- Laboratoire GBCM, EA 7528, Conservatoire National des Arts et Métiers, Hésam Université, Paris, France
Being in the center of both therapeutic and toxicological concerns, NRs are widely studied for drug discovery application but also to unravel the potential toxicity of environmental compounds such as pesticides, cosmetics or additives. High throughput screening campaigns (HTS) are largely used to detect compounds able to interact with this protein family for both therapeutic and toxicological purposes. These methods lead to a large amount of data requiring the use of computational approaches for a robust and correct analysis and interpretation. The output data can be used to build predictive models to forecast the behavior of new chemicals based on their in vitro activities. This atrticle is a review of the studies published in the last decade and dedicated to NR ligands in silico prediction for both therapeutic and toxicological purposes. Over 100 articles concerning 14 NR subfamilies were carefully read and analyzed in order to retrieve the most commonly used computational methods to develop predictive models, to retrieve the databases deployed in the model building process and to pinpoint some of the limitations they faced.
1 Introduction
Nuclear receptors (NRs) are a large family of transcription factors. They are involved in a wide variety of biological and physiological processes such as growth, metabolism, reproduction, cell proliferation, differentiation, development and homeostasis (1, 2). The NRs superfamily is composed of 48 members in humans and is divided in 7 subgroups. Apart from SHP and DAX, all the NRs share the overall same architecture comprising 5 domains, named A to E, each of which playing a specific role. The E domain is a structurally conserved allosteric signaling region forming the ligand binding domain LBD. This domain includes 12 helices and 4 β‐strands that create a buried hydrophobic ligand‐binding pocket (LBP) able to interact with small molecules ligands. The LBD and in particular the helix H12, called the activation function helix (AF‐H), change conformation upon ligand-binding facilitating the interaction of the LBD with co-activators or co-repressors proteins. The DNA binding domain (C domain) of the activated NR can bind to a specific DNA sequence and recruit co-regulator proteins that either promote or repress the DNA transcription and therefore, specific gene expression (1).
Although the mechanism of action of NRs is very well-adjusted, dysfunctions of their signaling pathways have been linked with diseases such as cancers, diabetes or auto-immune disorders. NRs biology and ability to interact with small lipophilic molecules in the LBD have led to their emergence as a major class of therapeutic drug targets for these diseases, accounting for more than 10% of FDA-approved drugs (1–5).
However, exogenous ligand binding may also cause dysfunctions of NRs pathways. As such, some (candidate) drugs side effects are related to their unforeseen interaction with NRs like nifedipine and the antifungal clotrimazole who are able to activate PXR (6). More recently, a category of compounds called the endocrine disrupting chemicals (EDCs), have been associated with NRs, especially the sex hormones receptors such as Estrogen Receptors (ER) and Androgen Receptors (AR). Although the concept has been introduced since 1958, the scientific outline has evolved to settle in 2012 on a definition proposed by the Endocrine society (7). EDCs are environmental compounds that can be found in fumes, cosmetic additives or pesticides that can enter the human body due to their high lipophilicity (8). They can affect the endocrine system through several mechanisms (8) (9) including “direct” and “indirect” mechanisms. In the “direct” mechanism, EDCs bind to human NRs (hNRs) LBD and dysregulate the functioning of the endocrine system either by excessively activating or repressing the associated biological activity (7, 9). In the “indirect” mechanism, EDCs can alter not only the synthesis, metabolism, transportation and fate of hormones in the body but also the hormone-receptors expression, the signal transduction as well as the epigenic behind (8). Up to 2019, more than 1000 suspected EDCs have been reported (10). It is to note that EDCs can also affect other protein families than the NRs (11–13). For this review, we will focus on the NRs related “direct” mechanism of action.
Identifying hNRs binders, i.e. compounds able to bind to hNRs, is important for both therapeutic and toxicologic purposes: to discover new therapeutic compounds for several NRs-related diseases, to predict potential off-target effects, to guarantee the safety of novel synthesized molecules and to identify potential EDCs in the exposome. In silico methods emerge as an asset to achieve this goal. The construction of predictive models of NRs ligand binding using in silico methods (14–16) enable to prioritize compounds that should be biologically evaluated to reduce the time, cost and technical issues associated with the experimental tests of a large number of compounds.
Previous reviews mostly focused on specific NRs as potential drug discovery targets (17, 18) while other evaluated models dedicated to EDCs prediction (7, 19). Herein, we present an exhaustive review of 89 in silico initiatives carried out on NRs on both therapeutic and toxicologic levels and published between 2010 and 2020. We provide a summary in order to 1) enumerate them in a referential, 2) list the available NRs-related data that can be used in modeling approaches and 3) learn from previous experiences to enable the elaboration of more accurate predictions.
2 General overview
This review focuses on 14 NRs subfamily members for which publications falling within the scope of our review were retrieved. 89 articles were carefully selected, read and analyzed. For each collected model, several aspects were identified including 1) the NR for which the model was developed, 2) the computational method used to build the model, 3) the DB used for the model training, testing and validation, 4) the level of reproducibility 5) if the study was prospective or retrospective, and 6) the purpose of the study (toxicological or therapeutic). The result of our bibliographic search is presented in Table 1–4 and point 2) to 4) are detailed in a following paragraph in this review.
In total, in this review, we identified 38 projects dedicated to the identification of therapeutic compounds, 44 to the prediction of toxicological compounds and 3 projects addressing both purposes. Distribution of publications related to each studied NR are depicted in Figure 1. The in silico methods used to construct the models are divided into two approaches: Ligand-Based (LB) and Structure-Based (SB) (Figure 2). The reviewed studies are also classified according to their study design into prospective and retrospective studies. Prospective studies are based on compounds for which no biological data is available for the query target. These compounds are subjected to virtual screening protocols in order to assess if they can be considered as potential NRs modulators. The obtained predictions are then validated by experimental assays. In retrospective studies, models that are developed aim at correctly forecasting the already known data activities. The models can then be used to achieve predictions for compounds with unknown activities as long as they remain within the activity domain. The reviewed papers were perfectly balanced in terms of study design as 43 model were retrospective and 43 were prospective with the latter including a majority of LB methods.
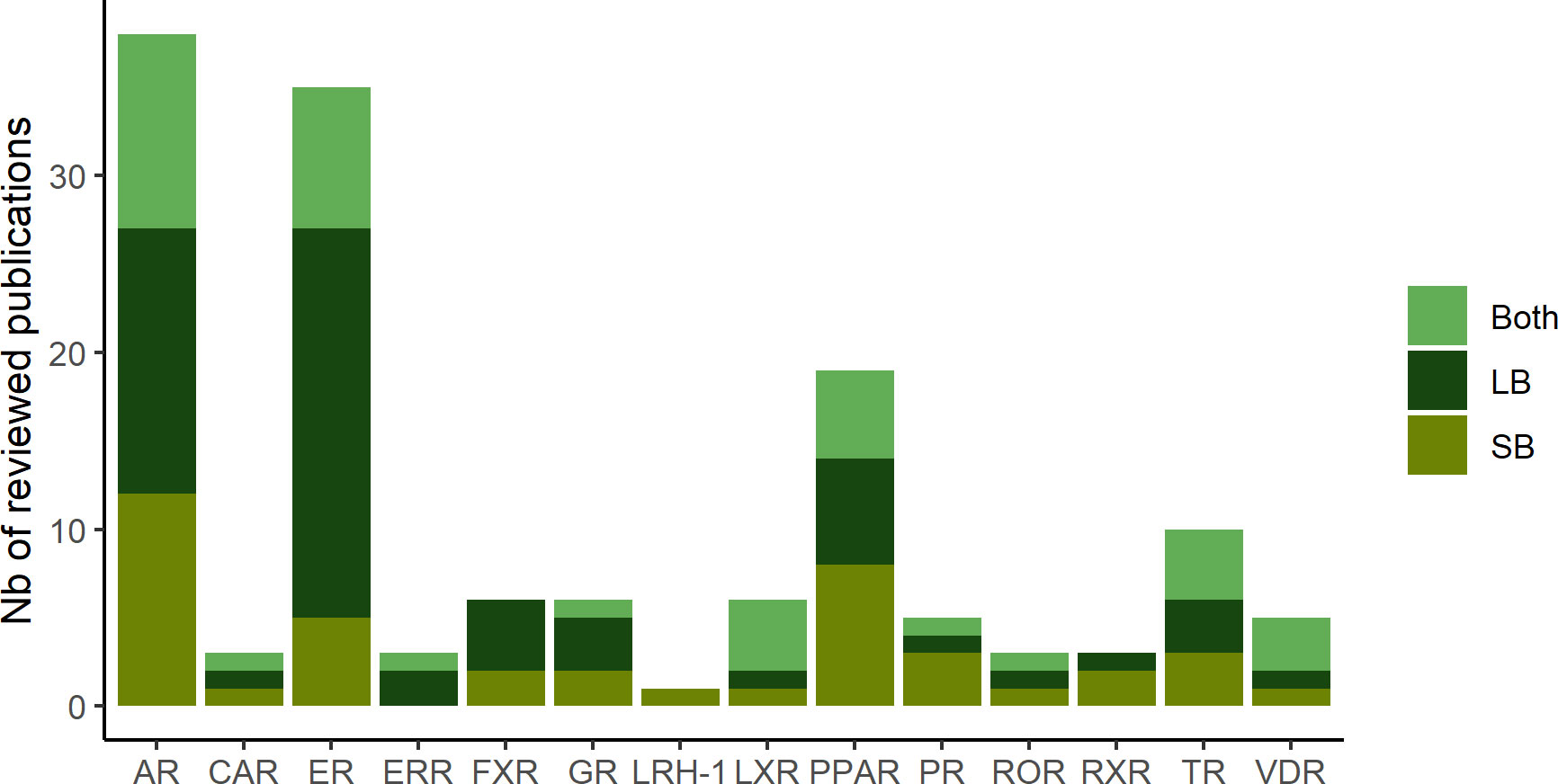
Figure 2 Distribution of the computational approach (SB: structure-based, LB: ligand-based and both: combination of SB and LB methods) in the reviewed publications for the different hNR.
3 Studied nuclear receptors
In addition to the IUPAC classification, NR can be distinguished according to structural differences, functions, tissue specificity, DNA binding motifs or the knowledge or not about an endogenous ligand. For this review, we divided the studied NR into three groups according to their structural similarities and function in three groups. 1) steroid hormones receptors, (Table 1) 2) RXR and its partners (Table 2) 3) monomeric orphan receptors (Table 3) (107, 108).
3.1 Steroid hormones receptors
The NR belonging to this class are all activated by an endogenous ligand presenting a steroid core. Upon binding to their native ligand, steroid hormones receptors undergo conformational changes leading to their homodimerization and subsequently to DNA binding. The reviewed articles dedicated to the steroid hormone receptors are listed in Table 1.
3.1.1 Estrogen receptors
Two isoforms of ER exist, namely ERα and ERβ. Both isoforms exhibit similar affinity for their native ligand, 17β Estradiol, but differential expression in the body and unique roles in estrogens action in vivo (55). Indeed, ERα’s effects are prominent in the mammary gland, uterus and in the preservation of skeletal homeostasis and the regulation of metabolism, while the activation of ERβ impacts the immune and central nervous systems. Moreover, ERβ exerts an anti-proliferative and pro-apoptotic activity that counteracts ERα’s actions towards cell growth and proliferation (54, 55). The established link between impairment of these ER pathways and diseases such as breast and endometrial cancers, osteoporosis, metabolic and cardiovascular diseases (55, 109) explains the number of published in silico studies with a therapeutic scope (8 up to the 23 ER-related reviewed studies). Additionally, ER are a well-known target for EDCs, and it has been shown that exposure to these chemicals increase the risk of breast cancer and immune diseases development (18). Consequently, several projects focused on this hNR as a central component of toxicological pathways including EDCs. It is the case of CERAPP, a large-scale screening project initiated by the US Environmental Protection Agency (EPA) (36). The project gathers models from American as well as European research groups to develop in silico models to evaluate thousands of chemicals for ER-related activity and prioritize them for further testing. CERAPP models, like most of the ER focused models reported in the literature, do not differentiate the two ER isoforms during the development of the models. It is to note that this distinction is crucial for therapeutic projects as many ligands display different affinities for each isoform (110). Designing selective ERβ ligands could help reducing ER related side effects besides exerting the desired estrogenic activity. Niu et al. (55), succeeded in building machine leaning models with high accuracies (77.10 to 88.34%) and AUCs greater than 0.8 that performed equally on an external validation dataset composed of ERβ selective agonists. It is to note that the negative data used for this study consisted of generated decoys rather than selective ERα ligands. Another particularity we could notice for ER models, was the predominance of Quantitative Structure Activity Relationship (QSAR) models based on machine learning (ML) algorithms. The most used ML algorithms were random forest, SVM and Naïve Bayes. Other computational approaches comprise molecular docking and pharmacophore modelling.
3.1.2 Androgen receptors
Modulated by dihydrotestosterone and testosterone, AR is involved in several physiological processes such as the male sexual differentiation, the development and maintenance of musculoskeletal and cardiovascular systems, as well as functionality of female ovarian follicles and ovulation (29, 111). The malfunctioning of these receptors is linked to several diseases including prostate, testicular and ovarian cancers, impaired reproduction system development and neuromuscular diseases (29, 112). Despite this large therapeutic potential, the 23 models and projects identified for AR during this review process were for a large majority conducted in the EDCs risk assessment perspective. Among them, the CoMPARA project (20) is the AR counterpart of the CERAPP project and falls under the Endocrine Disruptor Screening Program of the U.S. Environmental Protection Agency (EPA). The 25 international research teams forming the CoMPARA consortium used a common training set of 1,746 chemicals compiled from a data set of 11 ToxCast/Tox21 HTS in vitro assays to generate 91 predictive QSAR models for AR binding, agonist, and antagonist activity predictions. The resulting models were evaluated using non-overlapping curated literature data. Finally, all predictions were combined into consensus models with an average accuracy of 80%. In complement to the CoMPARA project, several computational LB models, obtained using deep learning and QSAR methods, were described in the literature. SB methods such as docking and MD were also used, albeit to a lesser extend because of a lack of AR structural data. In fact, there is no AR antagonist-bound crystal structure yet available in the PDB which can impair the prediction of AR antagonist molecules and the development of AR SB models. However, some groups managed to overcome this issue and relied on molecular dynamics simulations to generate AR structures in antagonist conformations that led to a vast improvement for the docking of AR antagonists (26).
