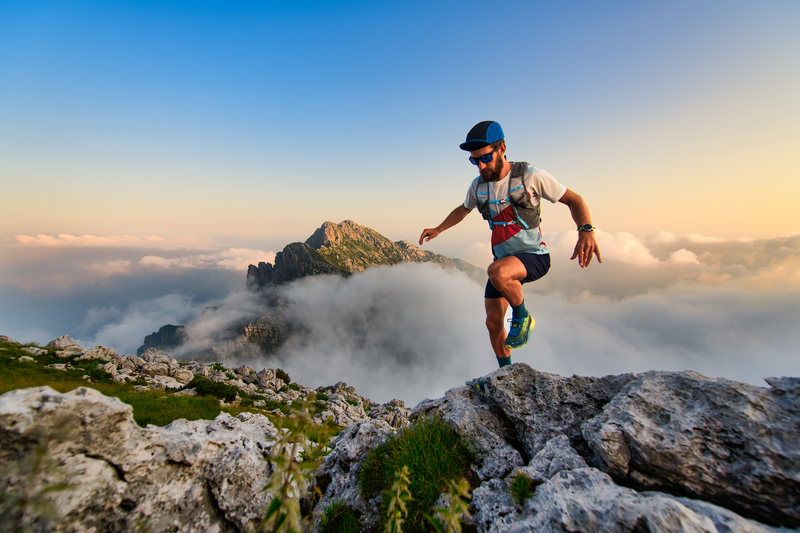
94% of researchers rate our articles as excellent or good
Learn more about the work of our research integrity team to safeguard the quality of each article we publish.
Find out more
ORIGINAL RESEARCH article
Front. Endocrinol. , 26 July 2022
Sec. Experimental Endocrinology
Volume 13 - 2022 | https://doi.org/10.3389/fendo.2022.963827
This article is part of the Research Topic Environmental Stressors and Metabolic Disease View all 8 articles
Background: Fine particulate matter (PM2.5), one of the most common air pollutants worldwide, has been associated with many adverse birth outcomes in some studies. Pre-pregnancy body mass index (BMI) is an important indicator of maternal obesity that may also contribute to a wide range of birthweight outcomes. Both PM2.5 and maternal obesity have been found associated with issues on neonatal birthweight respectively, and more attentions and interests are focusing on their combined effect on pregnancy outcomes.
Purpose: To explore the modifying effect of pre-pregnancy BMI on the association between gestational PM2.5 and birthweight; to investigate the interactive effect between gestational PM2.5 and pre-pregnancy BMI on birthweight among pregnant women during three trimesters and the whole pregnancy.
Methods: This nationwide cohort study used the National Free Preconception Health Examination Project (NFPHEP) data collected from January 1, 2010, to December 31, 2012. A total population of 248,501 Chinese women from 220 counties registered this project. Pre-pregnancy BMI as a common anthropometric examination was collected during preconception investigation, and gestational PM2.5 was derived from a hindcast model for historical PM2.5 estimation from satellite-retrieved aerosol optic depth. Subgroup analysis was conducted to explore a potential modifying effect on the association between PM2.5 and birthweight during pregnancy by four pre-pregnancy BMI subgroups. Interaction analysis by introducing product terms to multivariable linear regression was also used to examine whether there was an interactive relationship between PM2.5 and pre-pregnancy BMI.
Results: Totally, 193,461 participants were included in our study. The average concentration of PM2.5 was 75.33 μg/m3. Higher exposure of PM2.5 during the entire pregnancy was associated with higher birthweight (17.15 g per 10 μg/m3; 95% CI:16.15, 18.17). Each 10 μg/m3 increase in PM2.5 during the first, second, and third trimesters was associated with increases in birthweight by 14.93 g (95%CI: 13.96, 15.89), 13.75 g (95% CI: 12.81, 14.69), and 8.79 g (95% CI: 8.09, 9.49), respectively. Higher pre-pregnancy BMI per kg/m2 was associated with an increase of birthweight by 7.012 g (95% CI: 6.121, 7.902). Product terms between PM2.5 and pre-pregnancy BMI were significant for the first, second trimesters, and the entire duration of pregnancy.
Conclusions: Our results found both gestational PM2.5 exposure and pre-pregnancy BMI respectively correlated with the increase of birthweight. A negative interaction between pre-pregnancy BMI and gestational PM2.5 was discovered in term of birthweight gain. Avoidance of high-dose exposure to PM2.5 during the early and middle stages of pregnancy and pre-pregnancy overweight/obesity may help prevent high birthweight.
Birthweight is an important determinant of both maternal and neonatal health as well as lifelong well-being. High birthweight has been proved to be strongly correlated with health conditions such as cardiovascular diseases, obesity, type 2 diabetes mellitus (1, 2). High birthweight coupled with postnatal growth may increase the early presence of cardiometabolic risk factors and vascular imprinting (3). Studies have showed that the increased prevalence of high birthweight or large for gestational age (LGA) becomes a worldwide health issue (4). The prevalence of macrosomia in China was 6.9% in 2007-2008, and ranged from 7.3% to 8.7% in 2010-2014, higher than the average of 23 low-income and middle-income countries (5, 6), while the incidence of low birthweight in babies (≥28 gestational weeks) was unchanged between 2012-2018 at around 5.5% (5). Birthweight is closely related to intrauterine environment, whose establishment is rooted in multi-factorial interactions including maternal metabolic status, genetic expression, life style, and also physical environment (7–9).
