- 1Burns Department, Shanghai Jiao Tong University Affiliated Ruijin Hospital, Shanghai, China
- 2Department of General Surgery, Putuo Hospital Shanghai University of Traditional Chinese Medicine, Shanghai, China
- 3Department of Anesthesiology, Shanghai Jiao Tong University Affiliated Sixth People's Hospital, Shanghai, China
- 4Department of Endocrine and Metabolic Diseases, Shanghai Institute of Endocrine and Metabolic Diseases, Ruijin Hospital, Shanghai Jiao Tong University School of Medicine, Shanghai, China
- 5Shanghai National Clinical Research Center for Metabolic Diseases, Key Laboratory for Endocrine and Metabolic Diseases of the National Health Commission of the People's Republic (PR) China, Shanghai, China
- 6Shanghai Key Laboratory for Endocrine Tumor, State Key Laboratory of Medical Genomics, Ruijin Hospital, Shanghai Jiao Tong University School of Medicine, Shanghai, China
Background: Diabetic peripheral neuropathy (DPN) is a serious complication in Diabetes Mellitus (DM) patients and the underlying mechanism is yet unclear. Ferroptosis has been recently intensively researched as a key process in the pathogenesis of diabetes but there yet has been no related bioinformatics-based studies in the context of DPN
Methods: We used data mining and data analysis techniques to screen differentially expressed genes (DEGs) and immune cell content in patients with DPN, DM patients and healthy participants (dataset GSE95849). These DEGs were then intersected with the ferroptosis dataset (FerrDb) to obtain ferroptosis DEGs and the associated key molecules and miRNAs interactions were predicted.
Results: A total of 33 ferroptosis DEGs were obtained. Functional pathway enrichment analysis revealed 127 significantly related biological processes, 10 cellular components, 3 molecular functions and 30 KEGG signal pathways. The biological processes that were significantly enriched were in response to extracellular stimulus and oxidative stress. Key modules constructed by the protein–protein interaction network analysis led to the confirmation of the following genes of interest: DCAF7, GABARAPL1, ACSL4, SESN2 and RB1. Further miRNA interaction prediction revealed the possible involvement of miRNAs such as miR108b-8p, miR34a-5p, mir15b-5p, miR-5838-5p, miR-192-5p, miR-222-3p and miR-23c. Immune-environment content of samples between DM and DPN patients revealed significant difference in the levels of endothelial cells and fibroblasts, which further speculates their possible involvement in the pathogenesis of DPN.
Conclusion: Our findings could provide insight for investigations about the role of ferroptosis in the development of DPN.
1 Introduction
Diabetic peripheral neuropathy (DPN) is one of the most common complication of patients with Diabetes Mellitus (DM) and is characterized by the presence of symptoms and/or signs of peripheral nerve dysfunction (1, 2). The mechanisms underlying the natural course of DPN are yet unclear but the recent resurgence of interest in the possible role abnormalities in programmed cell-death (PCD) signaling cascades has led to new leads in investigation (3, 4). Non-apoptotic regulated cell death has been reported in the pathogenesis of various neurological diseases and first defined in 2012, ferroptosis is characterized by iron-dependent accumulation of lipid peroxides which leads to oxidative stress and cell death and has been linked with the pathogenesis of DM complications, such as myocardial ischemia, epithelial dysfunction and nerve function degeneration (5, 6).
Recently, it was shown that due to the disorder of cellular metabolic pathways associated with iron metabolism, redox homeostasis and mitochondrial activity, Ferroptosis is involved in the evolution of DM complications (7–9). In a Streptozotocin (STZ)-induced mouse DM model in vivo, Wang et al. discovered significant changes in markers related to ferroptosis in DPN mice, such as ACSL4 which marks lipid peroxide accumulation and GPX4 which marks regulation of redox homeostasis (10). It is believed that the imbalance of redox homeostasis leads to the accumulation of reactive oxygen species (ROS) that promote ferroptosis (11, 12). Previous reports have speculated the possible association of the enhancement of the HIF-1α/HO-1 pathway, the regulation of the NRF2 downstream targets by HMGB1 and salusin-β, and the regulation of prfdx6 gene by Sp-1 gene with the induction of ferroptosis, eventually leading to the incidence of DNP (13–16). Nevertheless, the underlying complete molecular mechanism and pathophysiological process still require further study.
At present, there have been no bioinformatics-based studies on the mechanism of ferroptosis genes in the development of DPN. Therefore, we used data mining and data analysis techniques to screen differentially expressed genes (DEGs) in patients with DPN, DM patients and healthy participants. These DEGs were then intersected with the ferroptosis dataset to obtain ferroptosis DEGs. Moreover, to identify crucial biomarkers and establish the pathogenesis of DPN at the molecular level, we investigated key proteins and predicted possible miRNAs interactions that may play principal roles in DPN. Furthermore, by comparing the immune-microenvironment content of DM and DPN pathology samples, we investigated the possibility of target cells, which might prompt further prevention or treatment options. Our results will help to understand the role of ferroptosis in the incidence of DPN after and provide new thoughts for therapeutic interventions in the management of DM and its complications.
2 Materials and methods
2.1 Data source and microarray data
The clinical data of DPN patients, DM patients and healthy participants was obtained from NCBI Gene Expression Omnibus (GEO) (dataset GSE95849) (17). At the same time, the probe annotation information of the corresponding platform (PL22448 Phalanx Human lncRNA OneArray v1_mRNA) was downloaded, the probes were converted into gene symbols, and the probes that were not aligned to gene symbols were removed. These datasets were used for further analysis and mining. For multiple probes mapped to the same gene symbol, the average value of the probe was calculated as the expression level of the gene. See the attachment for the original data. Since the clinical data were obtained from public databases, so the consent of patients and ethics committee approval were unnecessary.
2.2 Differential expression analysis
To observe the molecular mechanism of disease occurrence, limma package Version3.10.3 in r3.6.1 language was used (http://www.bioconductor.org/packages/2.9/bioc/html/limma.html) (18). The linear regression and empirical Bayes methods provided were used to analyze the differential expression genes (DEGs) of DPN vs DM, and the corresponding P value and log2FC. In addition, the Benjamini & Hochberg method was used for multiple test correction to obtain the corrected p value, i.e. adjusted P-value. We evaluated it from the two levels of difference multiple and significance. The difference expression threshold was set as follows: a | log2 (fold-change) |>1 and adjusted P-value<0.05. Pheatmap in r3.6.1 (https://cran.r-project.org/web/packages/pheatmap/index.html) Version 1.0.8 (19) was used to show the expression of top100 DEGs (50 up and 50 down-regulated genes).
