- 1Department of Ophthalmology, Peking University People’s Hospital Eye diseases, and Optometry Institute, Beijing, China
- 2Beijing Key Laboratory of Diagnosis and Therapy of Retinal and Choroid Diseases, Peking University People’s Hospital, Beijing, China
- 3College of Optometry, Peking University Health Science Center, Beijing, China
- 4Department of Ophthalmology, The Second Affiliated Hospital of Anhui Medical University, Hefei, China
Background: Pterygium is an ocular surface disease that can cause visual impairment if it progressively invades the cornea. Although many pieces of research showed ultraviolet radiation is a trigger of pterygium pathological progress, the underlying mechanism in pterygium remains indistinct.
Methods: In this study, we used microarray to evaluate the changes of transcripts between primary pterygium and adjacent normal conjunctiva samples in China. Then, we performed Gene Ontology (GO) and Kyoto Encyclopedia of Genes and Genomes (KEGG) functional enrichment analyses. Moreover, we constructed protein-protein interaction (PPI) and miRNA-mRNA regulatory networks to predict possible regulatory relationships. We next performed gene set enrichment analysis (GSEA) to explore the similarities and differences of transcripts between Asian studies from the Gene Expression Omnibus database. Furthermore, we took the intersection of differentially expressed genes (DEGs) with other data and identified hub genes of the development of pterygium. Finally, we utilized real-time quantitative PCR to verify the expression levels of candidate genes.
Results: A total of 49 DEGs were identified. The enrichment analyses of DEGs showed that pathways such as the Wnt-signaling pathway and metabolism-related pathways were upregulated, while pathways such as hormone-related and transcription factor-associated pathways were downregulated. The PPI and miRNA-mRNA regulatory networks provide ideas for future research directions. The GSEA of selecting Asian data revealed that epithelial-mesenchymal transition and myogenesis existed in the pathology of pterygium in the Asian group. Furthermore, five gene sets (interferon-gamma response, Wnt beta-catenin signaling, oxidative phosphorylation, DNA repair, and MYC targets v2) were found only in our Chinese datasets. After taking an intersection between selecting datasets, we identified two upregulated (SPP1 and MYH11) and five downregulated (ATF3, FOS, EGR1, FOSB, and NR4A2) hub genes. We finally chose night genes to verify their expression levels, including the other two genes (SFRP2 and SFRP4) involved in Wnt signaling; Their expression levels were significantly different between pterygium and conjunctiva.
Conclusions: We consider hormone-related, metabolic, and Wnt signaling pathways may be important in the pathology of pterygium development. Nine candidate genes we identified deserve further study and can be potential therapeutic targets.
1 Introduction
Pterygium is a benign ocular surface disease that typically appears as a fibrovascular conjunctiva thickening from the nasal side and may progressively extend onto the cornea, causing visual impairment (1, 2). The incidence of pterygium was 1.4% in the Asian population and 2.1% in the Chinese (3). Ultraviolet (UV) radiation, male gender, older age, ethnicity, outdoor occupation, and air pollution were associated with the occurrence and development of pterygium (3–5). Pterygium excision with conjunctival autograft and adjuvant therapy is the conventional treatment (6). Using fibrin glue in place of sutures for attaching conjunctival autograft during the surgery could further reduce the recurrence rate (0-4.5%) and surgical duration and help recover the cornea (7–9). Although many efforts have made progress, more surgical and non-surgical approaches are needed to be explored to decrease the recurrence further.
Currently, the underlying mechanism in pterygium remains indistinct. UV radiation is a well-recognized major cause of pterygium, and many research studies believe it triggers oxidative stress leading to DNA damage and subsequently stimulates multiple pathogenic factors (such as tumor suppressor P53, growth factors, pro-inflammatory cytokines, and extracellular matrix modulation) to induce a hyperproliferative state (10–15). On the other hand, hereditary factors and viruses (e.g., human papillomavirus) are also reported to contribute to the disease, so pterygium is considered an outcome of a combination of genetic and environmental factors (1, 15). Moreover, pterygium is regarded as a limbal stem cell disorder with precancerous features (16). In short, despite many studies on pterygium, there are lots of unsolved puzzles that need to be unraveled.
Many studies have utilized transcriptome technologies to identify changes in transcripts of pterygium in recent years (17–23). They have greatly advanced the research on pterygium and identified several groups of abnormally expressed genes in pterygium, such as keratins (KRT3, KRT4, KRT6B, KRT13, KRT14, KRT16, and KRT24); S100 calcium-binding proteins (S100A8, S100A9, S100A11, and S100P); collagens (COL1A1, COL8A1, and COL10A1). Furthermore, other studies have reported the involvement of various matrix metalloproteinases in the progression of pterygium (MMP1, MMP2, MMP3, MMP9, and MMP13) (24–26). On the other hand, it has been reported that the Chinese have a higher incidence of the disease than the whole Asian population (3), so the transcriptomic information needs to be more abundant. Moreover, identifying the similarities and differences of transcripts between Asian studies is necessary to reveal the underlying mechanisms of pterygium.
In this study, we used microarray to evaluate the changes of transcripts between primary pterygium samples and adjacent normal conjunctiva samples in China and perform multiple bioinformatic analyses. Next, we took the intersection of differentially expressed genes with other data in Asian countries and identified hub genes. Finally, we verified the expression levels of candidate genes from microarray data. Our study hopes to provide more evidence of the progression of pterygium and find potential treatment targets.
2 Material and methods
2.1 Specimens preparation
This study approval was provided by the Ethics Committee of the second affiliated hospital of Anhui Medical University in accordance with the Helsinki Declaration of 1975. Sixteen nasal primary pterygium samples and fourteen adjacent normal conjunctiva samples were obtained from 16 participants (aged 47-70 years, five males and 11 females) through pterygium excision surgery. All the procedures were performed by an experienced ophthalmologist in the second affiliated hospital, Anhui Medical University. All of the participants in this study signed the informed consent before they were enrolled in the study. After pterygium excision, the whole part of the tissues was immediately placed in RNALater™ RNA Stabilization Reagent for Animal Tissue (Beyotime, China, R0118) and infiltrated overnight at four degrees, then all of the tissues were stored at a −80 °C refrigerator until RNA extraction.
2.2 RNA isolation
The total RNA of the tissues was isolated using TRIzol™ Reagent (Invitrogen, USA, 15596026) according to the manufacturer’s protocol. For microarray analysis, two pairs of pterygium and adjacent normal conjunctiva samples were used. The other tissues were used in real-time quantitative polymerase chain reaction (qPCR). The quality control and quantification of RNA were performed by electrophoresis on 1% agarose gels and NanoDrop 2000 (Thermo Fisher Scientific, USA).
2.3 Microarray analysis
After the quality test of RNA, gene expression profiling was conducted by Shanghai Baygene Biotechnology Co.Ltd (Shanghai, China) using GeneChip® Human Transcriptome Array 2.0 (HTA 2.0). cDNA was synthesized, amplified, fragmented, and labeled for hybridization using the GeneChip® WT PLUS Reagent Kit (Affymetrix, USA, 902280) from 2 ugs isolated RNA following the manufacturer’s protocols. Then, GeneChip® Expression Wash, Stain, and Scan were performed following protocol from GeneChip® Hybridization Wash and Stain Kit (Affymetrix, USA, 900720). Probe cell intensity data (CEL) from microarrays are analyzed and normalized by Signal Space Transformation Robust Multichip Analysis (SST-RMA) algorithm in the Affymetrix® Expression Console™ software (v1.4). The normalized data were then subjected to R package limma (v3.42.2) to identify differentially expressed genes (DEGs) (27). Genes with |log2FC| >1 and p-value < 0.05 were identified as DEGs. Volcano plot visualization was exerted by ggplot2 (v3.3.0). Next, the DEGs were submitted to execute the Gene Ontology(GO) and the Kyoto Encyclopedia of Genes and Genomes(KEGG) functional enrichment analyses utilizing the R package ClusterProfiler (v3.14.3) (28). For Protein-protein interaction (PPI) network construction, we used the STRING database (https://string-db.org/cgi/input.pl) to recognize the possible connections between DEGs, the data exported from the STRING database was visualized by Cytoscape (v3.8.2), and the MCODE (v2.0.0) plugin of Cytoscape was applied to identify a key module of PPI network (29).
2.4 Data collection
For further bioinformatic analyses, we collected miRNA and microarray data from PubMed and the Gene Expression Omnibus database (GEO, www.ncbi.nlm.nih.gov/geo) based on our selection criteria. As for miRNA selection, we used “Pterygium” and “miRNA” as keywords to search on Pubmed and screen research from Asia. Next, we included 14 miRNAs that were validated by qPCR or could be found in at least two microarray data. The information on miRNA enrolled in our study is listed in Table 1 (30–37). The selection of microarray datasets for this study was based on screening the GEO database, and datasets from Asia were selected. The details of these microarray datasets are shown in Table 2.
