- 1Department of Rheumatology & Immunology, West China Hospital of Sichuan University, Chengdu, China
- 2Department of Orthopedics, People’s Hospital of Zhongjiang County, Deyang, China
- 3Department of Rheumatology& Immunology, China-Japan Friendship Hospital, Beijing, China
- 4Department of Pediatrics Surgery, Lok Nayak Hospital, New Delhi, India
- 5Department of Interventional Oncology, Renji Hospital, School of Medicine, Shanghai Jiao Tong University, Shanghai, China
Aims: This study aimed to assess the impact of different antidiabetic agents on individuals with diabetes and COVID-19.
Methods: We searched PubMed, Web of Science, Embase, and Cochrane Library databases from inception to October 31, 2021 and included seven antidiabetic agents. The data were pooled via traditional pairwise meta-analysis and Bayesian network meta-analysis.
Results: The pairwise meta-analysis included 35 studies. Metformin (odds ratio (OR), 0.74; P=0.001), dipeptidyl peptidase-4 inhibitors (DPP4i) (OR, 0.88; P=0.04), sodium-glucose cotransporter-2 inhibitors (SGLT2i) (OR, 0.82; P=0.001), and glucagon-like peptide-1 receptor agonists (GLP1RA) (OR, 0.91; P=0.02) treatment were associated with lower COVID-19 mortality in individuals with diabetes compared to respective non-users. However, insulin treatment resulted in higher mortality (OR, 1.8; P=0.001). Mortality did not significantly differ in sulfonylurea (OR, 0.97; P=0.56) and thiazolidinediones (TZDs) (OR, 1.00; P=0.96) users. Furthermore, due to limited data, we analyzed five antidiabetic agents (metformin, DPP4i, sulfonylurea, insulin, and SGLT2i) and found no association between them and severe disease risk (all P>0.05). The Bayesian network meta-analysis included 18 studies. GLP1RA and SGLT2i had the highest first and second rank probability (67.3% and 62.5%, respectively). Insulin showed the maximum probability of ranking seventh (97.0%). Metformin had the third and fourth highest rank probability of 44.8% and 38.9%, respectively. Meanwhile, DPP4i had the fifth-highest rank probability of 42.4%, followed by sulfonylurea at 45.1%.
Conclusion: Metformin, DPP4i, SGLT2i, and GLP1RA treatments were highly possible to reduced COVID-19 mortality risk in individuals with diabetes, while insulin might be related to increased mortality risk. Sulfonylurea and TZDs treatments were not associated with mortality. None of the antidiabetic agents studied were associated with the risk of severe disease. Additionally, GLP1RA probably had the most significant protective effect against death, followed by SGLT2i and metformin.
Systematic Review Registration: PROSPERO (CRD42021288200)
Introduction
The ongoing pandemic of Coronavirus disease-19 (COVID-19) is an emerging, rapidly evolving situation that poses challenges for diabetic patients. Previous studies have demonstrated that diabetes is an independent risk factor for worse outcomes and in-hospital mortality in COVID-19 patients [1]. The percentage of COVID-19 patients with diabetes in hospitalization was high, with adverse effects on their prognosis, and most COVID-19 fatalities occurred among diabetic patients. It is necessary to decide a relatively beneficial treatment plan for diabetic patients with COVID-19 among the massive diabetes treatment plans.
Antidiabetic agents have been demonstrated to exhibit an antidiabetic role and anti-inflammatory and immunomodulatory effects (1, 2). Numerous studies have been conducted to determine the impact of antidiabetic agents on clinical outcomes of diabetic patients with COVID-19. Additionally, some pairwise meta-analyses researched the role of a specific antidiabetic agent on the mortality and severe poor outcomes of COVID-19, including metformin, dipeptidyl peptidase 4 inhibitors (DPP4i), sulfonylurea, insulin, and glucagon-like peptide-1 receptor agonists (GLP1RA) (1, 3, 4). However, these investigations merely compared the outcomes of users with specific antidiabetic agents to those of non-users. The mortality risks between different antidiabetic agents in these patients remain unclear.
To date, no comprehensive network meta-analysis has been performed to explore the associations between different antidiabetic drugs and COVID-19 patients’ outcomes. The current analysis aims to evaluate the association between different antidiabetic agents with the risk of COVID-19 mortality, as well as the risk of severe disease outcomes among individuals with diabetes.
Material and Methods
Literature Search
The literatures of any design were searched in PubMed, Embase, and Cochrane Library databases from inception to October 30, 2021, following the Meta-analyses Of Observational Studies in Epidemiology (MOOSE) guidelines as described (5). The PROSPERO registration number is CRD42021288200. The search terms included antidiabetic agents, metformin, DPP4i, sulfonylurea, thiazolidinediones (TZDs), insulin, sodium-glucose cotransporter-2 inhibitors (SGLT2i), GLP1RA, glinides, α-glycosidase inhibitors, and COVID-19. Each search strategy included a combination of one antidiabetic agent and COVID-19. The exact search terms are shown in Supplementary Table 1 (S Table 1). We limited our search by the English language. The retrieved articles were imported into EndnoteX9 and extracted by screening their titles and abstracts. Duplicate studies and multiple reports using the same data were removed. After preliminary screening, nonconforming literature was excluded by reading the full text, and the final remaining articles were included in this study.
Inclusion and Exclusion Criteria
This study comprised studies that met the following criteria: (1) original studies evaluating the clinical outcomes of diabetic COVID-19 patients receiving specific antidiabetic agents at home or in the hospital; (2) study designs including randomized controlled trials (RCT), and observational studies; (3) studies published in English. The exclusion criteria included the following: (1) reviews, systematic reviews, correspondences, or in vitro animal studies; (2) studies with incomplete data and indeterminate outcome endpoints; (3) abstracts, unpublished studies, and retracted manuscripts.
Data Extraction and Quality Assessment
Two independent reviewers used a predetermined data collection table to extract relevant data. Disagreements were solved by discussion and following a third author’s opinion. The main extracted data included the following: study characteristics (first author, publication date, country, and study design), patient characteristics (sample size, mean age, and male proportion), as well as study’s outcomes data. Newcastle-Ottawa Quality Assessment Scale criteria were utilized to assess the risk of bias for the included studies (6, 7). According to this scale, a study can be awarded a maximum of nine stars; four stars for study selection, two stars for comparability, and three stars for outcome sections.