Recently, studies on the combined effect of metabolism and environmental factors on health have increased, of which maternal obesity and air pollution such as PM2.5 attract a lot of attention (10, 11). Obesity has emerged as a major issue. From 1995 to 2014, the prevalence of overweight raised from 4.2% to 14.0% and the obesity rate from 1.0% to 6.4% (5). Pre-pregnancy BMI, as an acceptable indicator of metabolic gradations of thinness and fatness, has profound effects on both mothers and fetuses by influencing the intrauterine environment. Pre-pregnancy overweight/obesity was found associated with higher risks of maternal gestational diabetes mellitus, pre-eclampsia, post-delivery weight retention and dysglycemia (12–14). Adverse outcomes on fetus due to pre-gestational overweight/obese could be preterm birth, stillbirth, caesarean delivery and LGA (15, 16). As for the environmental factors, adverse impacts of PM2.5 on public health have been huge concerns worldwide, especially in developing countries. However, studies on the adverse effects of gestational PM2.5 exposure on birthweight can be controversial and inconsistent (17, 18). Majority of studies demonstrated correlations between PM2.5 and low birthweight or small for gestational age (SGA) (19–25), while some studies confirmed associations between PM2.5 and LGA or macrosomia (10, 17, 18, 26). Previous studies pointed out that marked differences on PM2.5 pollution between rapidly developing and developed countries might cause inconsistency in results (17, 18). Air pollution was an extremely serious issue of China during 2010s, when many provinces and cities had annual average PM2.5 over 80 μg/m3 (18). A recent study showed that the five most polluted megacities (Delhi, Cairo, Xi’an, Tianjin and Chengdu) all had an annual average concentration of PM2.5 greater than 89 μg/m3 in 2013, while PM2.5 in many other countries could be much lower (23, 24, 27, 28). Race is another difference between countries and studies exemplified by the fact that the Asian prevalence of overweight/obesity is far less than Caucasian (29). Studies on the combination effect between gestational weight gain (GWG) and air pollutions such as PM2.5 have reported similar interactions on birth outcomes, and we hope to take further steps in exploring a potential interaction of Chinese population between maternal obesity and air pollutions on birthweight.
Our study was based on a large national cohort during 2010s when air pollution was extremely serious in China, compared to previously reported studies in developed countries. Combining both maternal metabolic status and atmospheric environment, it is necessary to explore their effects on birthweight respectively and jointly. We aimed to explore whether higher dose of PM2.5 exposure during pregnancy was associated with growth in birthweight nationwide in China; to explore a potential interaction effect between pre-pregnancy BMI and PM2.5 on birthweight.
Data for this national cohort study were derived from the National Free Preconception Health Examination Project (NFPHEP) operated by the National Research Institute for Family Planning and conducted in 220 counties from 31 provinces and province-level municipalities. The project was launched by the National Health Commission of China, lasted for 3 years from January 1, 2010, to December 31, 2012, with the aim to provide free preconception health examinations and follow-up of pregnancy outcomes for married couples planning a pregnancy within next 6 months. The health examinations were conducted by trained and qualified staff and the data was collected in a face-to-face way of investigation, including 371 items in total such as maternal medical history, contraception measures, familial disorders, physical and laboratory examinations, life style and demographic characteristics of parents as well as neonatal birth outcomes. Other detailed information on design, organization and implementation of this project were recorded elsewhere (30–32). The study was approved by the institutional review board of the National Research Institute for Family Planning, Beijing, China and received formal consent from the participants.
The outcome of our study was birthweight in continuous values recorded by information recorders. Infants with implausible birthweights (≤500 g or ≥5,000 g) were excluded for sensitivity analysis. PM2.5 concentrations in 31 provinces were obtained from the Chinese Center for Disease Control and Prevention estimating historical PM2.5 concentrations in China from satellite data by using an ensemble machine-learning model (33). The missing satellite data were filled by multiple imputation. The modeling domain was divided into seven regions using a spatial clustering method, and a set of machine learning models were trained in each region separately. A spatial cluster-based model was expected to capture the spatiotemporal variation in PM2.5 more accurately by controlling unobserved spatial heterogeneity. To put it simple, the machine-learning model was composed of two parts: three prediction models and the ensemble model. The prediction models for PM2.5 concentrations included generalized additive model, random forest model and extreme gradient boosting model, and the ensemble model was an ensemble GAM model combining the three individual models, which could obtain a spatially continuous prediction surface. The model was trained by satellite data and PM2.5 ground monitoring records at 1,593 monitoring stations across mainland China from 2013 to 2016 and data during the first months of 2017 was applied for hindcast evaluation. PM2.5 concentrations in 2008 in Beijing was also obtained to evaluate the model. The final ensemble prediction characterized the spatiotemporal distribution of daily PM2.5 well with the cross-validation (CV) R2 0.76, root mean square error, RMSE 16 μg/m3. Daily county-specific PM2.5 concentrations were collected and calculated in the form of trimester-specific mean values, in which first trimester refers to 1-3 months’ gestation, second trimester refers to 4-6 months’ gestation, and the third trimester refers to the rest months of gestation. In our study, subjects who moved during the follow-up were excluded to ensure the homogeneity of prenatal living environment. Pre-pregnancy BMI was segmented into four groups (BMI<18.5 kg/m2, BMI 18.5-23.9 kg/m2, BMI 24.0-28.0 kg/m2, BMI>28.0 kg/m2), corresponding to four distinct BMI levels, “underweight”, “normal”, “overweight” and “obese” respectively (31).
The variables selected and analyzed in our study were maternal and neonatal characteristics such as neonate’s sex, maternal age, gestational week, educational level, maternal smoking or alcohol intake during pregnancy, multiparity, pre-pregnancy diabetes mellitus, pre-pregnancy hypertension, birth weight, the season of delivery.