2.5 The miRNA-mRNA regulatory network construction
All enrolled miRNAs were submitted to ENCORI (the encyclopedia of RNA Interactomes, https://starbase.sysu.edu.cn/) to predict and acquire their targeted mRNAs (38). The ENCORI could also connect to seven other target mRNA-predicted programs (microT, miRanda, miRmap, PITA, RNA22, PicTar, and TargetScan); the mRNAs we selected were overlapped in at least four predicted programs. The miRNAs usually perform as negative regulators to affect the expression of the target mRNAs, upregulated miRNA led to downregulated target mRNA and vice versa. Considering such a regulatory pattern, we took the intersection of the acquired mRNAs with our microarray data based on the expression change direction. The visualization of the miRNA-mRNA regulatory network was carried out by TBtools (v1.0986988) (39).
2.6 Gene set enrichment analysis
The gene set enrichment analysis (GSEA) based on Hallmark gene sets in the molecular signatures database (v7.5.1) was performed by GSEA software (v4.2.3). The results of GSEA were subjected to TBtools to visualize as a heat map, and data was clustered using Euclidean distances with complete hierarchical clustering.
2.7 Hub genes identification and enrichment analysis
To identify hub genes, we processed microarray datasets GSE83627, GSE51995, and GSE2513 by limma (v3.42.2). Besides, the microarray dataset GSE151872 only has one pterygium sample and is not applicable for limma’s protocol, so we discarded this dataset to recognize hub genes. We took the intersection of DEGs from our microarray data and the above datasets, then submitted the results to the STRING database to perform further enrichment analysis. The visualizations were conducted by TBtools, Cytoscape, and ggplot2.
2.8 Real-time quantitative polymerase chain reaction
cDNA was synthesized from a total of 1 µg RNA with the ReverTra Ace® qPCR RT Master Mix with gDNA Remover (Toyobo Co., Ltd., Japan, Code No.FSQ-301). Real-time quantitative polymerase chain reactions (qPCR) were carried out using SYBR® Green Real-time PCR Master Mix (Toyobo Co., Ltd., Japan, Code No. QPK-201) with Roche LightCycler 480 (Roche Diagnostics Lid. Switzerland). Evaluating relative mRNA expression by using GAPDH as endogenous control via the 2−△CT methods. The experiments were repeated using three technical replicates. Table 3 lists all primers.
2.9 Statistical analysis
Analyses were performed and visualized by GraphPad Prism 8.3.0. After failing to pass the Shapiro-Wilk normality test, then comparisons of data were made using the Mann–Whitney nonparametric test. A p-value of <0.05 was considered a significant difference.
3 Results
3.1 Results of microarray data analysis
3.1.1 The differential gene expression analysis
The differential gene expression analysis identified a total of 49 DEGs (|log2FC| >1 and p-value < 0.05) on primary pterygium samples vs. adjacent normal conjunctiva samples. Among them, 21 were upregulated, and 28 were downregulated; all the DEGs and details are listed in Supplementary Table 1. The volcano plot (shown in Figure 1A) of the microarray data indicated the distribution of the DEGs. The upregulated DEGs were shown in yellow and downregulated were shown in green.
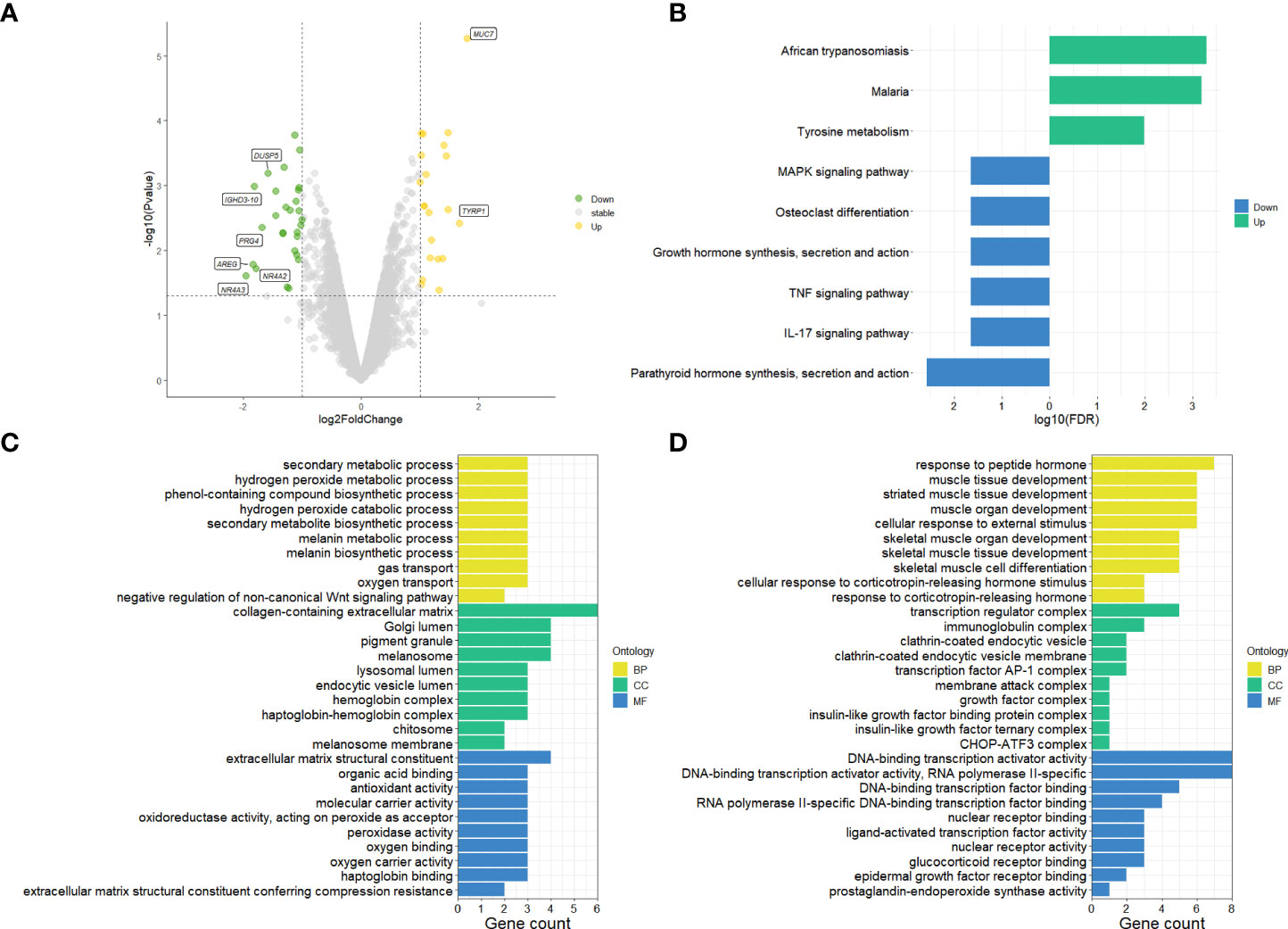
Figure 1 Results of microarray data analysis. (A) Volcano plot of microarray data, upregulated genes fill with yellow while downregulated genes fill with green. Grey dots mean genes are stable. The dotted horizontal lines indicate a p.value of 0.05, and the vertical lines indicate a log2FC of 1. Genes (|log2FC| > 1.5) labeled with their gene symbol are shown in the Figure. (B) KEGG analysis of DEGs of pterygium. The green color indicates upregulation, and blue indicates downregulation. (C) Top 30 upregulated GO terms for DEGs in different classifications. (D) Top 30 downregulated GO terms for DEGs in different classifications. The selection criteria of significant pathways or GO terms was FDR < 0.05. log2FC, log2foldchange; GO, Gene Ontology; KEGG, Kyoto Encyclopedia of Genes and Genomes (http://www.genome.jp/kegg/); FDR, false discovery rate. BP, biological process; CC, cellular component; MF, molecular function.
3.1.2 KEGG and GO pathway enrichment analysis
To get more bioinformatic signatures of the pterygium, we next performed enrichment analyses of the DEGs. Figure 1B presented the results of the KEGG pathway enrichment analysis. The upregulated pathways were related to infection and metabolism, and the downregulated were associated with inflammation, hormone, and cell differentiation. As for GO analysis, a total number of 195 GO terms of upregulated DEGs and 237 GO terms of downregulated DEGs were enriched (Supplementary Table 2 listed all the GO terms), the top 30 of them in different classifications (cellular component, molecular function, and biological process) were shown in Figures 1C, D for the up- and downregulated GO terms respectively. Negative regulation of the Wnt-signaling pathway, peroxide and oxygen related, and metabolism-related pathways could be found in upregulated GO terms (Figure 1C). Additionally, hormone and skeletal muscle related and transcription factor associated pathways were enriched in downregulated GO terms (Figure 1D).