3.1.3 Progesterone receptor
PR is expressed primarily in female reproductive tissues and in the central nervous system as two isomers, PRα and PRβ. Both isomers present identical structures except for the A/B domain but they target distinct gene networks in progesterone-responsive cells (113). PR is activated by binding its endogenous ligand, the progesterone. PR regulates a wide range of biological function in a context- and cell-specific manner including the development and differentiation processes in normal and malignant female tissues, in particular in patients suffering from breast cancer (114). Selective progesterone modulators (SPRMs) have thus been developed as drug candidates for breast cancer therapies (57). Moreover, PR is suspected to be involved in endocrine disruptor toxicity pathway as bisphenols and some pesticides have been characterized with a PR activity (107). The reviewed models reflect the involvement of this NRs in both aspects. Three in silico endocrine disruptors prediction tools (12, 100, 104, 105) include PR models. In particular, a prediction model of PR agonist compounds was constructed using a deep learning approach (100) and the Tox21 data. This model was associated with high prediction performance as measured by a Matthew correlation coefficient superior to 0.8 and an AUC value of 0.95. A structure-based prospective virtual screening to identify new PR inhibitors was also conducted (57) but no experimental validation of the results was provided.
3.1.4 Glucocorticoid receptors
Besides their well-known anti-inflammatory, anti-proliferative, pro-apoptotic and anti-angiogenic roles, glucocorticoids are involved in several other physiological processes affecting the nervous, cardiovascular, immune and respiratory systems (115, 116). Additionally, GR is incriminated in several toxicological pathways resulting in decreased male fertility and depression (35) and are a potential target for EDCs (19). GR is thus a relevant target for both therapeutic and toxicologic compounds. There are several isoforms of GR distributed through various tissues. GRα and GRβ present a similar sequence that differs only in the C-terminal region, whereas GRγ, GR-A, and GR-P are less characterized. It has been shown that GRβ negatively regulate the action of GRα as well as exerting its own. The remaining isoforms are associated to glucocorticoids insensitivity (115). In this review, only one therapeutic project was identified (106) whereas, 4 projects with toxicological applications for GR were found. Both SB, LB and their combination were applied to identify GR ligands. None of these projects did the distinction between all isoforms despite the difference in affinities towards glucocorticoids (115). It is to note that all the available GR structures in the PDB include the same mutation in helix 5 from a Phenylalanine residue to a Serine to overcome solubility problems during the crystallization experiments (117). However, this mutation is not located in the ligand binding site.
3.2 RXR and its partners
RXRα presents the particularity of forming heterodimers with one third of the 48 human NR (107) and is thus able to regulate a wide range of biological functions in a cell and tissue-specific manner (118). This class of NR can be subdivided in permissive heterodimers that can be activated by ligands of either RXR or its partner in the dimer (PPARs, LXRs, FXR, PXR and CAR) and non permissive heterodimers that generally require the RXR to be unbound in order to be activated by the native ligand of the dimer partner (TRs, RARs) (107). The reviewed articles focusing on RXR and its partners are presented in Table 2.
3.2.1 Retinoid X receptor alpha
As previously mentioned, RXRα can form heterodimers with several NR partners, but it can also be assembled and activated as an homodimer (108). A synthetical RXRα ligand, bexarotene, is notably marketed for the treatment of cutaneous T cell lymphoma (119). RXRα ligands have also demonstrated neuroprotective properties and are considered for the design of drug candidates for Alzheimer disease treatment (120). No study specifically dedicated to RXRα ligands prediction was identified during this review. However, 3 articles presenting models for several NRs including RXRα were available. Among them, two studies used SB methods. However, one of this study (106) is more focused on the comparison the performance of single and ensemble docking approaches with an active RXR data set limited to 11 compounds. In the other SB study, the RXR DUD-E data set (121) was used with a single docking approach to select the RXRα structure. Overall good performance in predicting RXR ligands was obtained with an AUC value close to 0.8 and an early enrichment factor value EF1% of 8.6. The third study presents a RXRα agonist ligands prediction model developed using a LB deep learning approach (100). The model was trained on the Tox21 database and was associated with an AUC value similar to those obtained in the previous study with the docking approach.
3.2.2 Peroxisome proliferator-activated receptors
Since the cloning of the first PPAR from the rat liver in 1990, the subfamily of PPAR has been enlarged and currently counts three members PPARα, PPARδ and PPARγ. Each isoform is differentially expressed in tissues and associated with different biological functions (122): PPARα is mainly expressed in the liver and regulates lipid metabolism (123); PPARγ is mainly expressed in adipose tissues and controls adipogenesis and carbohydrate metabolism (124); PPARδ is ubiquitously expressed and involved in atherosclerosis, pathologies of the nervous system, embryonic, organ and tissue development and metabolism of lipid and glucose (122). PPARδ is the less studied receptor of this group (75) while PPARα and PPARγ have been extensively investigated as therapeutic targets especially in metabolic diseases like type 2 diabetes (T2D) (68, 71, 78, 125, 126). Recently, PPARγ has also been proposed as a therapeutic target for ovarian cancer (77) and Alzheimer Disease (AD) (78). In this review, 11 models and projects dedicated to PPARs have been identified. The implication of this receptor in several pathologies explains the fact that all project specific to PPAR fall under the therapeutic application. Indeed, 8 studies described prospective virtual screening protocols combining several in silico methods for the prediction of dual PPARα/γ agonist ligands with therapeutic applications for T2D (70–72) and of PPARγ ligands (74–78). However, experimental validation was achieved for only 3 of these studies (75–77), which limits the evaluation of the protocols performance. The protocol presented by Kaserer et al. for PPARγ partial agonist ligands prediction (76) is of particular interest since a retrospective study was first achieved to select optimal models for the prospective screening. This protocol combines 3 pharmacophore models (associated with EF and AUC values of 6.5 and 0.92 respectively in the retrospective evaluation), 5 shape-based models (associated with EF and AUC values of 11.0 and 0.83 respectively in the retrospective evaluation), and a docking protocol (associated with EF and AUC values of 2.2 and 0.65 respectively in the retrospective evaluation). The top-ten ranked compounds by the three methods (29 compounds) were biologically evaluated and 9 novel PPARγ ligands were identified. Retrospective studies were also conducted on the PPARα/γ using 2D, 3D-QSAR, and docking (68) and to understand the structural factors responsible for PPARγ agonistic activity using a combined pharmacophoric/3D-QSAR approach (75). As PPARγ data is available from the Tox21 program, several studies trying to model the Tox21 data for a large panel of NRs also present PPARγ dedicated models (19, 100–103). All of these models were associated with high predictive power with AUC values comprised between 0.8 and 0.9.
3.2.3 Human pregnane X receptor
Due to its flexibility and the relative bulkiness of its binding site in comparison with other NRs (80), PXR activity can be modulated by binding to a wide range of endogenous compounds, from bile acids to steroid hormones but also xenobiotics, such as drugs or environmental chemicals (pesticides, phenols, cosmetics, phytoestrogens) that can dysregulate normal physiological functions (127–129). PXR is responsible for the modulation of the expression of enzymes and transporters associated with the metabolism and transport of several drugs. Thus, PXR can mediate drug-drug interactions either by reducing the therapeutic efficacy or by increasing the concentration of reactive metabolites leading to toxicity (86). PXR may also lead to the so called “cocktail effect” that is the adverse effects caused by several chemicals present at the same time exhibiting low individual toxicities (83, 129). In this sense, it is important to identify compounds that activate PXR to avoid such effect and modify the drug design during early stages. Several projects focused on developing models able to identify PXR agonists: Torimoto-Katori et al. (86), designed a pharmacophore with high accuracies (over 0.7) yet with lower sensitivities suggesting that reinforcing the methods with other in silico methods could help achieve better performances. This was done by Cui et al. (85), who combined pharmacophore and docking method to detect ingredients from herbs able to activate PXR in order to avoid herb-drug effects. Pharmacophore models achieved similar performances as the model described before with sensibilities equal to 0.54 and specificities of 0.8. Interestingly, adding docking methods on top of pharmacophore models enhanced the performances to a detection rate of 0.6 i.e. the ratio of positive hits among all the database compounds. Three other groups built QSAR models to identify potential activators of PXR. The first one (84), achieved performances of R² = 0.64 and was applied to identify 16 novel activators of PXR. Dybdahl et al. (83), built a model associated with a sensitivity of 82% and a specificity of 85% that was used to identify potential PXR activators in a database of environmental chemicals. Interestingly, these molecules were also linked to cause adverse effects such genotoxicity or teratogenicity for examples.
3.2.4 Liver X receptor
LXR presents two isoforms (LXRα and LXRβ) that are both ubiquitously expressed (65, 67, 130–132). LXR serves primarily as a reverse cholesterol transporter within the lipid metabolism, protecting cells from cholesterol excess (66, 67) and is involved in many physiological processes. Thus, LXR represents a promising therapeutic target for cardiovascular diseases, dyslipidemia and cancer treatment (66). However, it has been shown that LXRα activation can lead to undesired lipogenic effects like increased hepatic lipogenesis or liver steatosis whereas LXRβ activation does not and can even reduce them (67). Designing LXRβ selective compounds to treat dyslipidemia appears as a promising strategy yet difficult to achieve due to the high similarity between both isoforms LBD. For this reason, only two projects among the reviewed ones were dedicated to the identification of LXRβ selective agonists whereas the remaining two others did not make any distinction. Both projects used a virtual screening workflow based on a combination of LB and SB methods. In the first article, Chen et al. (67) built a selective LB model using an association of Kohonen maps and stepwise multiple regression. The model relied on the structures of newly reported dual agonists and was associated with R2 equal to 0.837 and 0.843 for train and test set respectively. This model was then used to perform predictions of potential selective ligands from the ZINC database falling within the applicability domain of the model. A promising compound was found with a predicted pEC50 = 7.0 for LXRβ and pEC50 = 6.095 for LXRα and was used as template to design potential inhibitors. The latter molecules were incorporated to a docking analysis to better understand the underlying mechanism of the selective activity. Similarly, the second article (65) was also a combination of LB (QSAR) and SB (docking) methods to firstly unravel new selective LXRβ ligands and then analyze the interaction mode using docking studies. No toxicological study related to LXR was collected during our bibliographic search.
3.2.5 Constitutive androstane receptor
CAR is involved in regulation of the transcription of genes encoding for the metabolism of xenobiotic and steroid (133). It is highly expressed in the liver and to less extend the small intestine. Although structurally similar to other NR, the LBD of CAR contains particular residues and motifs and thus present an original conformation. The CAR LBD in its unbound form present a similar conformation to those of other NR when bound to their endogenous ligands. Thus, in the absence of ligand CAR can recruit coactivators. CAR agonist compounds, referred to as “phenobarbital alike”, can increase coactivator recruitment and thus promote the expression of cytochrome P450 enzyme and other proteins involved in metabolism of xenobiotic compounds. Besides the agonist compounds, inverse agonists, such as the androstane metabolites, are also able to bind to CAR LBD. Upon binding to CAR, these compounds inhibit the CAR constitutive activity through the release of coactivators (134, 135). CAR is considered to be a sensor to several xenobiotics including EDCs such as phthalates and triclocarban but it is also considered as an interesting therapeutic target for metabolic diseases such as type 2 diabetes (79, 80). Only few attempts to develop in silico models for CAR were found (79, 80, 100). One therapeutic study was conducted with the dual objective of predicting CAR agonist compounds and collecting knowledge about CAR/ligand interactions. To do so, Lee et al. (79) developed a machine-learning model based on pharmacophoric descriptors with good predictive power with accuracy values equal to 0.875 and 0.854 for the training and test sets, respectively and MCCs values equal to 0.744 and 0.701 for the training and test sets, respectively. Additionally, the group identified the critical elements involved in the binding affinity with CAR. Additionally, two toxicological studies that aim to understand the effect of EDCs on the CAR were also retrieved.
3.2.6 Farnesoid X receptor
FXR is expressed in the liver, the kidney, the intestine, and the adrenal glands. It regulates the metabolism of glucose and lipids and the maintenance of the bile acids homeostasis. It is thus a suitable therapeutic target for the prevention and treatment of metabolic syndrome, dyslipidemia, atherosclerosis, and type 2 diabetes (59). Additionally, some exogenous compounds able to bind to FXR can induce the dysfunction of the receptor and are suspected to be responsible for liver toxicity and hepato-biliary injuries (61). In this review, 4 in silico models dedicated to the identification of FXR modulators for the treatment of hepatic and metabolic disorders have been analyzed. As a limited number of diverse known FXR modulators was available, SB methods used to be elected to identify potential therapeutic compounds. 3 studies present the generation of FXR SB pharmacophore models and their use in prospective screening in combination with experimental testing to identify FXR agonist hits (57, 59, 60). For example, in the study of Schuster et al. (58), a set of SB pharmacophore models was generated and theoretically evaluated by calculating the enrichment factors using several data sets. The combination of all pharmacophore models was able to retrieve 87.8% of a list of FXR actives but the performance obtained varied according to the model and to the data set studied. EF values were used to produce pharmacophore models ranking for each data set and the 3 most suitable pharmacophore models were selected accordingly for prospective screening. In complement, 3 LB approaches were described in the literature. The Tox21 FXR agonism and antagonism assay data were used in 2 different studies to obtain respectively FXR disruptors model using machine learning methods (61) and separated FXR agonism and FXR antagonism models using a deep learning approach (100). Both studies present high predictive accuracy with area under the ROC curve values reaching 0.8. The third study focus on different machine learning methods (counter-propagation artificial neural network, similarity of 3D pharmacophore feature distributions method and k-nearest neighbor learner) that were optimized and combined to identify new FXR modulators molecular frameworks (62). This ensemble machine learning approach was used in a prospective screening of 3 million commercially available compounds and enable the discovery of 4 new experimentally validated FXR agonist and 2 FXR antagonist compounds with original molecular frameworks.