Outcomes of Interest
Our primary outcome was the mortality of individuals with diabetes and COVID-19. The secondary outcome was the incidence of severe disease. Here, we defined the cases with poor outcomes as those requiring intensive care unit admission, mechanical ventilation of acute respiratory distress syndrome, disseminated intravascular coagulation, or others described in the individual study as severe disease.
Statistical Analysis
Meta-analysis and Bayesian network were conducted only in studies >3 per outcome. Meta-analysis was performed using Stata 16.0 (Stata Corp) software. Both chi-squared and I2 tests estimated heterogeneity between studies. The pooled effect sizes were analyzed using a random-effects model (I2 >0.5, P <0.1) and fixed-effects model (I2 <0.5, P >0.1). As reported by individual cohort studies, pooled OR was estimated by combining relative risk (RR) or odds ratio (OR) (hazards ratio was presumably equivalent to RR). If studies included unadjusted and covariate-adjusted data, we selected the latter. To stabilize the variances, we transformed each study’s effect estimates and their confidence intervals (CI) to natural logarithms (8, 9). If the number of studies was >5, publication bias was evaluated using Begg’s test, Egger’s test, and the trim-and-fill method. Subgroup analyses based on data types (adjusted or unadjusted data), effect size types (OR or RR), diabetes types (only T2DM or not only T2DM), and medication use timing (use before admission or in-hospital) would be established when the number of studies in a subgroup was greater than three. Meta-regression analysis was performed to assess the potential impact of the study’s sample size, patients’ mean age, and gender on the pooled results.
The Bayesian network meta-analysis was performed using R 4.1 software with gemtc and rjags packages as previously described (10). The network meta-analysis employed OR and 95% credibility intervals (95% CrIs). Our study’s consistency was determined by comparing the fit of consistency and inconsistency models using deviance information criteria (differences < 5 are considered consistency fitting) (11). We calculated the rank probabilities of the effects of all drugs available in the network. Additionally, the probability of the first to seventh of each drug was visualized using radar plots of ranking probability for the network. P ≤ 0.05 was considered statistically significant.
Results
Study Selection and Characteristics
The search strategy identified 681 potentially relevant studies, of which 249 were excluded due to duplication. Among the remaining 432 studies, 384 were excluded due to their titles or abstracts. Therefore, 48 studies were eligible for full-text review. After re-excluding six articles, this study comprised 42 full-text articles (Figure 1). The vast majority of them were observational studies (6 cross-sectional studies, 1 case-control study, and 34 cohort studies) and only one article was RCT. Table 1 summarizes the baseline characteristics of patients in the included studies.
Quality Assessment
Newcastle-Ottawa Quality Assessment measured the quality of studies. The quality scores of studies ranged from seven to nine stars with a median of eight stars (S Table 2).
Meta-analysis of the Impact of Using Antidiabetic Agents on Patients’ Clinical Outcomes
The pairwise meta-analysis included 35 studies (12, 14, 17, 18, 21, 24–53), of which 25 (12, 14, 17, 21, 24–27, 30–46) were related to metformin, 21 (12, 14, 17, 18, 21, 25–29, 36, 38, 41, 43, 46–52) were linked to DPP4i, 10 (12, 17, 21, 26, 27, 36, 41, 43, 46, 49) were associated with sulfonylurea, 5 (12, 18, 27, 36, 43) were related to TZDs, 14 (12, 14, 17, 21, 24–27, 36, 38, 41, 43, 45, 46) were linked to insulin, 8 (12, 14, 17, 27, 41, 43, 49, 53) were correlated with SGLT2i, 7 (12, 14, 18, 26, 27, 43, 46) were linked to GLP1RA, 2 (27, 38) were associated with acarbose (α-glycosidase inhibitors), and one (27) was correlated with glinides. Some of these studies included multiple different medications.
Qualitative meta-analysis revealed that metformin users had a significantly reduced mortality rate than non-users. (Figure 2A; pooled OR, 0.74; 95% CI: 0.67-0.81; P=0.00). Subgroup analyses based on data types, effect size types, and diabetes types respectively revealed lower mortality in metformin users in each subgroup (S Figures 1A, 2A and 3A, all subgroups P < 0.05). In the Subgroup analyses based on medication use timing, metformin use before admission was associated with a significantly reduced mortality rate (pooled OR, 0.76; 95% CI: 0.69-0.84), but no such an association was observed in in-hospital use subgroup (pooled OR, 0.41; 95% CI: 0.11-1.60) (S Figures 4A). Using DPP4i in individuals with diabetes was also associated with reduced mortality from COVID-19 (Figure 2B; pooled OR, 0.88; 95% CI: 0.78-1.00; P=0.04). Further subgroup analysis indicated that DPP4i use remained linked to a statistically decreased mortality after adjusting for age, gender, and other characteristics (S Figure 1B; pooled OR, 0.86; 95% CI: 0.75-0.98; P=0.02) whereas was not statistically significant in unadjusted data (P > 0.05). However, in subgroup analyses based on effect size types, diabetes types, and DPP4i use timing, there was no statistically significant difference in each subgroup (S Figures 2B, 3B, and 4B, all subgroups P > 0.05). Thus, caution is needed when interpreting the effect of DPP4i on individuals with diabetes and COVID-19. For sulfonylurea or TZDs medications, no significant difference in mortality was observed between medication users and non-users (sulfonylurea: Figure 2C; pooled OR, 0.97; 95% CI: 0.88-1.07; P=0.56, TZDs: Figure 2D; pooled OR, 1.00; 95% CI: 0.90-1.10; P=0.96). Subgroup analysis based on data types, effect size types, diabetes types, and sulfonylurea use timing demonstrated similar results respectively (S Figures 1C, 2C, 3C, and 4C; all subgroups P > 0.05). Insulin treatment was significantly associated with increased mortality compared to non-users (Figure 3A; pooled OR, 1.38; 95% CI: 1.24-1.54; P=0.00). In subgroup analysis based on data types, effect size types and diabetes types, there was also higher mortality in each insulin subgroup (S Figures 1D, 2D and 3D, all subgroups P < 0.05); while subgroup analysis based on insulin using timing revealed that higher mortality was only observed in before-admission use subgroup (S Figures 4D, pooled OR, 1.43; 95% CI: 1.25-1.64). Finally, therapy of SGLT2i or GLP1RA was significantly associated with a reduction in mortality compared to non-users (SGLT2i: Figure 3B; pooled OR, 0.82; 95% CI: 0.76-0.88; P=0.00, GLP1RA: Figure 3C; pooled OR, 0.91; 95% CI: 0.84-0.98; P=0.02). Subgroup analysis was not performed due to insufficient SGLT-2i or GLP-1RA treatment data. Furthermore, meta-regression analysis disclosed that the study’s sample size, patients’ mean age, and gender did not significantly impact the association of antidiabetic agents on patients’ mortality (all P > 0.05) (Table 2).