2.3 Identification of ferroptosis DEGs
We also obtained a dataset (FerrDb) that included 265 genes from the Ferroptosis Database (FerrDb; zhounan.org) and intersected it with GSE95849 to identify ferroptosis DEGs. The different iron death genes were obtained and used for subsequent analysis. The online tool Venny2.1 was employed to generate a Venn diagram of DEGs.
2.4 Functional enrichment analysis of ferroptosis DEGs
For the ferroptosis DEGs genes obtained in the previous step, the R package ‘clusterprofiler’ was used (http://bioconductor.org/packages/release/bioc/html/clusterProfiler.html, Version 4.0.5) (20). Kyoto Encyclopedia of Genes and Genomes (KEGG) analysis was obtained from the GSEA of WebGestalt. Gene ontology, referred to as GO, is an international standardized gene function classification system. It provides a set of dynamically updated standard vocabularies to comprehensively describe the properties of genes and gene products in organisms. GO function and KEGG pathway enrichment analysis were performed, focusing on the KEGG pathway analysis and KEGG enrichment analysis to find the pathways where the target gene is involved and analyze the significance of each pathway. The most significant TOP10 then obtained.
2.5 Ferroptosis DEGs protein–protein interaction network analysis
To predict protein–protein interactions (PPIs), STRING, (https://string-db.org/) an online database which can retrieve the interaction between a group of proteins, was utilized in the PPI network analysis. The PPI network was built and visualized by Galluvial package (version 0.12.3, https://CRAN.R-project.org/package=ggalluvial).
2.6 Prediction of miRNA-mRNA relationship
In addition to the abovementioned analytical tools, we also used the online database mirtarbase (http://mirtarbase.mbc.nctu.edu.tw/index.html) to predict targeted pivotal miRNAs and build the gene–miRNA interaction networks using Cytoscape v3.6.1 software (21).
2.7 Immune microenvironment analysis
For the analysis of the immune microenvironment, the following two algorithms are used to evaluate the immune microenvironment status of the samples, and Wilcoxon was used to test the difference of infiltration in DPN vs diabetes:
(1) MCPcounter is an absolute counting method. The R package MCPcounter (http://riskscore://github.com/ebecht/MCPcounter) (22) was used and based on the expression matrix of all mRNAs, the relative infiltration abundance of 10 immune cells in each sample was estimated.
(2) Using the ESTIMATE (23) algorithm, the stromal score and immune score of each sample were estimated from the expression data to represent the presence of stroma and immune cells.
3 Results
3.1 Acquisition of differentially expressed genes
Based on the expression matrix of geo data, limma package was used to analyze the differential expression between the comparison groups of DPN vs DM patients, and 1447 DEGs meeting the screening criteria were screened.
See Figures 1A Volcano map and 1B Heat map for details.
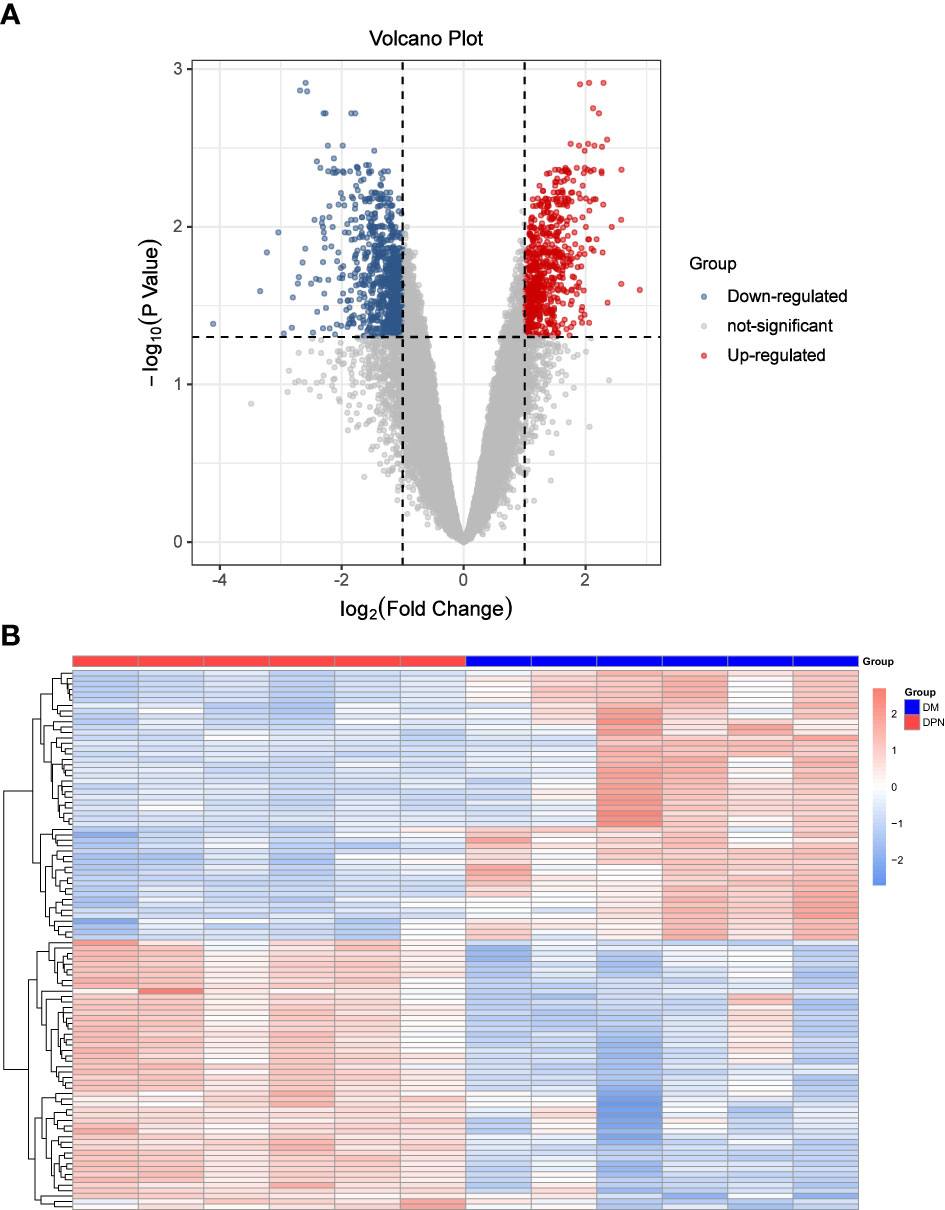
Figure 1 Differential Expression Analysis. (A) Volcano Plot: DEGs expression. (B) Heat map: Expression of top100 DEGs (50 up and 50 down-regulated genes).