3.2.7 Thyroid hormones receptors
TR, are regulated endogenously by the thyroid hormones (TH) that play major role in metabolism and growth processes (136). The 2 isoforms, TRα and TRβ, are expressed differently in tissues and play different roles in the TH signaling (137). Despite the great potency of TR as therapeutic target in the field of dyslipidemia and liver diseases, the development of TR modulators has been limited by selectivity problems and associated undesirable side effects on heart and bone. TRα activation being associated with the cardiac effects of TH, TRβ selective compounds are currently investigated for the treatment of metabolic and brain disorders (138). Several environmental chemicals have also been documented as TR modulators, some of them being suspected to be EDCs. It is notably the case of Hydroxylated polybrominated diphenyl ethers that present structural similarity with endogenous TH and may interfere with the TH binding to TR (87). TR are thus largely studied in the EDCs context (139, 140). In this review, 10 models dedicated to TR are listed, including 3 models specifically developed for TRβ and 4 tools to predict potential EDCs that include SB (12, 104, 105) and LB (19, 100) models for a panel of NRs and not only TR. It is to note that these LB initiatives trained their model using the TR Tox21 dataset and that the associated performance was among the lowest of all studied receptors. Among the TR focused studies, Zhang et al. (89) presented a virtual screening protocol combining ensemble docking and MM-GBSA rescoring to identify TRβ ligands. This protocol was developed and evaluated using the TRβ DUD-E data set and was associated with an AUC value of 0.865 and an EF10% value of 7.418. This protocol was then applied in a prospective virtual screening of an indoor dust contaminants inventory but as it was developed using the TRβ DUD-E dataset, it could also be applied to identify potential TRβ binders for therapeutic applications. Among the remaining studies, it is to note that one was dedicated to the classification of TR agonist and antagonist compounds using ML methods but was not applicable to the classification of TR binders and TR non binders (88), and 2 studies using QSAR models were associated with good predictive performance of TRβ agonist compounds (90) and TRβ binders (91) respectively, but both suffer from a limited (<30) number of compounds included in the training and test sets.
3.2.8 Vitamin D receptor
VDR is expressed in various tissues especially in the gastrointestinal tract and the kidneys. It plays a major role in the regulation of vitamin D thus controlling the calcium homeostasis, the bone mineralization and remodeling and immune pathways. Additionally, VDR is responsible for the detoxification of both endogenous ligands and xenobiotics. The natural ligand of VDR is the active form of vitamin D called D3. It has been reported in several studies that the lack of Vitamin D leads to hypocalcemia and hypophosphatemia resulting in chronic kidney disease (CKD) also found in patients under chronic hemodialysis, mineral-bone disorders, osteoporosis and cancer (92, 94, 141, 142). In this review, we collected 3 articles that aimed at identifying novel VDR modulators for therapeutic purpose. 2 articles both used a combination of LB (pharmacophore‐based 3D‐QSAR models) and SB (docking and molecular dynamics) methods to identify respectively VDR inhibitors (93) and VDR agonists (94). Both models are associated with good correlation value (R²=0.8869 and R²=0.8676 respectively) and predictive score on the training set (Q²=0.8870 and Q²=0.8523 respectively). However, the low diversity and limited number of compounds used to train the model in the first study (93) and the difficulty to understand and thus reproduce the protocol used in the second one (94) may limit the applicability of these 2 models. In the third study (92), de novo design, docking and molecular dynamics was used to design new potential VDR agonists. However, except a redocking evaluation of D3, the performance of the protocol was not assessed retrospectively and no experimental test was conducted to validate the predicted hits. Additionally, one article (100) used data from the Tox21 initiative to build deep learning toxicological models for 35 NRs including VDR.
3.3 Monomeric orphan receptors
The members of this category of NR are characterized by an incomplete knowledge about their endogenous ligands and their ability to be activated as a monomer (or homodimer) by opposition to the orphan receptor that are partners to RXR (108). 3 NR studied during this review process and acting as monomers belong to this category (Table 3).
3.3.1 Estrogen related receptors
ERR are orphan receptors, i.e. no endogenous ligands have yet been characterized for the ERR. They do not bind any natural hormones, not even estrogen despite their names (which has been chosen regarding the high sequence similarity in the DNA-binding domain between ERR and ER) but they do bind some synthetic estrogenic compounds (143). ERR are present in the human body under three main isoforms, ERRα, ERRβ and ERRγ that modulate cartilage development, mitochondrion organization and T-cell activation and differentiation. ERRα has particularly been investigated due to its tight similarity with ERα. Common DNA regions can be activated by both ERRα and ERα (144) and they shared common co-activators which may explain the fact that ERRα is involved in estrogen-related diseases. However, the lack of known endogenous ligand has impaired the establishment of protein-ligand interaction profile and the discovery of synthetic ligands. However, the Tox21 program has provide a huge amount of binding and activity data for ERR that could be used to build prediction models. Klimenko et al. (95), proposed a ligand-based (LB) approach to identify ERRα agonists by combining QSAR models. Each QSAR model is specific of a particular biological endpoint aiming at identifying potential ERRα agonists. The models were selected according to the balance between several statistical parameters i.e sensitivity, specificity, Accuracy, balanced accuracy, PPV and NPV. Other investigated ERRα ligands are inverse agonist and antagonist compounds that can be used for cancer patients with resistance to hormonal therapy. In order to identify such compounds, Chitrala et al. (96) used a library from KEGG COMPOUNDS, containing an ensemble of metabolic compounds, pharmaceutical and environmental compounds. This library was pre-filtered to select 8 compounds presenting a similarity score greater than 0.3 with an antagonist compound co-cristallized into the ERRα binding site. The ERRα structure in its antagonist conformation was then used to dock these compounds. Unfortunately, no biological evaluation of the proposed hit compound was achieved.
3.3.2 Liver receptor homolog 1
LRH-1, also called NR5A2, plays an essential role in the well-functioning of the liver, the pancreas and the intestines by controlling the level of cholesterol, bile acids and pancreatic enzymes. LRH-1 is also involved in cell differentiation and associated with key developmental pathways. However, dysregulated LRH-1 activity and unexpected re-activation of the previously mentioned developmental pathways has been linked with breast, endometrial, intestinal and pancreatic malignancies (97). Limited data is available for LRH-1. In particular, LRH-1 is still classified as an orphan receptor, but several studies pointed out phospholipid species as possible endogenous ligands. This has impacted the development of LRH-1 modulators and of in silico models dedicated to LRH-1. Only one article dedicated to LRH-1 was found during the review process (97). This article published in 2013 presents a prospective structure-based screening with the aim of identifying the first synthetic LRH-1 antagonist compound. Using a docking method, 2 new LRH-1 antagonists were discovered and proposed to be used as a probe to help decipher LRH-1 mechanism of action.
3.3.3 Retinoic acid-related orphan receptor
Three subtypes of ROR exist, namely RORα, RORβ and RORγ with its two isoforms, RORγ1 and RORγt (RORγ2). Each subtype presents a different pattern of expression, RORα being highly expressed in the brain, RORβ in the central nervous system, RORγ1 in the liver, the adipose tissue, the kidney, the small intestines, and the skeletal muscles and RORγt being exclusively expressed in cells of the immune system (145, 146). RORs are implicated in several key physiological functions. In particular, RORγt plays a major role in the differentiation of T-helper 17 (TH17) cells that produce the cytokine IL-17, itself involved in several diseases such as psoriasis, multiple sclerosis, rheumatoid arthritis and type 1 diabetes (98, 99). Additionally, the inhibition of RORγt stimulates the AR gene transcription and can be a strategy to follow for prostate cancer treatment (99). Identifying RORγt modulators emerges as a promising therapeutic strategy for all these conditions. However, until very recently, the known RORγt modulators were all sharing the same scaffold and efforts have been made towards the discovery of new RORγt modulators with original scaffolds. Two articles (98, 99) described SB in silico approaches to identify potent inverse agonists of RORγt that can be used in auto-immune diseases treatment. In the first article, docking and negative image-based methods (NIB) were both first evaluated retrospectively with RORγt experimental data extracted from the ChEMBL database to define docking score threshold and cutoff similarity value, respectively. These 2 methods were then used in parallel to screen a collection of more than 100000 molecules commercially available. Experimental tests validated as RORγt inverse agonists 11 up to the 34 predicted consensus hit compounds with an original scaffold.
3.4 Projects targeting several NR
Besides articles that were solely dedicated to a specific NR presented above, other projects focused on ensemble of NRs to provide more global NRs ligand identification models. Except one article (106), all these projects fall under a toxicological scope with the aim of identifying potential EDCs and understanding their mechanism of action. The most targeted NR combination is ER and AR due to extensive literature and data available. For example, Li et al. (31) studied the ability of some EDCs to present a dual activity by interacting with both AR and ER. To do so, they constructed ER binding models using a QSAR approach and these models were used to screen a database of AR antagonist compounds. It is to note that some of these projects are made available as webservers and software (12, 103–105). Open VirtualToxLab (105) is a software that estimates the toxicological potential of the screened compounds by relying on an automated combination of flexible docking and free energy calculations. Open VirtualToxLab focuses on 16 biological targets among which are 9 NR: AR, ERα, ERβ, GR, LXR, MR, PPARγ, PR, TRα, TRβ. The proof of concept of this software was established with a series of 2564 compounds yielding accurate predictions [C.f. Table 2 of (105)]. Endocrine disruptome (104) is a freely available open-source project that allows users to test the ED potential of their compounds against 14 NR involved in several biological processes: AR, ER α, ER β, GR, PPARα, PPARβ, PPARγ, PR, RXRα, and TRα and TRβ. This webserver is based on docking models elaborated for each NR individually. The major drawback of this tool is the use of DUD-E decoys to validate the protocol. These decoys are putative inactive compounds, and not experimentally validated inactive compounds, which may bias the evaluation of the performance of the models. ProTox-II (103) is a webserver predicting the potential toxicity of small molecules by using 33 models of different toxicity endpoints. Among these endpoints are AhR, AR, ER and PPARγ signaling pathways. The models for each one of these NRs performed with high accuracy on the Tox21 dataset [C.f Table S2 of (103)].
3.5 Related receptors: Aryl hydrocarbon receptors
Although AhR does not belong to the NR superfamily, this receptor displays functional and structural similarities with the members of this family, particularly the presence of a DBD and a LBD activated upon ligand binding (147). During the review process, we noticed that AhR is often associated with other NR in toxicological studies and in the EDCs context and we decided to list also the in silico studies dedicated to this receptor (Table S1). AhR is expressed in various tissues such as the lung, liver, kidney, skin, sleen and placenta. It can be activated by several hazardous chemicals such as polycyclic aromatic hydrocarbons (PAHs) and persistent organic pollutants (POPs) especially dioxins and polychlorinated biphenyls (PCBs). These chemicals result from combustion and are massively found in the air (148). Due to its pivotal role as an environmental pollution sensor and mediator, AhR emerges as a major target for toxic compounds which is illustrated by the predominance of reported toxicological projects (8 toxicological among 10 in total). Most of the data used in these projects are collected from the literature and these studies are in their large majority dedicated to specific chemical series of environmental compounds that have been proved experimentally to be able to interact with AhR (71, 149, 150). The corresponding models are associated with high predictive power, but their applicability domain is relatively limited. Additionally, data from the Tox21 project was also used with both SB and LB methods. However, the application of SB methods is limited by the fact that the 3D structure of this receptor has not been solved yet. A preliminary homology modelling step is thus necessary in all the studies relying on SB methods to obtain a model of the AhR LBD. AhR belongs to the PAS-domain protein group (151) and usually the structure of the PAS-B of the Hypoxia Inducible Factor 2 α (HIF-2α) is used (152, 153) sharing 31% of sequence similarity and 52% identity with the target considered as the highest sequence identity and similarity. More recently, potential therapeutic applications of AhR modulation have emerged. AhR activation has been shown to be involved in hematopoiesis and inflammation process especially the production of inflammatory cytokines whereas AhR repression has been shown to be beneficial in anti-cancer therapies especially for glioblastomas and breast cancers (154). Two therapeutic projects were analyzed in this review, the first being a LB model aiming to discover new AhR antagonists. For this purpose, Parks et al. used successively the Rapid Overlay of Chemical Structures (ROCS) and the electro-static overlap to identify one compound that yield promising experimental results in three in vitro and in vivo AhR-dependent assays (154). The second therapeutic project applied a SB protocol to decipher the molecular mechanisms behind the AhR activation or inhibition (152). In this article, several entries for the template protein were used to simulate the flexibility and plasticity of the binding domain and homology models were selected and used to perform the docking of 10 representative AhR agonists from different chemical classes. The outcome of docking coupled with MD simulations allowed the analysis of the predominant poses and thus the description of ligand binding and the identification of the interacting residues within AhR.
