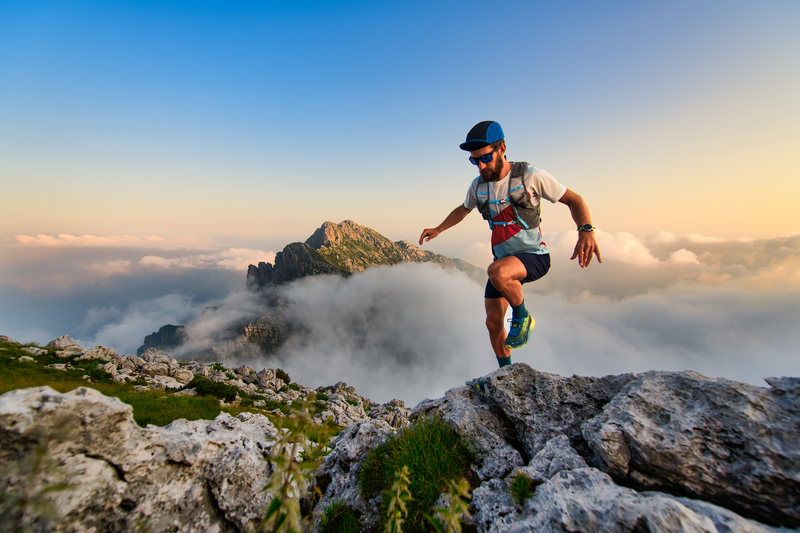
95% of researchers rate our articles as excellent or good
Learn more about the work of our research integrity team to safeguard the quality of each article we publish.
Find out more
ORIGINAL RESEARCH article
Front. Endocrinol. , 01 December 2022
Sec. Thyroid Endocrinology
Volume 13 - 2022 | https://doi.org/10.3389/fendo.2022.993856
Background: Papillary thyroid cancer (PTC) is the most common subtype of thyroid cancer and is characterized by an overall good prognosis and early-stage lymph node metastasis. The immune microenvironment is believed to play a crucial role in PTC initiation, progression and metastasis. However, to our knowledge, prognostic tools for thyroid cancer metastasis based on immune scores have not been adequately explored. This study aimed to construct a clinical nomogram to predict lymph node metastasis in patients with PTC.
Methods: The genomic data and clinical-pathological characteristics of 447 PTC subjects were obtained from TCGA (The Cancer Genome Atlas data). Logistic regression models were performed for univariate and multivariate analyses to identify significant prediction factors. A prognostic nomogram was built based on the multivariate analysis results. The concordance index (C-index) and calibration curve were used to assess the predictive accuracy and discriminative ability of the model.
Results: The patients were divided into two subgroups based on immune scores. We found that patients with high immune scores had significantly higher lymph node metastasis risks (OR and 95% confidence interval [CI]: 1.774[1.130-2.784]) than those with low immune scores. The C-index for lymph node metastasis was 0.722 (95% CI, 0.671‐0.774), which had a favorable performance for clinical prediction. The calibration curve for lymph node metastasis showed significant agreement between the nomogram prediction and actual observation.
Conclusion: High immune scores are significantly correlated with higher lymph node metastasis risk in patients with PTC. Immune score-based prognostic nomograms may help to predict lymph node metastasis and have potential clinical application possibilities.
Thyroid cancer is the most common malignancy in the endocrine system, and the incidence has steadily increased in most countries over the last three decades, with 586,000 new cases in 2020 (http://gco.iarc.fr). Thyroid cancer is now the fastest-growing malignancy worldwide (1, 2) and is mainly associated with the gradual rise in the number of papillary thyroid cancers (3). However, mortality rates have generally shown a stable or decreasing trend and vary significantly between countries. It was reported that the incidence and mortality of thyroid cancer were 9.61/105 and 0.35/105, respectively, in China in 2015, and women have been found to have a higher incidence and mortality than men of all ages (4, 5).