3.1.3 PPI network construction
For protein-coding genes of the DEGs, we next put them into the STRING for a PPI network (Figure 2A). There were 32 nodes and 188 interacted edges in the PPI network. Then, we used MCODE to identify a key module for the whole network (Figure 2B); this cluster scoring 8.444 had ten nodes and 76 edges and might play a role in the pterygium.
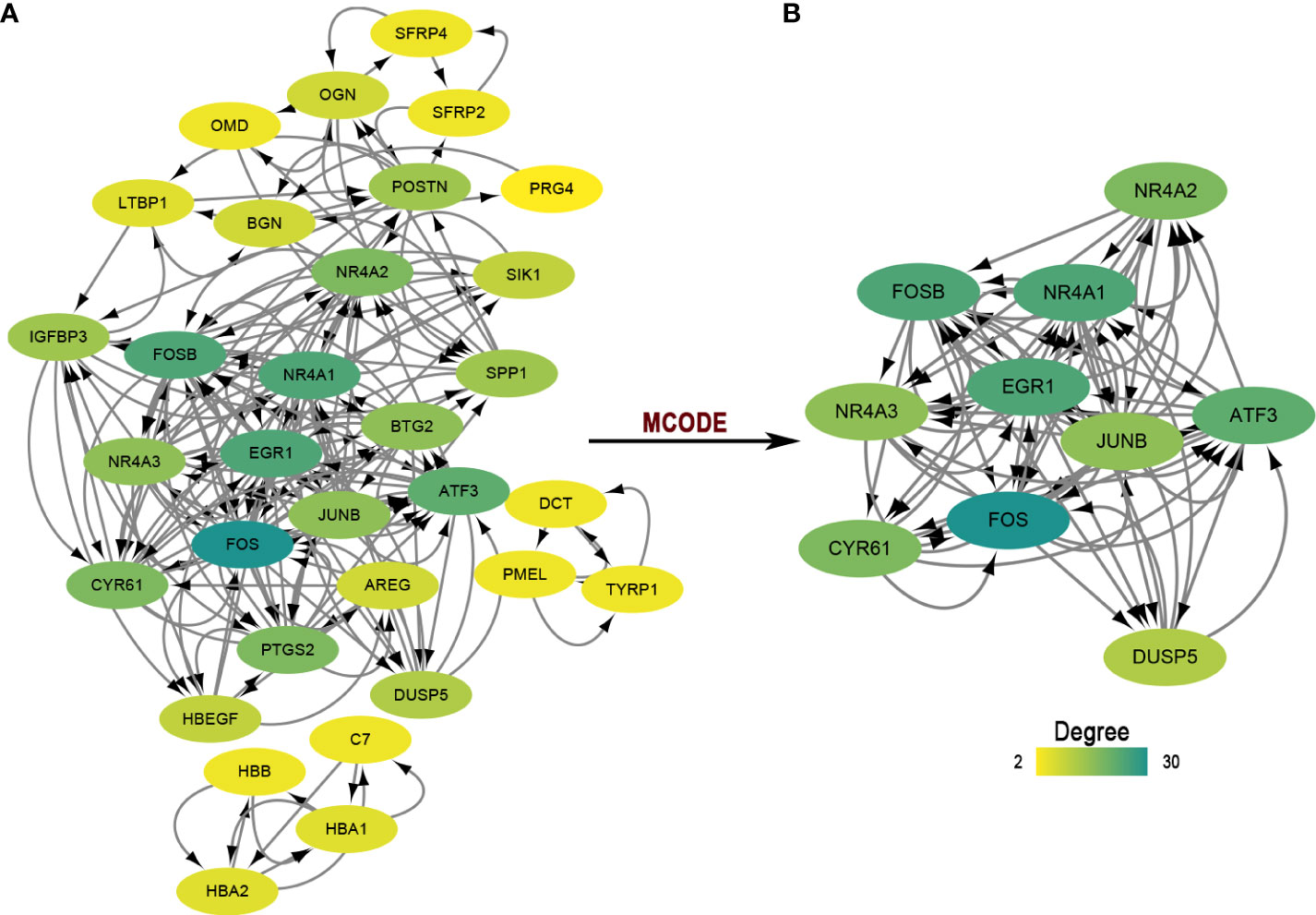
Figure 2 The PPI network and module analysis. (A) The entire PPI network of DEGs. Total of 32 nodes and 188 interacted edges in the PPI network. (B) A significant module of the PPI network. PPI, protein-protein interaction.
3.2 The miRNA-mRNA regulatory network construction
We searched on PubMed using the keywords “Pterygium” AND “miRNA” for all years, then selected the miRNAs in pterygium from Asian research based on the selection criteria. Table 1 summarizes the information on the selected miRNAs. Next, we put these miRNAs into ENCORI to predict the targeted mRNA and merged the results with our microarray data according to the screening criteria. Finally, a total of eight miRNAs and twelve mRNAs constructed 17 regulatory pairs. The miRNA-mRNA regulatory network was shown in Figure 3, and there existed a one-to-many regulatory relationship.
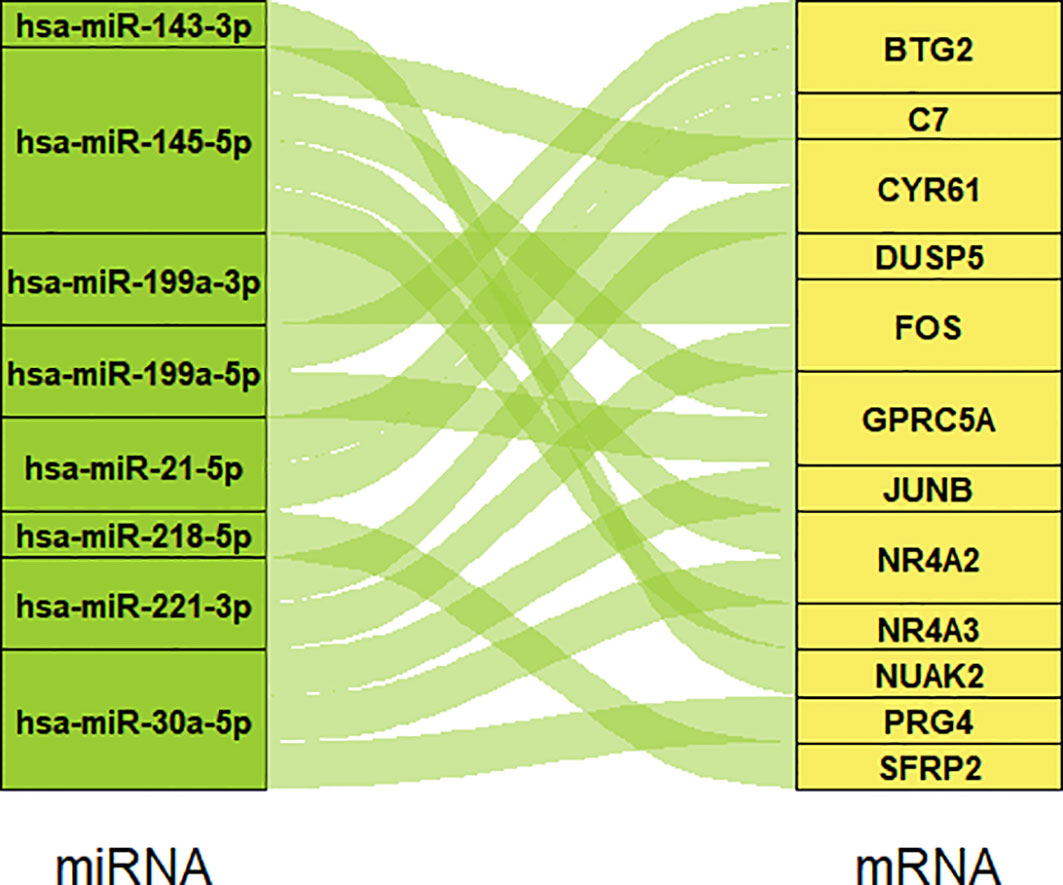
Figure 3 The miRNA-mRNA regulatory network. Eight miRNAs and 12 mRNAs construct 17 regulatory pairs.
3.3 To compare the similarities and differences between Asian studies by gene set enrichment analysis
We found four datasets from Gene Expression Omnibus (GEO) in Asia (Singapore and Japan) to perform the following analysis (Table 2). We subjected the whole expression matrixes of our data and four datasets from GEO to GSEA software (v4.2.3), selecting Hallmark gene sets in the molecular signatures database (v7.5.1) to find similar and different biological processes of pterygium in Asia. As shown in Figure 4, only two gene sets were enriched in all datasets (epithelial-mesenchymal transition and myogenesis). Three gene sets were in the results of the other four datasets but not ours (inflammatory response, angiogenesis, and coagulation). Moreover, five gene sets were found only in our microarray data (interferon-gamma response, Wnt beta-catenin signaling, oxidative phosphorylation, DNA repair, and MYC targets v2). Furthermore, there were several gene sets in our data that were enriched in the other three data. Our microarray data was indicated as a separate clustering in cluster analysis based on Euclidean distance. The complete results of GSEA are demonstrated in Supplementary Table 3. The bigger the NES, the stronger the effect. NES > 0 meant upregulated while NES < 0 represented downregulated.