Unadjusted and adjusted linear regressions were used to evaluate the associations between trimester-specific PM2.5 concentrations and neonatal birthweight as well as the association between pre-pregnancy BMI and birthweight. The confounding factors for adjustment included maternal age at delivery, neonatal sex (male, female), smoking or alcohol intake during pregnancy (still, quit, never), gestational week, maternal educational level (junior high school, senior high school, college), prolonged pregnancy (yes, no), multiparity (yes, no), pre-pregnancy diabetes mellitus (yes, no), and pre-pregnancy hypertension (yes, no) (17, 18). The dose-response relationships between PM2.5 concentration and birthweight were further investigated using restricted cubic spline models (node was 4). Subgroup analysis was conducted in four BMI intervals (“underweight”, “normal”, “overweight” and “obese”) to explore whether the dose-response relations between PM2.5 and birthweight would change under different BMI levels. Segments of PM2.5 concentrations used for calculating the average birthweights for each segment were conducted based on the IAQI (individual air quality index) recommended by WHO, which were 0-35 μg/m3 (Chinese guideline II), 35-75 μg/m3 (mild), 75-115 μg/m3 (moderate), 115-150 μg/m3 (severe) (34, 35). Multivariable linear regression was conducted involving main effect items, interaction effect item, and other confounding factors for adjustments. Centralized variables were multiplied as an interactive item and added in a regression model. β coefficients and 95% CI were reported. Sensitivity analysis was conducted by excluding some extreme observations in birthweight (≤500 g or ≥5,000 g). Multivariable linear regressions of PM2.5 and pre-pregnancy BMI with birthweight (without outliers), and interaction analysis between PM2.5 and pre-pregnancy BMI were conducted.
The data cleaning process and descriptive analysis were conducted using SPSS 26.0 (SPSS, Inc., Chicago, IL, USA). Absolute standardized difference was used to check the imbalance of baseline characteristics among different pre-pregnancy BMI subgroups, and a value larger than 0.1 was regarded as baseline imbalance (36). Since absolute standardized difference can only be calculated between two groups, the maximum value among different groups was used. Linear regressions, restricted cubic spline curves, interaction effect analysis and subgroup analysis were all performed using R version 4.1.2 (R Foundation for Statistical Computing, Vienna, Austria), and “foreign”, “rms”, “ggplo2”, “survival”, “MASS”, “splines” packages were applied in our analysis. A two-sided P-value <0.05 was considered statistically significant.
The process of inclusion and exclusion of participants was recorded in Figure 1. The distribution of PM2.5 across the entire pregnancy in China was calculated according to different provinces and recorded in supplementary materials (Table S1). An initial population of 248,501 participants were preliminarily screened by a standard of exclusion and inclusion in a flowchart (Figure 1). The definition of loss to follow-up was that participants had preconception examination but had not received pre-natal or post-natal examination and questionnaires yet by 1 month after the expected data of confinement. Participants whose birthplaces and follow-up places did not match were excluded. 241,587 participants were further screened by removing subjects of loss to follow-up (5,357), birth defects (254), medical abortions (2,476), induced labor (433), ectopic pregnancies (171), still births (574), preterm births (3,570), and non-singleton births (8,871). The selection process was further conducted by removing participants with unclear neonatal sex (15,326), extreme maternal age (<16 or >50) at delivery or missing (1,622), extreme pre-BMI (<12 or >50) or missing (6,688), unreported cigarette or alcohol consumption (1,521), unreported education levels (1,258) and unreported gestational week (5). The final 193,461 participants were included in our study. The distribution of PM2.5 over the entire pregnancy in 29 provinces of China was recorded in Table S1 (two provinces were not listed due to small numbers involved in our study). There were 15 provinces and 50,328 (26.0%) participants correspondingly having their average PM2.5 lower than 60 μg/m3.
Table 1 showed the baseline characteristics of pregnancy women and neonates included and excluded in our study. Male neonates (n=102,203) take the percentage of 52.8% of the population, which were more than female neonates (n=91,258). The mean duration of gestation was 39.25 weeks. The average birth weight of overall included newborns (n=193,461) was 3326.66 g. The overall average pre-pregnancy BMI was 21.04 kg/m2. The mean PM2.5 concentrations in each trimester and whole pregnancy were also calculated and the PM2.5 concentration in the third trimester was the highest among four periods. Other characteristics were also clearly shown in Table 1.
Both unadjusted and adjusted multivariable linear regression models presented in Table 2 indicated that PM2.5 was positively associated with birthweight. After adjusting for all confounding factors, every 10 μg/m3 increase in trimester-specific exposure to PM2.5 on birthweight was associated with increases in birthweight of 14.93 g (95%CI: 13.96, 15.89), 13.75 g(95%CI: 12.81, 14.69), and 8.79 g (95% CI: 8.09, 9.49) in the first, second, and third trimesters respectively. The newborn birthweight gained 17.15 g (95% CI: 16.15, 18.17) for every 10 μg/m3 rise of PM2.5 exposure throughout the entire pregnancy. Restricted cubic spline curves (Figure 2) illustrated nonlinear relationships between increase in birth weight and PM2.5. Curves for the first (A), second (B), and third trimesters (C) showed almost the same changing patterns, rising to a plateau at 50-60 μg/m3 of PM2.5 and keeping stable afterward. Variations at the terminal of the curves were considerable. However, the dose-response curve between PM2.5 and birthweight across the entire pregnancy (D) showed a nearly linear growth.
Table 2 Linear regression between PM2.5 concentration and birth weight a.