4 Studied methods and associated data
4.1 Computational methods
Along this review process, we analyzed models dedicated to the identification of NRs ligands obtained with a very large spectrum of in silico methods categorized in two approaches i.e SB and LB as shown in Figure 3.
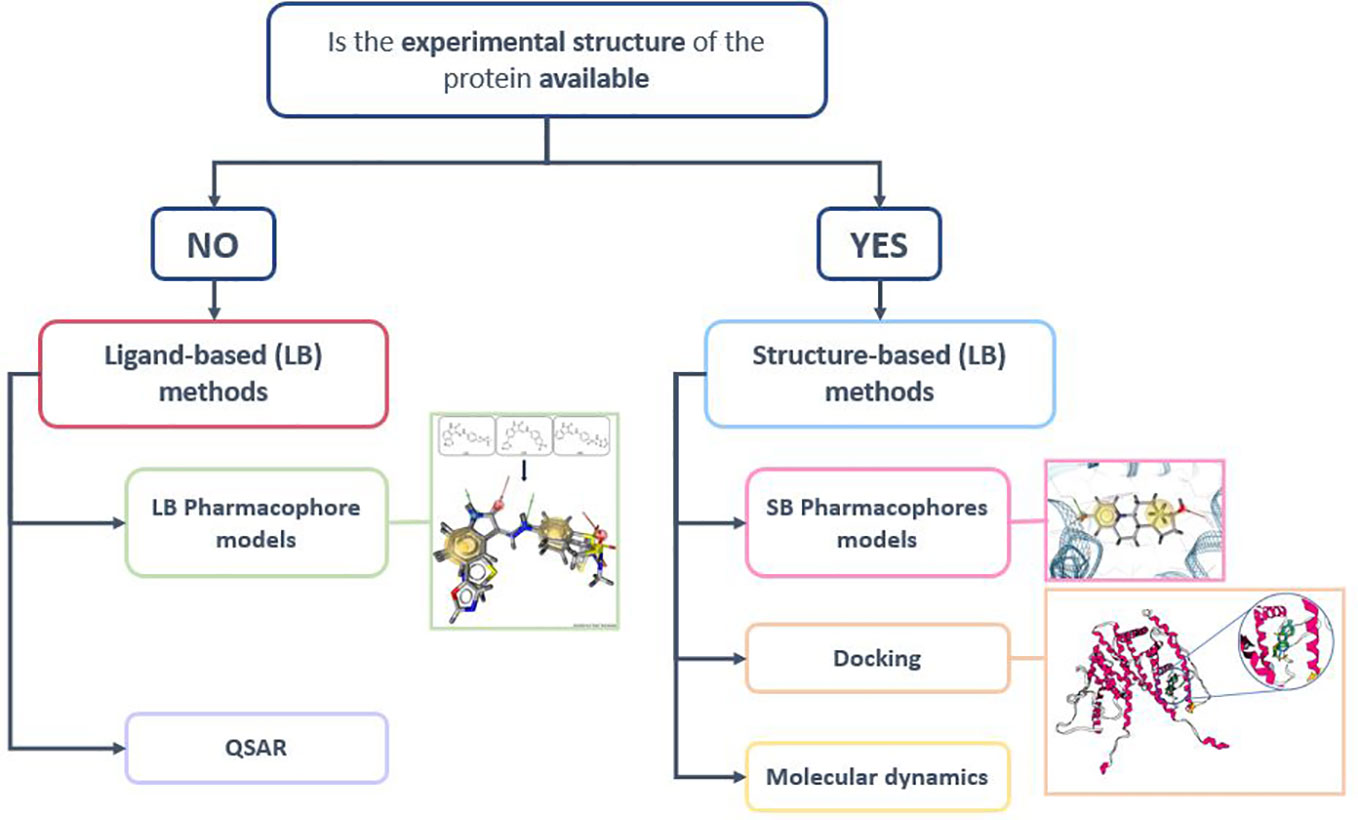
Figure 3 Structure-based (SB) and Ligand-based (LB) screening methods (155).
4.1.1 Molecular dynamics simulations
MD simulations are used to study the dynamic of a biological system as a function of time. The movements of the system are computed through the integration of Newton’s equation of motion. MD simulations can thus be used to consider the conformational changes associated with the NRs LBD. Indeed, the NRs LBD is characterized by its hydrophobicity and flexibility allowing binding of ligands of different sizes and shapes. NRs structures are subject to various modifications occurring after several events such as the DNA transcription or the binding of the ligand (15). The helix H12 is known to modify its orientation according to the bound-ligand profile. MD can thus be used to span the NRs flexibility and deliver unbiased theoretical structures, but also to assert the stability of a ligand-protein complex and study the time of residence of the ligand. In the reviewed articles, MD has been used for several projects relevant to AR, ER, LXR, PPAR, TR and VDR always in complement of another computational method such as QSAR (74, 150) or pharmacophore models (93, 94) and frequently prior or after docking simulations (66, 75, 152).
4.1.2 Docking
Protein-ligand docking is a SB method used to predict the ability of a compound to interact with a target. Docking methods used a search algorithm that generate multiple potential conformations of the molecular complex and a scoring function to rank them. Docking can be used to propose structural hypotheses on the dominant binding mode of a compound, to screen large libraries of compounds and to rank them according to docking scores. In this way, docking is used to prioritize and reduce the number of compounds that should be experimentally tested (156). Docking methods can be applied to any target for which an experimental 3D structure is available. Consequently, docking methods were largely employed to develop predictive models of NR-ligand interactions and for each of the 14 reviewed NR subfamily, at least one project using a docking method was available. However, the docking protocol used must be finely tuned for each NR. A first important criterion is the rational selection of the initial docking structure(s) since the NRs LBDs undergo significant conformational changes upon ligand binding, dependent of the pharmacological profile of the ligand. NRs agonist compounds tend to stabilize the interactions between the residues of helix H12 and other helices (H3, H5 and H11) of the LBD, creating a lid shape of the H12 over the ligand. In contrast, other compounds (antagonists or partial agonists) are not able to stabilize the previous interactions and the observed repositioning of the H12 is different. The choice of an agonist-bound or antagonist-bound structure may thus impact the docking performances in predicting NRs ligands binding (15). This is particularly important for docking protocols aiming to discover therapeutic compounds targeting the NRs as a specific pharmacological profile is usually targeted and the corresponding agonist- or antagonist-bound structure should be selected. In contrast, EDCs can present different pharmacological profiles and it is thus important to evaluate both type of structures to ensure that the screening is able to retrieve all EDCs regardless of their pharmacological profile. In the reviewed articles, two main docking approaches have been listed: single and an ensemble structure docking. In the ensemble structure docking approach, a ligand is successively docked against several protein conformations (Multiple PDB entries or snapshots extracted from MD simulations or a gaussian transformation of the atom localization) and the results are post processed to only keep the best score among all the structures. This approach provides a better picture of the protein flexibility but is more computationally and time expensive and it is not always associated to enhanced docking performances (157).
4.1.3 Pharmacophore modeling
A pharmacophore model is defined by the IUPAC as “an ensemble of steric and electronic features that is necessary to ensure the optimal supramolecular interactions with a specific biological target and to trigger (or block) its biological response” (158). Two types of pharmacophore models exist (1): LB pharmacophore models established upon the superimposition of known active molecules and the retrieval of the common chemical features that are necessary for the bioactivity (2); SB pharmacophore models based on the structure of a protein or more usually of a complex protein-ligand by probing the possible interactions in the macromolecule binding site (159).
In total, 17 reviewed articles used pharmacophore modelling. The generated pharmacophore models are in a large majority combined with other computational models (QSAR and docking models) and only two publications present a protocol combining SB and LB pharmacophores (76, 79). It is to note that both LB and SB pharmacophores taken individually have a predictive power limited by the data used to train the model, defining their applicability domain. The protocol combining pharmacophore models with other in silico methods are described in the reviewed articles and may help overcoming this limit.
4.1.4 QSAR models
QSAR (Quantitative Structure Activity Relationship) is a LB method relying on statistical models to predict the biological activity of compounds. Since their introduction, QSAR models have known several evolutions from simplistic linear and classification models to the use of more elaborated algorithms like artificial neural networks (ANN) and deep learning (DL). In total, 48 of the reviewed articles used QSAR models with the majority (19) adopting classical 2D machine learning methods like Random Forest or SVM. The remaining studies present models obtained using ANN (4), DNN (3) or 3D QSAR methods such as CoMFA and CoMSIA (7). The classical QSAR models rely on a set of descriptors that encode chemical structures, describe physiochemical properties and that can be obtained from quantum chemical calculations (14, 88). Because a huge number of predefined descriptors can be generated, it is important to correctly select the ones that will accurately translate the link between the chemical structure and the associated activity (160). A classical approach is illustrated in the publication of Wang et al. (88) in which QSAR models dedicated to the identification of agonist and antagonist ligands of TR are presented. In this study, the software Dragon was used to initially generate over 1600 descriptors. This number was then reduced by discarding the descriptors highly correlated and selecting only those directly related to the structure, intuitive and easy to understand. Conversely, DL methods emancipate from descriptors computation and selection. Instead, DL methods ensures automatic feature extractions during the training phase. DL methods are thus particularly adapted for areas where existing predefined descriptors have not been crafted yet like macrocycles or the modeling of therapeutic peptides (161). However, DL methods also present some drawbacks, the first being the so-called “black box effect”. DL methods compared to more simplistic algorithms are not easily understandable and deciphering the molecular mechanisms associated with the prediction may be a tough task. Moreover, DL requires higher computing resources which can be a limiting factor.
Similarly to the pharmacophore models, QSAR models’ predictions are only accurate for compounds that are similar to the ones used to train them (21, 45, 162). An applicability domain (AD) can thus be defined with descriptors used to build the QSAR model (21, 25).. Implementing the AD has proven to enhance the confidence in the models by reducing the number of false negatives (25). However, prediction will only be achieved for compounds that are within the limit of the AD, i.e. within a defined descriptors similarity threshold with their nearest neighbors of the training set (45). In order to enhance the chemical space for which prediction can be made, QSAR models can be combined with other ones with complementary ADs or with a SB model such as docking (67, 68, 74, 81).
4.1.5 Data splitting
An important step prior the generation of LB models is to split the available ligands data into train and test set (160). The stake of a good data split is to obtain a test set that is somehow representative of the training set chemical space. In most of the reviewed article, data was split randomly as it is commonly done to avoid biased evaluation of the model. However, this randomness may lead to an uneven distribution of active and inactive compounds between the train and the test set especially if the active/inactive ratio is initially low. In this sense, Capuzzi et al. (101) build QSAR models relying on two sets of databases: (1) the native Tox21 set of data with a ratio of active: inactive equal to 1:10 and (2) a balanced set generated down-sampled from the inactive data of the Tox21 native dataset i.e. for each active compounds an equivalent inactive was selected either randomly or based on the highest Tanimoto score within the inactive pool of compounds. It is to note that using the balanced set leads to a decrease in the accuracy of the model in comparison to when they used the native (unbalanced) dataset. Randomly splitting data may also result in a test set that is not representative of the train especially when the initial data are structurally diverse. Wang, Xing et al. (88) used self-organizing map (an ANN algorithm) to project an ensemble of structurally diverse compounds on a low dimensionality grid. This helped visualizing the space and the selection of a test set representative of the overall dataset and lead to average accuracies of 83.1–97.2%. In other studies, the trade-off between randomness and unbiased subdivision was achieved with bootstrapping. Several splits were done, and models were built an evaluated with the associated train/test output. The split with the best results was kept for the following model optimization (29, 163).
4.1.6 Combinations of methods
It is to note that each computational method has limitations. For example, QSAR methods are not suited on their own to be used for HTS. They are more efficient at generating focused data and requires exhaustive model training and validation steps. Moreover, QSAR and pharmacophores modelling share the common particularity of being only efficient on molecules falling within a domain of applicability (162). A crucial point in the docking workflow is the choice of the scoring function as no universal scoring function exists yet (164). Each individual method can be at the origin of new structural information that can be combined to enable a better understanding of the mechanism of action. A solution to overcome each method limitations and take advantage of their complementarity is to use a combination of methods (28, 165, 166). Computational methods can be combined in integrative approaches using hierarchical or consensus screening (27–29, 48, 60, 100, 163) (for further references c.f. Table 1–4).
4.2 Databases
A chemical database (DB) is an organized collection of compounds with relevant information on their chemical structures together with activity data collected from in vivo and/or in vitro experiments and sometimes in silico predicted activities. Several DB dedicated to NR exist and we decided in this review, to focus on the ones available in open access as presented in Table 5.
In silico studies focusing on NR are numerous and the choice of the databases to use to construct predictive models depends on the aim of the project. Moreover, and especially for drug design projects, some research teams chose to use in house databases i.e. a collection of compounds issued as a result of experimental work in the laboratory. These databases can be composed of one or several chemical series originating from a single hit or a known drug scaffold or, in some cases focused libraries. Finally, some projects rely on several existing databases, either to combine the molecules resulting in larger databases for the same purpose (therapeutic or toxicological) or use a database for the training step and another for the external validation. For example, Réau et al. (29) developed a docking and a pharmacophore modeling strategy for identifying AR agonist compounds relying on the NR-DBIND data and used as an external validation set the compounds from the tox21 challenge.