Papillary thyroid cancer (PTC) is the most common histological type of thyroid cancer (6) and the only subtype with a growing incidence in all countries. In many Asian countries, the incidence of PTC has continued to increase more than threefold in the past decade, likely due to the development and application of detection techniques such as ultrasound, CT and MRI and changes in health concepts (1, 5). The vast majority of PTC patients have slow progression and an overall favorable prognosis with a 10-year survival rate of >90% (5). Meanwhile, studies have reported that PTC has a higher risk of early lymph node metastasis, as 20%-90% of PTC patients have lymph node metastasis at the time of initial diagnosis, and the risk of lymph node metastasis and recurrence is as high as 50% and 20%, respectively (7).
Tumor cells and their environment together constitute the tumor microenvironment (TME), which consists of tumor cells, immune cells, stromal cells, microvasculature, and various chemokines and cytokines. In recent years, a growing body of research has shown the association between the TME and tumor cells, especially the crucial role of immune cells in tumor initiation, progression and metastasis (8, 9). Some studies have revealed that immune infiltration is associated with the malignancy level, recurrence and overall survival of PTC (8, 10, 11). Several studies have clarified that immunotherapy, represented by immune checkpoint inhibitors, has a considerable commitment in the treatment of advanced thyroid cancer (12, 13). Challenges remain with respect to identifying PTC patients who could benefit most from immunotherapy. Additionally, the overall infiltration of immune cells in tumor tissue could be estimated from gene expression data by the ESTIMATE algorithm (14), which produces the immune score. Specific immune cells, such as dendritic cells, mast cells, Tregs (12) and neutrophils (15), are correlated with lymph node metastasis and poor prognosis in thyroid cancer. Yet no study has demonstrated the involvement of immune scores in the prediction of lymph node metastasis in PTC.
This study aimed to evaluate the correlation between the immune score and clinical-pathological features of PTC, especially lymph node metastasis, and to construct a nomogram based on the immune score for predicting PTC lymph node metastasis.
The data used in this study were obtained from The Cancer Genome Atlas (TCGA) dataset (16). The TCGA database is the largest open source data platform for oncogenetic analysis, containing genetic and clinical information for over 200 kinds of tumors, as well as the measurement of DNA methylation and RNA sequencing (https://portal.gdc.cancer.gov/). Gene information is mainly TCGA mRNA transcriptome data downloaded via USCS Xena (https://xena.ucsc.edu/), with expression data for 19,303 genes from 497 samples. The key variable immune score, representing the immune cell infiltration level, was evaluated.
Corresponding clinical-pathological data were also obtained from USCS Xena (https://xena.ucsc.edu/), which included patient demographic data (e.g., TCGA patient number, age, sex, etc.), tumor-related data (e.g., tumor size, histological type, presence of multifocality, extrathyroid extension, etc.), lymph node metastases, and distant metastases (17, 18).
Inclusion criteria were cases with a pathological diagnosis of papillary thyroid cancer from 1993 to 2013. Exclusion criteria were unknown data on lymphadenopathy status and tumor size. A total of 447 cases could be available for further analysis after the exclusion of duplication.
To evaluate the infiltrating levels of immune cells involved in the tumor microenvironment (TME), the ESTIMATE algorithm (Estimation of STromal and Immune cells in MAlignant Tumors using Expression data) was applied based on the transcriptome data of PTC samples (14). Immune scores were calculated by performing the single-sample gene set enrichment analysis (ssGSEA) from the top-ranked 141 immune signature genes with a specific formula, indicating the sum total score of tumor-infiltrating immune cell enrichment like macrophages, dendritic cells, neutrophils, NK cells, B cells, T cells, mast cells.
Lymph node metastasis nomograms were formulated based on the results of multivariate regression analysis in R (version 4.1.2; R Foundation for Statistical Computing, Vienna, Austria). The nomogram was subjected to 1000 bootstrap resamples for internal validation of the analyzed database. The performance of the model was evaluated by calculating the concordance index (C-index). The C-index ranges from 0.5 to 1.0, with closer to 1.0 indicating better predictive ability and 0.5 indicating a random chance (19). The discriminatory ability of the model is shown by plotting a calibration curve (20).