3.2 Identification of ferroptosis DEGs
After the DEGs were obtained, they were intersected with 388 ferroptosis genes from the Ferroptosis Database (FerrDb): We found altogether 33 ferroptosis DEGs (Table 1), of which 14 genes were found to be up-regulated and the 19other down-regulated. The Venn diagram of the DEGs is shown in Figure 2.
3.3 Functional pathway enrichment analysis
GO function and KEGG signal pathway enrichment analyses were performed on the 33 ferroptosis DEGs obtained in the previous step to explore the functional term involved by key genes. Gene Ontology: the enrichment results are shown in Figure 3. With adjusted P-value < 0.05 and count > 2 as thresholds, 127 significantly related biological processes (BP) (Supplementary Table 1; Figure 3A), 10 cellular components (CC) (Table 2; Figure 3B), 3 molecular functions (MF) (Table 3; Figure 3C) and 30 KEGG signal pathways (Table 4; Figure 3D) were analyzed. The results showed that the biological processes that were significantly enriched were in response to extracellular stimulus.
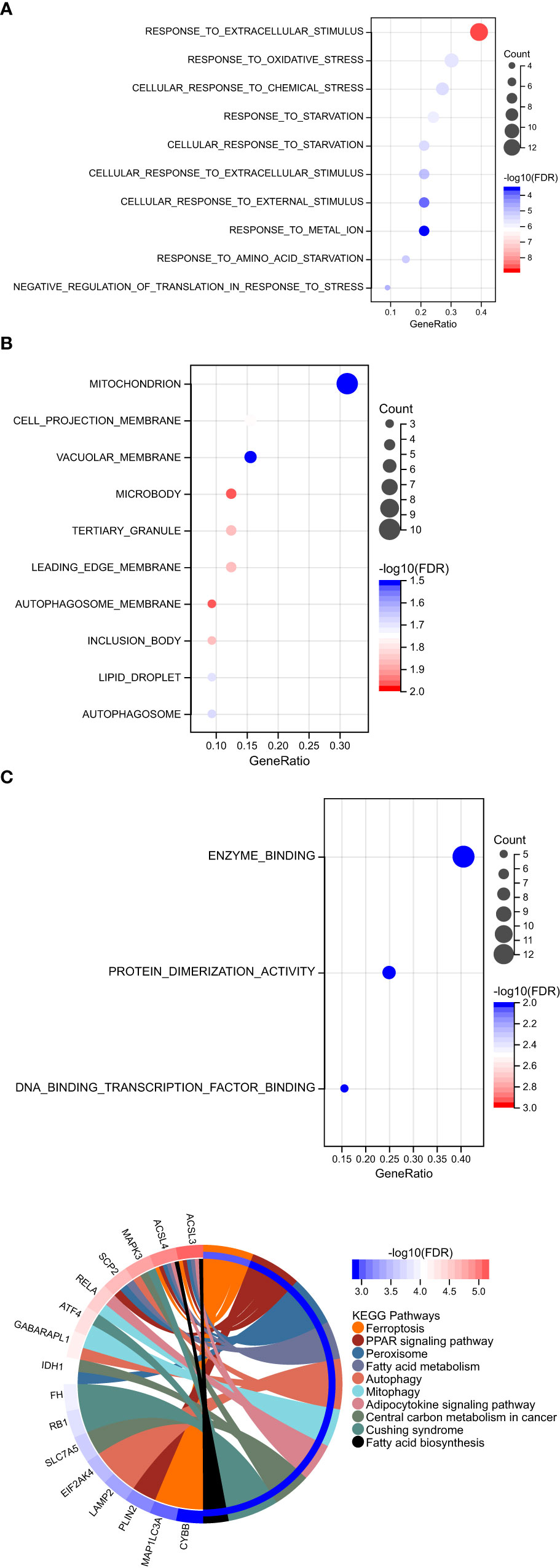
Figure 3 Functional pathway enrichment analysis. (A) 127 significantly related biological processes (BP) were involved. (B) 10 cellular components were involved. (C) 30 KEGG signal pathways were involved.
3.4 Protein–protein interaction network analysis of ferroptosis DEGs
In order to explore the association between the 33 differential ferroptosis genes, the protein interaction relationship among the 33 differential ferroptosis genes was analyzed by using the string database. The results predict that DCAF7, GABARAPL1, ACSL4, SESN2 and RB1 are key elements in the PPI network. The results are shown in Figure 4; Table 5.