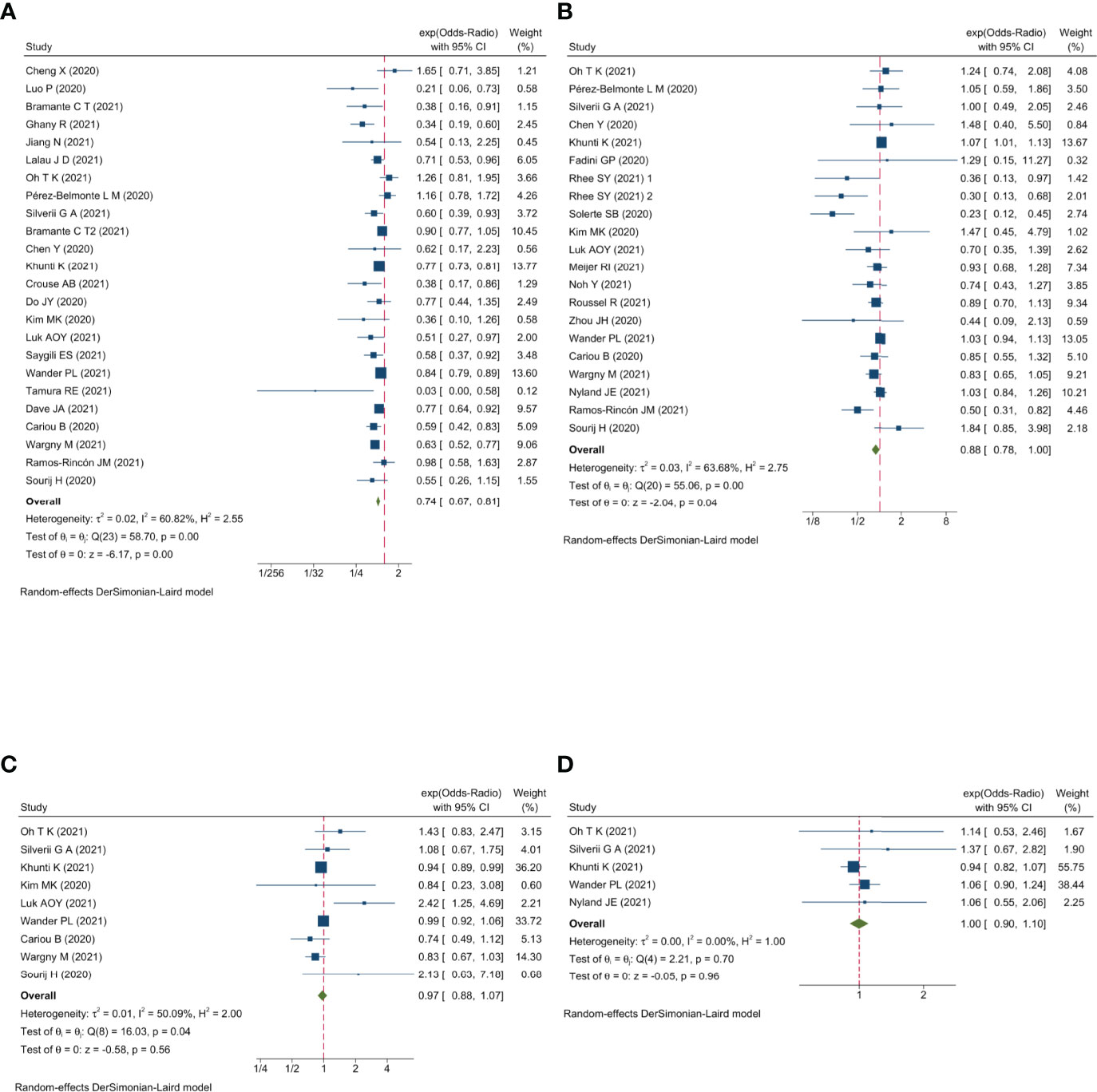
Figure 2 Forest plot showing the association between antidiabetic agents use and mortality. (A) Metformin; (B) DPP4i; (C) Sulfonylurea; (D) TZDs.
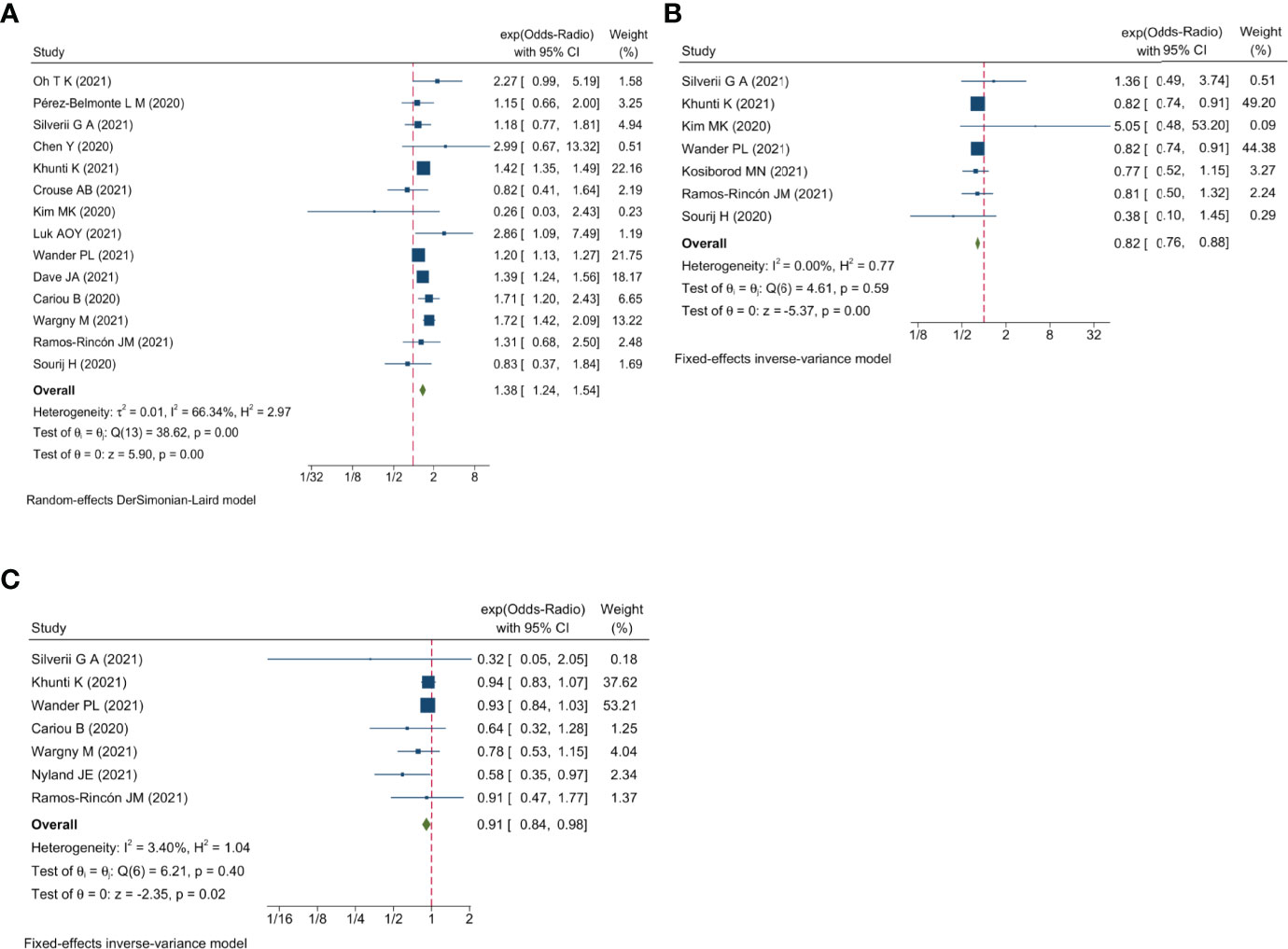
Figure 3 Forest plot showing the association between antidiabetic agents use and mortality. (A) Insulin; (B) SGLT2i; (C) GLP1RA.