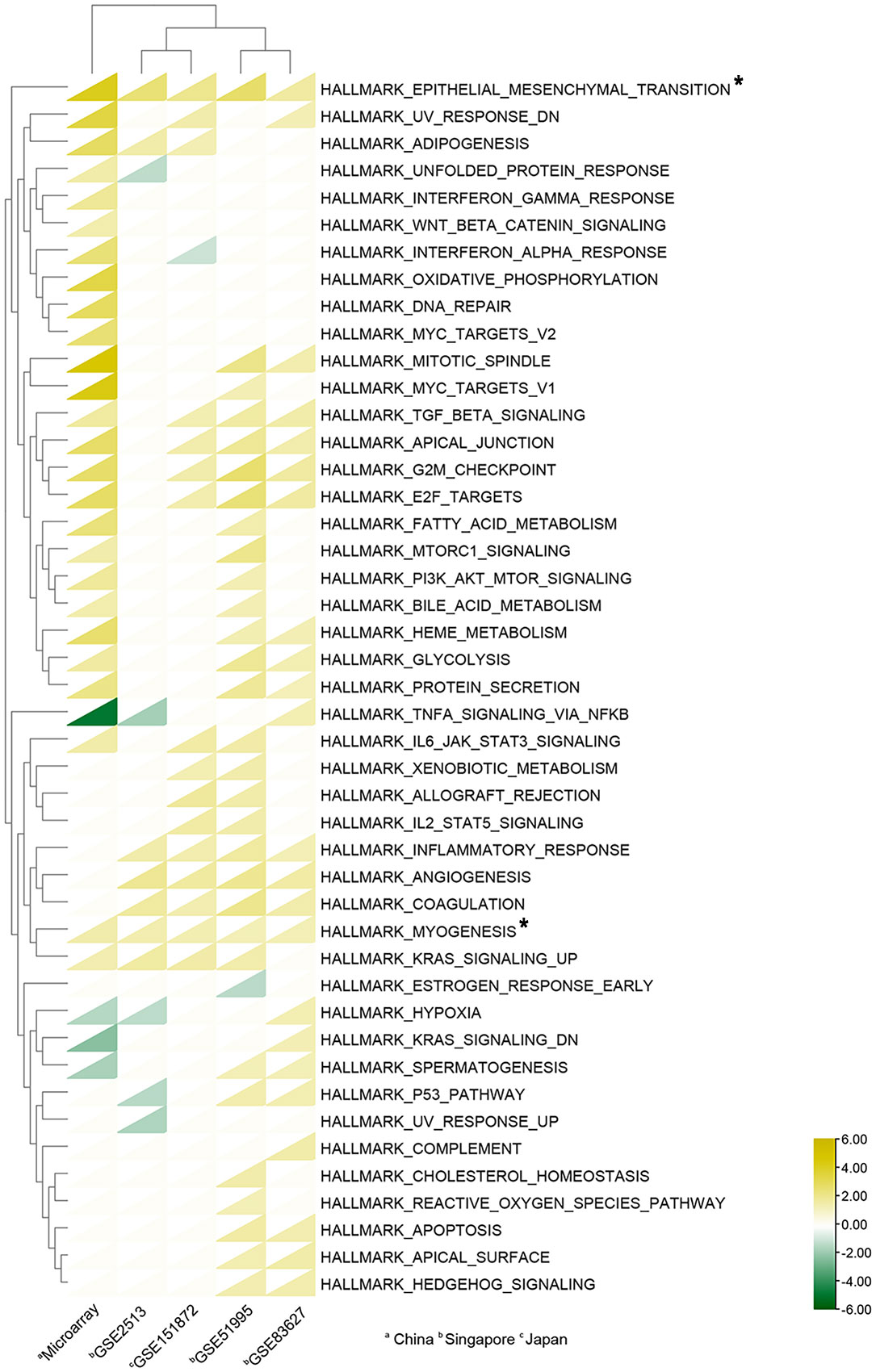
Figure 4 Heat map of Gene sets enriched in GSEA analysis. A total of 45 gene sets were enriched. The triangles in yellow indicate upregulated gene sets (NES > 0), while the triangles in green indicate downregulated gene sets (NES < 0); Triangles in white show no enrichment. Asterisks mean gene sets enriched in all datasets. The cluster analyses were based on Euclidean distance.
3.4 Identifying the hub genes by taking intersection with other microarray data
To identify the hub genes in the development of pterygium, we took an intersection between our data and other data from GEO datasets (Figures 5A, B). There were only two upregulated and five downregulated genes at the intersection of four microarray datasets (Table 4). All of them were protein-coding genes. Strikingly, all five downregulated genes were in the key cluster that MCODE identified (Figure 2B), so we next put these hub genes into STRING again and performed network enrichment analysis. Two upregulated genes showed no interaction, while five downregulated genes displayed a strong connection (Figure 5C). Moreover, the network enrichment analysis (Figures 5D, E) of these five genes showed similarity with enrichment analysis of downregulated DEGs (e.g., Parathyroid hormone synthesis, secretion, and action; IL-17 signaling pathway; Osteoclast differentiation). Some pathways associated with the organic and abiotic substance or stimulus resulted from network enrichment analysis but not from the whole DEGs’.
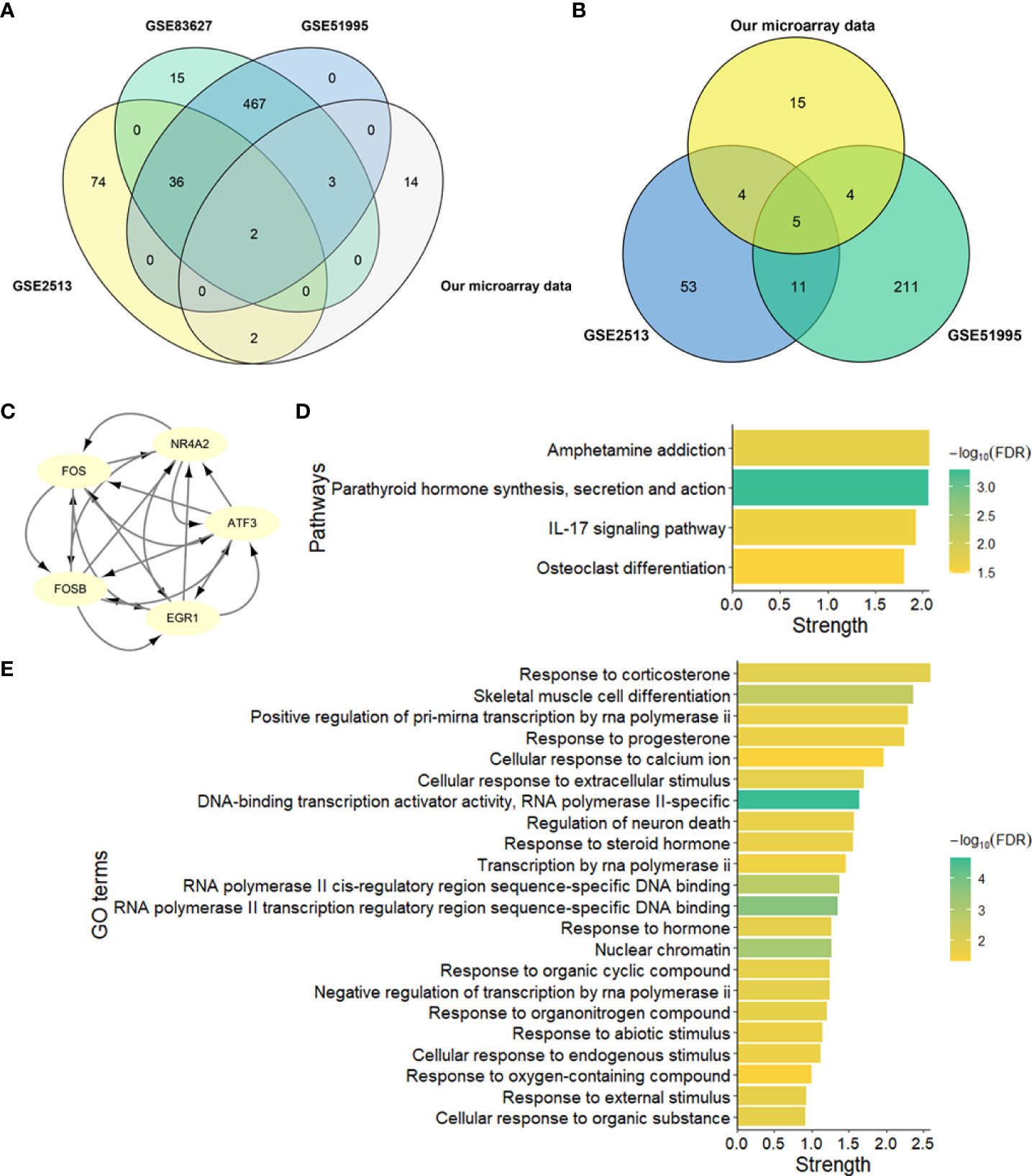
Figure 5 Identification and network enrichment analysis of hub genes. (A) Venn plot of upregulated genes of four microarray data. (B) Venn plot of downregulated genes of four microarray data. (C) PPI network of five downregulated hub genes. There are five nodes and ten edges. (D) KEGG pathway network enrichment analysis of five downregulated hub genes. (E) GO network enrichment analysis of five downregulated hub genes. Strength describes how significant the enrichment effect is.
