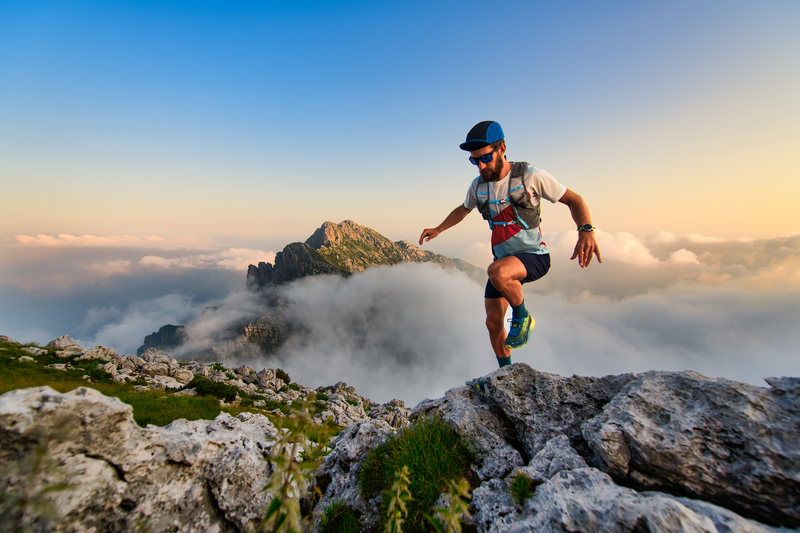
94% of researchers rate our articles as excellent or good
Learn more about the work of our research integrity team to safeguard the quality of each article we publish.
Find out more
ORIGINAL RESEARCH article
Front. Endocrinol. , 05 September 2022
Sec. Clinical Diabetes
Volume 13 - 2022 | https://doi.org/10.3389/fendo.2022.970825
This article is part of the Research Topic Gestational Diabetes Mellitus Risk Assessment, Screening, Diagnosis, and Management Before, During and After Pregnancy View all 12 articles
Aims: Gestation is linked to changes in gut microbiota composition and function. Since gestational diabetes mellitus (GDM) can develop at any time of the pregnancy, we stratified the women into four groups according to the time and test used for the diagnosis. We focused on the gut microbiota pattern in early pregnancy to detect changes which could be linked to later GDM development.
Methods: We collected stool samples from 104 pregnant women including obese individuals (first trimester body mass index median was 26.73). We divided the women into four groups according to routine screening of fasting plasma glucose (FPG) levels and oral glucose tolerance test (oGTT) in the first and third trimesters, respectively. We processed the stool samples for bacterial 16S rRNA and fungal ITS1 genes sequencing by Illumina MiSeq approach and correlated the gut microbiota composition with plasma short-chain fatty acid levels (SCFA).
Results: We found that gut bacterial microbiota in the first trimester significantly differs among groups with different GDM onset based on unweighted UniFrac distances (p=0.003). Normoglycemic women had gut microbiota associated with higher abundance of family Prevotellaceae, and order Fusobacteriales, and genus Sutterella. Women diagnosed later during pregnancy either by FGP levels or by oGTT had higher abundances of genera Enterococcus, or Erysipelotrichaceae UCG-003, respectively. We observed significant enrichment of fungal genus Mucor in healthy pregnant women whereas Candida was more abundant in the group of pregnant women with impaired oGTT. Using correlation analysis, we found that Holdemanella negatively correlated with Blautia and Candida abundances and that Escherichia/Shigella abundance positively correlated and Subdoligranulum negatively correlated with plasma lipid levels. Coprococcus, Akkermansia, Methanobrevibacter, Phascolarctobacterium and Alistipes positively correlated with acetate, valerate, 2-hydroxybutyrate and 2-methylbutyrate levels, respectively, in women with GDM.
Conclusions: We conclude that there are significant differences in the gut microbiota composition between pregnant women with and without GDM already at the early stage of pregnancy in our cohort that included also overweight and obese individuals. Specific microbial pattern associated with GDM development during early pregnancy and its correlation to plasma lipid or SCFA levels could help to identify women in higher risk of GDM development.
Gestational diabetes mellitus (GDM) is the most common medical complication of pregnancy that affects more than 14% of women worldwide (1). It is described as any degree of glucose intolerance that appears during pregnancy (2). GDM is associated with many health complications affecting woman and the offspring, including gestational hypertension, preeclampsia, or preterm birth and fetal macrosomia, hypoglycemia, respiratory distress syndrome or cardiomyopathy (3, 4). In addition, women with GDM have about 40% higher risk of developing type 2 diabetes mellitus (T2DM) in the next 10 – 15 years (5, 6). The offspring of women with GDM are in increased risk for developing diabetes and obesity as well (5, 7). Nevertheless, several studies have described that infants breastfed by women diagnosed with GDM may have reduced risk of obesity or T2DM development later in their life (8, 9). Factors transferred by milk from mother to offspring modulate its microbiome, immune system tuning or metabolic activity which are tightly associated with obesity or T2DM (10–12). Intrinsic and extrinsic factors accompanying the metabolic and immunological changes during pregnancy, especially increased insulin resistance, gestational weight gain, family history of diabetes, obesity and immune tolerance against the fetus and placenta, are the prerequisite for the development of GDM (13, 14). These changes are also associated with alterations in the energy metabolism of pregnant women. The beginning of pregnancy is strongly related with the storage of energy. However, in the third trimester, the energy metabolism pathways are activated, which results in the release of glucose and fatty acids into the bloodstream (15). As a consequence of these significant changes in the metabolism, predisposed pregnant women are prone to develop GDM. Based on the diagnostic criteria, two main subtypes of GDM may be distinguished. The first one is characterized by women with repeatedly increased fasting plasma glucose (FPG; FPG ≥ 5.1 mmol/l) while the second one is detected postprandially after oral glucose tolerance test (oGTT; plasma glucose ≥ 10.0 mmol/l at 1h and/or ≥ 8.5 mmol/l at 2h during oGTT). These two subtypes differ in their pathophysiological mechanisms and also in the severity of health complications associated with GDM (16). This means the earlier GDM develops the more severe complications it brings. Therefore, prompt diagnosis is crucial for early dietary intervention and mitigation of the consequences.
The composition and metabolic activity of the gut microbiota have been described as factors that can influence glucose metabolism. For instance, a specific gut microbiota pattern has been observed in subjects with obesity, prediabetes or T2DM (17–19). Moreover, microbial diversity and its function in the gut are altered during pregnancy. In the first trimester, the gut microbiome of a pregnant woman is mostly similar to a healthy non-pregnant woman, while in the third trimester, a high degree of dysbiosis is observed, especially in the decrease of short-chain fatty acids (SCFA)-producing bacteria and in the increase in Actinobacteria and Proteobacteria (20, 21). Although the relative abundance of the four dominant phyla (Firmicutes, Bacteroidetes, Proteobacteria and Actinobacteria) differs among mildly underweight pregnant women, pregnant women with normal body mass index (BMI), overweight, and obese pregnant women (22), any of these women can develop GDM. To date, few studies have focused on microbial or microbiota-associated metabolic changes in GDM development, and none of them have aimed at the differences in the gut microbiota composition among subtypes of GDM determined by FPG levels or oGTT.