Most studies did not report the outcome of severe disease. Due to a scarcity of data, a qualitative meta-analysis of only five antidiabetic agents was performed (metformin, DPP4i, sulfonylurea, insulin, and SGLT2i). The pooled results demonstrated no significant associations between antidiabetic agent use and risk of severe disease (all P > 0.05) (S Figure 5).
Begg’s and Egger’s tests revealed no publication bias for sulfonylurea, TZDs, insulin, and SGLT2i mortality (all P > 0.05), while Egger’s test manifested publication bias for metformin, DPP4i, and GLP1RA (Egger’s test P = 0.00, 0.04, and 0.04, respectively). The trim-and-fill analysis suggested no evidence of a significant difference between adjusted and original values of all antidiabetic agents (Table 2 and S Figure 6), implying that the results in this meta-analysis were relatively robust.
Bayesian Network Meta-Analysis of the Association Between Antidiabetic Agents and Mortality
The Bayesian network meta-analysis included 18 studies (12–29) and included seven different antidiabetic agents: metformin, DPP4i, sulfonylurea, TZDs, insulin, SGLT2i, and GLP1RA. Due to lack of relevant data, we assessed only the association between different antidiabetic agents and mortality in this Bayesian network meta-analysis and did not examine the risk of severe disease. The network plot is displayed in Figure 4.
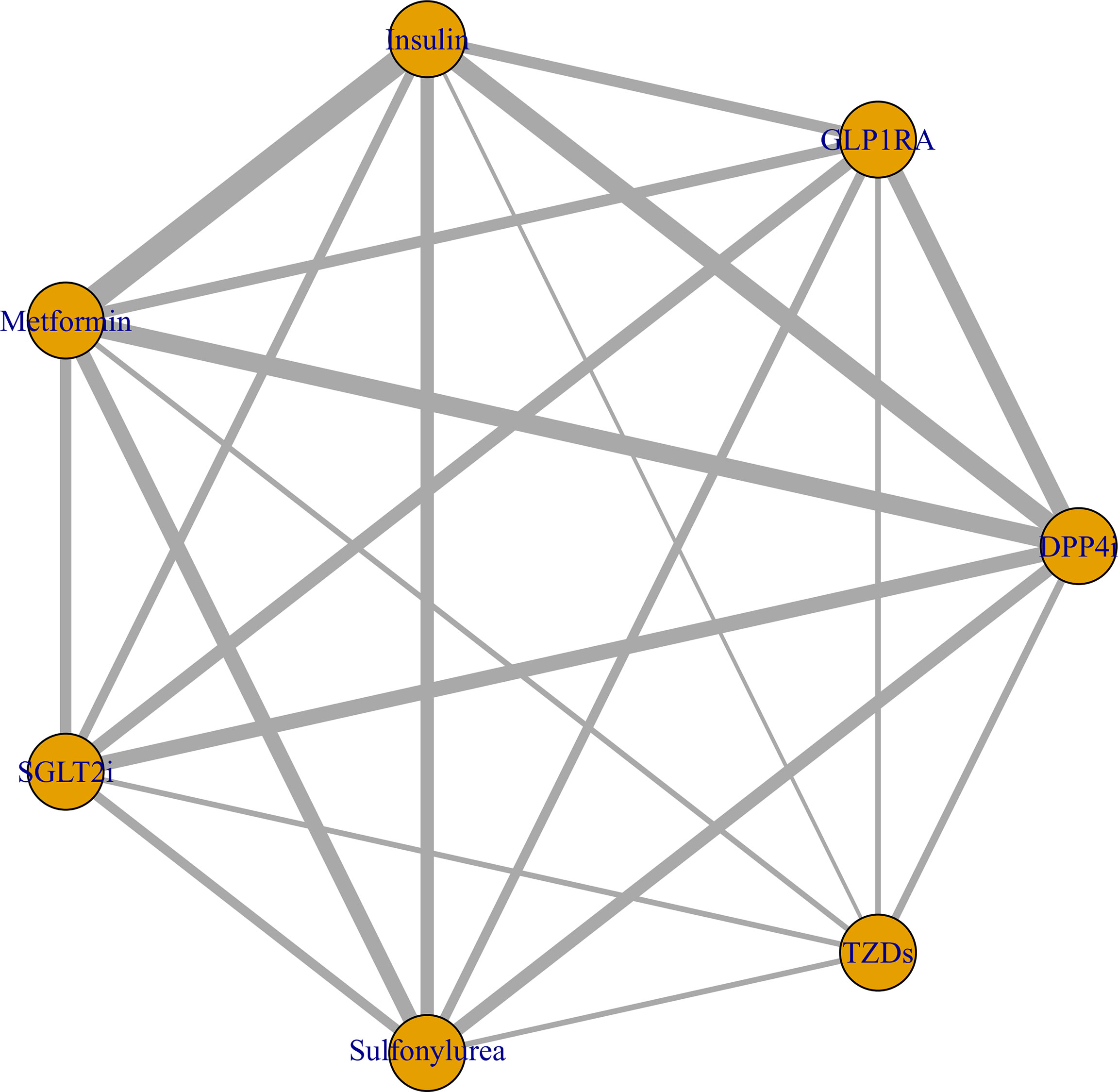
Figure 4 Network plot of the association between different antidiabetic agents and the risk of COVID-19 mortality in diabetic patients. The thickness of lines corresponds to the number of trials.