3.5 Validation of the mRNA expression levels of candidate genes by qPCR
Based on the previous analysis, we chose seven hub genes to verify their expression levels. We enrolled SFRP2 and SFRP4 in further analysis because they were DEGs involved in Wnt signaling. As illustrated in Figures 6A–I, the mRNA expression levels of candidate genes (SFRP2, SFRP4, SPP1, ATF3, FOS, EGR1, FOSB, NR4A2, and MYH11) were significantly different in primary pterygium samples compared to conjunctiva samples. Additionally, the correlation analysis of log2 foldchange between microarray and the qPCR quantitative data demonstrated a high correlation (R2 = 0.8934, Figure 6J) which presented the accuracy of our microarray data.
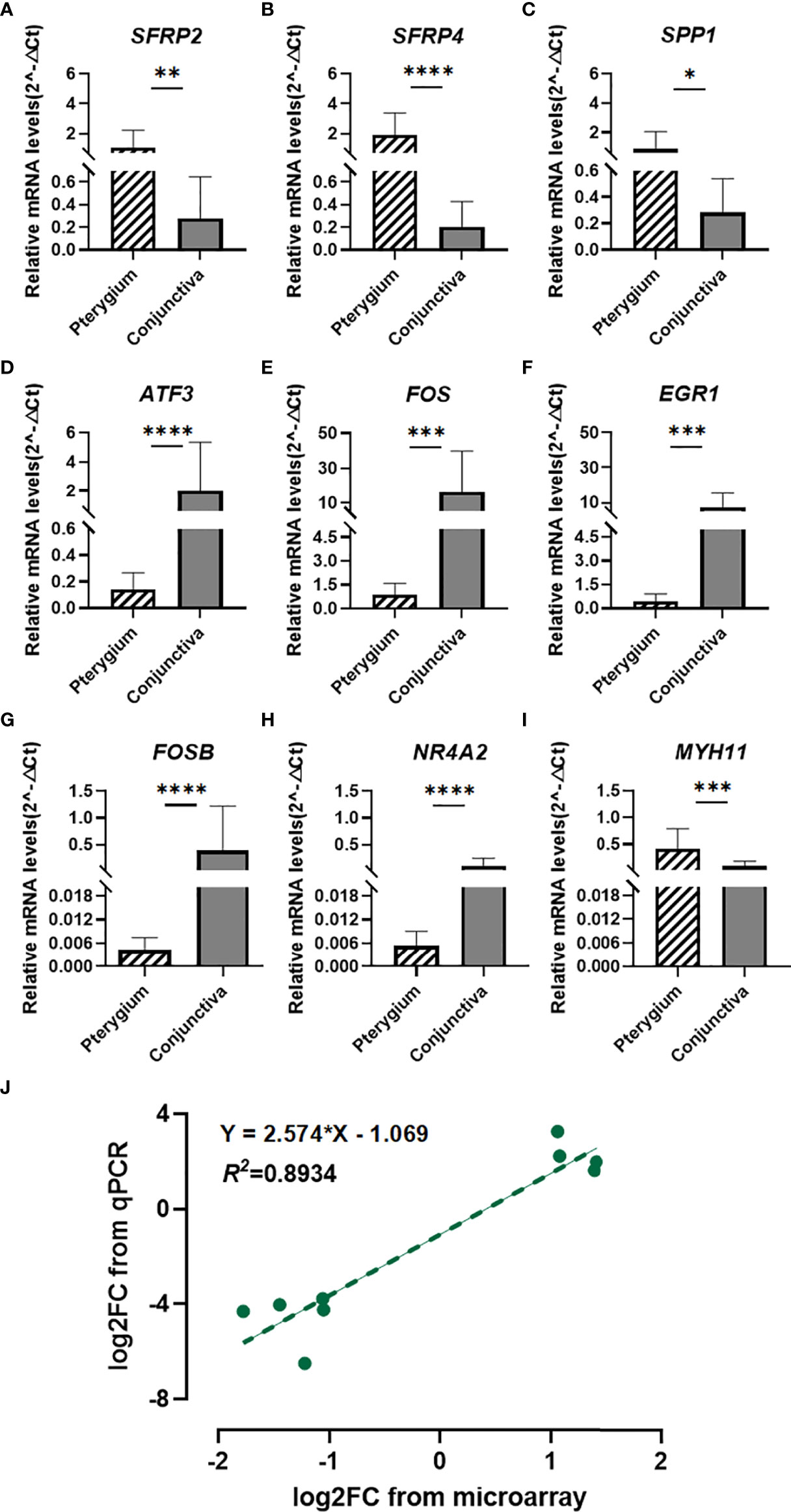
Figure 6 Quantitative real-time PCR verification and Correlation analysis. (A–I) Quantitative real-time PCR validation for SFRP2, SFRP4, SPP1, ATF3, FOS, EGR1, FOSB, NR4A2, and MYH11. n = 14 for primary pterygium samples and n = 12 for normal conjunctiva samples. *p < 0.05, **p < 0.01, ***p < 0.001, ****p < 0.0001. (J) Correlation analysis between microarray and qPCR data of candidate genes. Linear regression model (green dotted line): y = 2.574x - 1.069, R2 = 0.8934.
4 Discussion
Pterygium is a common fibrovascular degeneration of conjunctiva, and ultraviolet radiation is the main cause. The best way of treating pterygium is to perform surgery with some adjuvant treatments (6, 40). Currently, the concrete mechanisms of onset and progression of pterygium still remain obscure.
To explore the underlying mechanism of pterygium, we used microarray to compare changes in transcripts between primary pterygium samples and conjunctiva samples. 49 DEGs were identified in our study, and we next conducted multiple enrichment analyses to find the potential pathways of the disease. In the results, numbers of the metabolic and biosynthetic processes were upregulated (seven of the top ten GO BP terms), and these processes represent that the cells in pterygium were actively reacting to the compounds (e.g., hydrogen peroxide) involved in them. As part of reactive oxygen species, hydrogen peroxide is responsible for oxidative stress, while oxidative stress is considered a pathogenic mechanism of pterygium (12, 41). Additionally, metabolites from healthy and diseased cells can be directly released to tear, making the difference in tear proteome between pterygium and other diseases (42). The Wnt-signaling pathway, which can control stem cell biology and growth, is also upregulated; the changes in gene expression associated with Wnt-signaling in pterygium have been reported; however, how this pathway affects the development of pterygium needs to be studied in depth (43, 44). Downregulated pathways also provide us with pieces of evidence of disease progression. Hormone-related pathways are significantly downregulated; some research reported that hormone-related growth factors and receptors are implicated in pterygium (45, 46). Even though some hormones can affect corneal morphology, physiology, and metabolism, there is few of evidence indicating that hormones directly participate in the development of pterygium (47, 48). On the other hand, long-term UVA radiation to the eyes can affect the level of corticotropin-releasing hormone in the brain (49). So, we infer that the changes in hormone-related pathways are the response to UVA radiation. Furthermore, growth factors are also downregulated. Much evidence suggests that multiple growth factors are involved in pterygium, and their expression pattern may lead to a wide variation in the growth of pterygium (15). Other downregulated pathways (e.g., tumor necrosis factor (TNF) signaling, mitogen-activated protein kinase (MAPK) signaling, and transcription factor) have strong relationships with UV radiation or pterygium (50–52). The above results, to some extent, confirmed the reliability of our microarray data.
Nowadays, the PPI network and miRNA-mRNA regulatory network have been constructed in many studies (53–55). Since our new microarray data and new miRNA data from PubMed became available, we constructed new networks based on recent data. Our PPI network and miRNA-mRNA regulatory network hope to provide a new basis for further study of pterygium. Moreover, hsa-miR-199a-3p targets DUSP5 in our network have been confirmed in pterygium (33).
