 diabetes: a randomized controlled trial. J Gen Intern Med (2020) 35(10):2889–96. doi: 10.1007/s11606-020-06011-w
24. Lee JY, Chan CKY, Chua SS, Ng CJ, Paraidathathu T, Lee KKC, et al. Telemonitoring and team-based management of glycemic control on people with type 2 diabetes: a cluster-randomized controlled trial. J Gen Intern Med (2020) 35(1):87–94. doi: 10.1007/s11606-019-05316-9
25. NishanSiriwardena LSA, Sudarshana Wickramasinghe WA, Dussantha Perera KL, Marasinghe RB, Katulanda P, Hewapathirana R. A review of telemedicine interventions in diabetes care[J]. J Telemed Telecare (2012) 18(3):164–8. doi: 10.1258/jtt.2012.SFT110
26. Hansen AH, Wangberg SC, Arsand E. Lifestyle changes among people with type 2 diabetes are associated with participation in online groups and time since diagnosis. BMC Health Serv Res (2021) 21(1):688. doi: 10.1186/s12913-021-06660-5
27. Wu IXY, Kee JCY, Threapleton DE, Ma RCW, Lam VCK, Lee EKP, et al. Effectiveness of smartphone technologies on glycaemic control in patients with type 2 diabetes: systematic review with meta-analysis of 17 trials. Obes Rev (2018) 19(6):825–38. doi: 10.1111/obr.12669
28. Tchero H, Kangambega P, Briatte C, Brunet-Houdard S, Retali GR, Rusch E. Clinical effectiveness of telemedicine in diabetes mellitus: A meta-analysis of 42 randomized controlled trials. Telemed J E Health (2019) 25(7):569–83. doi: 10.1089/tmj.2018.0128
29. Moher D, Liberati A, Tetzlaff J, Altman DG, Group P. Preferred reporting items for systematic reviews and meta-analyses: the PRISMA statement. BMJ (2009) 339:b2535. doi: 10.1136/bmj.b2535
30. Hutton B, Salanti G, Caldwell DM, Chaimani A, Schmid CH, Cameron C, et al. The PRISMA extension statement for reporting of systematic reviews incorporating network meta-analyses of health care interventions: checklist and explanations. Ann Intern Med (2015) 162(11):777–84. doi: 10.7326/M14-2385
31. Yang J, Yang H, Wang Z, Wang X, Wang Y, Yu X, et al. Self-management among type 2 diabetes patients via the WeChat application: A systematic review and meta-analysis. J Clin Pharm Ther (2021) 46(1):4–16. doi: 10.1111/jcpt.13264
32. Tejedor M, Woldaregay AZ, Godtliebsen F. Reinforcement learning application in diabetes blood glucose control: A systematic review. Artif Intell Med (2020) 104:101836. doi: 10.1016/j.artmed.2020.101836
33. Seo HJ, Kim SY, Sheen SS, Cha Y. E-health interventions for community-dwelling type 2 diabetes: A scoping review. Telemed J E Health (2021) 27(3):276–85. doi: 10.1089/tmj.2019.0263
34. Sterne JAC, Savovic J, Page MJ, Wang X, Wang Y, Yu X, et al. RoB 2: a revised tool for assessing risk of bias in randomised trials. BMJ (2019) 366:l4898. doi: 10.1136/bmj.l4898
35. Egger M, Davey Smith G, Schneider M, Minder C. Bias in meta-analysis detected by a simple, graphical test. BMJ (1997) 315(7109):629–34. doi: 10.1136/bmj.315.7109.629
36. Joiner KL, Nam S, Whittemore R. Lifestyle interventions based on the diabetes prevention program delivered via eHealth: A systematic review and meta-analysis. Prev Med (2017) 100:194–207. doi: 10.1016/j.ypmed.2017.04.033
37. Hou C, Ben C, Jonathan H. Do mobile phone applications improve glycemic control (HbA1c) in the self-management of diabetes? a systematic review, meta-analysis, and GRADE of 14 randomized trials. Diabetes Care (2016) 39(11):2089–95. doi: 10.2337/dc16-0346/-/DC1
38. Piette JD, List J, Rana GK, Townsend W, Striplin D, Heisler M. Mobile health devices as tools for worldwide cardiovascular risk reduction and disease management. Circulation (2015) 132(21):2012–27. doi: 10.1161/circulationaha.114.008723
39. Huang L, Yan Z, Huang H. The effect of short message service intervention on glycemic control in diabetes: a systematic review and meta-analysis. Postgrad Med (2019) 131(8):566–71. doi: 10.1080/00325481.2019.1668723
40. Sarayani A, Mashayekhi M, Nosrati M, Jahangard-Rafsanjani Z, Javadi M, Saadat N, et al. Efficacy of a telephone-based intervention among patients with type-2 diabetes; a randomized controlled trial in pharmacy practice. Int J Clin Pharm (2018) 40(2):345–53. doi: 10.1007/s11096-018-0593-0