The microbiota can also modify metabolic processes in the body through their metabolites, such as SCFA, branched-chain fatty acids or bile acids. SCFA are produced by gut microbiota through anaerobic fermentation of non-digestible carbohydrates. Major types of SCFA are acetate, propionate and butyrate that modulate energy metabolism and that are involved in the maintaining of glucose homeostasis (23). However, type and amount of SCFA depends on diet that affects the gut microbiota composition and function (24). Recently, the impaired insulin sensitivity in pregnancy and the development of GDM have been linked to diet as a source of substrates that are further processed by the gut microbiota, resulting in the formation of metabolites, such as SCFA (25, 26).
In our study, we focused on the early gut microbiota pattern in pregnant women in order to identify changes which could predict later GDM development. For this purpose, we sequenced gut bacterial and fungal microbiota in 104 pregnant women, representing a common Czech population of women with low, normal and high BMI. The women were divided into four subgroups according to their FPG levels and oGTT as follows: healthy pregnant women, pregnant women with impaired FPG in the first trimester, pregnant women with impaired FPG in the third trimester and pregnant women with impaired oGTT in the third trimester. Moreover, we correlated the microbiota changes with basic biochemical parameters and SCFA levels in plasma. Our data could help to determine early pregnancy microbial patterns that are associated with GDM development later during pregnancy and thus could help with its early detection.
For this study, 104 pregnant women were enrolled during regular appointments at the Third Department of Internal Medicine – Nephrology, Rheumatology and Endocrinology, Olomouc University Hospital. The exclusion criteria for enrolment comprised of recent antibiotic treatment (at least three months before sampling) and a history of intestinal disease or major intestinal resection. Enrolled women were tested for GDM according to the recommendation of International Association of Diabetes and Pregnancy Study Groups (27). The detection and diagnosis of hyperglycemic disorders in pregnancy involves two phases. The first test is performed during an initial prenatal visit (usually in the first trimester) to reveal women with overt diabetes who have not been diagnosed before pregnancy. If the results are not sufficient for the diagnosis of overt diabetes but are abnormal (FPG ≥ 5.1 mmol/L but < 7.0 mmol/L), early GDM is suspected. Therefore, if overt diabetes is excluded, it is recommended to classify as GDM also the FPG values ≥ 5.1 mmol/L in early pregnancy. The second phase includes the 75g oGTT in 24th – 28th week of gestation in all women who had not previously been diagnosed with overt diabetes or GDM to detect GDM in this period. Clinical and biochemical parameters were collected in the first trimester of pregnancy from the patients’ registry and the SCFA levels were extracted from a publication by Ivanovova et al. (2021) which describes the same cohort of women with GDM (28). Samples of feces were collected at two time points during the first and the third trimesters of pregnancy. Samples were frozen within 5h after collection and stored at -20°C until the DNA extraction.
This study was approved by the Ethics Committee at Olomouc University Hospital (approval no. 120/17). An informed consent was obtained from all subjects before enrolment.
Total DNA was extracted using ZymoBIOMICS DNA Miniprep Kit (ZYMO Research, Irvine, CA, USA)according to the manufacture´s protocol with repeated bead-beating using FastPrep homogenizer (MP Biomedicals, Santa Ana, CA, USA). PCR targeting V3 and V4 regions of bacterial 16S was conducted using Kapa HiFi HotStart Ready mix (Roche, Penzberg, Germany) using 341F (5′-CCTACGGGNGGCWGCAG- 3′) and 806R (5′-GGACTACHVGGGTWTCTAAT- 3′) primers (Generi Biotech, Hradec Kralove, CZ). Cycling conditions consisted of initial denaturation (95°C, 4 min) followed by 30 cycles of denaturation (95°C, 30 s), annealing (55°C, 30 s), extension (72°C, 30 s) and final extension (72°C, 5 min). PCR targeting of fungal ITS1 region was performed also with Kapa Hifi HotStart Ready mix (Roche) using primers with barcodes ITS1-5.8Sfw (5′-AAGTTCAAAGAYTCGATGATTCAC-3′) and ITS1-5.8Srv (5′-AAGTTCAAAGAYTCGATGATTCAC-3′). Cycling conditions consisted of initial denaturation (95°C, 4 min) and 35 cycles of denaturation (95°C, 30 s), annealing (60°C, 30 s), extension (72°C, 30 s) and final extension (72°C, 5 min). PCR triplicates were pooled and purified by SequalPrep Normalization Plate Kit (Thermo Fisher Scientific, Waltham, MA, USA). Samples within library were pooled, concentrated (Eppendorf centrifugal vacuum concentrator), purified with DNA Clean&Concentrator kit (ZYMO Research)and sequencing adaptors were ligated using Kapa HyperPrep kit (Roche). Ligated libraries were quantified with KAPA Library Quantification Kit (Kapa Biosystems) and sequenced on MiSeq Illumina Platform using Miseq Reagent Kit v3 (Illumina) at The Genomics Core Facility, CEITEC (Brno, Czech Republic). Sequencing data were processed using QIIME version 1.9.1 (29). Raw reads were demultiplexed and quality filtered, allowing no N characters, a maximum of three consecutive low-quality base calls, a maximum unacceptable Phred quality of Q20, and a maximum of 1.5 barcode errors. Chimeric reads were detected and discarded using USEARCH algorithms (30). Fungal reads were in addition extracted for ITS1 region using ITSx package (31). Identification of representative sequences was done using RPD classifier (32) against bacterial GREENGENES database 13.8 (33) and fungal UNITE database 7.2 (UNITE Community (2017): UNITE QIIME release. Version 01.12.2017. UNITE Community. https://doi.org/10.15156/BIO/587481). Finally, OTU table was produced. The data are available in the Sequence Read Archive (SRA) https://www.ncbi.nlm.nih.gov/bioproject/PRJNA833950.
Briefly, for microbiota analysis, the number of observed OTUs (operational taxonomic unit) and Chao1, Shannon, Simpson and Faith Phylogenetic Diversity indexes were used to describe alpha diversity and Principle Coordinate Analysis (PCoA) based on weighted and unweighted UniFrac distance for bacteria and Bray-Curtis and Jaccard distance for fungi were used to characterize beta diversity. The permutational multivariate analysis of variance (PERMANOVA) was used for the determination of statistical differences among groups. Furthermore, Linear discriminant analysis effect size (LEfSe; RRID: SCR_014609) was used to determine the features discriminating communities in each group (29, 34). Functional potential of a bacterial metagenome was predicted by Phylogenetic Investigation of Communities by Reconstruction of Unobserved States (PICRUSt) tool, using the 16S rRNA amplicon data (35).