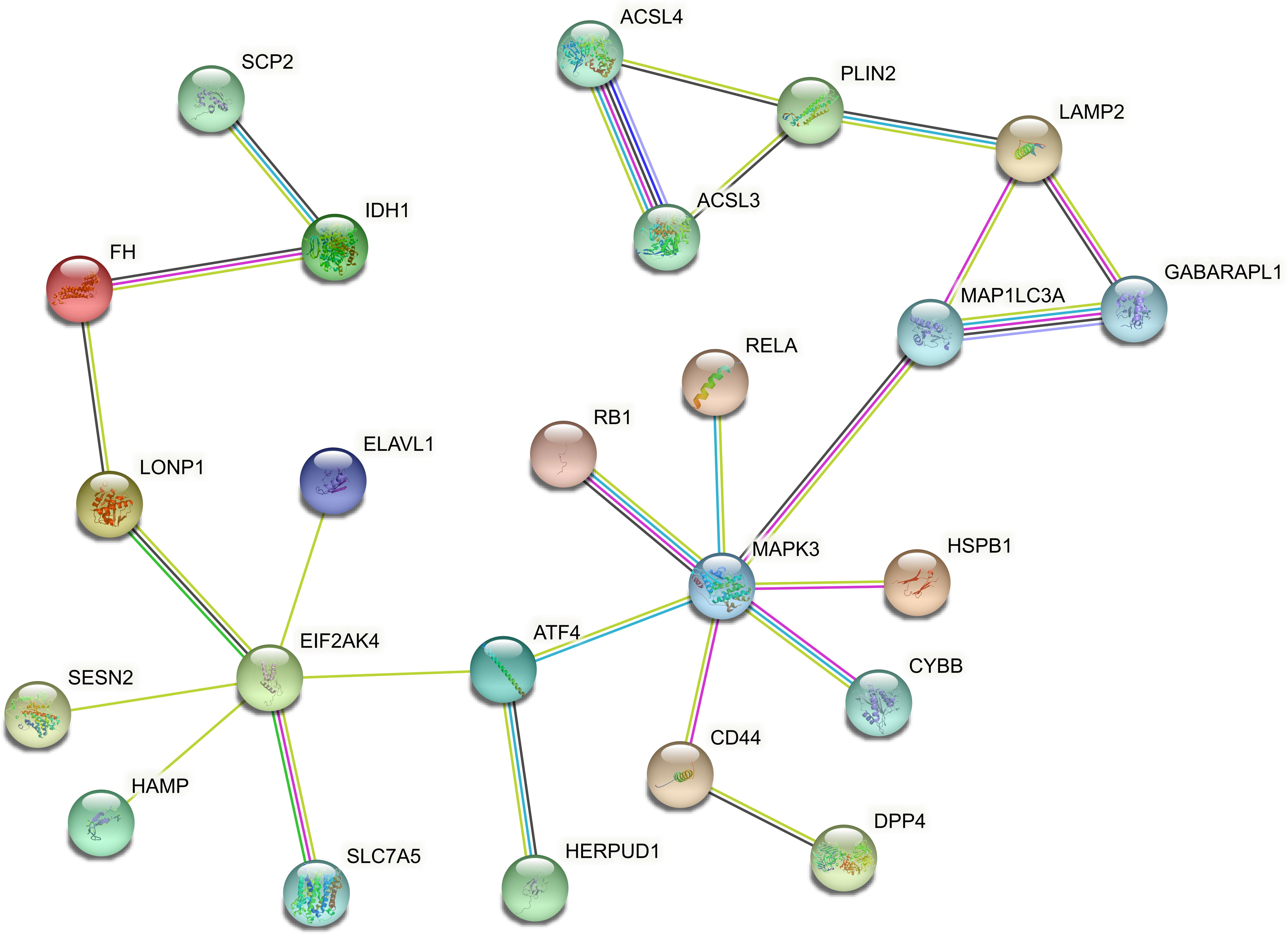
Figure 4 Protein–Protein Interaction Network Analysis of ferroptopsis DEGs: DCAF7, GABARAPL1, ACSL4, SESN2 and RB1 were found to be key elements in the PPI network.
3.5 Prediction of miRNA interaction
MiRNAs upstream of differential ferroptosis genes were obtained based on mirtarbase, and then the miRNA mRNA relationship pair network was constructed using Cytoscape, and analyzed using MiRwalk as shown in Table 6; Figure 5.
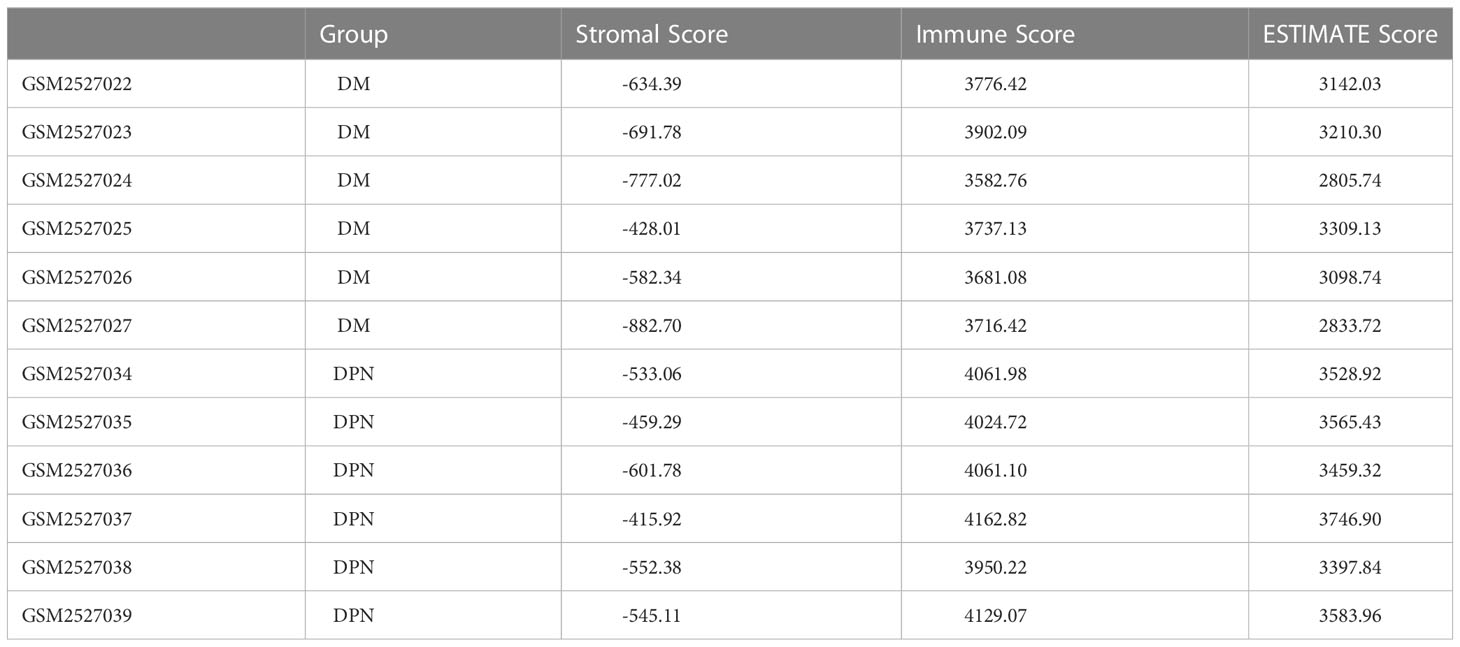
Table 6 Stromal score and immune score of the samples between the DM and DPN groups were estimated using the ESTIMATE algorithm.