The outcome event was lymph node metastasis in any location and number. The cutoff point of the immune score was calculated by SPSS (version 20.0; Illinois, Chicago, USA), whereby the immune score was transformed from a continuous variable to a binary variable.
The following statistics were made use of STATA (version 12.0; Stata Corp, College Station, TX). Correlation analysis of immune score and clinical pathological characteristics was analyzed using Pearson’s chi-square test or Fisher’s exact test. Univariate and multivariate logistic regression models were used to identify independent predictors of lymph node metastasis. After the adjustment of clinical-pathological characteristics, odds ratios (ORs) and 95% confidence intervals (CIs) were estimated. All statistical tests were two-sided, and P values of <0.05 were considered statistically significant.
A total of 447 patients who were pathologically diagnosed with PTC between 1993 and 2013 were included in this study, with a median follow-up time of 943 (535, 1474) days. They were divided into 2 groups according to the presence of lymph node metastases, of which 227 had no lymph node metastases and 220 had lymph node metastases. Among these patients, 122 (27.3%) were male, and 325 (72.7%) were female. The median age of the patients was 46 (36, 58). Pathological T distribution was T1 (29.3%), T2 (31.1%), T3 (34.9%), T4 (4.7%); pathological N distribution was N0 (50.7%), N1 (49.3%); pathological M distribution was M0 (97.8%), M1 (2.2%); overall stage distribution was I (55.7%), II (9.7%), III (23.4%) and IV (11.2%). The median immune infiltration score was 304.05 (-177.092, 913.311), with an optimal cutoff point of 459.93, according to which 191 (42.7%) were divided into a high immune score subgroup and 256 (57.2%) were divided into a low immune score subgroup.
The clinical-pathological characteristics of the different immune infiltration scores are detailed in Table 1. The high immune score group tended to have higher overall staging and was more likely to be in stages III-IV than the lower immune score group and a higher proportion of classic PTC.
Univariate analysis showed that clinical-pathological factors such as immune score, pathological T stage, sex, age, presence of multifocality, extrathyroid extension and histological type were significantly associated with lymph node metastasis (P<0.1), while tumor location and pathological M stage were not predictors of lymph node metastasis in patients with PTC (P>0.1). The results of the univariate analysis are detailed in Table 2.
Table 2 Univariate analyses of LNM among PTC patients according to clinical-pathological characteristics and immune scores.
Multivariate logistic regression analysis was performed on six variables, including immune score (low or high), age (<55, ≥55), sex (male or female), pathological T stage (I–IV), presence of multifocality (no or yes) and histological type (classical PTC or nonclassical PTC). The results revealed a significantly increased risk of lymph node metastasis in the high immune score group (OR (95% CI): 1.774 (1.130, 2.784)). In addition, age <55 years old, pathological T stage, unifocal lesion, and nonclassical PTC were associated with a higher risk of lymph node metastasis, whereas gender differences were not significantly associated with lymph node metastasis.
Patients aged ≥55 years had a lower risk of lymph node metastasis than patients with PTC aged <55 years (OR (95% CI): 0.465 (0.280, 0.773)). Patients with stage T2-4 had a significantly increased risk of lymph node metastasis compared to patients with stage T1 (OR (95% CI): 1.898 (1.064, 3.387), 3.705 (2.063, 6.654) and 14.600 (4.123, 51.668) for T2, T3 and T4, respectively). Patients with multifocal lesions (OR (95% CI): 1.595 (1.006, 2.529) and nonclassical histological type (OR (95% CI): 0.370 (0.215, 0.636)) had a higher risk of lymph node metastasis. The results of the multivariate analysis are detailed in Table 3.
Table 3 Multivariate analyses of LNM among PTC patients according to clinical-pathological characteristics and immune scores.