41. Dobler A, Herbeck Belnap B, Pollmann H, Farin E, Raspe H, Mittag O. Telephone-delivered lifestyle support with action planning and motivational interviewing techniques to improve rehabilitation outcomes. Rehabil Psychol (2018) 63(2):170–81. doi: 10.1037/rep0000224
42. Marcolino MS, Oliveira JAQ, D'Agostino M, Ribeiro AL, Alkmim MBM, Novillo-Ortiz D. The impact of mHealth interventions: Systematic review of systematic reviews. JMIR mHealth uHealth (2018) 6(1):e23. doi: 10.2196/mhealth.8873
43. Nelson LA, Mulvaney SA, Johnson KB, Osborn CY. mHealth intervention elements and user characteristics determine utility: A mixed-methods analysis. Diabetes Technol Ther (2017) 19(1):9–17. doi: 10.1089/dia.2016.0294
44. Peimani M, Rambod C, Omidvar M, Larijani B, Ghodssi-Ghassemabadi R, Tootee A, et al. Effectiveness of short message service-based intervention (SMS) on self-care in type 2 diabetes: A feasibility study. Prim Care Diabetes (2016) 10(4):251–8. doi: 10.1016/j.pcd.2015.11.001
45. Haider R, Sudini L, Chow CK, Cheung NW. Mobile phone text messaging in improving glycaemic control for patients with type 2 diabetes mellitus: A systematic review and meta-analysis. Diabetes Res Clin Pract (2019) 150:27–37. doi: 10.1016/j.diabres.2019.02.022
46. Middleton T, Constantino M, McGill M, D’Souza M, Twigg SM, Wu T, et al. An enhanced SMS text message-based support and reminder program for young adults with type 2 diabetes (TEXT2U): Randomized controlled trial. J Med Internet Res (2021) 23(10):e27263. doi: 10.2196/27263
47. Waller K, Furber S, Bauman A, Allman-Farinelli M, Dolder den van P, Hayes A, et al. Effectiveness and acceptability of a text message intervention (DTEXT) on HbA1c and self-management for people with type 2 diabetes. A randomized Controlled trial. Patient Educ Couns (2021) 104(7):1736–44. doi: 10.1016/j.pec.2020.11.038
48. Xu R, Xing M, Javaherian K, Peters R, Ross W, Bernal-Mizrachi C. Improving HbA1c with glucose self-monitoring in diabetic patients with EpxDiabetes, a phone call and text message-based telemedicine platform: A randomized controlled trial. Telemed J E Health (2020) 26(6):784–93. doi: 10.1089/tmj.2019.0035
49. Lin CL, Huang LC, Chang YT, Chen RY, Yang SH. Effectiveness of health coaching in diabetes control and lifestyle improvement: A randomized-controlled trial. Nutrients (2021) 13(11):3878. doi: 10.3390/nu13113878
50. Wang G, Zhang Z, Feng Y, Sun L, Xiao X, Wang G, et al. Telemedicine in the management of type 2 diabetes mellitus. Am J Med Sci (2017) 353(1):1–5. doi: 10.1016/j.amjms.2016.10.008
51. Agboola S, Jethwani K, Lopez L, Searl M, O'Keefe S, Kvedar J. Text to move: A randomized controlled trial of a text-messaging program to improve physical activity behaviors in patients with type 2 diabetes mellitus. J Med Internet Res (2016) 18(11):e307. doi: 10.2196/jmir.6439
52. Guo M, Meng F, Guo Q, Bai T, Hong Y, Song F, et al. Effectiveness of mHealth management with an implantable glucose sensor and a mobile application among Chinese adults with type 2 diabetes. J Telemed Telecare (2021) 1–9. doi: 10.1177/1357633X211020261
53. Wang Y, Li M, Zhao X, Pan X, Lu M, Lu J, et al. Effects of continuous care for patients with type 2 diabetes using mobile health application: A randomised controlled trial. Int J Health Plann Manage (2019) 34(3):1025–35. doi: 10.1002/hpm.2872
54. Hilmarsdottir E, Sigurethardottir AK, Arnardottir RH. A digital lifestyle program in outpatient treatment of type 2 diabetes: A randomized controlled study. J Diabetes Sci Technol (2021) 15(5):1134–41. doi: 10.1177/1932296820942286
55. Boels AM, Vos RC, Dijkhorst-Oei L-T, Rutten GEHM. Effectiveness of diabetes self-management education and support via a smartphone application in insulin-treated patients with type 2 diabetes: results of a randomized controlled trial (TRIGGER study). BMJ Open Diabetes Res Care (2019) 7(1):e000981. doi: 10.1136/bmjdrc-2019-000981
56. Vaughan EM, Hyman DJ, Naik AD, Samson SL, Razjouyan J, Foreyt JP. A telehealth-supported, integrated care with CHWs, and MEdication-access (TIME) program for diabetes improves HbA1c: a randomized clinical trial. J Gen Intern Med (2021) 36(2):455–63. doi: 10.1007/s11606-020-06017-4
57. Lindberg I, Torbjornsen A, Soderberg S, Ribu L. Telemonitoring and health counseling for self-management support of patients with type 2 diabetes: A randomized controlled trial. JMIR Diabetes (2017) 2(1):e10. doi: 10.2196/diabetes.6884