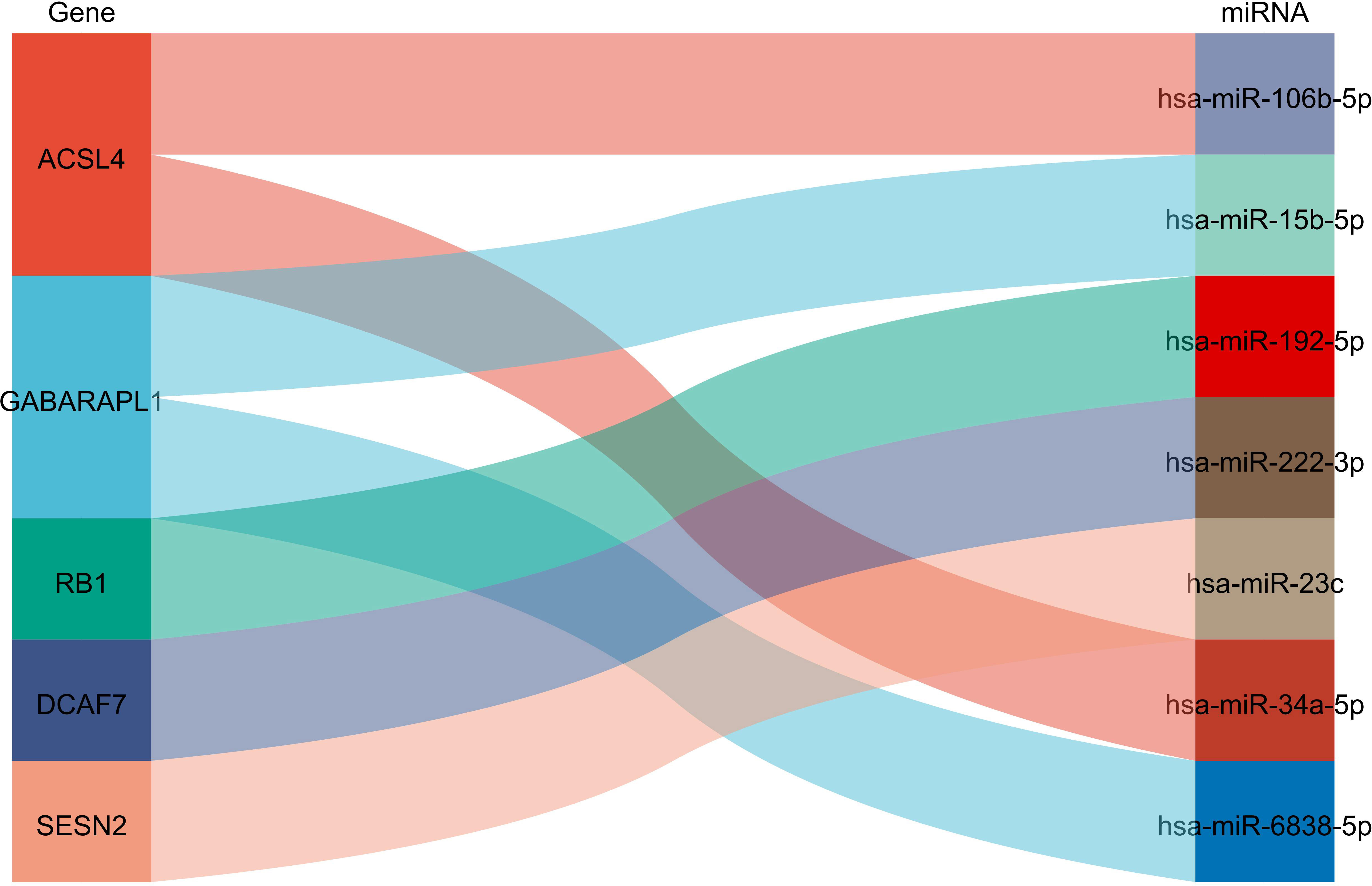
Figure 5 Prediction of miRNA interaction :ACSL4 could interact with miR108b-8p and miR34a-5p; GABARAPL1 could interact with mir15b-5p and miR-5838-5p, RB1 could interact with miR-192-5p, DCAF7 could interact with miR-222-3p while SESN2 could interact with miR-23c.
From Figure 5, it can be seen that ACSL4 can interact with miR108b-8p and miR34a-5p, GABARAPL1 interacts with mir15b-5p and miR-5838-5p, RB1 interacts with miR-192-5p, DCAF7 interacts with miR-222-3p, SESN2 interacts with miR-23c.
3.6 Immune microenvironment analysis
Based on the expression profile data of GEO samples, the MCPcounter algorithm was used to calculate the immune cell types of each sample, and a total of 10 immune cell types were obtained. See attached Table 4 for information. Then, we compared the difference of various immune cell ratios between DPN and DM. Taking P < 0.05 as the threshold, we obtained two kinds of differential immune cells (DICs) with significant differences: endothelial cells and fibroblasts. The comparison results between groups are shown in Figure 6A. The immune score and matrix score were calculated based on the estimate algorithm. The difference of immune score and matrix score in the infiltration level between DPN and DM was then analyzed. The results are shown in Figure 6B (1-3), Table 6.