Based on five independent risk factors screened through multivariate logistic regression, we constructed a prognostic model for the risk of lymph node metastasis in patients with PTC (Figure 1). The predictive ability of the model was evaluated by the concordance index (0.722 (95% CI: 0.671-0.774)) (Figure 2A). The calibration curve for the risk of lymph node metastasis showed good agreement between the predicted and actual observations in the nomogram (Figure 2B).
Figure 2 (A) The ROC curve of the immune-score-based nomogram. (B) The calibration curve of lymph node metastasis for papillary thyroid cancer.
In the current study, we evaluated the predictive significance of the immune score for lymph node metastasis in papillary thyroid cancer. After adjustment for clinical-pathological prognostic factors such as age, sex, T stage, presence of multifocality and histological type, immune scores were significantly associated with lymph node metastasis risk. Hence, we constructed an immune score-based prognostic nomogram for predicting the presence of lymph node metastasis in PTC.
Immune cells play a key role in tumor progression, and immune cell infiltration is considered a prognostic biomarker for immunotherapy (8). Our findings showed that a higher immune score is significantly related to a higher risk of lymph node metastasis. A high immune score suggests the predominance of immune cell infiltration in the tumor microenvironment. A likely explanation is that the immune microenvironment may be dominated by immunosuppressive cells like macrophages, neutrophils (15), dendritic cells (21) and mast cells (22). Studies have demonstrated that immune cells infiltrating in tumor tissue have both pro-tumor and anti-tumor functions. In papillary thyroid cancer, many infiltrating immune cells like tumor-associated macrophages (TAMs), tumor-associated mast cells (TAMCs), and regulatory T cells (Tregs) would contribute to tumor cell immune escape and be related to poor prognosis. Despite numerical superiority, most immune cells are phenotypically immature and functionally weakened, and effector cells, such as plasma cells (8), are decreased, which may reduce cytotoxic T lymphocyte, B cell and NK cell-mediated cancer cell killing through the release of cytokines (9). Thus, the overall immune response is reduced, leading to increased tumor aggressiveness (23–25). The pro-tumor behavior of infiltrating immune cells may be related to a higher BRAF V600E point mutation rate.
However, we did not successfully verify the clinical characteristics that were previously confirmed to have a significant association with aggressiveness and lymph node metastasis, such as multifocality (26–28). Possible reasons for this include the relatively small sample size, selection bias on the raw data, the different inclusion criteria and study setting compared with previous studies. Thus, the clinical-pathological characteristics, especially those significant during the univariate analysis but not in multivariate analysis, need to be further evaluated by more multicenter studies with larger sample sizes.
The results showed that younger age, larger tumor volumes (T2-4), and nonclassical histological type were relatively more likely to develop lymph node metastasis, which was in line with the findings of previous studies (29–33).
To our knowledge, our study built the first nomogram for predicting lymph node metastasis risk in PTC based on the immune score. This scoring system allows physicians to comprehensively assess the risk of lymph node metastasis in patients and develop treatment plans appropriate for individual patients, including lymph node dissection and I131 therapy.
There were still some limitations that should be mentioned. First, this study made use of TCGA public data, which is a nonrandomized, retrospective study. The TCGA database does not include preoperative cervical ultrasonography, so the analysis was based on pathological staging but not preoperative clinical staging. Additionally, there may have been some information bias and selection bias in the raw data due to the lack of genetic data available for immune score calculation. Second, some of the results in our study differed from those reported in previous studies, which may be related to differences in inclusion criteria and data selection. Third, the univariate and multivariate analyses and nomogram construction relied on logistic regression, which may overestimate the OR value when the incidence of outcome events is high (>10%) (34). Fourth, the nomogram lacks external validation, which limits its clinical application. Hence, more prospective cohorts for external validation are needed to assess the reliability and practicability of our nomogram.