Figure 2 Dose-response relationship between PM2.5 concentration and birth weight in first trimester (A), second trimester (B), third trimester (C), and whole pregnancy (D), using cubic restricted model, adjusted for maternal age at delivery, neonatal sex, smoking during pregnancy, drinking during pregnancy, gestational week, pre-pregnancy BMI, educational level, prolonged pregnancy, multiparity, pre-pregnancy diabetes mellitus, pre-pregnancy hypertension, seasons.
Multivariable linear regressions were also performed between pre-pregnancy BMI and birthweight with adjusted β coefficients 7.219 (95% CI: 6.342, 8.096) and adjusted β coefficient 7.012 (95% CI: 6.121, 7.902) (Table 3). Subgroup analysis was conducted in BMI-stratified subgroups (baseline characteristics shown in Table S2 (supplementary material), which were classified as underweight (n=25,821), normal (n=146,081), overweight (n=18,187), obese (n=3,372). PM2.5 was divided into four specific groups with an interval of about 40 μg/m3 based on the air quality index (AQI) derived from a four-year (January 2015-December 2018) EAQ data of Eastern China (EC) (9). In each subgroup, mean values (± 2SE, standard error) of birthweight were calculated at four PM2.5 groups of the whole pregnancy and demonstrated in a broken-line graph (Figure 3). Each subgroup presented a generally increasing trend of mean birth weight as PM2.5 levels rose, and such an increasing pattern was less obvious and even turned to decrease as the BMI levels reached “overweight” and finally “obese”. It was also clearly observed that variations increased as sample size of subgroups decreased, suggesting less convincing patterns in small-sized groups. Therefore, to examine a potential modifying effect of pre-pregnancy BMI on the association between PM2.5 and birthweight, an interaction analysis was further conducted.
Table 3 Linear regression between pre-pregnancy BMI (kg/m2) and birthweight a.
Figure 3 Mean birthweights by each PM2.5 level in four BMI subgroups. Four PM2.5 levels were: 0-35 (Chinese guideline II), 35-75 (mild), 75-115 (moderate), 115-150 (severe).
Table 4 showed the interaction between pre-pregnancy BMI and PM2.5 exposure in three trimesters and the whole pregnancy on the increase of birth weight. In the first trimester, the interaction effect was statistically significant between exposure to PM2.5 and pre-pregnancy BMI (β=-0.033; 95% CI, -0.048, -0.018; p=0.0.030), showing a negative interaction in which PM2.5 might have less increasing impact on birthweight affected by different levels of pre-pregnancy BMI. Similar interactions could also be seen in both second trimester (P<0.001) and whole pregnancy (P=0.001), while the third trimester showed no significant interaction (p=0.329). The interaction effects were shown in Figure 4. The slopes of regression lines decreased as BMI increased from 18.5 to 28.0, illustrating negative interactions in the first, second trimesters and the whole pregnancy. In sensitivity analysis including 192,326 women and neonates whose birthweight ranged between 530 and 4970 kg/m3, the results were consistent with the main findings (Tables S3, 4).
Figure 4 Interaction effects between PM2.5 and pre-pregnancy BMI in the first (A), second (B) trimesters and the whole pregnancy (C).
Our study innovatively explored the effect of intrauterine environment on neonatal birthweight from both the metabolic and physical environmental factors. An interaction between pre-pregnancy BMI and PM2.5 was discovered in the first and second trimesters as well as the whole pregnancy. We also found a nonlinear relationship between PM2.5 and birthweight increase, confirming the positive correlation between PM2.5 exposure and fetal weight gain. Over 190,000 pregnant women were included in our study, and the wide distribution of participants across the country provided good external validity.
First, the positive association between gestational PM2.5 and the neonatal birthweight denoted that exposure to PM2.5 during pregnancy related to higher risks of high birthweight and LGA. It should be noticed that birthweight increase should not be equal to better outcomes and high birthweight entailed higher risks of mortality and morbidity (35). The wide range of monthly-average PM2.5 in our study could cover from 0 to 210 μg/m3, while PM2.5 levels in many developed countries as well as some developing countries were far less than that in China (24, 25, 37). The restricted cubic spline curves generally presented an increasing trend, although appeared to reach a plateau at about 50-60 μg/m3 of PM2.5 (at the mild level). The curves implied that birthweight was more sensitive to slight or mild PM2.5 pollution, and pregnant women should be alert even at a slightly mild level of air pollution. It was noticed that PM2.5 exposure was highest in the third trimester. Seasonality is an important factor in which heavy PM2.5 usually occurs during more polluted seasons such as winter (19). Our data presented a higher percentage of neonates born in winter, which could partially explain a significant increase of PM2.5 exposure in the third trimester. A similar increasing effect of pre-pregnancy BMI on birthweight was also found in three trimesters. A study by Yu et al. found that pre-pregnancy overweight or obese increased the risk of LGA (OR=1.53; 95%, 1.44-1.63), high birthweight (OR=2.00; 95% CI, 1.84-2.18), macrosomia (OR=1.67; 95% 1.42-1.97), and probably subsequent offspring overweight or obese (38). In addition, maternal weight gain during pregnancy was found as a mediator between pre-pregnancy BMI and birthweight increase (39).