Data were analyzed using GraphPad Prism version 8.0.0 for Windows (GraphPad Software, San Diego, CA, USA; www.graphpad.com). Statistical differences between two groups were calculated by nonparametric Mann-Whitney U test. In the case of more groups, nonparametric Kruskal-Wallis test with Dunn´s post-hoc testing were used. Data were expressed as medians with first and third quartiles. Values of p < 0.05 were considered significantly different. Covariations of gut microbiota with other factors were calculated by Spearman´s correlation analysis with Bonferroni ´s adjustment for multiple comparisons.
For basic group differentiation, we compared clinical and biochemical parameters of healthy pregnant women (HC) and pregnant women with diabetes who had impaired FPG in the first (GDM1) or in the third trimester (GDM2), and with impaired oGTT in the third trimester (GMD3; Table 1). We found a significant increase in body weight (GDM2 p<0.01; GDM3 p<0.05) and BMI (GDM1 p<0.01; GDM2 p<0.001; GDM3 p<0.01) in women with GDM compared to healthy pregnant women mainly due to obese women inclusion. The GDM2 and GDM3 groups showed significant increase in cholesterol (GDM2 p<0.001; GDM3 p<0.01), triglycerides (GDM2 p<0.001; GDM3 p<0.001), low-density lipoprotein (LDL; GMD2 p<0.01; GDM3 p<0.05), non-high-density lipoprotein (nonHDL; GDM2 p<0.001; GDM3 p<0.01) and 3-hydroxybutyrate (GDM p<0.05; GDM p<0.001) compared to healthy pregnant women. The highest FPG levels were determined in the GDM1 (p<0.001) and GDM2 women (p<0.001).
Table 1 First trimester clinical and biochemical data of healthy pregnant women and pregnant women with impaired FPG or oGTT.
To characterize differences in gut microbiota between pregnant women with and without diabetes, we collected fecal samples in the first trimester and processed them for sequencing analysis. We found mostly non-significant reduction in all alpha diversity indexes in the samples from women with GDM, except for the Simpson and Faith phylogenetic diversity indexes which describe the richness of the samples. Women diagnosed later during pregnancy either by FPG levels or by oGTT had significantly lower diversity compared to healthy controls (Figure 1A). We observed significantly different composition of gut microbiota in normoglycemic pregnant women and pregnant women with GDM measured by PERMANOVA based on unweighted and weighted UniFrac with values p=3x10-3 and p=6x10-3, respectively (Figures 1B–E). Subsequent LEfSe analysis identified bacteria significantly different among groups based on their relative abundances (Figure 1F). Gut microbiota of normoglycemic pregnancies was associated with increased abundance of family Prevotellaceae, order Fusobacteriales and genus Sutterella. The women who developed impaired insulin resistance later in pregnancy (GDM2) had higher abundance of genera Enterococcus and Erysipelotrichaceae UCG-003.
Figure 1 Gut bacterial community composition in the first trimester of pregnancy. (A) Alpha diversity indexes. Beta diversity of gut bacteria on unweighted (B, C) and weighted (D, E) UniFrac distance metric-based PCoA graphs show comparison of control healthy pregnant women (HC) with those with gestational diabetes mellitus (GDM) diagnosed at any time during the pregnancy. (F) LEfSe analysis of significantly different strains among groups. Statistically significant differences were measured by Kruskal-Wallis test with Dunn´s post-hoc testing. The data are presented as medians with interquartile range. OTUs, operational taxonomic units; PD, phylogenetic diversity.
Prediction of metabolic pathways associated with the abundance of gut bacteria showed that most of them were linked to energy metabolism or active cell division, especially pathways producing components of cell membranes and cell walls (Figure S1).
To characterize the gut mycobiota, we sequenced the ITS region in the samples from healthy pregnant women and pregnant women with GDM. Fungal alpha diversity indexes showed significant reduction in GDM pregnant women as described by species richness (observed OTUs) and by species abundance (Chao 1 index, Figure 2A). We did not observe any significant clustering of groups in neither Bray-Curtis dissimilarity plot based on fungal abundance nor in Jaccard distance plot comparing fungal composition among samples (Figures 2B–E). Using LEfSe analysis, we found that there was significant enrichment in genus Mucor in healthy pregnant women and that genus Candida was more abundant in the group of pregnant women with impaired oGTT in the third trimester (Figure 2F).
Figure 2 Gut fungal microbiota composition in the first trimester of pregnancy. (A) Alpha diversity indexes. Beta diversity of gut fungi on Bray-Curtis (B, C) and Jaccard (D, E) distance metric-based PCoA graphs show comparison of control healthy pregnancies (HC) with those with gestational diabetes mellitus (GDM) diagnosed at any time during the pregnancy. (F) LEfSe analysis of significantly different strains among groups. Statistically significant differences were measured by Kruskal-Wallis test with Dunn´s post-hoc testing. The data are presented as medians with interquartile range. OTUs,ndash; operational taxonomic units.
Comparison of microbiota relative abundances in the samples collected during the first and third trimester showed significantly different patterns that distinguished healthy pregnant women and women with GDM. In the first trimester (V1), normoglycemic women were associated with higher abundance of bacterial classes Bacteroidia and γ-Proteobacteria, archeal class Methanobacteria, and fungal classes Mucuromycetes, Eurotiomycetes, Microbotryomycetes and Malasseziomycetes compared with pregnant women with GDM (Figures 3A, B). Interestingly, the differences in these classes were more or less narrowed later in the pregnancy. In the third trimester (V3), pregnant women with GDM showed significant increase in classes Negativicutes and Clostridia, especially of the family Oscillospiraceae, and lower abundance of classes Desulfovibrionea and Bacilli compared to normoglycemic women (Figure 3C). These two later classes included significantly more abundant genera Bilophila, Leuconostoc, Streptococcus and Erysipelotrichaceae UCG-003 in healthy women (Figure S2). Although the analysis of fungal community showed no differences at class level during the third trimester, we found significant enrichment of family Debaryomycetaceae and genus Rhodotorula in women with GDM (Figure S3).
Figure 3 Differently abundant classes of bacteria and fungi between normoglycemic women (HC) and women with early-diagnosed GDM (GDM 1) at the first trimester – V1 (A, B) and the bacterial classes in the third trimester – V3 (C). Only significantly different classes are shown. The data are presented as medians with interquartile range. The abundances were compared by Mann-Whitney U test and p < 0.05 was considered statistically significant.
Using Spearman correlation analysis, we found several significant associations among gut microbiota. Normoglycemic women (HC) showed a strong positive correlation of genera Bacteroides and Roseburia (r=0.75; p=6x10-5) and negative associations of genera Dialister with Phascolarctobacterium (r=-0.66; p=9x10-4) and Parabacteriodes with Romboutsia (r=-0.65; p=9x10-4) (Figure S4). Pregnant women with impaired FPG (GDM 1) had positive correlation of bacterial genus Prevotella with fungal Cladosporium (r=0.59; p=9x10-4) (Figure S5). Pregnant women with impaired FPG in the third trimester (GDM 2) showed strong negative correlations of genera Dialister with Phascolarctobacterium (r=-0.71; p=9x10-6) and Holdemanella with Blautia (r=-0.67; p=4x10-5). The GDM 2 group also showed several positive correlations, including the associations of genera Fusicatenibacter with Agathobacter (r=0.65; p=8x10-5), Bifidobacterium and Collinsella (r=0.64; p=10-4) and bacterial genus Phascolarctobacterium with archeal Methanobrevibacter (r=0.63; p=10-4) (Figure S6). Women with impaired oGTT in the third trimester (GDM 3) did not show any significant associations; the strongest one was negative correlation of bacterial genus Holdemanella with yeast Candida (r=0.62; p=4x10-3) (Figure S7).