4.2.1 Assay consideration
In the scientific literature, biological data available for NRs ligands represent various assays endpoints sometimes measured from different laboratories and companies. Indeed, two main assays were used in the reviewed studies to assess NRs ligands potency: binding assay and gene reporter assay (34). These assays attributed to each compound a quantitative value that may vary according to the laboratory in charge of the experiment. In the in silico modelling field, the experimental data are used to compute predictive models for molecules with no related experimental information. For some methods such as QSAR, the numerical value associated with the experimental test can directly be integrated to construct the models. For other method, such as docking and pharmacophoric modeling, these data usually need to be converted into a binary variable with 2 possible values: “active compounds” and “inactive compounds”. This is necessary to optimize and select the prediction models able to distinguish between both categories. Indeed, to evaluate the predictive power of a model, the number of correctly predicted active and inactive (TP and TN) as well as the number of wrongly predicted compounds (FP and FN) are used to compute metrics such as sensitivity, sensitivity and enrichment for example. The binarization of the data is a crucial but not straightforward step because a threshold must be defined to separate the data. Additionally, because various biological endpoints are considered, the concordance between the thresholds of activities defined for each assay should be assessed. Lunghini et al. (167) defined the “degree of agreement” parameter to pinpoint the differences between various assays used to label compounds that interact with ER and AR in the context of EDCs models. To do so, they performed multiple pairwise comparisons of various binding assays result among 4 data sources for ER binding compounds and 3 data sources for AR binding compounds. The degree of agreement was calculated as the average number of sources agreeing on a given label for each compound. Their analysis showed that there is a lack of concordance between experiments for 42% of the compounds. This study highlighted the danger of merging assays with different biological meanings (167) and considering the positive outputs as interacting compounds regardless of the mechanism of action. However, it is a commonly used protocol, often driven by the lack of appropriate data or the blurry definition of the mechanism of action. This is especially the case in the toxicological context of the study related to EDCs and in this review, we came across several studies performing the merging of data obtained from different assays and different sources. Some studies, aware of this issue, also developed various approaches to limit the associated bias. Sakkiah et al. (56) calculated the concordance between the train and the test set since originated respectively from binding and amplification assays. Manganelli et. al (28), compared the train and the test data to define concordance and exclude a part of the data that falls under a “dangerous” segment. Finally, Zhang et al. (128) normalized the activity of their test set to their training data when constructing their models.
4.2.2 Data balance
Along with active compounds, it is also important to construct, optimize and evaluate NRs prediction models to include negative data (168). Positive data are usually resulting from biological or cellular in vivo assays but, negative data has long been constituted by presumed inactive compounds (121). Since negative data have a crucial impact in influencing the performance of a model (168), recent efforts have been made to include carefully selected and experimentally validated inactive compounds [NR-DBIND (169)]. An additional issue is the proportionality between active and inactive compounds in DB. When collecting data from scientific literature, very few inactive compounds are retrieved. Conversely, in databases presenting high throughput screening results, inactive compounds usually outnumbered the active ones. In this case, the active substances represent a low proportion out of all tested chemicals (95). A performant model is dependent on clean, diverse and accurate data (95, 165), and unbalanced data (either in favor of the active or the inactive counterpart) can impact prediction models building (55). Clustering methods can be used to select smaller subsets of representative active or inactive compounds. For example, Another solution is the use of a structural similarity filter based on fingerprints and the application of a similarity metric cutoff (for example Tanimoto) in order to select sparse compounds to scour the entire chemical space (170). In the case of unbalanced data sets, the classical metrics are not the best suited to evaluate the predictive power of a model. In this case some metrics like the MCC and the F1 score can help in assessing the ability of a model to correctly predict each category of compound.
4.3 Level of reproducibility
Reproductible science is a quality standard that the scientific community values as it helps producing high quality, reliable and efficient research project (171). It has been proven that reproducibility together with methods, reporting, dissemination, evaluation and incentives are key elements for the scientific process (172). However, it has also been asserted that the field of preclinical research suffers from the inability to replicate findings published in high-profile journals (171). Although it is difficult to exactly replicate results in biology systems due to their inherent variability, some recommendations on good practice could be listed to alleviate the trustworthiness of the scientific work and the validity of the major conclusions (171). Reinforcing the policies on data and code sharing is one of these recommendations (171, 173).
Through this review, we decided to evaluate this point in the NRs in silico research field. For each reviewed model, the level of reproducibility was graded according to the availability of databases and the model procedure’s parameters and/or code. The “high” grade was used to describe models providing both components within the article, the “medium” was associated with articles with partial available data. Finally, the reproducibility was described as “low” for models where no data were available. For most of the reviewed articles’ reproducibility was rated “medium” (52 out of 89 articles) and only a low proportion was described to have a “high” reproducibility (16 out of 89). These results focused on the NRs are in concordance with other large-scale studies (173). For example, in a study of 2011, the analysis of 500 papers published in the top 50 journals across scientific fields showed that only 9% of these papers enclosed full primary data (171). The reinforcement of collaborations between research teams following the example of COMPARA (20) and CERAPP (36) consortiums or the Tox21 challenge (174) can improve the reproducibility since it creates accountability in exchange of a benchmarked data (172). However, according to our classification, no particular tendency was observed over the 10 year covered by this review neither towards increase of the number of articles labeled with “high” reproducibility nor towards decrease of the number of articles associated with “low” reproducibility.
5 Conclusion
Nuclear receptors are a large family of transcription factors involved in several biological process and their impairment result in several pathologies. Moreover, this protein family can be targeted by toxic compounds leading to the disruption of the normal functioning of NRs and especially the disruption of the endocrine system by a family of compounds called endocrine disrupting chemicals or EDCs. Being in the center of both therapeutic and toxicological concerns, NRs are widely studied to find new cures but also to unravel the potential toxicity of environmental compounds such as pesticides, cosmetics or additives. In the last decades and with the emergence of bioinformatics and virtual screening techniques, computational models dedicated to NR were developed. The computational capabilities were put to use to interpretate and analyze the experimental data and build predictive models to unravel new drug hit and forecast potential toxicants. This article is a review of the studies dedicated to NR ligands prediction for both therapeutic and toxicological purposes, published in the last decade. 89 articles concerning 14 NR subfamilies were carefully read and analyzed in order to retrieve the most commonly used computational methods to develop predictive models, to retrieve the databases deployed in the model building process. Some issues facing the model building process were addressed like the assays endpoint discrepancies and data balance were also addressed and how authors managed to overcome them. This review emerged from the need to identify most of the in silico initiatives undertaken on NR and can be used as a starting point for future investigations on the subject not only to appreciate the importance of NR in both therapeutic and toxicological fields, but also to learn from previous experiences, encourage the elaboration of more accurate predictions and motivate collaborations.
Author contributions
Conceptualization: AS, MR, and NL. Methodology: AS, MR, and NL. Validation: NL, MR, and MM. Formal analysis: AS and NL. Investigation: AS. Resources: AS, MR, NL. Data collection and curation: AS. Writing—original draft preparation: AS. Writing—review and editing: AS, MR, NL, and MM. Supervision: NL. Project administration: MM. Funding acquisition: AS, NL, and MM. All authors contributed to the article and approved the submitted version.
Funding
AS is recipient of a MESRI (Ministère de l’Enseignement supérieur, de la Recherche et de l’Innovation) fellowship.
Conflict of interest
The authors declare that the research was conducted in the absence of any commercial or financial relationships that could be construed as a potential conflict of interest.
Publisher’s note
All claims expressed in this article are solely those of the authors and do not necessarily represent those of their affiliated organizations, or those of the publisher, the editors and the reviewers. Any product that may be evaluated in this article, or claim that may be made by its manufacturer, is not guaranteed or endorsed by the publisher.
Supplementary material
The Supplementary Material for this article can be found online at: https://www.frontiersin.org/articles/10.3389/fendo.2022.986016/full#supplementary-material
References
1. Weikum ER, Liu X, Ortlund EA. The nuclear receptor superfamily: A structural perspective. Protein Sci (2018) 27(11):1876–92. doi: 10.1002/pro.3496
2. Zhang Y, Luo X, Wu Dh, Xu Y. ROR nuclear receptors: structures, related diseases, and drug discovery. Acta Pharmacol Sin (2015) 36(1):71–87. doi: 10.1038/aps.2014.120
3. Sladek FM. Nuclear receptors as drug targets: New developments in coregulators, orphan receptors and major therapeutic areas. Expert Opin Ther Targets (2003) 7(5):679–84. doi: 10.1517/14728222.7.5.679
4. Kinch MS, Hoyer D, Patridge E, Plummer M. Target selection for FDA-approved medicines. Drug Discovery Today (2015) 20(7):784–9. doi: 10.1016/j.drudis.2014.11.001
5. Santos R, Ursu O, Gaulton A, Bento AP, Donadi RS, Bologa CG, et al. A comprehensive map of molecular drug targets. Nat Rev Drug Discovery (2017) 16(1):19–34. doi: 10.1038/nrd.2016.230
6. Bartolini D, De Franco F, Torquato P, Marinelli R, Cerra B, Ronchetti R, et al. Garcinoic acid is a natural and selective agonist of pregnane X receptor. J Med Chem (2020) 63(7):3701–12. doi: 10.1021/acs.jmedchem.0c00012
7. Schug TT, Johnson AF, Birnbaum LS, Colborn T, Guillette LJ Jr, Crews DP, et al. Minireview: Endocrine disruptors: Past lessons and future directions. Mol Endocrinol (2016) 30(8):833–47. doi: 10.1210/me.2016-1096
8. La Merrill MA, Vandenberg LN, Smith MT, Goodson W, Browne P, Patisaul HB, et al. Consensus on the key characteristics of endocrine-disrupting chemicals as a basis for hazard identification. Nat Rev Endocrinol (2020) 16(1):45–57. doi: 10.1038/s41574-019-0273-8
9. Combarnous Y, Nguyen TMD. Comparative overview of the mechanisms of action of hormones and endocrine disruptor compounds. Toxics (2019) 7(1):5. doi: 10.3390/toxics7010005
10. Search the TEDX list [Internet]. TEDX - the endocrine disruption exchange (2021). Available at: https://endocrinedisruption.org/interactive-tools/tedx-list-of-potential-endocrine-disruptors/search-the-tedx-list.
11. Audouze K, Sarigiannis D, Alonso-Magdalena P, Brochot C, Casas M, Vrijheid M, et al. Integrative strategy of testing systems for identification of endocrine disruptors inducing metabolic disorders–an introduction to the oberon project. Int J Mol Sci (2020) 21(8):2988. doi: 10.3390/ijms21082988
12. Vedani A, Dobler M, Smieško M. VirtualToxLab — a platform for estimating the toxic potential of drugs, chemicals and natural products. Toxicol Appl Pharmacol (2012) 261(2):142–53. doi: 10.1016/j.taap.2012.03.018
13. Marroqui L, Tudurí E, Alonso-Magdalena P, Quesada I, Nadal Á, Dos Santos RS. Mitochondria as target of endocrine-disrupting chemicals: Implications for type 2 diabetes. J Endocrinol (2018) 239(2):R27–45. doi: 10.1530/JOE-18-0362
14. Chen Y, Cheng F, Sun L, Li W, Liu G, Tang Y. Computational models to predict endocrine-disrupting chemical binding with androgen or oestrogen receptors. Ecotoxicol Environ Safety (2014) 110:280–7. doi: 10.1016/j.ecoenv.2014.08.026
15. Computational approaches to nuclear receptors [Internet] (2012). Available at: https://pubs.rsc.org/en/content/ebook/978-1-84973-364-9.