In our study, a negative interaction effect was unprecedentedly established between gestational PM2.5 exposure and pre-pregnancy BMI on neonatal birthweight in the first, second trimesters and the whole pregnancy, in which pre-pregnancy BMI compromised the increasing effect of PM2.5 on birthweight. Pregnancy is a well-known stage of susceptibility in oxidative stress, mainly produced by a normal systemic inflammatory response (40). Obesity is characterized by chronic inflammation along with many other metabolic syndromes such as diabetes mellitus and hypertension (11). The interpretation of such a negative interaction can be put in two aspects: the lifestyle and biological factors. It is well-known that obesity is related to an individual’s living habits. Overweight or obese people may have unhealthy lifestyle habits such as overeating and less outdoor exercise, and the amount of outdoor exercise directly affects the amount of PM2.5 exposure. Therefore, we speculate that overweight and obese individuals have reduced PM2.5 exposure by spending less time outdoors compared to normal weight, thereby weakening the original role of PM2.5 in increasing birthweight. For the biological factors, it has been discovered that maternal metabolic environment affects ovum production, gene expression of zygote and placental development during pregnancy (8). Obesity increases the susceptibility of environmental pollution during the pregnancy mainly by epigenetic inheritance in fetus (41). Studies have shown that the activity of insulin-sensitive genes in obese pregnant women is significantly down-regulated in early pregnancy, leading to elevated insulin levels (42). High insulin levels enhance the activity of the IGF-1 pathway in obese pregnant women and affect fetal growth and development through the placenta, increasing the risk of fetal overweight (43). Elevated concentrations of TNF-α, IL-1b, IL-6 and leptin may also worsen insulin resistance and increase fetal overgrowth (44). Similarly, the mechanism of how PM2.5 affects fetal weight can also attribute to epigenetic inheritance. Recent studies have found that PM2.5 may affect fetal growth and development by changing fetal IGF2 gene expression level (41). IGF-2 is a growth factor in homology with IGF-1, which is expressed by an imprinted gene on paternal chromosome 11 (45). The loss of paternalistic imprinting leads to IGF-2 overexpression and fetal overgrowth (45). IGF-1 receptor (IGF1R) is widely expressed in different tissues and both insulin, IGF-1 and IGF-2 can bind to IFG1R to activate downstream signal transduction, while IGH-2 receptor can only be specifically bound by IGF-2 (46). Based on these evidence, we conclude that obese women with pre-pregnancy BMI may increase maternal and placental deposition of metabolites such as lipids through high levels of IGF-1, and this strong effect may cover up the PM2.5’s effect on IGF-2 gene expression. However, the mechanism of environmental factors and metabolic factors on fetal growth and development is rather complex. Further research is necessary to explore the mechanisms of biological interaction during the pregnancy. It is noteworthy that there is no interaction found in the third trimester, which should be the crucial period of fetal growth and least affected by genetic factors. These mechanisms suggest that such an interaction may interfere the early and middle stage of pregnancy by mainly affecting the regulation of epigenetics and growth factors and thus play a profound role in late pregnancy as well as postnatal growth.
This is the first analysis to our knowledge of the potential interaction between pre-pregnancy BMI and gestational PM2.5 in a large Chinese cohort study. Interactions between pre-pregnancy BMI and many other factors were also discovered by previous studies. An interaction between pre-pregnancy BMI and gestational passive smoking was found related to macrosomia and LGA (47) and the effect of smoking during pregnancy on SGA and birth weight was markedly reduced among obese and overweight women (48). Interactive effects between different types of metabolic disorders were also assessed. Pre-pregnancy overweight/obesity and hypertensive disorders jointly increasing the risks of obesity and hypertension in offspring or throughout their life course (28, 49). In our study, the negative interaction effect implied a biological interaction between in vivo and in vitro factors, which could be conceptualized in one of two ways: PM2.5 might dampen BMI’s effect on birthweight, and/or higher pre-pregnancy BMI might attenuate PM2.5’s effects on birthweight. As for the contradictions of opposite adverse outcomes of PM2.5 on birthweight in different studies, we suppose that different metabolic status between different races and lifestyles, especially maternal overweight/obesity, can interfere the consistency in how PM2.5 affects birthweight. However, the detailed mechanisms remained unclear.
Our study has some strengths and several limitations. First, a large nationwide cohort study was conducted aiming to find a potential interaction between air pollution and metabolic status on birthweight. Another strength was to contradict the conventional opinion that pre-pregnancy BMI and air pollutions jointly had additive effect on birthweight (11), which shed light on future studies. In addition, to our current knowledge, this is the first study on a large Chinese cohort exploring the biological interaction between pre-pregnancy BMI and PM2.5 on birthweight, linking the metabolic status and environmental stressors in a combined manner. Furthermore, our study also provided a reasonable assumption that such an interaction may interfere fetal growth signal pathways. One of the limitations of our study was that we applied the estimated PM2.5 concentration instead of the ground monitored levels as the result of no ground monitoring station had been established until 2013. Another limitation of our study was the lacks of records of the actual residence of pregnant women. Although we ruled out individuals whose labor places were not coincident with their registered residence, mobility could still probably exist. We suggest that the public and researchers pay more attention to the potential risk of the complex relationship between air pollution and metabolic abnormalities on pregnancy outcomes, and hope to provide some enlightenment for future research.
Both maternal exposure to PM2.5 during pregnancy and pre-pregnancy BMI have positive associations with birth weight throughout pregnancy. More importantly, our findings indicate a negative interaction between pre-pregnancy BMI and PM2.5 on birthweight in the first, second trimesters and the whole pregnancy. Further experiments and researches are necessary to identify the biological mechanism of interaction.
The data analyzed in this study is subject to the following licenses/restrictions: Our research data were derived from the National Free Preconception Health Examination Project (NFPHEP). Requests to access these datasets should be directed to HP, PanHui@pumch.cn.