Using ranking probabilities, Bayesian analysis depicted an overall comparison of the effect of each antidiabetic drug on mortality risk in the network (Figure 5). GLP1RA and SGLT2i had the first and second highest rank probabilities of reducing mortality (67.3% and 62.5%, respectively). Insulin had the highest seventh rank probability (97.0%). Metformin had the third and fourth rank probability of 44.8% and 38.9%, respectively. Meanwhile, DPP4i had the fifth-highest rank probability of 42.4%, followed by sulfonylurea at 45.1%.
When taking insulin as a reference, all other antidiabetic agents were associated with a decreased mortality (OR for metformin, 0.56; 95% CrI, 0.42-0.73, OR for DPP4i, 0.66; 95% CrI, 0.49-0.88, OR for sulfonylurea, 0.68; 95% CrI, 0.47-0.97, OR for TZDs, 0.57; 95% CrI, 0.36-0.93, OR for SGLT2i, 0.36; 95% CrI, 0.24-0.55, OR for GLP1RA, 0.33; 95% CrI, 0.23-0.48). Compared with metformin, DPP4i, or sulfonylurea, only GLP1RA and SGLT2i could significantly reduce the mortality, whereas insulin increased the mortality (Table 3). All but SGLT2i increased mortality when compared to GLP1RA. Compared with SGLT2i, significantly increased mortality was observed in DPP4i, insulin, metformin, and sulfonylurea treatment groups (Table 3).
Consistency results of direct and indirect estimates were detected with relevant P-value larger than 0.05 in any closed loops using the node-splitting method (all P > 0.05, S Figure 7). The Brooks-Gelman-Rubin plots indicated that the model had a sufficient convergence in the entire network (S Figure 8). Global I2 was employed to evaluate heterogeneity which was 0.4% for mortality. The model fit was estimated by comparing the posterior mean residual deviance with the number of data points, and the results were similar (S Table 3). Additionally, network meta-regression analysis revealed that study sample size, mean age, and gender did not significantly impact mortality (S Figures 9–11).
Discussion
This is the first comprehensive Bayesian network meta-analysis of different antidiabetic agents for individuals with diabetes and COVID-19. When multiple treatment options are available, network meta-analysis with Bayesian statistics allows for robust mixed treatment comparison analysis. Furthermore, it enables combined direct and indirect comparisons of competing treatments while retaining the advantages of randomization (54). In this study, we assessed the association between various antidiabetic agents and mortality as well as the risk of severe disease in individuals with diabetes and COVID-19. This analysis included 42 studies and seven antidiabetic agents: metformin, DPP4i, sulfonylurea, TZDs, insulin, SGLT2i, and GLP1RA. The results revealed that metformin, DPP4i, SGLT2i, and GLP1RA treatment exhibited lower mortality than respective non-users, while insulin was linked to a higher mortality rate. Treatment with sulfonylurea and TZDs had no impact on mortality. Due to limited data, a meta-analysis of five antidiabetic agents (metformin, DPP4i, sulfonylurea, insulin, and SGLT2i) revealed no association with the risk of incidence of severe disease. Additionally, GLP1RA had the most significant protective effect against mortality among individuals with diabetes and COVID-19, followed by SGLT2i and metformin. Insulin was associated with the highest risk of mortality.
In this meta-analysis, metformin use was associated with decreased COVID-19 mortality, consistent with several previous studies (1, 3, 55). Moreover, statistical significance was observed between subgroups in the subgroup analyses, demonstrating that the overall results about metformin were stable and reliable. Inflammation has long been recognized as a critical factor in the development and severity of COVID-19. Severe COVID-19 is correlated with a hyperinflammatory state and cytokine storm, which can cause multi-organ damage (56). Metformin is the first-line drug in the treatment of diabetes. It has anti-inflammatory characteristics and has been shown to decrease circulating inflammatory biomarkers (2), which might explain why it is beneficial in diabetic patients with COVID-19. Furthermore, some scholars pointed out that metformin’s ability to reduce neutrophil counts and neutrophil extracellular traps might contribute to these protective mechanisms (57, 58).
In addition, we found that DPP4i use was associated with lower mortality, even when subgroup pooled adjusted data were included. A consistent conclusion has not been drawn in the previously published meta-analyses about DPP4i. The study (59) of Yang et al. concluded that DPP4i increased the mortality of patients with COVID-19 and diabetes, while Rakhmat’s study (60) concluded that DPP4i use was linked to decreased mortality. The other two meta-analyses (56, 61) suggested that DPP4i use was unrelated to mortality. The result of this contradictory evidence could be explained by the uncertain anti-inflammatory properties of DPP4i. In some studies, DPP4i treatment attenuated inflammasome activation and decreased human plasma levels of inflammatory biomarkers such as IL-6, IL-18, CRP, and so on (28, 62). However, some studies concluded that DPP4i did not affect human plasma levels of inflammatory biomarkers (56, 63). Additionally, the impact of DPP4i on active intact cytokines and chemokines can be paradoxical. By preventing inflammatory factors from being degraded enzymatically by DPP4, DPP4i may potentially enhance the activity of certain inflammatory networks (64), which might be the underlying reason for the high mortality observed by Yang et al. (59).
The pooled results of sulfonylurea from nine studies revealed no connection between sulfonylurea treatment and COVID-19 mortality. This finding contradicts a previous meta-analysis, in which five studies were included (3). Luk AOY et al. (21) reported a database that captured all COVID-19 patients in China Hong Kong, revealing that sulfonylurea use was associated with increased mortality. However, no statistical significance was observed in the remaining included studies. The specific potential mechanism remains unclear. TZDs is another glucose-lowering agent with the properties of modulating inflammatory and oxidative stress. However, some scholars pointed out that TZDs therapy was associated with weight gain, oedema, and heart failure, implying that it was unsuitable for COVID-19 patients (65). There was no meta-analysis about the effect of TZDs on COVID-19 so far. In this study, pooled results from five studies did not show any significant association between TZDs treatment and COVID-19 mortality as well as the risk of severe disease in diabetic patients.