The high immune score group was significantly correlated with lymph node metastasis. Age <55 years, T-stage, unifocal lesions, and nonclassical PTC were also associated with a higher risk of lymph node metastasis. A nomogram constructed based on immune scores and other clinical-pathological features can help predict lymph node metastasis and has potential clinical application.
Publicly available datasets were analyzed in this study. This data can be found here: https://xenabrowser.net/datapages/?cohort=GDC%20TCGA%20Thyroid%20Cancer%20(THCA)&removeHub=https%3A%2F%2Fxena.treehouse.gi.ucsc.edu%3A443.
KG contributed to the conception and design of the study. KG and YL downloaded and organized the database. YL performed the statistical analysis. YL wrote the first draft of the manuscript. KQ, MF wrote sections of the manuscript. All authors contributed to the article and approved the submitted version.
This study was supported by National Natural Science Foundation of China (81972496); Outstanding Academic Leaders Plan, Huangpu District, Shanghai; Adolescents Science & Technology Innovation studio, Shanghai Jiao Tong University School of Medicine.
The authors declare that the research was conducted in the absence of any commercial or financial relationships that could be construed as a potential conflict of interest.
The reviewer SX declared a shared affiliation with the authors to the handling editor at the time of review.
All claims expressed in this article are solely those of the authors and do not necessarily represent those of their affiliated organizations, or those of the publisher, the editors and the reviewers. Any product that may be evaluated in this article, or claim that may be made by its manufacturer, is not guaranteed or endorsed by the publisher.
The Supplementary Material for this article can be found online at: https://www.frontiersin.org/articles/10.3389/fendo.2022.993856/full#supplementary-material
1. Vaccarella S, Franceschi S, Bray F, Wild CP, Plummer M, Dal Maso L. Worldwide thyroid-cancer epidemic? The increasing impact of overdiagnosis. N Engl J Med (2016) 375(7):614–7. doi: 10.1056/NEJMp1604412
2. Li M, Dal Maso L, Vaccarella S. Global trends in thyroid cancer incidence and the impact of overdiagnosis. Lancet Diabetes Endocrinol (2020) 8(6):468–70. doi: 10.1016/S2213-8587(20)30115-7
3. Lim H, Devesa SS, Sosa JA, Check D, Kitahara CM. Trends in thyroid cancer incidence and mortality in the United States, 1974-2013. JAMA (2017) 317(13):1338–48. doi: 10.1001/jama.2017.2719
4. Wang JY. Thyroid cancer: Incidence and mortality trends in China, 2005-2015. Endocrine (2020) 68:163–73. doi: 10.1007/s12020-020-02207-6
5. Chen W, Zheng R, Baade PD, Zhang S, Zeng H, Bray F, et al. Cancer statistics in China, 2015. CA Cancer J Clin (2016) 66(2):115–32. doi: 10.3322/caac.21338
7. Brassard M, Borget I, Edet-Sanson A, Giraudet AL, Mundler O, Toubeau M, et al. Long-term follow-up of patients with papillary and follicular thyroid cancer: A prospective study on 715 patients. J Clin Endocrinol Metab (2011) 96(5):1352–9. doi: 10.1210/jc.2010-2708
8. Na KJ, Choi H. Immune landscape of papillary thyroid cancer and immunotherapeutic implications. Endocr Relat Cancer (2018) 25(5):523–31. doi: 10.1530/ERC-17-0532
9. Ferrari SM, Fallahi P, Galdiero MR, Ruffilli I, Elia G, Ragusa F, et al. Immune and inflammatory cells in thyroid cancer microenvironment. Int J Mol Sci (2019) 20(18):E4413. doi: 10.3390/ijms20184413
10. Sun J, Shi R, Zhang X, Fang D, Rauch J, Lu S, et al. Characterization of immune landscape in papillary thyroid cancer reveals distinct tumor immunogenicity and implications for immunotherapy. Oncoimmunology (2021) 10(1):e1964189. doi: 10.1080/2162402X.2021.1964189
11. Yang Z, Wei X, Pan Y, Xu J, Si Y, Min Z, et al. A new risk factor indicator for papillary thyroid cancer based on immune infiltration. Cell Death Dis (2021) 12(1):51. doi: 10.1038/s41419-020-03294-z
12. Varricchi G, Loffredo S, Marone G, Modestino L, Fallahi P, Ferrari SM, et al. The immune landscape of thyroid cancer in the context of immune checkpoint inhibition. Int J Mol Sci (2019) 20(16):E3934. doi: 10.3390/ijms20163934
13. French JD, Bible K. Leveraging the immune system to treat advanced thyroid cancers. Lancet Diabetes Endocrinol (2016) 5(6):469–81.