To observe early associations between bacteria and plasma parameters measured in the first trimester, Spearman correlation analysis was used (Figure 4A). In normoglycemic women, we found very strong negative correlation of genus Subdoligranulum with plasma levels of LDL (r=-0.75; p=9x10-5), nonHDL (r=-0.74; p=10-4) and cholesterol (r=-0.68; p=7x10-4) and genus Holdemanella which was also negatively associated with the level of CP-RI (r=-0.70; p=10-3). Interestingly, these correlations were not detected in early diagnosed group of pregnant women with GDM (GDM 1). The pregnant women with later onset of the GDM showed different associations, including negative correlation of genus Prevotella with cholesterol (r=-0.57; p=10-3) and genus Collinsella with CP-RI (r=-0.58; p=10-3) and positive correlation of genus Anaerostipes with CP-RI (r=0.61; p=5x10-4) and Escherichia/Shigella group with nonHDL (r=0.82; p=3x10-6), LDL (r=0.70; p=3x10-4) and triglycerides (r=0.67; p=6x10-4) levels.
Figure 4 Correlations of serum biochemical parameters (A) and levels of short-chain fatty acids (B) with bacterial abundances. Within each column, the subcolumns are in order: healthy pregnant women, GDM1, GDM2 and GDM3. The strength and polarity of correlation is color-coded, e.g. negative correlation in shades of blue. All p-values were adjusted for multiple comparisons, p<0.001 was considered statistically significant and significant correlations were marked with the asterisks.
Comparison of the associations of bacterial relative abundance with the levels of SCFA showed no specific pattern in normoglycemic women whereas GDM promoted some covariations (Figure 4B). In the GDM 1 group, genus Akkermansia positively correlated with the levels of valerate (r=0.58; p=10-3) and genus Streptococcus showed strong negative correlation with the levels of 4-methylvalerate (r=-0.67; p=8x10-4). In the GDM 2 group, archeal genus Methanobrevibacter positively correlated with the levels of valerate (r=0.61; p=4x10-4) and bacterial genus Phascolarctobacterium with 2-hydroxybutyrate levels (r=0.62; p=3x10-4). In the GDM 3 group, genera Coprococcus and Alistipes positively correlated with the levels of acetate (r=0.67; p=10-3) and 2-methylbutyrate (r=0.69; p=5x10-4), respectively.
In this study, we examined gut microbiome pattern of women in early stage of pregnancy to identify changes that are associated with GDM development. Systematic reviews have shown that although most of the studies observed an association between GDM and gut microbiota dysbiosis, no GDM-specific gut microbiota was identified (36, 37). Moreover, the contribution of gut mycobiome is often neglected. Though, there is a presumption that gut microbiota composition and function may contribute to the development of GDM (36). For this purpose, we focused on the composition of gut microbiota in early pregnancies.
In healthy population, gut microbiome contains six bacterial phyla: Firmicutes, Bacteroidetes, Proteobacteria, Actinobacteria, Fusobacteria, and Verrucomicrobia, with the dominance of the first two (38). Previous studies showed significant microbiota changes in normoglycemic women during pregnancy (20, 39, 40). Whether these changes contribute to or are a consequence of the development of GDM is a debated question. Several studies have reported increased abundance of Firmicutes or Actinobacteria and Proteobacteria in women with GDM (20, 21, 40, 41). In addition, enrichment of genera Parabacteroides, Ruminococcus, Eubacterium, Prevotella, Collinsella, Rothia, and Desulfovibrio has been also observed in pregnant women with GDM compared to normoglycemic controls (21, 42–44). On the contrary, increased abundance of Bacteroidetes and Actinobacteria as well as enrichment of Faecalibacterium, Methanobrevibacter, Alistipes, Bifidobacterium or Eubacterium has been described in normoglycemic pregnant women (40, 42, 43, 45). Most of these studies focused on the microbiota composition at the third trimester, i.e. after the onset of GDM. Therefore, we aimed more on the microbiota pattern in early pregnancy that could predict development of GDM. In our cohort of pregnant women, gut microbiota of normoglycemic women was associated with increased abundance of family Prevotellaceae, order Fusobacteriales and genus Sutterella. Interestingly, Wang et al. (2020) identified a significant decrease of the family Alcaligenaceae (including genus Sutterella) in the ascending colon of patients with T2DM. Subsequent experimental study showed increased abundance of Sutterella in the cecum of T2DM rats that underwent Roux-en-Y gastric bypass surgery (46). Thus, suggesting that this genus may beneficially affect glucose metabolism. Furthermore, we found that women who developed impaired insulin resistance later in pregnancy had higher abundance of genera Enterococcus or Erysipelotrichaceae UCG-003. This is in agreement with Ferrocino et al. (2018) who found that insulin resistance positively correlated with class Erysipelotrichia (45). Meanwhile, Crusell et al. (2018) observed reduction of Erysipelotrichaceae in women with GDM (21). Though, our results are supported by another study that found higher levels of Erysipelotrichaceae also in obese individuals (47). Since our study included obese individuals our results may be affected by this fact as well. Individuals with obesity have different profile of the gut microbiota in comparison to non-obese individuals (48). Moreover, obesity and GDM can influence many maternal and neonatal processes, includingthe breast milk microbiota and simultaneously the offspring gut microbiota. For example, compared to control samples, colostrum of women with either obesity or GDM was enriched in genera Staphylococcus or Prevotella, respectively (44).
Our study is one of the first to investigate the association between gut fungi and the GDM. Fungal communities in the gut constitute a minor component of the entire gut microbes thus are still poorly understood. According to recent shotgun metagenomic sequencing analysis, fungi represent approximately 0.1% of the total gut microbes (49). In our study, we found a significant enrichment of genus Mucor in healthy pregnant women. Members of this genus have been negatively correlated with obesity suggesting their association with microbiota of healthy lean individuals (50). Indeed, our cohort of normoglycemic pregnant women included only 4.5% of obese individuals. Recently, genus Penicillium has been associated with the gut mycobiota of healthy pregnant women (51) but we did not observe higher levels of this genus in our groups. In the group of pregnant women with impaired oGTT, we observed increased abundance of genus Candida in the third trimester. This is in agreement with very recent study by Ferrocino et al. (2022) who observed an increasing abundance of Candida between the second and third trimesters (52). Candida albicans inhabits the gastrointestinal tract, mouth and vaginal mucosa in 40 – 60% of healthy adults as a commensal organism, but it may cause disease in immunocompromised individuals (53, 54). Several studies have already reported increased abundance of Candida albicans in obese individuals and in patients with type 1 diabetes mellitus (T1DM) and T2DM (50, 55–57). Moreover, it is generally assumed that pregnant woman with GDM are more prone to Candida vaginal infection (58–60).