In our meta-analysis, insulin administration was significantly associated with increased COVID-19 mortality, consistent with previous studies (1, 66). The underlying mechanism between the association of insulin use and COVID-19 remains unclear. Insulin exerted variable antioxidant and anti-inflammatory effects, including induction of nitric oxide production and inhibition of reactive oxygen species (67). Additionally, insulin therapy was proved reducing reactive oxygen species and macrophage infiltration into the liver and omentum in a diabetic rat model, resulting in a reduction in systematic inflammatory status (68). But in another sepsis model of diabetic rats, insulin showed a pro-inflammation ability in the lung (69). The lung was the main targeted organ affected by COVID-19. From a clinical perspective, some scholars worried that because insulin therapy was typically administered to patients with late-stage diabetes, it is difficult to exclude the negative effect of late-stage diabetes on the increased mortality. For instance, when insulin users were admitted to the hospital, they showed higher inflammatory markers, more comorbidities and lower lymphocyte counts than non-users (21). Based on the above, careful assessment of the benefits and adverse effects of insulin was required.
It is known that GLP1RA not only lowers glucose but also controls inflammation-induced lung injury through pulmonary protective effects and reduces major cardiovascular complications (70). Due to the prevalence of cardiovascular disease in diabetic patients, GLP1RA might be beneficial for glucose management in diabetic patients with COVID-19 (2). Moreover, GLP1RA works on GLP1 receptors primarily located on epithelial of the lung and immune cells. Rogliani et al. (71) reported that GLP1RA improved lung function in diabetic patients, while non-GLP1RA treatment or insulin treatment did not. Our meta-analysis corroborated the benefits of GLP1RA in managing COVID-19: lower mortality in GLP1RA users was observed compared to non-users.
SGLT2i is a new class of glucose-lowering agents, which has remarkable cardiorenal protective properties, especially in populations with atherosclerosis, heart failure, and reduced renal function (2, 72). Meanwhile, it has been shown to suppress systemic inflammation and fibrosis and alleviate organ damage (72, 73). The only RCT (53) included showed that patients treated with dapagliflozin had a lower but non-significant mortality. Our meta-analysis identified a significantly reduced mortality in SGLT2i users compared with non-users. Before this study, there have been no meta-analyses on the effect of SGLT2i on individuals with diabetes and COVID-19.
By conducting a Bayesian network meta-analysis on these seven antidiabetic agents to compare COVID-19 mortality between different antidiabetic agents, we discovered that GLP1RA had the most significant protective effect on COVID-19 mortality among individuals with diabetes, followed by SGLT2i and metformin. In contrast, insulin was associated with the highest risk of mortality. When metformin was used as a reference, GLP1RA and SGLT2i reduced mortality, whereas insulin increased mortality; no statistical significance was observed in other agents. Our Bayesian network meta-analysis demonstrated a good consistency of direct and indirect comparisons, indicating that the overall results were stable and reliable.
Although our comprehensive meta-analysis established a link between antidiabetic agents and the risk of COVID-19 clinical outcomes, our study has numerous limitations. First, most included studies were observational, including some confounding variables. We performed regression analysis on sample size, mean age, and male proportion. These results showed that these factors did not influence meta-analysis results. However, other confounding factors may remain. Second, in our pairwise meta-analysis, we did not perform an OR-to-RR effective size conversion and pooled OR and RR together. But we performed a subgroup analysis based on OR and RR. The results of subgroup analyses were consistent with those from the overall meta-analysis. Third, most of the included studies were observational, while only one RCT was included in the currently available literatures; thus, these results should be interpreted with caution.
Conclusion
In conclusion, our thorough pairwise meta-analysis and Bayesian network meta-analysis revealed that using metformin, DPP4i, GLP1RA, and SGLT2i was high likely associated with decreased mortality in individuals with diabetes and COVID-19, while insulin treatment might contribute to the increased mortality. Using Sulfonylurea or TZDs had no statistically significant association with mortality. Furthermore, the meta-analysis found no association between the risk of severe disease and five antidiabetic agents (metformin, DPP4i, sulfonylurea, insulin, and SGLT2i). Comparative data between these antidiabetic agents revealed that GLP1RA probably had the most significant protective effect on COVID-19 mortality among individuals with diabetes, followed by SGLT2i and metformin. Insulin was correlated with the highest risk of mortality. More rigorous randomized controlled design investigations are required to further validate these findings.
Data Availability Statement
The original contributions presented in the study are included in the article/Supplementary Material. Further inquiries can be directed to the corresponding author.
Author Contributions
YC, XL, and SL: Conceptualization, methodology, data curation, investigation, and writing - original draft preparation. MA and MD: Supervision, writing - reviewing and editing, and funding acquisition. All authors contributed to the article and approved the submitted version.
Funding
The authors gratefully acknowledge the financial support by the science and technology Program of Science and Technology Commission Shanghai Municipality under Grant numbers 19441907000.
Conflict of Interest
The authors declare that the research was conducted in the absence of any commercial or financial relationships that could be construed as a potential conflict of interest.
Publisher’s Note
All claims expressed in this article are solely those of the authors and do not necessarily represent those of their affiliated organizations, or those of the publisher, the editors and the reviewers. Any product that may be evaluated in this article, or claim that may be made by its manufacturer, is not guaranteed or endorsed by the publisher.