Besides performing analyses of our microarray data, we also compare our data with other Asian datasets from GEO. The hallmark gene sets include 50 gene sets, while 45 gene sets were enriched through GSEA. As displayed in Figure 4, epithelial-mesenchymal transition (EMT) and myogenesis are found upregulated in all datasets. EMT, playing an essential role in wound healing and tissue remodeling, is a biological process that induces the transition of polarized and immotile epithelial cells into motile mesenchymal fibroblast-like cells (56). EMT and myogenesis in our results represent epithelial cells converted to myofibroblasts, serving as a key feature of pathological tissue repair and playing an essential role in pterygium progression (33, 57). As for the differences between our data and other Asian data, we found five significant pathways only upregulated in our results: interferon-gamma response, Wnt beta-catenin signaling, oxidative phosphorylation, DNA repair, and MYC targets v2. As outlined previously, oxidative phosphorylation and DNA repair are related to oxidative stress and DNA damage which are downstream of UV radiation. Interferon-gamma response belongs to immune responses which have been associated with pterygium (58). MYC, as a proto-oncogene is also involved in the disease (59). The result of Wnt beta-catenin signaling is consistent with our previous GO analysis. On the other hand, three pathways (inflammatory response, angiogenesis, and coagulation) correlating with pterygium are not present in our data but exist in the other four datasets (15). Interestingly, three datasets show upregulation of the pathways named UV response downregulated. This result probably shows that the response to UV radiation is different between pterygium cells and healthy cells, while pterygium epithelium possibly has resistance to UV-induced apoptosis (14). In conclusion, the results of GSEA have offered a shred of potential evidence for the cause of differences in pterygium incidence in Asia. The pathways that are unique and absent in our datasets should be worth attention.
To further investigate the common mechanism of pathogenesis in pterygium, we took the intersection between our data and other GEO datasets we selected. A total of seven genes were identified as hub genes. The two upregulated genes show no interactions, and the network enrichment analysis shows no results. We later subjected them to ClusterProfiler, and the results were similar to previous GO terms that they involved (data not shown). SPP1 encodes osteopontin playing important roles in wound healing. Osteopontin is suggested to affect metalloproteinase (MMP) secretion and influence fibroblast proliferation dependent on the growth factors (60). This process is highly concordant with mechanisms that are reported in the progression of pterygium (25). MYH11 encoding myosin heavy chain 11 has been confirmed its involvement in several types of cancer and is probably related to metabolism-related genes (61). Five downregulated hub genes are included in the key module that MCODE identified and have interactions with each other, which means they are in the important section of disease development. These five hub genes are transcription factors. Network enrichment analysis shows they are also significantly associated with hormone-related pathways. Among them, FOS and FOSB, as members of the AP-1 transcription factor complex, were upregulated in corneal epithelial cell layers when under UV exposure for one to six hours (62). Furthermore, the c-fos mRNA level is intermediate-early induced by UV radiation in cultured pterygium epithelial cells but downregulated after 12h (52). Additionally, after applying oxidative stress to human retinal pigment epithelium, the changes of expression of ATF3 (another member of AP-1 transcription factors), FOS, and FOSB are in a dose-dependent manner (63). Moreover, overexpression of SPP1 in cells can downregulate the level of c-fos, and SPP1 plays a role in wound healing (64). So, since AP-1 transcription factors are downregulated in this study, we infer that their levels depend on the balance of effects of UV exposure and wound healing. EGR1 encodes early growth response, critically participating in neovascularization, tumor angiogenesis, and growth, together with ATF3, which can influence the limbal epithelial cell proliferation (65, 66). NR4A2 is also associated with tumor proliferation, migration, and invasion (67). In summary, we speculate these hub genes are associated with pterygium proliferation and may response for resistance to UV exposure, these effects may be involved in inactivation of hormone-related pathways.
Finally, we verified the mRNA levels of nine candidate genes through qPCR. Correlation analysis shows the qPCR data are consistent with microarray data, suggesting the credibility of our results. Besides seven hub genes, we also validated two upregulated gene expressions (SFRP2 and SFRP4). They are served as classical antagonists of Wnt signaling and can interact with Wnt protein directly through both autocrine and paracrine modes. In some cases, they also can promote Wnt signaling (68). Wnt signaling can control adult stem cell biology and growth (69); meanwhile, pterygium is thought to be a limbal stem cell disorder (16). The results of these two genes remind us that genes located upstream of the Wnt signaling pathway are involved in the progression of pterygium and probably can be used as therapeutic targets. Additionally, the Wnt beta-catenin signaling is the unique gene set in the results of GSEA in our data, so further in-depth studies are required, especially for the Chinese. Three hub genes (FOS, NR4A2, and SFRP2) are also in our miRNA-mRNA network; the predicted regulatory relationships are worthy of future research.
There were some deficiencies in our study. 1) Our sample size was insufficient and may cause a potential bias. 2) The validations of protein levels of our candidate genes were not conducted. The following study is currently ongoing to remedy the shortcomings of this study.
In conclusion, we consider hormone-related, metabolic, and Wnt signaling pathways may be important in developing pterygium besides its classical mechanisms. Nine candidate genes we identified deserve further study and can be potential therapeutic targets. In short, our findings shed important light on the further study of pterygium.
Data availability statement
The data presented in the study are deposited in the GEO repository, accession number GSE208384.
Ethics statement
The studies involving human participants were reviewed and approved by The Ethics Committee of The Second Affiliated Hospital of Anhui Medical University. The patients/participants provided their written informed consent to participate in this study.
Author contributions
JL and TT wrote this manuscript and performed bioinformatic analyses. YY, NX, and WD conducted data collection and experiment process. ZJ carried out a surgical procedure. MZ and LH provided the idea for this study, and LH revised this manuscript. JL and TT have contributed equally to this work. All authors contributed to the article and approved the submitted version.
Funding
This work was supported by the Beijing-Tianjin-Hebei Special Project (Grant Number J200014), the Science and technology innovation project of the Chinese Academy of medical sciences(2019-RC-HL-019), the National Natural Science Foundation of China (Grant Number 81670870). National Key R&D Program of China (Grant No. 2020YFC2008200). The funders had no role in the study design, data collection, analysis, decision to publish, or manuscript preparation.
Acknowledgments
We are grateful to all the participants and their families for their generosity and support.
Conflict of interest
The authors declare that the research was conducted in the absence of any commercial or financial relationships that could be construed as a potential conflict of interest.
Publisher’s note
All claims expressed in this article are solely those of the authors and do not necessarily represent those of their affiliated organizations, or those of the publisher, the editors and the reviewers. Any product that may be evaluated in this article, or claim that may be made by its manufacturer, is not guaranteed or endorsed by the publisher.