This study was reviewed and approved by Institutional Review Board of the National Research Institute for Family Planning, Beijing, China. The patients/participants provided their written informed consent to participate in this study. Written informed consent was obtained from the individual(s) for the publication of any potentially identifiable images or data included in this article.
HD, YS, YZ, SW, HZ, SC, and HPcontributed to the study concept and design. HD drafted the work. YZ has made substantial contributions to the acquisition, analysis, or interpretation of data for the work. YS conducted the statistical analysis. HD and YS analyzed the results. YS wrote the first draft. HD and SC provided editing and writing assistance for important intellectual content. HD, YS, and SC finalized the manuscript. All authors contributed to the article and approved the submitted version.
This study was supported by the CAMS Initiative for Innovative Medicine (grant no. 2016-I2M-1-008), Health Science Promotion Project of Beijing (grant no. 2018-TG-35), Beijing Municipal Natural Science Foundation (grant no. 7192153), and National Natural Science Foundation of China (grant no. 81673184).
The authors declare that they do not have any commercial or associative interest that represents a conflict of interest in connection with the work submitted.
All claims expressed in this article are solely those of the authors and do not necessarily represent those of their affiliated organizations, or those of the publisher, the editors and the reviewers. Any product that may be evaluated in this article, or claim that may be made by its manufacturer, is not guaranteed or endorsed by the publisher.
The Supplementary Material for this article can be found online at: https://www.frontiersin.org/articles/10.3389/fendo.2022.963827/full#supplementary-material
1. Knop MR, Geng TT, Gorny AW, Ding R, Li C, Ley SH, et al. Birth weight and risk of type 2 diabetes mellitus, cardiovascular disease, and hypertension in adults: A meta-analysis of 7 646 267 participants from 135 studies. J Am Heart Assoc (2018) 7(23):e008870. doi: 10.1161/JAHA.118.008870
2. Zhao Y, Wang SF, Mu M, Sheng J. Birth weight and overweight/obesity in adults: a meta-analysis. Eur J Pediatr (2012) 171(12):1737–46. doi: 10.1007/s00431-012-1701-0
3. Lurbe E, Ingelfinger J. Developmental and early life origins of cardiometabolic risk factors: Novel findings and implications. Hypertension (2021) 77(2):308–18. doi: 10.1161/HYPERTENSIONAHA.120.14592
4. Magnusson Å, Laivuori H, Loft A, Oldereid NB, Pinborg A, Petzold M, et al. The association between high birth weight and long-term outcomes-implications for assisted reproductive technologies: a systematic review and meta-analysis. Front Pediatr (2021) 9:675775. doi: 10.3389/fped.2021.675775
5. Qiao J, Wang Y, Li X, Jiang F, Zhang Y, Ma J, et al. A lancet commission on 70 years of women's reproductive, maternal, newborn, child, and adolescent health in China. Lancet (2021) 397(10293):2497–536. doi: 10.1016/S0140-6736(20)32708-2
6. Brant WO, Rajimwale A, Lovell MA, Travers SH, Furness PD 3rd, Sorensen M, et al. Gonadoblastoma and turner syndrome. J Urol (2006) 175(5):1858–60. doi: 10.1016/S0022-5347(05)00932-8
7. Vargas-Terrones M, Nagpal TS, Barakat R. Impact of exercise during pregnancy on gestational weight gain and birth weight: an overview. Braz J Phys Ther (2019) 23(2):164–9. doi: 10.1016/j.bjpt.2018.11.012
8. Catalano PM, Shankar K. Obesity and pregnancy: mechanisms of short term and long term adverse consequences for mother and child. BMJ (2017) 356:j1. doi: 10.1136/bmj.j1
9. Wang Z, Shi X, Pan C, Wang S. Spatial and temporal characteristics of environmental air quality and its relationship with seasonal climatic conditions in Eastern China during 2015-2018. Int J Environ Res Public Health (2021) 18(9):4524. doi: 10.3390/ijerph18094524
10. Liu Z, Szpiro AA, Workalemahu T, Young MT, Kaufman JD, Enquobahrie DA. Associations of perinatal exposure to PM(2.5) with gestational weight gain and offspring birth weight. Environ Res (2022) 204(Pt B):112087. doi: 10.1016/j.envres.2021.112087
11. Lakshmanan A, Chiu YH, Coull BA, Just AC, Maxwell SL, Schwartz J, et al. Associations between prenatal traffic-related air pollution exposure and birth weight: Modification by sex and maternal pre-pregnancy body mass index. Environ Res (2015) 137:268–77. doi: 10.1016/j.envres.2014.10.035
12. Chen LW, Soh SE, Tint MT, Loy SL, Yap F, Tan KH, et al. Combined analysis of gestational diabetes and maternal weight status from pre-pregnancy through post-delivery in future development of type 2 diabetes. Sci Rep (2021) 11(1):5021. doi: 10.1038/s41598-021-82789-x
13. Bellamy L, Casas JP, Hingorani AD, Williams D. Type 2 diabetes mellitus after gestational diabetes: a systematic review and meta-analysis. Lancet (2009) 373(9677):1773–9. doi: 10.1016/S0140-6736(09)60731-5