58. Haak T, Hanaire H, Ajjan R, Hermanns N, Riveline JP, Rayman G. Flash glucose-sensing technology as a replacement for blood glucose monitoring for the management of insulin-treated type 2 diabetes: a multicenter, open-label randomized controlled trial. Diabetes Ther (2017) 8(1):55–73. doi: 10.1007/s13300-016-0223-6
59. Diez-Fernandez A, Rodriguez-Huerta MD, Miron-Gonzalez R, Laredo-Aguilera JA, Martin-Espinosa NM. Flash glucose monitoring and patient satisfaction: A meta-review of systematic reviews. Int J Environ Res Public Health (2021) 18(6):3123. doi: 10.3390/ijerph18063123
60. Turnin MC, Gourdy P, Martini J, Buisson JC, Chauchard MC, Delaunay J, et al. Impact of a remote monitoring programme including lifestyle education software in type 2 diabetes: Results of the educ@dom randomised multicentre study. Diabetes Ther (2021) 12(7):2059–75. doi: 10.1007/s13300-021-01095-x
61. Kerfoot BP, Gagnon DR, McMahon GT, Orlander JD, Kurgansky KE, Conlin PR. A team-based online game improves blood glucose control in veterans with type 2 diabetes: A randomized controlled trial. Diabetes Care (2017) 40(9):1218–25. doi: 10.2337/dc17-0310
62. El-Gayar O, Ofori M, Nawar N. On the efficacy of behavior change techniques in mHealth for self-management of diabetes: A meta-analysis. J BioMed Inform (2021) 119:103839. doi: 10.1016/j.jbi.2021.103839
63. Ramallo-Farina Y, Garcia-Bello MA, Garcia-Perez L, Boronat M, Wagner AM, Rodriguez-Rodriguez L, et al. Effectiveness of Internet-based multicomponent interventions for patients and health care professionals to improve clinical outcomes in type 2 diabetes evaluated through the INDICA study: Multiarm cluster randomized controlled trial. JMIR Mhealth Uhealth (2020) 8(11):e18922. doi: 10.2196/18922
64. Ross J, Stevenson F, Lau R, Murray E. Factors that influence the implementation of e-health: a systematic review of systematic reviews (an update). Implement Sci (2016) 11(1):146. doi: 10.1186/s13012-016-0510-7
65. White IR, Barrett JK, Jackson D, Higgins JP. Consistency and inconsistency in network meta-analysis: model estimation using multivariate meta-regression. Res Synth Methods (2012) 3(2):111–25. doi: 10.1002/jrsm.1045
66. Duval S, Tweedie R. Trim and fill: A simple funnel-plot-based method of testing and adjusting for publication bias in meta-analysis. Biometrics (2000) 56(2):455–63. doi: 10.1111/j.0006-341X.2000.00455.x
Keywords: type 2 diabetes, E-health, glycemic control, Bayesian, network meta-analysis
Citation: Zhang X, Zhang L, Lin Y, Liu Y, Yang X, Cao W, Ji Y and Chang C (2023) Effects of E-health-based interventions on glycemic control for patients with type 2 diabetes: a Bayesian network meta-analysis. Front. Endocrinol. 14:1068254. doi: 10.3389/fendo.2023.1068254
Received: 12 October 2022; Accepted: 22 February 2023;
Published: 04 May 2023.
Edited by:
Giulio Romeo, Joslin Diabetes Center and Harvard Medical School, United StatesReviewed by:
Prateek Shukla, University of Massachusetts Medical School, United StatesCopyright © 2023 Zhang, Zhang, Lin, Liu, Yang, Cao, Ji and Chang. This is an open-access article distributed under the terms of the Creative Commons Attribution License (CC BY). The use, distribution or reproduction in other forums is permitted, provided the original author(s) and the copyright owner(s) are credited and that the original publication in this journal is cited, in accordance with accepted academic practice. No use, distribution or reproduction is permitted which does not comply with these terms.
*Correspondence: Chun Chang, Y2hhbmdjaHVuQGJqbXUuZWR1LmNu
†These authors have contributed equally to this work
Disclaimer: All claims expressed in this article are solely those of the authors and do not necessarily represent those of their affiliated organizations, or those of the publisher, the editors and the reviewers. Any product that may be evaluated in this article or claim that may be made by its manufacturer is not guaranteed or endorsed by the publisher.
Research integrity at Frontiers
Learn more about the work of our research integrity team to safeguard the quality of each article we publish.