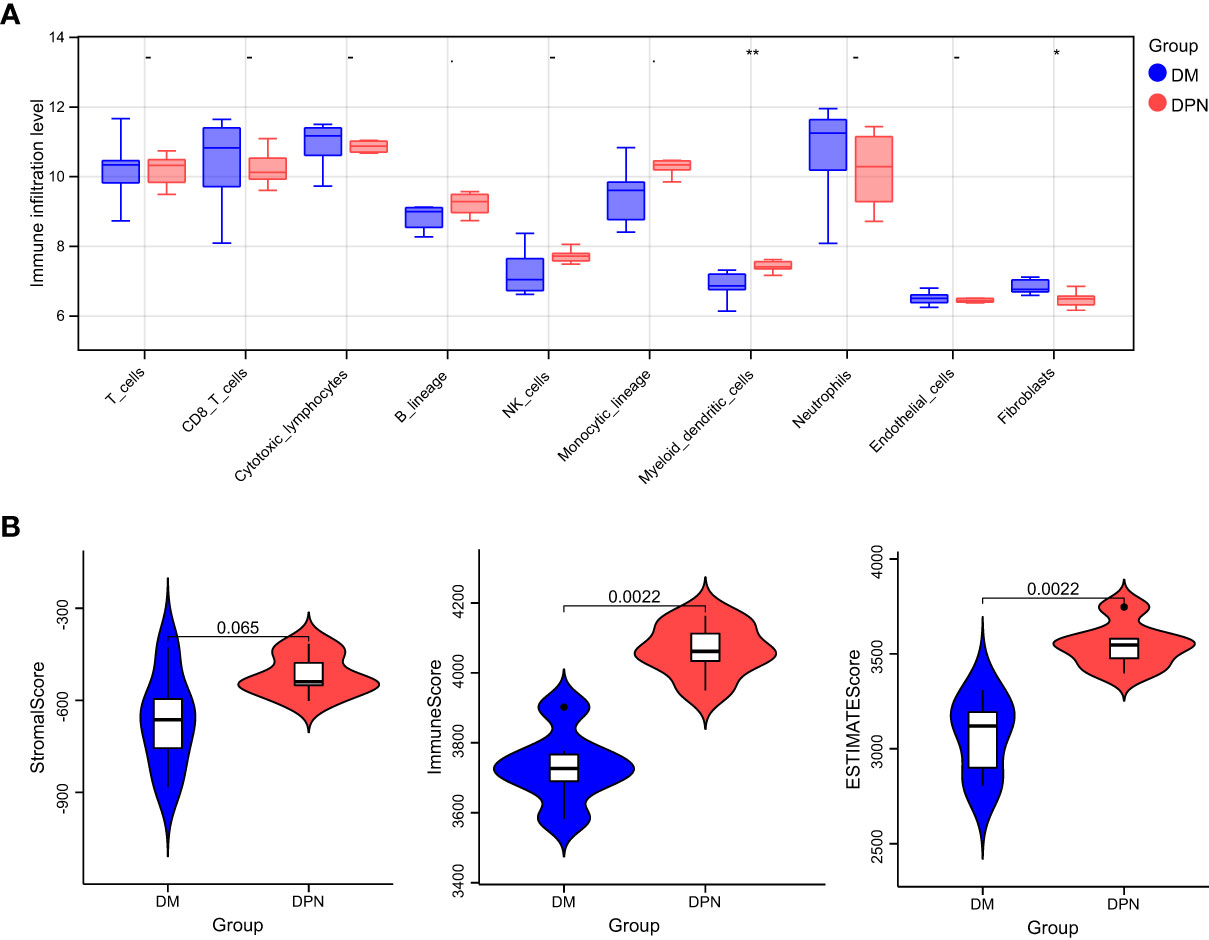
Figure 6 Immune microenvironment analysis. (A) Comparison of the various immune cell ratios between DPN and DM subjects. (B) Comparison of the immune score and matrix score in the infiltration level between the DPN and DM subjects.
4 Discussion
The complications associated with DM, such as DPN, have serious effects on the standard of life and socioeconomic welfare of patients (1). Henceforth, recent researches have been focusing on deciphering the underlying mechanism leading to the disease progression from DM to complication with DPN: In a study by Dewanjee et al., the implication of different molecular ad signaling pathways, oxidative stress, interleukins, nerve growth factor, and autophagy was thoroughly assessed in the investigation of the pathogenesis and progression of diabetic neuropathy (24). Along the same line of thought, we investigated the possible implication of ferroptosis in the pathogenesis and possible treatment of DPN. In this study, we identified the key genes involved in ferroptosis and further explored the mechanisms of ferroptosis in the pathogenesis of DPN. From the intersection of DEGs of the datasets GSE95849 and FerrDb, our study obtained 33 DEGs, including 19 downregulated genes (TFAP2C, HAMP, NNMT, MT3, HERPUD1, GRIA3, HIC1, MAP1LC3A, ATF4, HSPB1, RELA, BRD2, GABARAPL1, MAPK3, PLIN2, SLC7A5, YY1AP1, SESN2, LONP1) and 14 upregulated genes (DCAF7, RB1, ELAVL1, ANO6, SCP2, CYBB, CD44, LAMP2, ACSL4, ACSL3, IDH1, FH, EIF2AK4 and DPP4). Then, GO function and KEGG signal pathway enrichment analyses revealed that the 33 DEGs were involved in 27 biological processes, 10 cellular components, 3 molecular functions and 30 KEGG signal pathways. The genes were found to be mainly involved in response to extracellular stimulus and oxidative stress and related to ferroptosis signaling pathway. Further PPI network analysis predicted that DCAF7, GABARAPL1, ACSL4, SESN2 and RB1could be the key proteins while MirWalk predicted that miR108b-8p, miR34a-5p, mir15b-5p, miR-5838-5p, miR-192-5p, miR-222-3p and miR-23c could be mapped MiRNAs in the pathogenesis of DPN.
From 2012 till now, there have been only few studies that have highlighted the role of ferroptosis in the development of DPN: For instance, in a study by Feng et al., it was revealed that ferroptosis along the HIF-1α/HO-1 pathway lead to renal tubular injury and fibrosis in DM mice, partly also due to the increased production of ROS and accumulation of lipid peroxidation (13). In further studies related to the accumulation of lipid peroxidation revealed another key element, ACSL4, a key marker of ferroptosis and lipid peroxidation accumulation, which when down-regulated resulted in the inhibition of ferroptosis, finally improving the damage of renal tubular cells (25). Nevertheless, the role of ACSL4 in the mechanism of DPN has not been previously investigated. In a study by Cui et al, it was seen that ACSL4 promoted neuronal death via enhancing lipid peroxidation, identifying ACSL4 as a marker of ferroptosis, novel regulator of neuronal death and neuro-inflammation (26). Another marker of ferroptosis, SESN2 has been shown to play pivotal role in oxidative stress: In a study by Zhang et al., SESN2 was shown to influence oxidative stress of neurons by decreasing the expression of Nrf2 (27). Ferroptosis is characterized by the intracellular collection of lipid ROS, which are closely related and eventually result in the oxidation of lipids, thereby leading to cell membrane injury and cell death, which partly sustains the activity of ACSL4 and SESN2 (27, 28). Nevertheless, in this study, the bioinformatics analysis of the database revealed the possible involvement of 3 other targets which were not reported before: DCAF7, GABARAPL1 and RB1. After reviewing literature, we found that these genes have been related to ferroptosis in the mechanistic analysis of tumor development but they have not yet been mentioned in the context of DPN. These novel genes provide new concepts in the role ferroptosis in DPN.
When investigating the underlying pathways and mechanisms that could lead to the development of DPN in DM patients, we found that the differential genes were mainly involved in response to extracellular stimulus and oxidative stress mapped on the ferroptosis signaling pathway (13–16). The imbalance between the production of reactive oxygen species and their removal by antioxidant mechanisms over time can lead to tissue and organ damage (29). Henceforth we can speculate the possibility of ROS regulated ferroptosis in the mechanism behind the development of DPN.