16. Gellatly N, Sewell F. Regulatory acceptance of in silico approaches for the safety assessment of cosmetic-related substances. Comput Toxicol (2019) 11:82–9. doi: 10.1016/j.comtox.2019.03.003
17. Mazaira GI, Zgajnar NR, Lotufo CM, Daneri-Becerra C, Sivils JC, Soto OB, et al. The nuclear receptor field: A historical overview and future challenges. Nucl Receptor Res (2018) 5:101320. doi: 10.11131/2018/101320
18. Zhao L, Zhou S, Gustafsson JÅ. Nuclear receptors: Recent drug discovery for cancer therapies. Endocrine Rev (2019) 40(5):1207–49. doi: 10.1210/er.2018-00222
19. Sun L, Yang H, Cai Y, Li W, Liu G, Tang Y. In silico prediction of endocrine disrupting chemicals using single-label and multilabel models. J Chem Inf Model (2019) 59(3):973–82. doi: 10.1021/acs.jcim.8b00551
20. Mansouri K, Kleinstreuer N, Abdelaziz AM, Alberga D, Alves VM, Andersson PL, et al. CoMPARA: Collaborative modeling project for androgen receptor activity. Environ Health Perspect (2020) 128(2):027002. doi: 10.1289/EHP5580
21. Li J, Gramatica P. Classification and virtual screening of androgen receptor antagonists. J Chem Inf Model (2010) 50(5):861–74. doi: 10.1021/ci100078u
22. Wang X, Li X, Shi W, Wei S, Giesy JP, Yu H, et al. Docking and CoMSIA studies on steroids and non-steroidal chemicals as androgen receptor ligands. Ecotoxicol Environ Safety (2013) 89:143–9. doi: 10.1016/j.ecoenv.2012.11.020
23. Wu Y, Doering JA, Ma Z, Tang S, Liu H, Zhang X, et al. Identification of androgen receptor antagonists: In vitro investigation and classification methodology for flavonoid. Chemosphere (2016) 158:158:72–9. doi: 10.1016/j.chemosphere.2016.05.059
24. Yang X, Liu H, Yang Q, Liu J, Chen J, Shi L. Predicting anti-androgenic activity of bisphenols using molecular docking and quantitative structure-activity relationships. Chemosphere (2016) 163:373–81. doi: 10.1016/j.chemosphere.2016.08.062
25. Trisciuzzi D, Alberga D, Mansouri K, Judson R, Novellino E, Mangiatordi GF, et al. Predictive structure-based toxicology approaches to assess the androgenic potential of chemicals. J Chem Inf Model (2017) 57(11):2874–84. doi: 10.1021/acs.jcim.7b00420
26. Wahl J, Smieško M. Endocrine disruption at the androgen receptor: Employing molecular dynamics and docking for improved virtual screening and toxicity prediction. Int J Mol Sci (2018) 19(6):1784. doi: 10.3390/ijms19061784
27. Grisoni F, Consonni V, Ballabio D. Machine learning consensus to predict the binding to the androgen receptor within the compara project. J Chem Inf Model (2019) 59(5):1839–48. doi: 10.1021/acs.jcim.8b00794
28. Manganelli S, Roncaglioni A, Mansouri K, Judson RS, Benfenati E, Manganaro A, et al. Development, validation and integration of in silico models to identify androgen active chemicals. Chemosphere (2019) 220:204–15. doi: 10.1016/j.chemosphere.2018.12.131
29. Réau M, Lagarde N, Zagury JF, Montes M. Hits discovery on the androgen receptor: In silico approaches to identify agonist compounds. Cells (2019) 8(11):1431. doi: 10.3390/cells8111431
30. Zorn KM, Foil DH, Lane TR, Hillwalker W, Feifarek DJ, Jones F, et al. Comparison of machine learning models for the androgen receptor. Environ Sci Technol (2020) 54(21):13690–700. doi: 10.1021/acs.est.0c03984
31. Li J, Gramatica P. QSAR classification of estrogen receptor binders and pre-screening of potential pleiotropic EDCs. SAR QSAR Environ Res (2010) 21(7–8):657–69. doi: 10.1080/1062936X.2010.528254
32. Rybacka A, Rudén C, Tetko IV, Andersson PL. Identifying potential endocrine disruptors among industrial chemicals and their metabolites–development and evaluation of in silico tools. Chemosphere (2015) 139:372–8. doi: 10.1016/j.chemosphere.2015.07.036
33. Bhhatarai B, Wilson DM, Price PS, Marty S, Parks AK, Carney E. Evaluation of OASIS QSAR models using ToxCastTM in vitro estrogen and androgen receptor binding data and application in an integrated endocrine screening approach. Environ Health Perspect (2016) 124(9):1453–61. doi: 10.1289/EHP184
34. Tan H, Wang X, Hong H, Benfenati E, Giesy JP, Gini GC, et al. Structures of endocrine-disrupting chemicals determine binding to and activation of the estrogen receptor α and androgen receptor. Environ Sci Technol (2020) 54(18):11424–33. doi: 10.1021/acs.est.0c02639
35. Allen TEH, Nelms MD, Edwards SW, Goodman JM, Gutsell S, Russell PJ. In silico guidance for in vitro androgen and glucocorticoid receptor ToxCast assays. Environ Sci Technol (2020) 54(12):7461–70. doi: 10.1021/acs.est.0c01105
36. Mansouri K, Abdelaziz A, Rybacka A, Roncaglioni A, Tropsha A, Varnek A, et al. CERAPP: Collaborative estrogen receptor activity prediction project. Environ Health Perspect (2016) 124(7):1023–33. doi: 10.1289/ehp.1510267
37. Stojić N, Erić S, Kuzmanovski I. Prediction of toxicity and data exploratory analysis of estrogen-active endocrine disruptors using counter-propagation artificial neural networks. J Mol Graphics Modelling (2010) 29(3):450–60. doi: 10.1016/j.jmgm.2010.09.001
38. Zhang L, Sedykh A, Tripathi A, Zhu H, Afantitis A, Mouchlis VD, et al. Identification of putative estrogen receptor-mediated endocrine disrupting chemicals using QSAR- and structure-based virtual screening approaches. Toxicol Appl Pharmacol (2013) 272(1):67–76. doi: 10.1016/j.taap.2013.04.032
39. Zang Q, Rotroff DM, Judson RS. Binary classification of a large collection of environmental chemicals from estrogen receptor assays by quantitative structure-activity relationship and machine learning methods (2021) 53(12):3244–61. doi: 10.1021/ci400527b
40. Trisciuzzi D, Alberga D, Mansouri K, Judson R, Cellamare S, Catto M, et al. Docking-based classification models for exploratory toxicology studies on high-quality estrogenic experimental data. Future Med Chem (2015) 7(14):1921–36. doi: 10.4155/fmc.15.103
41. Zhang Q, Yan L, Wu Y, Ji L, Chen Y, Zhao M, et al. A ternary classification using machine learning methods of distinct estrogen receptor activities within a large collection of environmental chemicals. Sci total Environ (2017) 580:1268–75. doi: 10.1016/j.scitotenv.2016.12.088
42. Russo DP, Zorn KM, Clark AM, Zhu H, Ekins S. Comparing multiple machine learning algorithms and metrics for estrogen receptor binding prediction. Mol Pharm (2018) 15(10):4361–70. doi: 10.1021/acs.molpharmaceut.8b00546
43. Balabin IA, Judson RS. Exploring non-linear distance metrics in the structure-activity space: QSAR models for human estrogen receptor. J Cheminform (2018) 10(1):47. doi: 10.1186/s13321-018-0300-0
44. Zorn KM, Foil DH, Lane TR, Russo DP, Hillwalker W, Feifarek DJ, et al. Machine learning models for estrogen receptor bioactivity and endocrine disruption prediction. Environ Sci Technol (2020) 54(19):12202–13. doi: 10.1021/acs.est.0c03982
45. Ciallella HL, Russo DP, Aleksunes LM, Grimm FA, Zhu H. Predictive modeling of estrogen receptor agonism, antagonism, and binding activities using machine- and deep-learning approaches. Lab Invest (2021) 101(4):490–502. doi: 10.1038/s41374-020-00477-2
46. Chang YH, Chen JY, Hor CY, Chuang YC, Yang CB, Yang CN. Computational study of estrogen receptor-alpha antagonist with three-dimensional quantitative structure-activity relationship, support vector regression, and linear regression methods. Int J Medicinal Chem (2013) 2013:e743139. doi: 10.1155/2013/743139
47. Ng HW, Zhang W, Shu M, Luo H, Ge W, Perkins R, et al. Competitive molecular docking approach for predicting estrogen receptor subtype α agonists and antagonists. BMC Bioinf (2014) 15 Suppl 11:S4. doi: 10.1186/1471-2105-15-S11-S4
48. McRobb FM, Kufareva I, Abagyan R. In silico identification and pharmacological evaluation of novel endocrine disrupting chemicals that act via the ligand-binding domain of the estrogen receptor α. Toxicological Sci (2014) 141(1):188–97. doi: 10.1093/toxsci/kfu114
49. TilakVijay J, Vivek Babu K, Uma A. Virtual screening of novel compounds as potential ER-alpha inhibitors. Bioinformation (2019) 15(5):321–32. doi: 10.6026/97320630015321
50. Ng HW, Doughty SW, Luo H, Ye H, Ge W, Tong W, et al. Development and validation of decision forest model for estrogen receptor binding prediction of chemicals using large data sets. Chem Res Toxicol (2015) 28(12):2343–51. doi: 10.1021/acs.chemrestox.5b00358
51. Agatonovic-Kustrin S, Alexander M, Morton DW, Turner JV. Pesticides as estrogen disruptors: QSAR for selective ERα and ERβ binding of pesticides. Comb Chem High Throughput Screen (2011) 14(2):85–92. doi: 10.2174/138620711794474097
52. Taha MO, Tarairah M, Zalloum H, Abu-Sheikha G. Pharmacophore and QSAR modeling of estrogen receptor beta ligands and subsequent validation and in silico search for new hits. J Mol Graph Model (2010) 28(5):383–400. doi: 10.1016/j.jmgm.2009.09.005
53. Chen L, Wu D, Bian Hp, Kuang Gl, Jiang J, Li Wh, et al. Selective ligands of estrogen receptor β discovered using pharmacophore mapping and structure-based virtual screening. Acta Pharmacol Sin (2014) 35(10):1333–41. doi: 10.1038/aps.2014.69
54. Tuccinardi T, Poli G, Dell'Agnello M, Granchi C, Minutolo F, Martinelli A, et al. Receptor-based virtual screening evaluation for the identification of estrogen receptor β ligands. J Enzym Inhibition and Med Chem (2015) 30(4):662–70. doi: 10.3109/14756366.2014.959946
55. Niu Aq, Xie Lj, Wang H, Zhu B, Wang S. Prediction of selective estrogen receptor beta agonist using open data and machine learning approach. Drug Des Devel Ther (2016) 10:2323–31. doi: 10.2147/DDDT.S110603
56. Sakkiah S, Selvaraj C, Gong P, Zhang C, Tong W, Hong H. Development of estrogen receptor beta binding prediction model using large sets of chemicals. Oncotarget (2017) 8(54):92989–3000. doi: 10.18632/oncotarget.21723
57. Zarezade V, Abolghasemi M, Rahim F, Veisi A, Behbahani M. In silico assessment of new progesterone receptor inhibitors using molecular dynamics: A new insight into breast cancer treatment. J Mol Model (2018) 24(12):337. doi: 10.1007/s00894-018-3858-6
58. Schuster D, Markt P, Grienke U, Mihaly-Bison J, Binder M, Noha SM, et al. Pharmacophore-based discovery of FXR agonists. part I: Model development and experimental validation. Bioorganic Medicinal Chem (2011) 19(23):7168–80. doi: 10.1016/j.bmc.2011.09.056
59. Grienke U, Mihály-Bison J, Schuster D, Afonyushkin T, Binder M, et al. Pharmacophore-based discovery of FXR-agonists. part II: Identification of bioactive triterpenes from ganoderma lucidum. Bioorganic Medicinal Chem (2011) 19(22):6779–91. doi: 10.1016/j.bmc.2011.09.039
60. Sindhu T, Srinivasan P. Identification of potential dual agonists of FXR and TGR5 using e-pharmacophore based virtual screening. Mol Biosyst (2015) 11(5):1305–18. doi: 10.1039/C5MB00137D
61. Chen Y, Yang H, Wu Z, Liu G, Tang Y, Li W. Prediction of farnesoid X receptor disruptors with machine learning methods. Chem Res Toxicol (2018) 31(11):1128–37. doi: 10.1021/acs.chemrestox.8b00162
62. Merk D, Grisoni F, Schaller K, Friedrich L, Schneider G. Discovery of novel molecular frameworks of farnesoid X receptor modulators by ensemble machine learning. ChemistryOpen (2019) 8(1):7–14. doi: 10.1002/open.201800156
63. von Grafenstein S, Mihaly-Bison J, Wolber G, Bochkov VN, Liedl KR, Schuster D. Identification of novel liver X receptor activators by structure-based modeling. J Chem Inf Model (2012) 52(5):1391–400. doi: 10.1021/ci300096c
64. Heitel P, Achenbach J, Moser D, Proschak E, Merk D. DrugBank screening revealed alitretinoin and bexarotene as liver X receptor modulators. Bioorganic Medicinal Chem Letters (2017) 27(5):1193–8. doi: 10.1016/j.bmcl.2017.01.066
65. Salum LB, Andricopulo AD, Honório KM. A fragment-based approach for ligand binding affinity and selectivity for the liver X receptor beta. J Mol Graphics Modelling (2012) 32:19–31. doi: 10.1016/j.jmgm.2011.09.007
66. Wang X, Lu K, Luo H, Liang D, Long X, Yuan Y, et al. In silico identification of small molecules as novel LXR agonists for the treatment of cardiovascular disease and cancer. J Mol Model (2018) 24(3):57. doi: 10.1007/s00894-018-3578-y
67. Chen M, Yang F, Kang J, Gan H, Yang X, Lai X, et al. Identfication of potent LXRβ-selective agonists without LXRα activation by in silico approaches. Molecules (2018) 23(6):1349. doi: 10.3390/molecules23061349
68. Carrieri A, Giudici M, Parente M, De Rosas M, Piemontese L, Fracchiolla G, et al. Molecular determinants for nuclear receptors selectivity: Chemometric analysis, dockings and site-directed mutagenesis of dual peroxisome proliferator-activated receptors α/γ agonists. Eur J Medicinal Chem (2013) 63:321–32. doi: 10.1016/j.ejmech.2013.02.015
69. Verma N, Chouhan U. Chemometric modelling of PPAR-α and PPAR-γ dual agonists for the treatment of type-2 diabetes. Curr Science (2016) 111(2):356–67. doi: 10.18520/cs/v111/i2/356-367
70. Liu X, Jing Z, Jia WQ, Wang SQ, Ma Y, Xu WR, et al. Identification of novel PPARα/γ dual agonists by virtual screening, ADMET prediction and molecular dynamics simulations. J Biomol Struct Dyn (2018) 36(11):2988–3002. doi: 10.1080/07391102.2017.1373706