14. Yoshihara K, Shahmoradgoli M, Martínez E, Vegesna R, Kim H, Torres-Garcia W, et al. Inferring tumour purity and stromal and immune cell admixture from expression data. Nat Commun (2013) 4:2612. doi: 10.1038/ncomms3612
15. Bergdorf K, Ferguson DC, Mehrad M, Ely K, Stricker T, Weiss VL. Papillary thyroid carcinoma behavior: Clues in the tumor microenvironment. Endocr Relat Cancer (2019) 26(6):601–14. doi: 10.1530/ERC-19-0074
16. Cancer Genome Atlas Research Network. Integrated genomic characterization of papillary thyroid carcinoma. Cell (2014) 159(3):676–90.
17. Bian F, Li C, Han D, Xu F, Lyu J. Competing-risks model for predicting the postoperative prognosis of patients with papillary thyroid adenocarcinoma based on the surveillance, epidemiology, and end results (SEER) database. Med Sci Monit Int Med J Exp Clin Res (2020) 26:e924045. doi: 10.12659/MSM.924045
18. van Gerwen M, Alpert N, Sinclair C, Kale M, Genden E, Taioli E. Assessing non-aggressiveness of untreated, local and regional, papillary thyroid cancer. Oral Oncol (2020) 105:104674. doi: 10.1016/j.oraloncology.2020.104674
19. Longato E, Vettoretti M, Di Camillo B. A practical perspective on the concordance index for the evaluation and selection of prognostic time-to-event models. J BioMed Inform (2020) 108:103496. doi: 10.1016/j.jbi.2020.103496
20. Alba AC, Agoritsas T, Walsh M, Hanna S, Iorio A, Devereaux PJ, et al. Discrimination and calibration of clinical prediction models: Users’ guides to the medical literature. JAMA (2017) 318(14):1377–84. doi: 10.1001/jama.2017.12126
21. Janco JMT. Tumor-infiltrating dendritic cells in cancer pathogenesis. J Immunol (2015) 194(7):2985–91. doi: 10.4049/jimmunol.1403134
22. Xie Z, Li X, He Y, Wu S, Wang S, Sun J, et al. Immune cell confrontation in the papillary thyroid carcinoma microenvironment. Front Endocrinol (2020) 11:570604. doi: 10.3389/fendo.2020.570604
23. Joyce JA, Fearon DT. T Cell exclusion, immune privilege, and the tumor microenvironment. Science (2015) 348(6230):74–80. doi: 10.1126/science.aaa6204
24. Yu H, Huang X, Liu X, Jin H, Zhang G, Zhang Q, et al. Regulatory T cells and plasmacytoid dendritic cells contribute to the immune escape of papillary thyroid cancer coexisting with multinodular non-toxic goiter. Endocrine (2013) 44(1):172–81. doi: 10.1007/s12020-012-9853-2
25. Hilly O, Koren R, Raz R, Rath-Wolfson L, Mizrachi A, Hamzany Y, et al. The role of s100-positive dendritic cells in the prognosis of papillary thyroid carcinoma. Am J Clin Pathol (2013) 139(1):87–92. doi: 10.1309/AJCPAKYDO56NKMYZ
26. Al Afif A, Williams BA, Rigby MH, Bullock MJ, Taylor SM, Trites J, et al. Multifocal papillary thyroid cancer increases the risk of central lymph node metastasis. Thyroid Off J Am Thyroid Assoc (2015) 25(9):1008–12. doi: 10.1089/thy.2015.0130
27. Feng JW, Qu Z, Qin AC, Pan H, Ye J, Jiang Y. Significance of multifocality in papillary thyroid carcinoma. Eur J Surg Oncol J Eur Soc Surg Oncol Br Assoc Surg Oncol (2020) 46(10 Pt A):1820–8. doi: 10.1016/j.ejso.2020.06.015