Decreased abundance of Roseburia and Bacteroides was observed in the GDM women compared to healthy pregnant women (42). In accordance, we determined positive correlation of these two bacteria in healthy women but not in the GDM group. In the groups of pregnant women with impaired FPG/oGTT in the third trimester, we found negative correlation of Holdemanella with Blautia and with yeast Candida, respectively. Romaní-Pérez et al. (2021) showed that Holdemanella, an intestinal bacterium isolated from metabolically healthy individuals, had anti-diabetic effect through glucagon-like peptide 1 signaling pathway and its supplementation improved glucose tolerance in a diet-induced obese mouse model (61). Increased abundance of Collinsella and reduced abundance of Bifidobacterium have been reported in pregnant women with GDM compared to healthy controls (21, 43). Nevertheless, we found positive correlation of Collinsella with Bifidobacterium in pregnant women with impaired FPG in the third trimester. In the same group, we also observed a positive correlation of Methanobrevibacter smithii and Phascolartobacterium. On the other hand, Phascolartobacterium negatively correlated with genus Dialister in the GDM2 and normoglycemic groups. Increased abundance of Dialister and reduced abundance of Phascolartobacterium have been related to impaired insulin sensitivity in obese individuals (62).
Healthy pregnancy is characterized by complex metabolic and hormonal changes. Plasma lipid concentrations change during pregnancy due to increasing insulin resistance. Serum levels of high-density lipoprotein-cholesterol (HDL-C), low-density lipoprotein-cholesterol (LDL-C), total cholesterol, and to lesser extent triglycerides (TG) are elevated throughout the pregnancy (63–65). In our study, we observed significantly higher levels of cholesterol, LDL-C, and TG in women with GDM compared to healthy women which is consistent with other studies (66–69). Moreover, it has been shown that gut microbiota can influence the levels of blood lipids (70, 71). Here, we found that lipid levels were linked to specific gut microbiota. In normoglycemic women, we found very strong negative correlation of genus Subdoligranulum with serum levels of LDL, nonHDL, and cholesterol. In contrast, in women with later onset of GDM, we found positive correlation of Escherichia/Shigella group with LDL, nonHDL, and triglycerides and negative correlation of genus Prevotella with total cholesterol. While the SCFA-producing genus Subdoligranulum has been connected with health promoting effects, the family Enterobacteriaceae has been enriched in GDM and has already been linked to T2DM and obesity (19, 43, 72).
In contrast to a well described role of lipids, the role of SCFA in pregnancy is still poorly understood. SCFA are derived from fermentation of carbohydrates and proteins by the gut microorganisms (73). They provide energy to colonocytes and maintain intestinal homeostasis by acting as signaling molecules that transmit messages between microbiota and host organs (74). We found positive correlations of valerate with genus Akkermansia and archaeon Methanobrevibacter in pregnant women with impaired FPG in the first and third trimester, respectively. In the GDM2 group, genus Phascolarctobacterium positively correlated with 2-hydroxybutyrate levels. In the study of Dudzik et al. (2017), an increase in 2-hydroxybutyrate in patients with diagnosed GDM in the second trimester of pregnancy was detected. Moreover, 2-hydroxybutyrate levels were significantly higher in GDM women that developed T2DM after parturition. Therefore, 2-hydroxybutyrate may serve as a prognostic tool for the prediction of early onset of the complications related to diabetes in women with GDM after delivery (75).
Overall, our study revealed significant differences in gut bacterial and fungal microbiota composition between healthy pregnant women and women who develop GDM in the first half of pregnancy. Furthermore, we identified correlations between individual microorganisms and plasma biochemical parameters, including SCFA levels. We found several microbial patterns that could be used in specific diagnostic test in the first trimester to identify women in higher risk of GDM. Nevertheless, our results need to be validated by further studies.
The datasets presented in this study can be found in online repositories. The names of the repository/repositories and accession number(s) can be found below: https://www.ncbi.nlm.nih.gov/bioproject/PRJNA833950.
The studies involving human participants were reviewed and approved by Ethics Committee at University Hospital Olomouc, Olomouc, Czechia (approval no. 120/17). The patients/participants provided their written informed consent to participate in this study.
KK and DK designed the study. OK and DK collected clinical samples and biochemical data. NG and MV processed the samples for microbiota sequencing. MK processed sequencing data and bioinformatics. EI, DF and JF processed fatty acids analysis. MV, RR, ZZ, DF, MH and KK drafted the manuscript. All the authors read and approved the final version of the manuscript.
This work was supported by the Ministry of Health of the Czech Republic (grant number NV18-01-00139), by Institute of Microbiology of the Czech Academy of Sciences Institutional Research Concept (RVO: 61388971), and by University Hospital Olomouc, MH CZ – DRO (FNOl, 00098892).
The authors declare that the research was conducted in the absence of any commercial or financial relationships that could be construed as a potential conflict of interest.
All claims expressed in this article are solely those of the authors and do not necessarily represent those of their affiliated organizations, or those of the publisher, the editors and the reviewers. Any product that may be evaluated in this article, or claim that may be made by its manufacturer, is not guaranteed or endorsed by the publisher.