Supplementary Material
The Supplementary Material for this article can be found online at: https://www.frontiersin.org/articles/10.3389/fendo.2022.895458/full#supplementary-material
References
1. Han T, Ma S, Sun C, Zhang H, Qu G, Chen Y, et al. The Association Between Anti-Diabetic Agents and Clinical Outcomes of Covid-19 in Patients With Diabetes: A Systematic Review and Meta-Analysis. Arch Med Res (2021) S0188-4409(21):00167–3. doi: 10.1016/j.arcmed.2021.08.002
2. Pranata R, Henrina J, Raffaello WM, Lawrensia S, Huang I. Diabetes and Covid-19: The Past, the Present, and the Future. Metabolism (2021) 121:154814. doi: 10.1016/j.metabol.2021.154814
3. Kan C, Zhang Y, Han F, Xu Q, Ye T, Hou N, et al. Mortality Risk of Antidiabetic Agents for Type 2 Diabetes With Covid-19: A Systematic Review and Meta-Analysis. Front Endocrinol (2021) 12:708494. doi: 10.3389/fendo.2021.708494
4. Hariyanto TI, Intan D, Hananto JE, Putri C, Kurniawan A. Pre-Admission Glucagon-Like Peptide-1 Receptor Agonist (Glp-1ra) and Mortality From Coronavirus Disease 2019 (Covid-19): A Systematic Review, Meta-Analysis, and Meta-Regression. Diabetes Res Clin Pract (2021) 179:109031. doi: 10.1016/j.diabres.2021.109031
5. Stroup DF, Berlin JA, Morton SC, Olkin I, Williamson GD, Rennie D, et al. Meta-Analysis of Observational Studies in Epidemiology: A Proposal for Reporting. Meta-Analysis of Observational Studies in Epidemiology (Moose) Group. Jama (2000) 283(15):2008–12. doi: 10.1001/jama.283.15.2008
6. Stang A. Critical Evaluation of the Newcastle-Ottawa Scale for the Assessment of the Quality of Nonrandomized Studies in Meta-Analyses. Eur J Epidemiol (2010) 25(9):603–5. doi: 10.1007/s10654-010-9491-z
7. Zhou JB, Tang X, Han M, Yang J, Simó R. Impact of Antidiabetic Agents on Dementia Risk: A Bayesian Network Meta-Analysis. Metabolism (2020) 109:154265. doi: 10.1016/j.metabol.2020.154265
8. Bavishi C, Whelton PK, Mancia G, Corrao G, Messerli FH. Renin-Angiotensin-System Inhibitors and All-Cause Mortality in Patients With Covid-19: A Systematic Review and Meta-Analysis of Observational Studies. J Hypertens (2021) 39(4):784–94. doi: 10.1097/hjh.0000000000002784
9. Higgins JP, Thomas J, Chandler J, Cumpston M, Li T, Page MJ, et al. Cochrane Handbook for Systematic Reviews of Interventions. Chichester: John Wiley & Sons (2019). 264 p.
10. Dai M, Zhang F, Li K, Jin G, Chen Y, Zhang X. The Effect of Prophylactic Balloon Occlusion in Patients With Placenta Accreta Spectrum: A Bayesian Network Meta-Analysis. Eur Radiol (2022) 32(5):3297–308. doi: 10.1007/s00330-021-08423-6
11. Dias S, Welton NJ, Sutton AJ, Caldwell DM, Lu G, Ades A. Evidence Synthesis for Decision Making 4: Inconsistency in Networks of Evidence Based on Randomized Controlled Trials. Med Decision Making (2013) 33(5):641–56. doi: 10.1177/0272989X12455847
12. Silverii GA, Monami M, Cernigliaro A, Vigneri E, Guarnotta V, Scondotto S, et al. Are Diabetes and Its Medications Risk Factors for the Development of Covid-19? Data From a Population-Based Study in Sicily. Nutrition Metabolism Cardiovasc Dis NMCD (2021) 31(2):396–8. doi: 10.1016/j.numecd.2020.09.028
13. Kahkoska AR, Abrahamsen TJ, Alexander GC, Bennett TD, Chute CG, Haendel MA, et al. Association Between Glucagon-Like Peptide 1 Receptor Agonist and Sodium-Glucose Cotransporter 2 Inhibitor Use and Covid-19 Outcomes. Diabetes Care (2021) 44(7):1564–72. doi: 10.2337/dc21-0065
14. Ramos-Rincón JM, Pérez-Belmonte LM, Carrasco-Sánchez FJ, Jansen-Chaparro S, De-Sousa-Baena M, Bueno-Fonseca J, et al. Cardiometabolic Therapy and Mortality in Very Old Patients With Diabetes Hospitalized Due to Covid-19. journals gerontology Ser A Biol Sci Med Sci (2021) 76(8):e102–e9. doi: 10.1093/gerona/glab124
15. Orioli L, Servais T, Belkhir L, Laterre P-F, Thissen J-P, Vandeleene B, et al. Clinical Characteristics and Short-Term Prognosis of in-Patients With Diabetes and Covid-19: A Retrospective Study From an Academic Center in Belgium. Diabetes Metab syndrome (2021) 15(1):149–57. doi: 10.1016/j.dsx.2020.12.020
16. Israelsen SB, Pottegård A, Sandholdt H, Madsbad S, Thomsen RW, Benfield T. Comparable Covid-19 Outcomes With Current Use of Glp-1 Receptor Agonists, Dpp-4 Inhibitors or Sglt-2 Inhibitors Among Patients With Diabetes Who Tested Positive for Sars-Cov-2. Diabetes Obes Metab (2021) 23(6):1397–401. doi: 10.1111/dom.14329
17. Sourij H, Aziz F, Bräuer A, Ciardi C, Clodi M, Fasching P, et al. Covid-19 Fatality Prediction in People With Diabetes and Prediabetes Using a Simple Score Upon Hospital Admission. Diabetes Obes Metab (2021) 23(2):589–98. doi: 10.1111/dom.14256
18. Nyland JE, Raja-Khan NT, Bettermann K, Haouzi PA, Leslie DL, Kraschnewski JL, et al. Diabetes, Drug Treatment and Mortality in Covid-19: A Multinational Retrospective Cohort Study. Diabetes (2021) 70(12):2903–16. doi: 10.2337/db21-0385
19. Cheng X, Xin S, Chen Y, Li L, Chen W, Li W, et al. Effects of Metformin, Insulin on Covid-19 Patients With Pre-Existed Type 2 Diabetes: A Multicentral Retrospective Study. Life Sci (2021) 275:119371. doi: 10.1016/j.lfs.2021.119371
20. Elibol A, Eren D, Erdoğan MD, Elmaağaç M, Dizdar OS, Çelik İ, et al. Factors Influencing on Development of Covid-19 Pneumonia and Association With Oral Anti-Diabetic Drugs in Hospitalized Patients With Diabetes Mellitus. Primary Care Diabetes (2021) 15(5):806–12. doi: 10.1016/j.pcd.2021.08.001