Supplementary material
The Supplementary Material for this article can be found online at: https://www.frontiersin.org/articles/10.3389/fendo.2022.943275/full#supplementary-material
References
1. Liu T, Liu Y, Xie L, He X, Bai J. Progress in the pathogenesis of pterygium. Curr Eye Res (2013) 38(12):1191–7. doi: 10.3109/02713683.2013.823212
2. Van Acker SI, Van den Bogerd B, Haagdorens M, Siozopoulou V, Ni Dhubhghaill S, Pintelon I, et al. Pterygium-the good, the bad, and the ugly. Cells (2021) 10(7):1567. doi: 10.3390/cells10071567
3. Fang XL, Chong CCY, Thakur S, Da Soh Z, Teo ZL, Majithia S, et al. Ethnic differences in the incidence of pterygium in a multi-ethnic Asian population: The Singapore epidemiology of eye diseases study. Sci Rep (2021) 11(1):501. doi: 10.1038/s41598-020-79920-9
4. Moran DJ, Hollows FC. Pterygium and ultraviolet radiation: A positive correlation. Br J Ophthalmol (1984) 68(5):343–6. doi: 10.1136/bjo.68.5.343
5. Fu Q, Mo Z, Gu Y, Lu B, Hao S, Lyu D, et al. Association between outpatient visits for pterygium and air pollution in hangzhou, China. Environ pollut (2021) 291:118246. doi: 10.1016/j.envpol.2021.118246
6. Fonseca EC, Rocha EM, Arruda GV. Comparison among adjuvant treatments for primary pterygium: A network meta-analysis. Br J Ophthalmol (2018) 102(6):748–56. doi: 10.1136/bjophthalmol-2017-310288
7. Ghiasian L, Samavat B, Hadi Y, Arbab M, Abolfathzadeh N. Recurrent pterygium: A review. J Curr Ophthalmol (2021) 33(4):367–78. doi: 10.4103/joco.joco_153_20
8. Lan A, Xiao F, Wang Y, Luo Z, Cao Q. Efficacy of fibrin glue versus sutures for attaching conjunctival autografts in pterygium surgery: A systematic review with meta-analysis and trial sequential analysis of evidence. Oncotarget (2017) 8(25):41487–97. doi: 10.18632/oncotarget.17195
9. Hilmi MR, Kamal K, Azemin M. Pterygium recurrence and corneal stabilization point after pterygium excision using the controlled partial avulsion fibrin glue technique. Makara J Health Res (2020) 24:140–6. doi: 10.7454/msk.v24i2.1195
10. Yajima H, Lee KJ, Zhang S, Kobayashi J, Chen BP. DNA Double-strand break formation upon uv-induced replication stress activates atm and DNA-pkcs kinases. J Mol Biol (2009) 385(3):800–10. doi: 10.1016/j.jmb.2008.11.036
11. Zhou WP, Zhu YF, Zhang B, Qiu WY, Yao YF. The role of ultraviolet radiation in the pathogenesis of pterygia (Review). Mol Med Rep (2016) 14(1):3–15. doi: 10.3892/mmr.2016.5223
12. Kormanovski A, Parra F, Jarillo-Luna A, Lara-Padilla E, Pacheco-Yepez J, Campos-Rodriguez R. Oxidant/Antioxidant state in tissue of prymary and recurrent pterygium. BMC Ophthalmol (2014) 14:149. doi: 10.1186/1471-2415-14-149
13. Han SJ, Min HJ, Yoon SC, Ko EA, Park SJ, Yoon JH, et al. Hmgb1 in the pathogenesis of ultraviolet-induced ocular surface inflammation. Cell Death Dis (2015) 6:e1863. doi: 10.1038/cddis.2015.199
14. Di Girolamo N, Chui J, Coroneo MT, Wakefield D. Pathogenesis of pterygia: Role of cytokines, growth factors, and matrix metalloproteinases. Prog Retin Eye Res (2004) 23(2):195–228. doi: 10.1016/j.preteyeres.2004.02.002
15. Bradley JC, Yang W, Bradley RH, Reid TW, Schwab IR. The science of pterygia. Br J Ophthalmol (2010) 94(7):815–20. doi: 10.1136/bjo.2008.151852
16. Chui J, Coroneo MT, Tat LT, Crouch R, Wakefield D, Di Girolamo N. Ophthalmic pterygium: A stem cell disorder with premalignant features. Am J Pathol (2011) 178(2):817–27. doi: 10.1016/j.ajpath.2010.10.037
17. Shibata N, Ishida H, Kiyokawa E, Singh DP, Sasaki H, Kubo E. Relative gene expression analysis of human pterygium tissues and uv radiation-evoked gene expression patterns in corneal and conjunctival cells. Exp Eye Res (2020) 199:108194. doi: 10.1016/j.exer.2020.108194
18. Jaworski CJ, Aryankalayil-John M, Campos MM, Fariss RN, Rowsey J, Agarwalla N, et al. Expression analysis of human pterygium shows a predominance of conjunctival and limbal markers and genes associated with cell migration. Mol Vis (2009) 15:2421–34.
19. Hou AH, Lan WW, Law KP, Khoo SCJ, Tin MQ, Lim YP, et al. Evaluation of global differential gene and protein expression in primary pterygium: S100a8 and S100a9 as possible drivers of a signaling network. PloS One (2014) 9(5):e97402. doi: 10.1371/journal.pone.0097402
20. Wolf J, Hajdu RI, Boneva S, Schlecht A, Lapp T, Wacker K, et al. Characterization of the cellular microenvironment and novel specific biomarkers in pterygia using rna sequencing. Front Med-Lausanne (2021) 8:714458. doi: 10.3389/fmed.2021.714458
21. de Guimaraes JA, Hounpke BW, Duarte B, Boso ALM, Viturino MGM, de Carvalho Baptista L, et al. Transcriptomics and network analysis highlight potential pathways in the pathogenesis of pterygium. Sci Rep (2022) 12(1):286. doi: 10.1038/s41598-021-04248-x
22. Liu J, Ding X, Yuan L, Zhang X. Identification of pterygium-related mrna expression profiling by microarray analysis. Eye (Lond) (2017) 31(12):1733–9. doi: 10.1038/eye.2017.116
23. Chen Y, Wang H, Jiang Y, Zhang X, Wang Q. Transcriptional profiling to identify the key genes and pathways of pterygium. PeerJ (2020) 8:e9056. doi: 10.7717/peerj.9056
24. Kim YH, Jung JC, Jung SY, Kim YI, Lee KW, Park YJ. Cyclosporine a downregulates mmp-3 and mmp-13 expression in cultured pterygium fibroblasts. Cornea (2015) 34(9):1137–43. doi: 10.1097/Ico.0000000000000477
25. Yang SF, Lin CY, Yang PY, Chao SC, Ye YZ, Hu DN. Increased expression of gelatinase (Mmp-2 and mmp-9) in pterygia and pterygium fibroblasts with disease progression and activation of protein kinase c. Invest Ophth Vis Sci (2009) 50(10):4588–96. doi: 10.1167/iovs.08-3147
26. Li DQ, Lee SB, Gunja-Smith Z, Liu YQ, Solomon A, Meller D, et al. Overexpression of collagenase (Mmp-1) and stromelysin (Mmp-3) by pterygium head fibroblasts. Arch Ophthalmol-Chic (2001) 119(1):71–80.
27. Ritchie ME, Phipson B, Wu D, Hu Y, Law CW, Shi W, et al. Limma powers differential expression analyses for rna-sequencing and microarray studies. Nucleic Acids Res (2015) 43(7):e47. doi: 10.1093/nar/gkv007
28. Yu G, Wang LG, Han Y, He QY. Clusterprofiler: An r package for comparing biological themes among gene clusters. OMICS (2012) 16(5):284–7. doi: 10.1089/omi.2011.0118
29. Shannon P, Markiel A, Ozier O, Baliga NS, Wang JT, Ramage D, et al. Cytoscape: A software environment for integrated models of biomolecular interaction networks. Genome Res (2003) 13(11):2498–504. doi: 10.1101/gr.1239303
30. Wu CW, Cheng YW, Hsu NY, Yeh KT, Tsai YY, Chiang CC, et al. Mirna-221 negatively regulated downstream P27kip1 gene expression involvement in pterygium pathogenesis. Mol Vis (2014) 20:1048–56.
31. Li X, Dai Y, Xu J. Mir-21 promotes pterygium cell proliferation through the Pten/Akt pathway. Mol Vis (2018) 24:485–94.