The Supplementary Material for this article can be found online at: https://www.frontiersin.org/articles/10.3389/fendo.2022.970825/full#supplementary-material
1. Jovanovic L, Pettitt DJ. Gestational diabetes mellitus. JAMA (2001) 286(20):2516–8. doi: 10.1001/jama.286.20.2516
2. American Diabetes Association. Diagnosis and classification of diabetes mellitus. Diabetes Care (2009) 32 Suppl 1:S62–7. doi: 10.2337/dc09-S062
3. Metzger BE, Lowe LP, Dyer AR, Trimble ER, Chaovarindr U, Coustan DR, et al. Hyperglycemia and adverse pregnancy outcomes. N Engl J Med (2008) 358(19):1991–2002. doi: 10.1056/NEJMoa0707943
4. Dabelea D, Pettitt DJ. Intrauterine diabetic environment confers risks for type 2 diabetes mellitus and obesity in the offspring, in addition to genetic susceptibility. J Pediatr Endocrinol Metab (2001) 14(8):1085–91. doi: 10.1515/jpem-2001-0803
5. Damm P, Houshmand-Oeregaard A, Kelstrup L, Lauenborg J, Mathiesen ER, Clausen TD. Gestational diabetes mellitus and long-term consequences for mother and offspring: a view from Denmark. Diabetologia (2016) 59(7):1396–9. doi: 10.1007/s00125-016-3985-5
6. Lauenborg J, Hansen T, Jensen DM, Vestergaard H, Molsted-Pedersen L, Hornnes P, et al. Increasing incidence of diabetes after gestational diabetes: a long-term follow-up in a Danish population. Diabetes Care (2004) 27(5):1194–9. doi: 10.2337/diacare.27.5.1194
7. Dabelea D. The predisposition to obesity and diabetes in offspring of diabetic mothers. Diabetes Care (2007) 30 Suppl 2:S169–74. doi: 10.2337/dc07-s211
8. Schaefer-Graf UM, Hartmann R, Pawliczak J, Passow D, Abou-Dakn M, Vetter K, et al. Association of breast-feeding and early childhood overweight in children from mothers with gestational diabetes mellitus. Diabetes Care (2006) 29(5):1105–7. doi: 10.2337/dc05-2413
9. Young TK, Martens PJ, Taback SP, Sellers EA, Dean HJ, Cheang M, et al. Type 2 diabetes mellitus in children: prenatal and early infancy risk factors among native canadians. Arch Pediatr Adolesc Med (2002) 156(7):651–5. doi: 10.1001/archpedi.156.7.651
10. Kordy K, Gaufin T, Mwangi M, Li F, Cerini C, Lee DJ, et al. Contributions to human breast milk microbiome and enteromammary transfer of bifidobacterium breve. PloS One (2020) 15(1):e0219633. doi: 10.1371/journal.pone.0219633
11. Parnanen K, Karkman A, Hultman J, Lyra C, Bengtsson-Palme J, Larsson DGJ, et al. Maternal gut and breast milk microbiota affect infant gut antibiotic resistome and mobile genetic elements. Nat Commun (2018) 9(1):3891. doi: 10.1038/s41467-018-06393-w
12. Wang J, Zheng J, Shi W, Du N, Xu X, Zhang Y, et al. Dysbiosis of maternal and neonatal microbiota associated with gestational diabetes mellitus. Gut (2018) 67(9):1614–25. doi: 10.1136/gutjnl-2018-315988
13. Mor G, Cardenas I. The immune system in pregnancy: a unique complexity. Am J Reprod Immunol (2010) 63(6):425–33. doi: 10.1111/j.1600-0897.2010.00836.x
14. Lain KY, Catalano PM. Metabolic changes in pregnancy. Clin Obstet Gynecol (2007) 50(4):938–48. doi: 10.1097/GRF.0b013e31815a5494
15. Hasain Z, Mokhtar NM, Kamaruddin NA, Mohamed Ismail NA, Razalli NH, Gnanou JV, et al. Gut microbiota and gestational diabetes mellitus: A review of host-gut microbiota interactions and their therapeutic potential. Front Cell Infect Microbiol (2020) 10:188. doi: 10.3389/fcimb.2020.00188
16. Plows JF, Stanley JL, Baker PN, Reynolds CM, Vickers MH. The pathophysiology of gestational diabetes mellitus. Int J Mol Sci (2018) 19(11): 3342. doi: 10.3390/ijms19113342
17. Allin KH, Tremaroli V, Caesar R, Jensen BAH, Damgaard MTF, Bahl MI, et al. Aberrant intestinal microbiota in individuals with prediabetes. Diabetologia (2018) 61(4):810–20. doi: 10.1007/s00125-018-4550-1
18. Karlsson FH, Tremaroli V, Nookaew I, Bergstrom G, Behre CJ, Fagerberg B, et al. Gut metagenome in European women with normal, impaired and diabetic glucose control. Nature (2013) 498(7452):99–103. doi: 10.1038/nature12198
19. Qin J, Li Y, Cai Z, Li S, Zhu J, Zhang F, et al. A metagenome-wide association study of gut microbiota in type 2 diabetes. Nature (2012) 490(7418):55–60. doi: 10.1038/nature11450
20. Koren O, Goodrich JK, Cullender TC, Spor A, Laitinen K, Backhed HK, et al. Host remodeling of the gut microbiome and metabolic changes during pregnancy. Cell (2012) 150(3):470–80. doi: 10.1016/j.cell.2012.07.008
21. Crusell MKW, Hansen TH, Nielsen T, Allin KH, Ruhlemann MC, Damm P, et al. Gestational diabetes is associated with change in the gut microbiota composition in third trimester of pregnancy and postpartum. Microbiome (2018) 6(1):89. doi: 10.1186/s40168-018-0472-x
22. Abdullah B, Daud S, Aazmi MS, Idorus MY, Mahamooth MIJ. Gut microbiota in pregnant Malaysian women: a comparison between trimesters, body mass index and gestational diabetes status. BMC Pregnancy Childbirth (2022) 22(1):152. doi: 10.1186/s12884-022-04472-x
23. Canfora EE, Jocken JW, Blaak EE. Short-chain fatty acids in control of body weight and insulin sensitivity. Nat Rev Endocrinol (2015) 11(10):577–91. doi: 10.1038/nrendo.2015.128
24. Macfarlane S, Macfarlane GT. Regulation of short-chain fatty acid production. Proc Nutr Soc (2003) 62(1):67–72. doi: 10.1079/PNS2002207
25. Hernandez TL, Mande A, Barbour LA. Nutrition therapy within and beyond gestational diabetes. Diabetes Res Clin Pract (2018) 145:39–50. doi: 10.1016/j.diabres.2018.04.004
26. Ponzo V, Fedele D, Goitre I, Leone F, Lezo A, Monzeglio C, et al. Diet-gut microbiota interactions and gestational diabetes mellitus (GDM). Nutrients (2019) 11(2):330. doi: 10.3390/nu11020330
27. Metzger BE, Gabbe SG, Persson B, Buchanan TA, Catalano PA, Damm P, et al. International association of diabetes and pregnancy study groups recommendations on the diagnosis and classification of hyperglycemia in pregnancy. Diabetes Care (2010) 33(3):676–82. doi: 10.2337/dc10-0719
28. Ivanovová E, Piskláková B, Friedecká J, Krystyník O, Friedecký D, Karásek D. Plasma short-chain fatty acids and their derivatives in women with gestational diabetes mellitus. Separations (2021) 8(10):188. doi: 10.3390/separations8100188