28. Genpeng L, Jianyong L, Jiaying Y, Ke J, Zhihui L, Rixiang G, et al. Independent predictors and lymph node metastasis characteristics of multifocal papillary thyroid cancer. Med (Baltimore) (2018) 97(5):e9619. doi: 10.1097/MD.0000000000009619
29. Liu C, Xiao C, Chen J, Li X, Feng Z, Gao Q, et al. Risk factor analysis for predicting cervical lymph node metastasis in papillary thyroid carcinoma: a study of 966 patients. BMC Cancer (2019) 19(1):622. doi: 10.1186/s12885-019-5835-6
30. Yang Z, Heng Y, Lin J, Lu C, Yu D, Tao L, et al. Nomogram for predicting central lymph node metastasis in papillary thyroid cancer: A retrospective cohort study of two clinical centers. Cancer Res Treat (2020) 52(4):1010–8. doi: 10.4143/crt.2020.254
31. Ganly I, Ibrahimpasic T, Rivera M, Nixon I, Palmer F, Patel SG, et al. Prognostic implications of papillary thyroid carcinoma with tall-cell features. Thyroid Off J Am Thyroid Assoc (2014) 24(4):662–70. doi: 10.1089/thy.2013.0503
32. Nath MC, Erickson LA. Aggressive variants of papillary thyroid carcinoma: Hobnail, tall cell, columnar, and solid. Adv Anat Pathol (2018) 25(3):172–9. doi: 10.1097/PAP.0000000000000184
33. Mao J, Zhang Q, Zhang H, Zheng K, Wang R, Wang G. Risk factors for lymph node metastasis in papillary thyroid carcinoma: A systematic review and meta-analysis. Front Endocrinol (2020) 11:265. doi: 10.3389/fendo.2020.00265
Keywords: immune score, immune microenvironment, nomogram, lymph node metastasis, papillary thyroid cancer (PTC)
Citation: Lu Y, Qian K, Fei M, Guo K, Shi Y and Wang Z (2022) A prognostic nomogram for papillary thyroid cancer lymph node metastasis based on immune score. Front. Endocrinol. 13:993856. doi: 10.3389/fendo.2022.993856
Received: 14 July 2022; Accepted: 17 November 2022;
Published: 01 December 2022.
Edited by:
Terry Francis Davies, Icahn School of Medicine at Mount Sinai, United StatesReviewed by:
Shujun Xia, Shanghai Jiao Tong University, ChinaCopyright © 2022 Lu, Qian, Fei, Guo, Shi and Wang. This is an open-access article distributed under the terms of the Creative Commons Attribution License (CC BY). The use, distribution or reproduction in other forums is permitted, provided the original author(s) and the copyright owner(s) are credited and that the original publication in this journal is cited, in accordance with accepted academic practice. No use, distribution or reproduction is permitted which does not comply with these terms.
*Correspondence: Zhuoying Wang, emh1b3lpbmd3YW5nQGhvdG1haWwuY29t
†These authors have contributed equally to this work
Disclaimer: All claims expressed in this article are solely those of the authors and do not necessarily represent those of their affiliated organizations, or those of the publisher, the editors and the reviewers. Any product that may be evaluated in this article or claim that may be made by its manufacturer is not guaranteed or endorsed by the publisher.
Research integrity at Frontiers
Learn more about the work of our research integrity team to safeguard the quality of each article we publish.