71. Nath V, Agrawal R, Kumar V. Structure based docking and molecular dynamics studies: Peroxisome proliferator-activated receptors –α/γ dual agonists for treatment of metabolic disorders. J Biomolecular Structure Dynamics (2020) 38(2):511–23. doi: 10.1080/07391102.2019.1581089
72. Feng XY, Ding TT, Liu YY, Xu WR, Cheng XC. In-silico identification of peroxisome proliferator-activated receptor (PPAR)α/γ agonists from ligand expo components database. J Biomolecular Structure Dynamics (2021) 39(5):1853–64. doi: 10.1080/07391102.2020.1745279
73. Sonawane LV, Bari SB. Ligand-based in silico 3D-QSAR study of PPAR-γ agonists. Med Chem Res (2011) 20(7):1005–14. doi: 10.1007/s00044-010-9428-9
74. Chen KC, Chen CYC. In silico identification of potent PPAR- agonists from traditional Chinese medicine: A bioactivity prediction, virtual screening, and molecular dynamics study. Evidence-Based Complementary Altern Med (2014) 2014:e192452. doi: 10.1155/2014/192452
75. Maltarollo VG, Togashi M, Nascimento AS, Honorio KM. Structure-based virtual screening and discovery of new PPARδ/γ dual agonist and PPARδ and γ agonists. PloS One (2015) 10(3):e0118790. doi: 10.1371/journal.pone.0118790
76. Kaserer T, Obermoser V, Weninger A, Gust R, Schuster D. Evaluation of selected 3D virtual screening tools for the prospective identification of peroxisome proliferator-activated receptor (PPAR) γ partial agonists. Eur J Medicinal Chem (2016) 124:49–62. doi: 10.1016/j.ejmech.2016.07.072
77. Zhang GL, Liu FY, Zhang J, Wang LP, Jia EX, Lv SM. Integrated in silico–in vitro screening of ovarian cancer peroxisome proliferator-activated receptor-γ agonists against a biogenic compound library. Med Chem Res (2018) 27(1):341–9. doi: 10.1007/s00044-017-2060-1
78. Kotha S, Swapna B, Kulkarni VM, Setty. RS, Kumar BH, Harisha R. An in-silico approach: identification of PPAR-γ agonists from seaweeds for the management of alzheimer’s disease. J Biomolecular Structure Dynamics (2021) 39(6):2210–29. doi: 10.1080/07391102.2020.1747543
79. Lee K, You H, Choi J, No KT. Development of pharmacophore-based classification model for activators of constitutive androstane receptor. Drug Metab Pharmacokinetics (2017) 32(3):172–8. doi: 10.1016/j.dmpk.2016.11.005
80. Verma G, Khan MF, Shaquiquzzaman M, Akhtar W, Akhter M, Hasan SM, et al. Molecular interactions of dioxins and DLCs with the xenosensors (PXR and CAR): An in silico risk assessment approach. J Mol Recognit (2017) 30(12):e2651. doi: 10.1002/jmr.2651
81. Kortagere S, Krasowski MD, Reschly EJ, Venkatesh M, Mani S, Ekins S. Evaluation of computational docking to identify pregnane X receptor agonists in the ToxCast database. Environ Health Perspect (2010) 118(10):1412–7. doi: 10.1289/ehp.1001930
82. Matter H, Anger LT, Giegerich C, Güssregen S, Hessler G, Baringhaus KH. Development of in silico filters to predict activation of the pregnane X receptor (PXR) by structurally diverse drug-like molecules. Bioorganic Medicinal Chem (2012) 20(18):5352–65. doi: 10.1016/j.bmc.2012.04.020
83. Dybdahl M, Nikolov NG, Wedebye EB, Jónsdóttir SÓ, Niemelä JR. QSAR model for human pregnane X receptor (PXR) binding: Screening of environmental chemicals and correlations with genotoxicity, endocrine disruption and teratogenicity. Toxicol Appl Pharmacol (2012) 262(3):301–9. doi: 10.1016/j.taap.2012.05.008
84. Ratajewski M, Grzelak I, Wiśniewska K, Ryba K, Gorzkiewicz M, Walczak-Drzewiecka A, et al. Screening of a chemical library reveals novel PXR-activating pharmacologic compounds. Toxicol Letters (2015) 232(1):193–202. doi: 10.1016/j.toxlet.2014.10.009
85. Cui Z, Kang H, Tang K, Liu Q, Cao Z, Zhu R. Screening ingredients from herbs against pregnane X receptor in the study of inductive herb-drug interactions: Combining pharmacophore and docking-based rank aggregation. BioMed Res Int (2015) 2015:e657159. doi: 10.1155/2015/657159
86. Torimoto-Katori N, Huang R, Kato H, Ohashi R, Xia M. In silico prediction of hPXR activators using structure-based pharmacophore modeling. J Pharm Sci (2017) 106(7):1752–9. doi: 10.1016/j.xphs.2017.03.004
87. Chen Q, Wang X, Shi W, Yu H, Zhang X, Giesy JP. Identification of thyroid hormone disruptors among HO-PBDEs: In vitro investigations and coregulator involved simulations. Environ Sci Technol (2016) 50(22):12429–38. doi: 10.1021/acs.est.6b02029
88. Wang F, Xing J. Classification of thyroid hormone receptor agonists and antagonists using statistical learning approaches. Mol Divers (2019) 23(1):85–92. doi: 10.1007/s11030-018-9857-9
89. Zhang J, Li Y, Gupta AA, Nam K, Andersson PL. Identification and molecular interaction studies of thyroid hormone receptor disruptors among household dust contaminants. Chem Res Toxicol (2016) 29(8):1345–54. doi: 10.1021/acs.chemrestox.6b00171
90. Wang FF, Yang W, Shi YH, Cheng XR, Le GW. Structure-based approach for the study of thyroid hormone receptor binding affinity and subtype selectivity. J Biomol Struct Dyn (2016) 34(10):2251–67. doi: 10.1080/07391102.2015.1113384
91. Li F, Xie Q, Li X, Li N, Chi P, Chen J, et al. Hormone activity of hydroxylated polybrominated diphenyl ethers on human thyroid receptor-beta: in vitro and in silico investigations. Environ Health Perspect (2010) 118(5):602–6. doi: 10.1289/ehp.0901457
92. Shen XL, Takimoto-Kamimura M, Wei J, Gao QZ. Computer-aided de novo ligand design and docking/molecular dynamics study of vitamin d receptor agonists. J Mol Model (2012) 18(1):203–12. doi: 10.1007/s00894-011-1066-8
93. Yadav DK, Kumar S, Teli MK, Kim MH. Ligand-based pharmacophore modeling and docking studies on vitamin d receptor inhibitors. J Cell Biochem (2020) 121(7):3570–83. doi: 10.1002/jcb.29640
94. Jayaraj JM, Reteti E, Kesavan C, Muthusamy K. Structural insights on vitamin d receptor and screening of new potent agonist molecules: Structure and ligand-based approach. J Biomolecular Structure Dynamics (2021) 39(11):4148–59. doi: 10.1080/07391102.2020.1775122
95. Klimenko K. In silico identification of endogenous and exogenous agonists of estrogen-related receptor α. Comput Toxicol (2019) 10:105–12. doi: 10.1016/j.comtox.2019.01.005
96. Chitrala KN, Yeguvapalli S. Prediction and analysis of ligands against estrogen related receptor alpha. Asian Pac J Cancer Prev (2013) 14(4):2371–5. doi: 10.7314/APJCP.2013.14.4.2371
97. Benod C, Carlsson J, Uthayaruban R, Hwang P, Irwin JJ, Doak AK, et al. Structure-based discovery of antagonists of nuclear receptor LRH-1*. J Biol Chem (2013) 288(27):19830–44. doi: 10.1074/jbc.M112.411686
98. Rauhamäki S, Postila PA, Lätti S, Niinivehmas S, Multamäki E, Liedl KR, et al. Discovery of retinoic acid-related orphan receptor γt inverse agonists via docking and negative image-based screening. ACS Omega (2018) 3(6):6259–66. doi: 10.1021/acsomega.8b00603
99. Meijer FA, Doveston RG, de Vries RMJM, Vos GM, Vos AAA, Leysen S, et al. Ligand-based design of allosteric retinoic acid receptor-related orphan receptor γt (RORγt) inverse agonists. J Med Chem (2020) 63(1):241–59. doi: 10.1021/acs.jmedchem.9b01372
100. Matsuzaka Y, Uesawa Y. Molecular image-based prediction models of nuclear receptor agonists and antagonists using the deepsnap-deep learning approach with the tox21 10k library. Molecules (2020) 25(12):2764. doi: 10.3390/molecules25122764
101. Capuzzi SJ, Politi R, Isayev O, Farag S, Tropsha A. QSAR modeling of Tox21 challenge stress response and nuclear receptor signaling toxicity assays. Front Environ Sci (2016) 4:3/full. doi: 10.3389/fenvs.2016.00003/full
102. Mayr A, Klambauer G, Unterthiner T, Hochreiter S. DeepTox: Toxicity prediction using deep learning. Front Environ Sci (2016) 3:80. doi: 10.3389/fenvs.2015.00080
103. Banerjee P, Eckert AO, Schrey AK, Preissner R. ProTox-II: A webserver for the prediction of toxicity of chemicals. Nucleic Acids Res (2018) 46(W1):W257–63. doi: 10.1093/nar/gky318
104. Kolšek K, Mavri J, Sollner Dolenc M, Gobec S, Turk S. Endocrine disruptome–an open source prediction tool for assessing endocrine disruption potential through nuclear receptor binding. J Chem Inf Modeling (2014) 54(4):1254–67. doi: 10.1021/ci400649p
105. Vedani A, Dobler M, Hu Z, Smieško M. OpenVirtualToxLab–a platform for generating and exchanging in silico toxicity data. Toxicol Letters (2015) 232(2):519–32. doi: 10.1016/j.toxlet.2014.09.004
106. Park SJ, Kufareva I, Abagyan R. Improved docking, screening and selectivity prediction for small molecule nuclear receptor modulators using conformational ensembles. J Comput Aided Mol Des (2010) 24(5):459–71. doi: 10.1007/s10822-010-9362-4
107. Toporova L, Balaguer P. Nuclear receptors are the major targets of endocrine disrupting chemicals. Mol Cell Endocrinol (2020) 502:110665. doi: 10.1016/j.mce.2019.110665
108. Porter BA, Ortiz MA, Bratslavsky G, Kotula L. Structure and function of the nuclear receptor superfamily and current targeted therapies of prostate cancer. Cancers (Basel) (2019) 11(12):E1852. doi: 10.3390/cancers11121852
109. Jia M, Dahlman-Wright K, Gustafsson JÅ. Estrogen receptor alpha and beta in health and disease. Best Pract Res Clin Endocrinol Metab (2015) 29(4):557–68. doi: 10.1016/j.beem.2015.04.008
110. Matthews J, Gustafsson JA. Estrogen signaling: a subtle balance between ER alpha and ER beta. Mol Interv (2003) 3(5):281–92. doi: 10.1124/mi.3.5.281
111. Tan ME, Li J, Xu HE, Melcher K, Yong El. Androgen receptor: structure, role in prostate cancer and drug discovery. Acta Pharmacol Sin (2015) 36(1):3–23. doi: 10.1038/aps.2014.18
112. Fujita K, Nonomura N. Role of androgen receptor in prostate cancer: A review. World J Mens Health (2018) 37(3):288–95. doi: 10.5534/wjmh.180040
113. Schumacher M, Zhu X, Guennoun R. 3.11 - progesterone: Synthesis, metabolism, mechanism of action, and effects in the nervous system. In: Pfaff DW, Joëls M, editors. Hormones, brain and behavior, 3rd ed. Oxford: Academic Press (2017). p. 215–44. Available at: https://www.sciencedirect.com/science/article/pii/B9780128035924000547.
114. Grimm SL, Hartig SM, Edwards DP. Progesterone receptor signaling mechanisms. J Mol Biol (2016) 428(19):3831–49. doi: 10.1016/j.jmb.2016.06.020
115. Kadmiel M, Cidlowski JA. Glucocorticoid receptor signaling in health and disease. Trends Pharmacol Sci (2013) 34(9):518–30. doi: 10.1016/j.tips.2013.07.003
116. Oakley RH, Cidlowski JA. Cellular processing of the glucocorticoid receptor gene and protein: New mechanisms for generating tissue-specific actions of glucocorticoids. J Biol Chem (2011) 286(5):3177–84. doi: 10.1074/jbc.R110.179325
117. Bledsoe RK, Montana VG, Stanley TB, Delves CJ, Apolito CJ, McKee DD, et al. Crystal structure of the glucocorticoid receptor ligand binding domain reveals a novel mode of receptor dimerization and coactivator recognition. Cell (2002) 110(1):93–105. doi: 10.1016/S0092-8674(02)00817-6
118. Sharma S, Shen T, Chitranshi N, Gupta V, Basavarajappa D, Sarkar S, et al. Retinoid X receptor: Cellular and biochemical roles of nuclear receptor with a focus on neuropathological involvement. Mol Neurobiol (2022) 59(4):2027–50. doi: 10.1007/s12035-021-02709-y
119. Tanaka T, De Luca LM. Therapeutic potential of “rexinoids” in cancer prevention and treatment. Cancer Res (2009) 69(12):4945–7. doi: 10.1158/0008-5472.CAN-08-4407
120. Cramer PE, Cirrito JR, Wesson DW, Lee CYD, Karlo JC, Zinn AE, et al. ApoE-directed therapeutics rapidly clear β-amyloid and reverse deficits in AD mouse models. Science (2012) 335(6075):1503–6. doi: 10.1126/science.1217697
121. Mysinger MM, Carchia M, JohnJ I, Shoichet BK. Directory of useful decoys, enhanced (dud-e): Better ligands and decoys for better benchmarking. J Medicinal Chem (2012) 55(14):6582–94. doi: 10.1021/jm300687e
122. Kahremany S, Livne A, Gruzman A, Senderowitz H, Sasson S. Activation of PPARδ: From computer modelling to biological effects. Br J Pharmacol (2015) 172(3):754–70. doi: 10.1111/bph.12950
123. Pyper SR, Viswakarma N, Yu S, Reddy JK. PPARalpha: Energy combustion, hypolipidemia, inflammation and cancer. Nucl Recept Signal (2010) 8:e002. doi: 10.1621/nrs.08002
124. Astapova O, Leff T. Adiponectin and PPARγ: Cooperative and interdependent actions of two key regulators of metabolism. Vitam Horm (2012) 90:143–62. doi: 10.1016/B978-0-12-398313-8.00006-3
125. Grienke U, Mihály-Bison J, Schuster D, Afonyushkin T, Binder M, Guan S, et al. Identification of novel PPARα/γ dual agonists by virtual screening, ADMET prediction and molecular dynamics simulations. J Biomolecular Structure Dynamics (2021) 36(11):2988–3002. doi: 10.1080/07391102.2017.1373706?journalCode=tbsd20
126. Porskjær Christensen L, Bahij El-Houri R. Development of an in vitro screening platform for the identification of partial pparγ agonists as a source for antidiabetic lead compounds. Molecules (2018) 23(10):2431. doi: 10.3390/molecules23102431