29. Caporaso JG, Kuczynski J, Stombaugh J, Bittinger K, Bushman FD, Costello EK, et al. QIIME allows analysis of high-throughput community sequencing data. Nat Methods (2010) 7(5):335–6. doi: 10.1038/nmeth.f.303
30. Edgar RC, Flyvbjerg H. Error filtering, pair assembly and error correction for next-generation sequencing reads. Bioinformatics (2015) 31(21):3476–82. doi: 10.1093/bioinformatics/btv401
31. Bengtsson-Palme JRM, Hartmann M, Branco S, Wang Z, Godhe A, De Wit P, et al. Improved software detection and extraction of ITS1 and ITS2 from ribosomal ITS sequences of fungi and other eukaryotes for analysis of environmental sequencing data. Methods Ecol Evol (2013) 4):914–9. doi: 10.1111/2041-210X.12073
32. Wang Q GG, Tiedje JM, Cole JR. Naïve Bayesian classifier for rapid assignment of rRNA sequences into the new bacterial taxonomy. Appl Environ Microbiol (2007) 73(16):5261–7. doi: 10.1128/AEM.00062-07
33. DeSantis TZ, Hugenholtz P, Larsen N, Rojas M, Brodie EL, Keller K, et al. Greengenes, a chimera-checked 16S rRNA gene database and workbench compatible with ARB. Appl Environ Microbiol (2006) 72(7):5069–72. doi: 10.1128/AEM.03006-05
34. Segata N, Izard J, Waldron L, Gevers D, Miropolsky L, Garrett WS, et al. Metagenomic biomarker discovery and explanation. Genome Biol (2011) 12(6):R60. doi: 10.1186/gb-2011-12-6-r60
35. Findley K, Oh J, Yang J, Conlan S, Deming C, Meyer JA, et al. Topographic diversity of fungal and bacterial communities in human skin. Nature (2013) 498(7454):367–70. doi: 10.1038/nature12171
36. Medici Dualib P, Ogassavara J, Mattar R, Mariko Koga da Silva E, Atala Dib S, de Almeida Pititto B. Gut microbiota and gestational diabetes mellitus: A systematic review. Diabetes Res Clin Pract (2021) 180:109078. doi: 10.1016/j.diabres.2021.109078
37. Rold LS, Bundgaard-Nielsen C, Niemann Holm-Jacobsen J, Glud Ovesen P, Leutscher P, Hagstrom S, et al. Characteristics of the gut microbiome in women with gestational diabetes mellitus: A systematic review. PLoS One (2022) 17(1):e0262618. doi: 10.1371/journal.pone.0262618
38. Arumugam M, Raes J, Pelletier E, Le Paslier D, Yamada T, Mende DR, et al. Enterotypes of the human gut microbiome. Nature (2011) 473(7346):174–80. doi: 10.1038/nature09944
39. Zheng W, Xu Q, Huang W, Yan Q, Chen Y, Zhang L, et al. Gestational diabetes mellitus is associated with reduced dynamics of gut microbiota during the first half of pregnancy. mSystems (2020) 5(2): e00109–20. doi: 10.1128/mSystems.00109-20
40. DiGiulio DB, Callahan BJ, McMurdie PJ, Costello EK, Lyell DJ, Robaczewska A, et al. Temporal and spatial variation of the human microbiota during pregnancy. Proc Natl Acad Sci U S A (2015) 112(35):11060–5. doi: 10.1073/pnas.1502875112
41. Wang X, Liu H, Li Y, Huang S, Zhang L, Cao C, et al. Altered gut bacterial and metabolic signatures and their interaction in gestational diabetes mellitus. Gut Microbes (2020) 12(1):1–13. doi: 10.1080/19490976.2020.1840765
42. Cortez RV, Taddei CR, Sparvoli LG, Angelo AGS, Padilha M, Mattar R, et al. Microbiome and its relation to gestational diabetes. Endocrine (2019) 64(2):254–64. doi: 10.1007/s12020-018-1813-z
43. Kuang YS, Lu JH, Li SH, Li JH, Yuan MY, He JR, et al. Connections between the human gut microbiome and gestational diabetes mellitus. Gigascience (2017) 6(8):1–12. doi: 10.1093/gigascience/gix058
44. Gamez-Valdez JS, Garcia-Mazcorro JF, Montoya-Rincon AH, Rodriguez-Reyes DL, Jimenez-Blanco G, Rodriguez MTA, et al. Differential analysis of the bacterial community in colostrum samples from women with gestational diabetes mellitus and obesity. Sci Rep (2021) 11(1):24373. doi: 10.1038/s41598-021-03779-7
45. Ferrocino I, Ponzo V, Gambino R, Zarovska A, Leone F, Monzeglio C, et al. Changes in the gut microbiota composition during pregnancy in patients with gestational diabetes mellitus (GDM). Sci Rep (2018) 8(1):12216. doi: 10.1038/s41598-018-30735-9
46. Wang C, Zhang H, Liu H, Bao Y, Di J, Hu C. The genus sutterella is a potential contributor to glucose metabolism improvement after roux-en-Y gastric bypass surgery in T2D. Diabetes Res Clin Pract (2020) 162:108116. doi: 10.1016/j.diabres.2020.108116
47. Zhang H, DiBaise JK, Zuccolo A, Kudrna D, Braidotti M, Yu Y, et al. Human gut microbiota in obesity and after gastric bypass. Proc Natl Acad Sci U S A (2009) 106(7):2365–70. doi: 10.1073/pnas.0812600106
48. Crovesy L, Masterson D, Rosado EL. Profile of the gut microbiota of adults with obesity: a systematic review. Eur J Clin Nutr (2020) 74(9):1251–62. doi: 10.1038/s41430-020-0607-6
49. Qin J, Li R, Raes J, Arumugam M, Burgdorf KS, Manichanh C, et al. A human gut microbial gene catalogue established by metagenomic sequencing. Nature (2010) 464(7285):59–65. doi: 10.1038/nature08821
50. Mar Rodriguez M, Perez D, Javier Chaves F, Esteve E, Marin-Garcia P, Xifra G, et al. Obesity changes the human gut mycobiome. Sci Rep (2015) 5:14600. doi: 10.1038/srep14600
51. Wu N, Mo H, Mu Q, Liu P, Liu G, Yu W. The gut mycobiome characterization of gestational diabetes mellitus and its association with dietary intervention. Front Microbiol (2022) 13:892859. doi: 10.3389/fmicb.2022.892859
52. Ferrocino I, Ponzo V, Pellegrini M, Goitre I, Papurello M, Franciosa I, et al. Mycobiota composition and changes across pregnancy in patients with gestational diabetes mellitus (GDM). Sci Rep (2022) 12(1):9192. doi: 10.1038/s41598-022-13438-0
53. Erdogan A, Rao SS. Small intestinal fungal overgrowth. Curr Gastroenterol Rep (2015) 17(4):16. doi: 10.1007/s11894-015-0436-2
54. Martins N, Ferreira IC, Barros L, Silva S, Henriques M. Candidiasis: predisposing factors, prevention, diagnosis and alternative treatment. Mycopathologia (2014) 177(5-6):223–40. doi: 10.1007/s11046-014-9749-1
55. Gosiewski T, Salamon D, Szopa M, Sroka A, Malecki MT, Bulanda M. Quantitative evaluation of fungi of the genus candida in the feces of adult patients with type 1 and 2 diabetes - a pilot study. Gut Pathog (2014) 6(1):43. doi: 10.1186/s13099-014-0043-z
56. Soyucen E, Gulcan A, Aktuglu-Zeybek AC, Onal H, Kiykim E, Aydin A. Differences in the gut microbiota of healthy children and those with type 1 diabetes. Pediatr Int (2014) 56(3):336–43. doi: 10.1111/ped.12243