14. Bellamy L, Casas JP, Hingorani AD, Williams DJ. Pre-eclampsia and risk of cardiovascular disease and cancer in later life: systematic review and meta-analysis. BMJ (2007) 335(7627):974. doi: 10.1136/bmj.39335.385301.BE
15. Villamor E, Cnattingius S. Interpregnancy weight change and risk of adverse pregnancy outcomes: a population-based study. Lancet (2006) 368(9542):1164–70. doi: 10.1016/S0140-6736(06)69473-7
16. Cnattingius S, Villamor E, Johansson S, Edstedt Bonamy AK, Persson M, Wikström AK, et al. Maternal obesity and risk of preterm delivery. JAMA (2013) 309(22):2362–70. doi: 10.1001/jama.2013.6295
17. Luo Y, Zhang Y, Pan H, Chen S. Maternal secondhand smoke exposure enhances macrosomia risk among pregnant women exposed to PM(2.5): A new interaction of two air pollutants in a nationwide cohort. Front Public Health (2021) 9:735699. doi: 10.3389/fpubh.2021.735699
18. Chen S, Wang S, Li T, Zhu H, Liang S, Xu K, et al. Effect of PM2.5 on macrosomia in China: A nationwide prospective cohort study. Pediatr Obes (2020) 15(2):e12584. doi: 10.1111/ijpo.12584
19. Yuan L, Zhang Y, Gao Y, Tian Y. Maternal fine particulate matter (PM(2.5)) exposure and adverse birth outcomes: an updated systematic review based on cohort studies. Environ Sci pollut Res Int (2019) 26(14):13963–83. doi: 10.1007/s11356-019-04644-x
20. Liu Y, Xu J, Chen D, Sun P, Ma X. The association between air pollution and preterm birth and low birth weight in guangdong, China. BMC Public Health (2019) 19(1):3. doi: 10.1186/s12889-018-6307-7
21. Basu R, Harris M, Sie L, Malig B, Broadwin R, Green R. Effects of fine particulate matter and its constituents on low birth weight among full-term infants in California. Environ Res (2014) 128:42–51. doi: 10.1016/j.envres.2013.10.008
22. Bell ML, Belanger K, Ebisu K, Gent JF, Lee HJ, Koutrakis P, et al. Prenatal exposure to fine particulate matter and birth weight: variations by particulate constituents and sources. Epidemiology (2010) 21(6):884–91. doi: 10.1097/EDE.0b013e3181f2f405
23. Ebisu K, Berman JD, Bell ML. Exposure to coarse particulate matter during gestation and birth weight in the U.S. Environ Int (2016) 94:519–24. doi: 10.1016/j.envint.2016.06.011
24. Tapia VL, Vasquez BV, Vu B, Liu Y, Steenland K, Gonzales GF. Association between maternal exposure to particulate matter (PM(2.5)) and adverse pregnancy outcomes in Lima, Peru. J Expo Sci Environ Epidemiol (2020) 30(4):689–97. doi: 10.1038/s41370-020-0223-5
25. Rodríguez-Fernández A, Ramos-Castillo N, Ruiz-De la Fuente M, Parra-Flores J, Maury-Sintjago E. Association of prematurity and low birth weight with gestational exposure to PM2.5 and PM10 particulate matter in chileans newborns. Int J Environ Res Public Health (2022) 19(10):6133. doi: 10.3390/ijerph19106133
26. Zhao N, Qiu J, Ma S, Zhang Y, Lin X, Tang Z, et al. Effects of prenatal exposure to ambient air pollutant PM10 on ultrasound-measured fetal growth. Int J Epidemiol (2018) 47(4):1072–81. doi: 10.1093/ije/dyy019
27. Cheng Z, Luo L, Wang S, Wang Y, Sharma S, Shimadera H, et al. Status and characteristics of ambient PM2.5 pollution in global megacities. Environ Int (2016) 89-90:212–21. doi: 10.1016/j.envint.2016.02.003
28. Sun J, Mei H, Xie S, Wu L, Wang Y, Mei W, et al. The interactive effect of pre-pregnancy overweight and obesity and hypertensive disorders of pregnancy on the weight status in infancy. Sci Rep (2019) 9(1):15960. doi: 10.1038/s41598-019-52140-6
29. Chooi YC, Ding C, Magkos F. The epidemiology of obesity. Metabol: Clin Exp (2019) 92:6–10. doi: 10.1016/j.metabol.2018.09.005
30. Zhou Q, Zhang S, Wang Q, Shen H, Zhang Y, Tian W, et al. Association between preconception paternal smoking and birth defects in offspring: evidence from the database of the national free preconception health examination project in China. BJOG Int J Obstet Gynecol (2020) 127(11):1358–64. doi: 10.1111/1471-0528.16277
31. Buckton KE, Jacobs PA, Rae LA, Newton MS, Sanger R. An inherited X-autosome translocation in man. Ann Hum Genet (1971) 35(2):171–8. doi: 10.1111/j.1469-1809.1956.tb01390.x
32. Goswami R, Goswami D, Kabra M, Gupta N, Dubey S, Dadhwal V. Prevalence of the triple X syndrome in phenotypically normal women with premature ovarian failure and its association with autoimmune thyroid disorders. Fertil Steril (2003) 80(4):1052–4. doi: 10.1016/S0015-0282(03)01121-X
33. Xiao Q, Chang HH, Geng G, Liu Y. An ensemble machine-learning model to predict historical PM(2.5) concentrations in China from satellite data. Environ Sci Technol (2018) 52(22):13260–9. doi: 10.1021/acs.est.8b02917
34. Liu Y, Ding H, Chang ST, Lu R, Zhong H, Zhao N, et al. Exposure to air pollution and scarlet fever resurgence in China: a six-year surveillance study. Nat Commun (2020) 11(1):4229. doi: 10.1038/s41467-020-17987-8
35. Cogswell ME, Yip R. The influence of fetal and maternal factors on the distribution of birthweight. Semin Perinatol (1995) 19(3):222–40. doi: 10.1016/S0146-0005(05)80028-X