127. Todorov MP. In silico identification of human pregnane x receptor activators. Ecol Safety (2015) 9(1):9–17.
128. Zhang Ym, Chang Mj, Yang Xs, Han X. In silico investigation of agonist activity of a structurally diverse set of drugs to hPXR using HM-BSM and HM-PNN. J Huazhong Univ Sci Technol [Med Sci] (2016) 36(3):463–8. doi: 10.1007/s11596-016-1609-4
129. Balaguer P, Delfosse V, Grimaldi M, Bourguet W. Structural and functional evidences for the interactions between nuclear hormone receptors and endocrine disruptors at low doses. Comptes Rendus Biologies (2017) 340(9–10):414–20. doi: 10.1016/j.crvi.2017.08.002
130. Ma Z, Deng C, Hu W, Zhou J, Fan C, Di S, et al. Liver X receptors and their agonists: Targeting for cholesterol homeostasis and cardiovascular diseases. Curr Issues Mol Biol (2017) 22:41–64. doi: 10.21775/cimb.022.041
131. Buñay J, Fouache A, Trousson A, de Joussineau C, Bouchareb E, Zhu Z, et al. Screening for liver X receptor modulators: Where are we and for what use? Br J Pharmacol (2021) 178(16):3277–93. doi: 10.1111/bph.15286
132. Piccinin E, Cariello M, Moschetta A. Lipid metabolism in colon cancer: Role of liver X receptor (LXR) and stearoyl-CoA desaturase 1 (SCD1). Mol Aspects Med (2021) 78:100933. doi: 10.1016/j.mam.2020.100933
133. Auerbach SS, Ramsden R, Stoner MA, Verlinde C, Hassett C, Omiecinski CJ. Alternatively spliced isoforms of the human constitutive androstane receptor. Nucleic Acids Res (2003) 31(12):3194–207. doi: 10.1093/nar/gkg419
134. Forman BM, Tzameli I, Choi HS, Chen J, Simha D, Seol W, et al. Androstane metabolites bind to and deactivate the nuclear receptor CAR-beta. Nature (1998) 395(6702):612–5. doi: 10.1038/26996
135. Tzameli I, Moore DD. Role reversal: New insights from new ligands for the xenobiotic receptor CAR. Trends Endocrinol Metab (2001) 12(1):7–10. doi: 10.1016/S1043-2760(00)00332-5
136. Liu YY, Brent GA. Thyroid hormone crosstalk with nuclear receptor signaling in metabolic regulation. Trends Endocrinol Metab (2010) 21(3):166–73. doi: 10.1016/j.tem.2009.11.004
137. Mullur R, Liu YY, Brent GA. Thyroid hormone regulation of metabolism. Physiol Rev (2014) 94(2):355–82. doi: 10.1152/physrev.00030.2013
138. Saponaro F, Sestito S, Runfola M, Rapposelli S, Chiellini G. Selective thyroid hormone receptor-beta (TRβ) agonists: New perspectives for the treatment of metabolic and neurodegenerative disorders. Front Med (Lausanne) (2020) 7:331. doi: 10.3389/fmed.2020.00331
139. Zoeller RT. Environmental chemicals as thyroid hormone analogues: new studies indicate that thyroid hormone receptors are targets of industrial chemicals? Mol Cell Endocrinol (2005) 242(1–2):10–5. doi: 10.1016/j.mce.2005.07.006
140. Kim MJ, Park YJ. Bisphenols and thyroid hormone. Endocrinol Metab (Seoul) (2019) 34(4):340–8. doi: 10.3803/EnM.2019.34.4.340
141. Pal S, Kumar V, Kundu B, Bhattacharya D, Preethy N, Reddy MP, et al. Ligand-based pharmacophore modeling, virtual screening and molecular docking studies for discovery of potential topoisomerase i inhibitors. Comput Struct Biotechnol J (2019) 17:291–310. doi: 10.1016/j.csbj.2019.02.006
142. Yaghmaei S, Roberts C, Ai R, Mizwicki MT, Chang CEA. Agonist and antagonist binding to the nuclear vitamin d receptor: Dynamics, mutation effects and functional implications. In Silico Pharmacol (2013) 1:2. doi: 10.1186/2193-9616-1-2
143. Giguère V. To ERR in the estrogen pathway. Trends Endocrinol Metab (2002) 13(5):220–5. doi: 10.1016/S1043-2760(02)00592-1
144. Gallet M, Vanacker JM. ERR receptors as potential targets in osteoporosis. Trends Endocrinol Metab (2010) 21(10):637–41. doi: 10.1016/j.tem.2010.06.008
145. Kumar N, Solt LA, Conkright J, Wang Y, Istrate MA, Busby SA, et al. Campaign to identify novel modulators of the retinoic acid receptor-related orphan receptors (ROR). In: Probe reports from the NIH molecular libraries program [Internet]. Bethesda (MD: National Center for Biotechnology Information (US (2010). Available at: http://www.ncbi.nlm.nih.gov/books/NBK56239/.
146. Nishiyama Y, Nakamura M, Misawa T, Nakagomi M, Makishima M, Ishikawa M, et al. Structure–activity relationship-guided development of retinoic acid receptor-related orphan receptor gamma (RORγ)-selective inverse agonists with a phenanthridin-6(5H)-one skeleton from a liver X receptor ligand. Bioorganic Medicinal Chem (2014) 22(9):2799–808. doi: 10.1016/j.bmc.2014.03.007
147. Balaguer P, Delfosse V, Bourguet W. Mechanisms of endocrine disruption through nuclear receptors and related pathways. Curr Opin Endocrine Metab Res (2019) 7:1–8. doi: 10.1016/j.coemr.2019.04.008
148. Vogel CFA, Van Winklea LS, Esser C, Haarmann-Stemmann T. The aryl hydrocarbon receptor as a target of environmental stressors – implications for pollution mediated stress and inflammatory responses. RedOx Biol (2020) 34:101530. doi: 10.1016/j.redox.2020.101530
149. Gu C, Goodarzi M, Yang X, Bian Y, Sun C, Jiang X. Predictive insight into the relationship between AhR binding property and toxicity of polybrominated diphenyl ethers by PLS-derived QSAR. Toxicol Lett (2012) 208(3):269–74. doi: 10.1016/j.toxlet.2011.11.010
150. Cao F, Li X, Ye L, Xie Y, Wang X, Shi W, et al. Molecular docking, molecular dynamics simulation, and structure-based 3D-QSAR studies on the aryl hydrocarbon receptor agonistic activity of hydroxylated polychlorinated biphenyls. Environ Toxicol Pharmacol (2013) 36(2):626–35. doi: 10.1016/j.etap.2013.06.004
151. Wu D, Potluri N, Kim Y, Rastinejad F. Structure and dimerization properties of the aryl hydrocarbon receptor pas-a domain. Mol Cell Biol (2013) 33(21):4346–56. doi: 10.1128/MCB.00698-13
152. Giani Tagliabue S, Faber SC, Motta S, Denison MS, Bonati L. Modeling the binding of diverse ligands within the ah receptor ligand binding domain. Sci Rep (2019) 9:10693. doi: 10.1038/s41598-019-47138-z
153. Motto I, Bordogna A, Soshilov AA, Denison MS, Bonati L. New aryl hydrocarbon receptor homology model targeted to improve docking reliability. J Chem Inf Modeling (2021) 51(11):2868–81. doi: 10.1021/ci2001617
154. Parks AJ, Pollastri MP, Hahn ME, Stanford EA, Novikov O, Franks DG, et al. In silico identification of an aryl hydrocarbon receptor antagonist with biological activity in vitro and In vivo. Mol Pharmacol (2014) 86(5):593–608. doi: 10.1124/mol.114.093369
155. Seidel T, Ibis G, Bendix F, Wolber G. Strategies for 3D pharmacophore-based virtual screening. Drug Discovery Today: Technologies (2010) 7(4):e221–8. doi: 10.1016/j.ddtec.2010.11.004
156. Morris GM, Lim-Wilby M. Molecular docking. In: Kukol A, editor. Molecular modeling of proteins. Totowa: NJ: Humana Press (2008). p. 365–82. doi: 10.1007/978-1-59745-177-2_19
157. Ben Nasr N, Guillemain H, Lagarde N, Zagury JF, Montes M. Multiple structures for virtual ligand screening: defining binding site properties-based criteria to optimize the selection of the query. J Chem Inf Model (2013) 53(2):293–311. doi: 10.1021/ci3004557
158. International union of pure and applied chemistry. IUPAC. Available at: https://iupac.org/
159. Yang SY. Pharmacophore modeling and applications in drug discovery: Challenges and recent advances. Drug Discovery Today (2010) 15(11):444–50. doi: 10.1016/j.drudis.2010.03.013
160. Gedeck P, Rohde B, Bartels C. QSAR – how good is it in practice? comparison of descriptor sets on an unbiased cross section of corporate data sets. J Chem Inf Model (2006) 46(5):1924–36. doi: 10.1021/ci050413p
161. Jiménez-Luna J, Grisoni F, Weskamp N, Schneider G. Artificial intelligence in drug discovery: recent advances and future perspectives. Expert Opin Drug Discov (2021) 16(9):949–59. doi: 10.1080/17460441.2021.1909567
162. Weaver S, Gleeson MP. The importance of the domain of applicability in QSAR modeling. J Mol Graphics Modelling (2008) 26(8):1315–26. doi: 10.1016/j.jmgm.2008.01.002
163. Sellami A, Montes M, Lagarde N. Predicting potential endocrine disrupting chemicals binding to estrogen receptor α (ERα) using a pipeline combining structure-based and ligand-based in silico methods. Int J Mol Sci (2021) 22(6):2846. doi: 10.3390/ijms22062846
164. Hein M, Zilian D, Sotriffer CA. Docking compared to 3D-pharmacophores: the scoring function challenge. Drug Discovery Today: Technologies (2010) 4(7):e229–36. doi: 10.1016/j.ddtec.2010.12.003
165. Vuorinen A, Odermatt A, Schuster D. In silico methods in the discovery of endocrine disrupting chemicals. J Steroid Biochem Mol Biol (2013) 137:137:18–26. doi: 10.1016/j.jsbmb.2013.04.009
166. Vázquez J, López M, Gibert E, Herrero E, Luque FJ. Merging ligand-based and structure-based methods in drug discovery: An overview of combined virtual screening approaches. Molecules (2020) 25(20):4723. doi: 10.3390/molecules25204723
167. Lunghini F, Marcou G, Azam P, Bonachera F, Enrici MH, Van Miert E, et al. Endocrine disruption: The noise in available data adversely impacts the models’ performance. SAR QSAR Environ Res (2021) 32(2):111–31. doi: 10.1080/1062936X.2020.1864468
168. Réau M, Langenfeld F, Zagury JF, Lagarde N, Montes M. Decoys selection in benchmarking datasets: Overview and perspectives. Front Pharmacol (2018) 9:11. doi: 10.3389/fphar.2018.00011
169. Réau M, Lagarde N, Zagury JF, Montes M. Nuclear receptors database including negative data (nr-dbind): a database dedicated to nuclear receptors binding data including negative data and pharmacological profile. J Med Chem (2019) 62(6):2894–904. doi: 10.1021/acs.jmedchem.8b01105
170. Goya-Jorge E, Giner RM, Sylla-Iyarreta Veitía M, Gozalbes R, Barigye SJ. Predictive modeling of aryl hydrocarbon receptor (AhR) agonism. Chemosphere (2020) 256:127068. doi: 10.1016/j.chemosphere.2020.127068
171. Begley CG, Ioannidis JPA. Reproducibility in science. Circ Res (2015) 116(1):116–26. doi: 10.1161/CIRCRESAHA.114.303819
172. Munafò MR, Nosek BA, Bishop DVM, Button KS, Chambers CD, du Sert NP, et al. A manifesto for reproducible science. Nat Hum Behav (2017) 1:0021. doi: 10.1038/s41562-016-0021
173. Stodden V, Guo P, Ma Z. Toward reproducible computational research: An empirical analysis of data and code policy adoption by journals. PloS One (2013) 8(6):e67111. doi: 10.1371/journal.pone.0067111
Keywords: nuclear receptors, in silico, endocrine disrupting chemicals, docking, pharmacophore model, QSAR
Citation: Sellami A, Réau M, Montes M and Lagarde N (2022) Review of in silico studies dedicated to the nuclear receptor family: Therapeutic prospects and toxicological concerns. Front. Endocrinol. 13:986016. doi: 10.3389/fendo.2022.986016
Received: 04 July 2022; Accepted: 08 August 2022;
Published: 13 September 2022.
Edited by:
Marica Cariello, University of Bari Aldo Moro, ItalyReviewed by:
Elena Piccinin, University of Bari Aldo Moro, ItalyJean-Marc A. Lobaccaro, Université Clermont Auvergne, France
Copyright © 2022 Sellami, Réau, Montes and Lagarde. This is an open-access article distributed under the terms of the Creative Commons Attribution License (CC BY). The use, distribution or reproduction in other forums is permitted, provided the original author(s) and the copyright owner(s) are credited and that the original publication in this journal is cited, in accordance with accepted academic practice. No use, distribution or reproduction is permitted which does not comply with these terms.
*Correspondence: Nathalie Lagarde, nathalie.lagarde@lecnam.net