Nevertheless, there is currently still a lack of understanding regarding potential biomarkers that can be used to specifically identify ferroptosis in clinical settings. Although proteins related to iron metabolism, such as ferritin, are elevated in the serum of patients with diabetes and related complications, their specificity cannot meet the requirements of clinical diagnosis. While investigating the immune-environment content of samples between DM and DPN patients, we found significant difference in the levels of endothelial cells and fibroblasts, which further speculates their possible involvement in the complication progression of DM. In a study by Li et al., it was found that exposure to hyperglycemic conditions induced excessive oxidative stress responses by fibroblasts and vascular endothelial cells, leading to lipid peroxidation and ferroptosis, a finding very similar to ours (30). In their study, Li et al. discovered that the inhibition of ferroptosis by Fer-1 reduced inflammation and promoted cell migration and proliferation, leading to accelerated wound healing.
The implication of ferroptosis in the pathogenesis of DPN is still work in progress. Henceforth, some of the limitations of our study are that by comparing established patient datasets, we obtained some theoretical targets marking the possible underlying mechanism defining the role of ferroptosis in DPN. Even though our results can provide insight for further in vivo and in vitro experiments to further decipher the role of ferroptosis in DPN, further validation and experiments are required to evaluate the findings of the study with further investigations about the therapeutic implications of the DEGs in the management of peripheral neuropathy in diabetic patients.
5 Conclusion
DPN is a serious complication in diabetic patient and the underlying mechanism is yet unclear. Ferroptosis has been recently intensively researched as a key process in the pathogenesis of diabetes. Henceforth, by means of bioinformatics analysis, 33 differential genes were first screened out. Functional pathway enrichment analysis revealed 127 significantly related biological processes, 10 cellular components, 3 molecular functions and 30 KEGG signal pathways. The biological processes that were significantly enriched were in response to extracellular stimulus. Key modules constructed by the protein–protein interaction network analysis and screening of genes related to the ferroptosis signaling pathway led to the confirmation of the following genes of interest: DCAF7, GABARAPL1, ACSL4, SESN2 and RB1. Further miRNA interaction prediction revealed the possible involvement of miRNAs such as miR108b-8p and miR34a-5p, mir15b-5p and miR-5838-5p, miR-192-5p, miR-222-3p, and miR-23c. While further investigating the immune-environment content of samples between DM and DPN patients, we found significant difference in the levels of endothelial cells and fibroblasts, which further speculates their possible involvement in the complication progression of DM.
Data availability statement
The original contributions presented in the study are included in the article/Supplementary Material. Further inquiries can be directed to the corresponding author.
Ethics statement
Ethical review and approval was not required for the study on human participants in accordance with the local legislation and institutional requirements. Written informed consent for participation was not required for this study in accordance with the national legislation and the institutional requirements.
Author contributions
WZ is the corresponding author. WZ has designed the concept of the study and supervised the progress of the study. MT and YZ are co-first authors. MT and YC developed the concept and performed the numerical simulations. MT, FP and YC made the figures. MT, YC and AW wrote the paper. AW, HJ, RH and WZ supervised the project. All authors contributed to the article and approved the submitted version.
Funding
This study has been supported by the following funds: (1) Pudong New Area Science and Technology Development Fund (PKJ2020-Y10) (2) Shanghai Sixth People's Hospital Scientific research Fund (YNXG202212) (3) National Natural Science Foundation of China No.81670752 (4) Putuo District Clinical Characteristic Special Disease Construction Project- Lower Limbs Arteriosclerosis obliterans (2020TZSB01).
Conflict of interest
The authors declare that the research was conducted in the absence of any commercial or financial relationships that could be construed as a potential conflict of interest.
Publisher’s note
All claims expressed in this article are solely those of the authors and do not necessarily represent those of their affiliated organizations, or those of the publisher, the editors and the reviewers. Any product that may be evaluated in this article, or claim that may be made by its manufacturer, is not guaranteed or endorsed by the publisher.
Supplementary material
The Supplementary Material for this article can be found online at: https://www.frontiersin.org/articles/10.3389/fendo.2023.1048856/full#supplementary-material
References
1. Selvarajah D, Kar D, Khunti K, Davies MJ, Scott AR, Walker J, et al. Diabetic peripheral neuropathy: advances in diagnosis and strategies for screening and early intervention. Lancet Diabetes Endocrinol (2019) 7(12):938–48. doi: 10.1016/S2213-8587(19)30081-6
2. Carmichael J, Fadavi H, Ishibashi F, Shore AC, Tavakoli M. Advances in screening, early diagnosis and accurate staging of diabetic neuropathy. Front Endocrinol (Lausanne). (2021) 12:671257. doi: 10.3389/fendo.2021.671257
3. Yang XD, Yang YY. Ferroptosis as a novel therapeutic target for diabetes and its complications. Front Endocrinol (Lausanne). (2022) 13:853822. doi: 10.3389/fendo.2022.853822
4. Xie Y, Hou W, Song X, Yu Y, Huang J, Sun X, et al. Ferroptosis: process and function. Cell Death Differ (2016) 23:369–79. doi: 10.1038/cdd.2015.158
5. Li W, Li W, Leng Y, Xiong Y, Xia Z. Ferroptosis is involved in diabetes myocardial Ischemia/Reperfusion injury through endoplasmic reticulum stress. DNA Cell Biol (2020) 39(2):210–25. doi: 10.1089/dna.2019.5097