36. Austin PC. Balance diagnostics for comparing the distribution of baseline covariates between treatment groups in propensity-score matched samples. Stat Med (2009) 28(25):3083–107. doi: 10.1002/sim.3697
37. Nachman RM, Mao G, Zhang X, Hong X, Chen Z, Soria CS, et al. Intrauterine inflammation and maternal exposure to ambient PM2.5 during preconception and specific periods of pregnancy: the Boston birth cohort. Environ Health Perspect (2016) 124(10):1608–15. doi: 10.1289/EHP243
38. Yu Z, Han S, Zhu J, Sun X, Ji C, Guo X. Pre-pregnancy body mass index in relation to infant birth weight and offspring overweight/obesity: a systematic review and meta-analysis. PloS One (2013) 8(4):e61627. doi: 10.1371/journal.pone.0061627
39. Stamnes Koepp UM, Frost Andersen L, Dahl-Joergensen K, Stigum H, Nass O, Nystad W. Maternal pre-pregnant body mass index, maternal weight change and offspring birthweight. Acta Obstet Gynecol Scand (2012) 91(2):243–9. doi: 10.1111/j.1600-0412.2011.01321.x
40. Chiarello DI, Abad C, Rojas D, Toledo F, Vázquez CM, Mate A, et al. Oxidative stress: Normal pregnancy versus preeclampsia. Biochim Biophys Acta Mol Basis Dis (2020) 1866(2):165354. doi: 10.1016/j.bbadis.2018.12.005
41. Wang C, Plusquin M, Ghantous A, Herceg Z, Alfano R, Cox B, et al. DNA Methylation of insulin-like growth factor 2 and H19 cluster in cord blood and prenatal air pollution exposure to fine particulate matter. Environ Health Global Access Sci Source (2020) 19(1):129. doi: 10.1186/s12940-020-00677-9
42. Lassance L, Haghiac M, Leahy P, Basu S, Minium J, Zhou J, et al. Identification of early transcriptome signatures in placenta exposed to insulin and obesity. Am J Obstet Gynecol (2015) 212(5):647.e1–11. doi: 10.1016/j.ajog.2015.02.026
43. Jansson N, Rosario FJ, Gaccioli F, Lager S, Jones HN, Roos S, et al. Activation of placental mTOR signaling and amino acid transporters in obese women giving birth to large babies. J Clin Endocrinol Metab (2013) 98(1):105–13. doi: 10.1210/jc.2012-2667
44. Westermeier F, Sáez PJ, Villalobos-Labra R, Sobrevia L, Farías-Jofré M. Programming of fetal insulin resistance in pregnancies with maternal obesity by ER stress and inflammation. BioMed Res Int (2014) 2014:917672. doi: 10.1155/2014/917672
45. Jurkiewicz D, Kugaudo M, Skórka A, Śmigiel R, Smyk M, Ciara E, et al. A novel IGF2/H19 domain triplication in the 11p15.5 imprinting region causing either beckwith-wiedemann or silver-Russell syndrome in a single family. Am J Med Genet Part A (2017) 173(1):72–8. doi: 10.1002/ajmg.a.37964
46. van Beijnum JR, Pieters W, Nowak-Sliwinska P, Griffioen AW. Insulin-like growth factor axis targeting in cancer and tumour angiogenesis - the missing link. Biol Rev Cambridge Philos Soc (2017) 92(3):1755–68. doi: 10.1111/brv.12306
47. Cui D, Yang W, Shao P, Li J, Wang P, Leng J, et al. Interactions between prepregnancy overweight and passive smoking for macrosomia and Large for gestational age in Chinese pregnant women. Obes Facts (2021) 14(5):520–30. doi: 10.1159/000517846
48. La Merrill M, Stein CR, Landrigan P, Engel SM, Savitz DA. Prepregnancy body mass index, smoking during pregnancy, and infant birth weight. Ann Epidemiol (2011) 21(6):413–20. doi: 10.1016/j.annepidem.2010.11.012
Keywords: air pollution, birthweight, negative interaction, metabolic status, obesity, fine particulate matter (PM2.5), pre-pregnancy body mass index
Citation: Du H, Sun Y, Zhang Y, Wang S, Zhu H, Chen S and Pan H (2022) Interaction of PM2.5 and pre-pregnancy body mass index on birth weight: A nationwide prospective cohort study. Front. Endocrinol. 13:963827. doi: 10.3389/fendo.2022.963827
Received: 08 June 2022; Accepted: 05 July 2022;
Published: 26 July 2022.
Edited by:
Qinghua Sun, Zhejiang Chinese Medical University, ChinaReviewed by:
Renjie Chen, Fudan University, ChinaCopyright © 2022 Du, Sun, Zhang, Wang, Zhu, Chen and Pan. This is an open-access article distributed under the terms of the Creative Commons Attribution License (CC BY). The use, distribution or reproduction in other forums is permitted, provided the original author(s) and the copyright owner(s) are credited and that the original publication in this journal is cited, in accordance with accepted academic practice. No use, distribution or reproduction is permitted which does not comply with these terms.
*Correspondence: Shi Chen, Y3MwMDgzQDEyNi5jb20=; Hui Pan, cGFuaHVpMjAxMTExMTFAMTYzLmNvbQ==
†These authors have contributed equally to this work and share first authorship
Disclaimer: All claims expressed in this article are solely those of the authors and do not necessarily represent those of their affiliated organizations, or those of the publisher, the editors and the reviewers. Any product that may be evaluated in this article or claim that may be made by its manufacturer is not guaranteed or endorsed by the publisher.
Research integrity at Frontiers
Learn more about the work of our research integrity team to safeguard the quality of each article we publish.