32. Teng Y, Yam GH, Li N, Wu S, Ghosh A, Wang N, et al. Microrna regulation of Mdm2-P53 loop in pterygium. Exp Eye Res (2018) 169:149–56. doi: 10.1016/j.exer.2018.01.015
33. He S, Huang Y, Dong S, Qiao C, Yang G, Zhang S, et al. Mir-199a-3p/5p participated in tgf-beta and egf induced emt by targeting Dusp5/Map3k11 in pterygium. J Transl Med (2020) 18(1):332. doi: 10.1186/s12967-020-02499-2
34. Lan W, Chen S, Tong L. Microrna-215 regulates fibroblast function: Insights from a human fibrotic disease. Cell Cycle (2015) 14(12):1973–84. doi: 10.1080/15384101.2014.998077
35. Wu CW, Peng ML, Yeh KT, Tsai YY, Chiang CC, Cheng YW. Inactivation of P53 in pterygium influence mir-200a expression resulting in Zeb1/Zeb2 up-regulation and emt processing. Exp Eye Res (2016) 146:206–11. doi: 10.1016/j.exer.2016.03.012
36. Han S, Chen Y, Gao Y, Sun B, Kong Y. Microrna-218-5p inhibit the migration and proliferation of pterygium epithelial cells by targeting egfr Via Pi3k/Akt/Mtor signaling pathway. Exp Eye Res (2019) 178:37–45. doi: 10.1016/j.exer.2018.09.010
37. Cui YH, Li HY, Gao ZX, Liang N, Ma SS, Meng FJ, et al. Regulation of apoptosis by mir-122 in pterygium Via targeting bcl-W. Invest Ophthalmol Vis Sci (2016) 57(8):3723–30. doi: 10.1167/iovs.16-19402
38. Li JH, Liu S, Zhou H, Qu LH, Yang JH. Starbase V2.0: Decoding mirna-cerna, mirna-ncrna and protein-rna interaction networks from Large-scale clip-seq data. Nucleic Acids Res (2014) 42:D92–7. doi: 10.1093/nar/gkt1248
39. Chen C, Chen H, Zhang Y, Thomas HR, Frank MH, He Y, et al. Tbtools: An integrative toolkit developed for interactive analyses of big biological data. Mol Plant (2020) 13(8):1194–202. doi: 10.1016/j.molp.2020.06.009
40. Chu WK, Choi HL, Bhat AK, Jhanji V. Pterygium: New insights. Eye (Lond) (2020) 34(6):1047–50. doi: 10.1038/s41433-020-0786-3
41. Ahmad A, Ahsan H. Biomarkers of inflammation and oxidative stress in ophthalmic disorders. J Immunoassay Immunochem (2020) 41(3):257–71. doi: 10.1080/15321819.2020.1726774
42. de Almeida Borges D, Alborghetti MR, Franco Paes Leme A, Ramos Domingues R, Duarte B, Veiga M, et al. Tear proteomic profile in three distinct ocular surface diseases: Keratoconus, pterygium, and dry eye related to graft-Versus-Host disease. Clin Proteomics (2020) 17(1):42. doi: 10.1186/s12014-020-09307-5
43. Li J, Li C, Wang G, Liu Z, Chen P, Yang Q, et al. Apr-246/Prima-1met inhibits and reverses squamous metaplasia in human conjunctival epithelium. Invest Ophthalmol Vis Sci (2016) 57(2):444–52. doi: 10.1167/iovs.15-17519
44. Zhu CP, Pan FY, Ge LP, Zhou J, Chen LLL, Zhou T, et al. Serpina3k plays antioxidant roles in cultured pterygial epithelial cells through regulating ros system. PloS One (2014) 9(10):e108859. doi: 10.1371/journal.pone.0108859
45. Solomon A, Grueterich M, Li DQ, Meller D, Lee SB, Tseng SC. Overexpression of insulin-like growth factor-binding protein-2 in pterygium body fibroblasts. Invest Ophthalmol Vis Sci (2003) 44(2):573–80. doi: 10.1167/iovs.01-1185
46. Qin YJ, Chu WK, Huang L, Ng CHY, Chan TCY, Cao D, et al. Induction of apoptosis in pterygium cells by antagonists of growth hormone-releasing hormone receptors. Invest Ophthalmol Vis Sci (2018) 59(12):5060–6. doi: 10.1167/iovs.18-24751
47. Baser H, Cuhaci N, Topaloglu O, Yulek F, Ugurlu N, Ersoy R, et al. Is there any association between primary hyperparathyroidism and ocular changes, such as central corneal thickness, retinal thickness, and intraocular pressure? Endocrine (2016) 51(3):545–50. doi: 10.1007/s12020-015-0724-5
48. Stuard WL, Titone R, Robertson DM. The Igf/Insulin-igfbp axis in corneal development, wound healing, and disease. Front Endocrinol (Lausanne) (2020) 11:24. doi: 10.3389/fendo.2020.00024
49. Hiramoto K, Yamate Y. Long-term uva exposure to the eye compromises memory and learning ability in mice Via corticotropin-releasing hormone type 2 receptor. Int J Biol Sci (2020) 16(12):2170–9. doi: 10.7150/ijbs.45967
50. Kuo CH, Miyazaki D, Yakura K, Araki-Sasaki K, Inoue Y. Role of periostin and interleukin-4 in recurrence of pterygia. Invest Ophthalmol Vis Sci (2010) 51(1):139–43. doi: 10.1167/iovs.09-4022
51. Siak JJ, Ng SL, Seet LF, Beuerman RW, Tong L. The nuclear-factor kappab pathway is activated in pterygium. Invest Ophthalmol Vis Sci (2011) 52(1):230–6. doi: 10.1167/iovs.10-5735
52. Di Girolamo N, Coroneo M, Wakefield D. Epidermal growth factor receptor signaling is partially responsible for the increased matrix metalloproteinase-1 expression in ocular epithelial cells after uvb radiation. Am J Pathol (2005) 167(2):489–503. doi: 10.1016/s0002-9440(10)62992-6
53. He S, Sun H, Huang Y, Dong S, Qiao C, Zhang S, et al. Identification and interaction analysis of significant genes and micrornas in pterygium. BioMed Res Int (2019) 2019:2767512. doi: 10.1155/2019/2767512
54. Xu Y, Qiao C, He S, Lu C, Dong S, Wu X, et al. Identification of functional genes in pterygium based on bioinformatics analysis. BioMed Res Int (2020) 2020:2383516. doi: 10.1155/2020/2383516
55. Xu N, Cui Y, Dong J, Huang L. Exploring the molecular mechanisms of pterygium by constructing lncrna-Mirna-Mrna regulatory network. Invest Ophthalmol Vis Sci (2020) 61(8):12. doi: 10.1167/iovs.61.8.12
56. Yang J, Weinberg RA. Epithelial-mesenchymal transition: At the crossroads of development and tumor metastasis. Dev Cell (2008) 14(6):818–29. doi: 10.1016/j.devcel.2008.05.009
57. Shu DY, Lovicu FJ. Myofibroblast transdifferentiation: The dark force in ocular wound healing and fibrosis. Prog Retin Eye Res (2017) 60:44–65. doi: 10.1016/j.preteyeres.2017.08.001
58. Liu L, Yang D. Immunological studies on the pathogenesis of pterygium. Chin Med Sci J (1993) 8(2):84–8.
59. Cui D, Pan Z, Zhang S, Zheng J, Huang Q, Wu K. Downregulation of c-myc in pterygium and cultured pterygial cells. Clin Exp Ophthalmol (2011) 39(8):784–92. doi: 10.1111/j.1442-9071.2011.02531.x
60. Icer MA, Gezmen-Karadag M. The multiple functions and mechanisms of osteopontin. Clin Biochem (2018) 59:17–24. doi: 10.1016/j.clinbiochem.2018.07.003
61. Wang J, Xu P, Hao Y, Yu T, Liu L, Song Y, et al. Interaction between Dnmt3b and Myh11 Via hypermethylation regulates gastric cancer progression. BMC Cancer (2021) 21(1):914. doi: 10.1186/s12885-021-08653-3
62. Gillardon F, Zimmermann M, Uhlmann E. Expression of c-fos and c-jun in the cornea, lens, and retina after ultraviolet irradiation of the rat eye and effects of topical antisense oligodeoxynucleotides. Br J Ophthalmol (1995) 79(3):277–81. doi: 10.1136/bjo.79.3.277
63. Chaum E, Yin J, Yang H, Thomas F, Lang JC. Quantitative ap-1 gene regulation by oxidative stress in the human retinal pigment epithelium. J Cell Biochem (2009) 108(6):1280–91. doi: 10.1002/jcb.22358
64. Liu X, Sun Y, Li H, Li Y, Li M, Yuan Y, et al. Effect of Spp1 on nerve degeneration and regeneration after rat sciatic nerve injury. BMC Neurosci (2017) 18(1):30. doi: 10.1186/s12868-017-0348-1
65. Hsueh YJ, Kuo PC, Chen JK. Transcriptional regulators of the Deltanp63: Their role in limbal epithelial cell proliferation. J Cell Physiol (2013) 228(3):536–46. doi: 10.1002/jcp.24160
66. Fahmy RG, Dass CR, Sun LQ, Chesterman CN, Khachigian LM. Transcription factor egr-1 supports fgf-dependent angiogenesis during neovascularization and tumor growth. Nat Med (2003) 9(8):1026–32. doi: 10.1038/nm905
67. Jing CY, Fu YP, Zhou C, Zhang MX, Yi Y, Huang JL, et al. Hepatic stellate cells promote intrahepatic cholangiocarcinoma progression Via Nr4a2/Osteopontin/Wnt signaling axis. Oncogene (2021) 40(16):2910–22. doi: 10.1038/s41388-021-01705-9
68. Pawar NM, Rao P. Secreted frizzled related protein 4 (Sfrp4) update: A brief review. Cell Signal (2018) 45:63–70. doi: 10.1016/j.cellsig.2018.01.019
Keywords: Pterygium, microarray, ocular surface disease, conjunctiva, hormone, metabolism
Citation: Li J, Tao T, Yu Y, Xu N, Du W, Zhao M, Jiang Z and Huang L (2022) Expression profiling suggests the involvement of hormone-related, metabolic, and Wnt signaling pathways in pterygium progression. Front. Endocrinol. 13:943275. doi: 10.3389/fendo.2022.943275
Received: 13 May 2022; Accepted: 24 August 2022;
Published: 14 September 2022.
Edited by:
Stephen A. Whelan, Cedars-Sinai, United StatesReviewed by:
Yun Feng, Peking University Third Hospital, ChinaPir Salim Mahar, Aga Khan University, Pakistan
Mohd Radzi Hilmi, International Islamic University Malaysia, Malaysia
Copyright © 2022 Li, Tao, Yu, Xu, Du, Zhao, Jiang and Huang. This is an open-access article distributed under the terms of the Creative Commons Attribution License (CC BY). The use, distribution or reproduction in other forums is permitted, provided the original author(s) and the copyright owner(s) are credited and that the original publication in this journal is cited, in accordance with accepted academic practice. No use, distribution or reproduction is permitted which does not comply with these terms.
*Correspondence: Lvzhen Huang, aHVhbmdsdnpoZW5AMTI2LmNvbQ==; Zhengxuan Jiang, amlhbmd6aGVuZ3h1YW5AYWhtdS5lZHUuY24=
†These authors share first authorship