57. Garcia-Gamboa R, Kirchmayr MR, Gradilla-Hernandez MS, Perez-Brocal V, Moya A, Gonzalez-Avila M. The intestinal mycobiota and its relationship with overweight, obesity and nutritional aspects. J Hum Nutr Diet (2021) 34(4):645–55. doi: 10.1111/jhn.12864
58. Li D, Chi XZ, Zhang L, Chen R, Cao JR, Sun XY, et al. Vaginal microbiome analysis of healthy women during different periods of gestation. Biosci Rep (2020) 40(7): BSR20201766. doi: 10.1042/BSR20201766
59. Rodrigues CF, Rodrigues ME, Henriques M. Candida sp. infections in patients with diabetes mellitus. J Clin Med (2019) 8(1):76. doi: 10.3390/jcm8010076
60. Zhang X, Liao Q, Wang F, Li D. Association of gestational diabetes mellitus and abnormal vaginal flora with adverse pregnancy outcomes. Med (Baltimore) (2018) 97(34):e11891. doi: 10.1097/MD.0000000000011891
61. Romani-Perez M, Lopez-Almela I, Bullich-Vilarrubias C, Rueda-Ruzafa L, Gomez Del Pulgar EM, Benitez-Paez A, et al. Holdemanella biformis improves glucose tolerance and regulates GLP-1 signaling in obese mice. FASEB J (2021) 35(7):e21734. doi: 10.1096/fj.202100126R
62. Naderpoor N, Mousa A, Gomez-Arango LF, Barrett HL, Dekker Nitert M, de Courten B. Faecal microbiota are related to insulin sensitivity and secretion in overweight or obese adults. J Clin Med (2019) 8(4):452. doi: 10.3390/jcm8040452
63. Farias DR, Franco-Sena AB, Vilela A, Lepsch J, Mendes RH, Kac G. Lipid changes throughout pregnancy according to pre-pregnancy BMI: results from a prospective cohort. BJOG (2016) 123(4):570–8. doi: 10.1111/1471-0528.13293
64. Vahratian A, Misra VK, Trudeau S, Misra DP. Prepregnancy body mass index and gestational age-dependent changes in lipid levels during pregnancy. Obstet Gynecol (2010) 116(1):107–13. doi: 10.1097/AOG.0b013e3181e45d23
65. Emet T, Ustuner I, Guven SG, Balik G, Ural UM, Tekin YB, et al. Plasma lipids and lipoproteins during pregnancy and related pregnancy outcomes. Arch Gynecol Obstet (2013) 288(1):49–55. doi: 10.1007/s00404-013-2750-y
66. Li G, Kong L, Zhang L, Fan L, Su Y, Rose JC, et al. Early pregnancy maternal lipid profiles and the risk of gestational diabetes mellitus stratified for body mass index. Reprod Sci (2015) 22(6):712–7. doi: 10.1177/1933719114557896
67. Savvidou M, Nelson SM, Makgoba M, Messow CM, Sattar N, Nicolaides K. First-trimester prediction of gestational diabetes mellitus: examining the potential of combining maternal characteristics and laboratory measures. Diabetes (2010) 59(12):3017–22. doi: 10.2337/db10-0688
68. Shen H, Liu X, Chen Y, He B, Cheng W. Associations of lipid levels during gestation with hypertensive disorders of pregnancy and gestational diabetes mellitus: a prospective longitudinal cohort study. BMJ Open (2016) 6(12):e013509. doi: 10.1136/bmjopen-2016-013509
69. Ryckman KK, Spracklen CN, Smith CJ, Robinson JG, Saftlas AF. Maternal lipid levels during pregnancy and gestational diabetes: a systematic review and meta-analysis. BJOG (2015) 122(5):643–51. doi: 10.1111/1471-0528.13261
70. Kenny DJ, Plichta DR, Shungin D, Koppel N, Hall AB, Fu B, et al. Cholesterol metabolism by uncultured human gut bacteria influences host cholesterol level. Cell Host Microbe (2020) 28(2):245–57.e6. doi: 10.1016/j.chom.2020.05.013
71. Le Roy T, Lecuyer E, Chassaing B, Rhimi M, Lhomme M, Boudebbouze S, et al. The intestinal microbiota regulates host cholesterol homeostasis. BMC Biol (2019) 17(1):94. doi: 10.1186/s12915-019-0715-8
72. Van Hul M, Le Roy T, Prifti E, Dao MC, Paquot A, Zucker JD, et al. From correlation to causality: the case of subdoligranulum. Gut Microbes (2020) 12(1):1–13. doi: 10.1080/19490976.2020.1849998
73. He J, Zhang P, Shen L, Niu L, Tan Y, Chen L, et al. Short-chain fatty acids and their association with signalling pathways in inflammation, glucose and lipid metabolism. Int J Mol Sci (2020) 21(17):6356. doi: 10.3390/ijms21176356
74. Parada Venegas D, de la Fuente MK, Landskron G, Gonzalez MJ, Quera R, Dijkstra G, et al. Short chain fatty acids (SCFAs)-mediated gut epithelial and immune regulation and its relevance for inflammatory bowel diseases. Front Immunol (2019) 10:277. doi: 10.3389/fimmu.2019.00277
Keywords: microbiome, mycobiome, early diagnosis, plasma metabolites, short-chain fatty acids, correlation
Citation: Vavreckova M, Galanova N, Kostovcik M, Krystynik O, Ivanovova E, Roubalova R, Jiraskova Zakostelska Z, Friedecky D, Friedecka J, Haluzik M, Karasek D and Kostovcikova K (2022) Specific gut bacterial and fungal microbiota pattern in the first half of pregnancy is linked to the development of gestational diabetes mellitus in the cohort including obese women. Front. Endocrinol. 13:970825. doi: 10.3389/fendo.2022.970825
Received: 16 June 2022; Accepted: 15 August 2022;
Published: 05 September 2022.
Edited by:
A.Seval Ozgu-Erdinc, Ankara City Hospital, TurkeyReviewed by:
Cuauhtemoc Licona-Cassani, Monterrey Institute of Technology and Higher Education (ITESM), MexicoCopyright © 2022 Vavreckova, Galanova, Kostovcik, Krystynik, Ivanovova, Roubalova, Jiraskova Zakostelska, Friedecky, Friedecka, Haluzik, Karasek and Kostovcikova. This is an open-access article distributed under the terms of the Creative Commons Attribution License (CC BY). The use, distribution or reproduction in other forums is permitted, provided the original author(s) and the copyright owner(s) are credited and that the original publication in this journal is cited, in accordance with accepted academic practice. No use, distribution or reproduction is permitted which does not comply with these terms.
*Correspondence: Klara Kostovcikova, a2xpbWVzb3ZAYmlvbWVkLmNhcy5jeg==
Disclaimer: All claims expressed in this article are solely those of the authors and do not necessarily represent those of their affiliated organizations, or those of the publisher, the editors and the reviewers. Any product that may be evaluated in this article or claim that may be made by its manufacturer is not guaranteed or endorsed by the publisher.
Research integrity at Frontiers
Learn more about the work of our research integrity team to safeguard the quality of each article we publish.