6. He J, Li Z, Xia P, Shi A, FuChen X, Zhang J, et al. Ferroptosis and ferritinophagy in diabetes complications. Mol Metab (2022) 60:101470. doi: 10.1016/j.molmet.2022.101470
7. Sha W, Hu F, Xi Y, Chu Y, Bu S. Mechanism of ferroptosis and its role in type 2 diabetes mellitus. J Diabetes Res (2021) 2021:9999612. doi: 10.1155/2021/9999612
8. Duan JY, Lin X, Xu F, Shan SK, Guo B, Li FX, et al. Ferroptosis and its potential role in metabolic diseases: a curse or revitalization? Front Cell Dev Biol (2021) 9:701788. doi: 10.3389/fcell.2021.701788
9. Otasevic V, Vucetic M, Grigorov I, Martinovic V, Stancic A. Ferroptosis in different pathological contexts seen through the eyes of mitochondria. Oxid Med Cell Longev (2021) 2021:5537330. doi: 10.1155/2021/5537330
10. Wang Y, Bi R, Quan F, Cao Q, Lin Y, Yue C, et al. Ferroptosis involves in renal tubular cell death in diabetic nephropathy. Eur J Pharmacol (2020) 888:173574. doi: 10.1016/j.ejphar.2020.173574
11. Wu H, Wang F, Ta N, Zhang T, Gao W. The multifaceted regulation of mitochondria in ferroptosis. Life (Basel). (2021) 11(3):222. doi: 10.3390/life11030222
12. Lei P, Bai T, Sun Y. Mechanisms of ferroptosis and relations with regulated cell death: a review. Front Physiol (2019) 10:139. doi: 10.3389/fphys.2019.00139
13. Feng X, Wang S, Sun Z, Dong H, Yu H, Huang M, et al. Ferroptosis enhanced diabetic renal tubular injury via HIF-1α/HO-1 pathway in db/db mice. Front Endocrinol. (2021) 12:626390. doi: 10.3389/fendo.2021.626390
14. Wu Y, Zhao Y, Yang HZ, Wang YJ, Chen Y. HMGB1 regulates ferroptosis through Nrf2 pathway in mesangial cells in response to high glucose. Bioscience Rep (2021) 41(2). doi: 10.1042/BSR20202924
15. Wang WJ, Jiang X, Gao CC, Chen ZW. Salusin-β participates in high glucose-induced HK-2 cell ferroptosis in a nrf-2-dependent manner. Mol Med Rep (2021) 24(3). doi: 10.3892/mmr.2021.12313
16. Zhang Q, Hu Y, Hu JE, Ding Y, Shen Y, Xu H, et al. Sp1-mediated upregulation of Prdx6 expression prevents podocyte injury in diabetic nephropathy via mitigation of oxidative stress and ferroptosis. Life Sci (2021) 278:119529. doi: 10.1016/j.lfs.2021.119529
17. Zhang J, Fu C, Cui K, Ma X. Papillary thyroid carcinoma with tracheal invasion: a case report. Med (Baltimore) (2019) 98(38):e17033. doi: 10.1097/MD.0000000000017033
18. Ritchie ME, Phipson B, Wu D, Hu Y, Law CW, Shi W, et al. Limma powers differential expression analyses for RNA-sequencing and microarray studies. Nucleic Acids Res (2015) 43(7):e47. doi: 10.1093/nar/gkv007
19. Wang L, Cao C, Ma Q, Zeng Q, Wang H, Cheng Z, et al. RNA-Seq analyses of multiple meristems of soybean: novel and alternative transcripts, evolutionary and functional implications. BMC Plant Biol (2014) 14:169. doi: 10.1186/1471-2229-14-169
20. Yu G, Wang LG, Han Y, He QY. clusterProfiler: an r package for comparing biological themes among gene clusters. OMICS (2012) 16(5):284–7. doi: 10.1089/omi.2011.0118
21. Shannon P, Markiel A, Ozier O, Baliga NS, Wang JT, Ramage D, et al. Cytoscape: a software environment for integrated models of biomolecular interaction networks. Genome Res (2003) 13(11):2498–504. doi: 10.1101/gr.1239303
22. Shi J, Jiang D, Yang S, Zhang X, Wang J, Liu Y, et al. LPAR1, correlated with immune infiltrates, is a potential prognostic biomarker in prostate cancer. Front Oncol (2020) 10:846. doi: 10.3389/fonc.2020.00846
23. Hu D, Zhou M, Zhu X. Deciphering immune-associated genes to predict survival in clear cell renal cell cancer. BioMed Res Int (2019) 2019:2506843. doi: 10.1155/2019/2506843
24. Dewanjee S, Das S, Das AK, Bhattacharjee N, Dihingia A, Dua TK, et al. Molecular mechanism of diabetic neuropathy and its pharmacotherapeutic targets. Eur J Pharmacol (2018) 833:472–523. doi: 10.1016/j.ejphar.2018.06.034
25. Doll S, Proneth B, Tyurina YY, Panzilius E, Kobayashi S, Ingold I, et al. ACSL4 dictates ferroptosis sensitivity by shaping cellular lipid composition. Nat Chem Biol (2017) 13(1):91–8. doi: 10.1038/nchembio.2239
26. Cui Y, Zhang Y, Zhao X, Shao L, Liu G, Sun C, et al. ACSL4 exacerbates ischemic stroke by promoting ferroptosis-induced brain injury and neuroinflammation. Brain Behav Immun (2021) 93:312–21. doi: 10.1016/j.bbi.2021.01.003
27. Zhang LL, Zhang ZJ. Sestrin2 aggravates oxidative stress of neurons by decreasing the expression of Nrf2. Eur Rev Med Pharmacol Sci (2018) 22(11):3493–501. doi: 10.26355/eurrev_201806_15176
28. Miao M, Wang Y, Zeng S, Han Y, Zhu R, Yu P, et al. Identification and validation of ferroptosis-related genes in sevoflurane-induced hippocampal neurotoxicity. Oxid Med Cell Longev (2022) 2022:4435161. doi: 10.1155/2022/4435161
29. van Dam PS. Oxidative stress and diabetic neuropathy: pathophysiological mechanisms and treatment perspectives. Diabetes Metab Res Rev (2002) 18(3):176–84. doi: 10.1002/dmrr.287
Keywords: diabetic peripheral neuropathy, ferroptosis, bioinformatics, oxidative stress, immune-environment
Citation: Tian M, Zhi JY, Pan F, Chen YZ, Wang AZ, Jia HY, Huang R and Zhong WH (2023) Bioinformatics analysis identifies potential ferroptosis key genes in the pathogenesis of diabetic peripheral neuropathy. Front. Endocrinol. 14:1048856. doi: 10.3389/fendo.2023.1048856
Received: 20 September 2022; Accepted: 10 April 2023;
Published: 12 May 2023.
Edited by:
Cheng-Hsien Lu, Kaohsiung Chang Gung Memorial Hospital, TaiwanCopyright © 2023 Tian, Zhi, Pan, Chen, Wang, Jia, Huang and Zhong. This is an open-access article distributed under the terms of the Creative Commons Attribution License (CC BY). The use, distribution or reproduction in other forums is permitted, provided the original author(s) and the copyright owner(s) are credited and that the original publication in this journal is cited, in accordance with accepted academic practice. No use, distribution or reproduction is permitted which does not comply with these terms.
*Correspondence: Wen Hui Zhong, Q2FwdGFpbi0xOTc2QHNqdHUuZWR1LmNu; Hui Ying Jia, amlheWluZzc4MTAxOUAxNjMuY29t; Rong Huang, aHVhbmdyb25nc2NpQDE2My5jb20=
†These authors share first authorship