21. Luk AOY, Yip TCF, Zhang X, Kong APS, Wong VW, Ma RCW, et al. Glucose-Lowering Drugs and Outcome From Covid-19 Among Patients With Type 2 Diabetes Mellitus: A Population-Wide Analysis in Hong Kong. BMJ Open (2021) 11(10):e052310. doi: 10.1136/bmjopen-2021-052310
22. Li J, Wei Q, McCowen KC, Xiong W, Liu J, Jiang W, et al. Inpatient Use of Metformin and Acarbose Is Associated With Reduced Mortality of Covid-19 Patients With Type 2 Diabetes Mellitus. Endocrinol Diabetes Metab (2022) 5(1):e00301. doi: 10.1002/edm2.301
23. Lally MA, Tsoukas P, Halladay CW, O'Neill E, Gravenstein S, Rudolph JL. Metformin Is Associated With Decreased 30-Day Mortality Among Nursing Home Residents Infected With Sars-Cov2. J Am Med Dir Assoc (2021) 22(1):193–8. doi: 10.1016/j.jamda.2020.10.031
24. Crouse AB, Grimes T, Li P, Might M, Ovalle F, Shalev A. Metformin Use Is Associated With Reduced Mortality in a Diverse Population With Covid-19 and Diabetes. Front Endocrinol (Lausanne) (2020) 11:600439. doi: 10.3389/fendo.2020.600439
25. Pérez-Belmonte LM, Torres-Peña JD, López-Carmona MD, Ayala-Gutiérrez MM, Fuentes-Jiménez F, Huerta LJ, et al. Mortality and Other Adverse Outcomes in Patients With Type 2 Diabetes Mellitus Admitted for Covid-19 in Association With Glucose-Lowering Drugs: A Nationwide Cohort Study. BMC Med (2020) 18(1):359. doi: 10.1186/s12916-020-01832-2
26. Wargny M, Potier L, Gourdy P, Pichelin M, Amadou C, Benhamou PY, et al. Predictors of Hospital Discharge and Mortality in Patients With Diabetes and Covid-19: Updated Results From the Nationwide Coronado Study. Diabetologia (2021) 64(4):778–94. doi: 10.1007/s00125-020-05351-w
27. Khunti K, Knighton P, Zaccardi F, Bakhai C, Barron E, Holman N, et al. Prescription of Glucose-Lowering Therapies and Risk of Covid-19 Mortality in People With Type 2 Diabetes: A Nationwide Observational Study in England. Lancet Diabetes Endocrinol (2021) 9(5):293–303. doi: 10.1016/s2213-8587(21)00050-4
28. Solerte SB, D'Addio F, Trevisan R, Lovati E, Rossi A, Pastore I, et al. Sitagliptin Treatment at the Time of Hospitalization Was Associated With Reduced Mortality in Patients With Type 2 Diabetes and Covid-19: A Multicenter, Case-Control, Retrospective, Observational Study. Diabetes Care (2020) 43(12):2999–3006. doi: 10.2337/dc20-1521
29. Meijer RI, Hoekstra T, van den Oever NCG, Simsek S, van den Bergh JP, Douma RA, et al. Treatment With a Dpp-4 Inhibitor at Time of Hospital Admission for Covid-19 Is Not Associated With Improved Clinical Outcomes: Data From the Covid-Predict Cohort Study in the Netherlands. J Diabetes Metab Disord (2021) 20(2)1–6. doi: 10.1007/s40200-021-00833-z
30. Cheng X, Liu YM, Li H, Zhang X, Lei F, Qin JJ, et al. Metformin Is Associated With Higher Incidence of Acidosis, But Not Mortality, in Individuals With Covid-19 and Pre-Existing Type 2 Diabetes. Cell Metab (2020) 32(4):537–47.e3. doi: 10.1016/j.cmet.2020.08.013
31. Luo P, Qiu L, Liu Y, Liu XL, Zheng JL, Xue HY, et al. Metformin Treatment Was Associated With Decreased Mortality in Covid-19 Patients With Diabetes in a Retrospective Analysis. Am J Trop Med Hygiene (2020) 103(1):69–72. doi: 10.4269/ajtmh.20-0375
32. Bramante CT, Buse J, Tamaritz L, Palacio A, Cohen K, Vojta D, et al. Outpatient Metformin Use Is Associated With Reduced Severity of Covid-19 Disease in Adults With Overweight or Obesity. J Med Virol (2021) 93(7):4273–9. doi: 10.1002/jmv.26873
33. Ghany R, Palacio A, Dawkins E, Chen G, McCarter D, Forbes E, et al. Metformin Is Associated With Lower Hospitalizations, Mortality and Severe Coronavirus Infection Among Elderly Medicare Minority Patients in 8 States in USA. Diabetes Metab Syndrome (2021) 15(2):513–8. doi: 10.1016/j.dsx.2021.02.022
34. Jiang N, Chen Z, Liu L, Yin X, Yang H, Tan X, et al. Association of Metformin With Mortality or Ards in Patients With Covid-19 and Type 2 Diabetes: A Retrospective Cohort Study. Diabetes Res Clin Pract (2021) 173:108619. doi: 10.1016/j.diabres.2020.108619
35. Lalau JD, Al-Salameh A, Hadjadj S, Goronflot T, Wiernsperger N, Pichelin M, et al. Metformin Use Is Associated With a Reduced Risk of Mortality in Patients With Diabetes Hospitalised for Covid-19. Diabetes Metab (2021) 47(5):101216. doi: 10.1016/j.diabet.2020.101216
36. Oh TK, Song IA. Metformin Use and Risk of Covid-19 Among Patients With Type Ii Diabetes Mellitus: An Nhis-Covid-19 Database Cohort Study. Acta Diabetol (2021) 58(6):771–8. doi: 10.1007/s00592-020-01666-7
37. Bramante CT, Ingraham NE, Murray TA, Marmor S, Hovertsen S, Gronski J, et al. Metformin and Risk of Mortality in Patients Hospitalised With Covid-19: A Retrospective Cohort Analysis. Lancet Healthy Longevity (2021) 2(1):e34–41. doi: 10.1016/s2666-7568(20)30033-7
38. Chen Y, Yang D, Cheng B, Chen J, Peng A, Yang C, et al. Clinical Characteristics and Outcomes of Patients With Diabetes and Covid-19 in Association With Glucose-Lowering Medication. Diabetes Care (2020) 43(7):1399–407. doi: 10.2337/dc20-0660
39. Do JY, Kim SW, Park JW, Cho KH, Kang SH. Is There an Association Between Metformin Use and Clinical Outcomes in Diabetes Patients With Covid-19? Diabetes Metab (2021) 47(4):101208. doi: 10.1016/j.diabet.2020.10.006
40. Gao Y, Liu T, Zhong W, Liu R, Zhou H, Huang W, et al. Risk of Metformin in Patients With Type 2 Diabetes With Covid-19: A Preliminary Retrospective Report. Clin Transl Sci (2020) 13(6):1055–9. doi: 10.1111/cts.12897
41. Kim MK, Jeon JH, Kim SW, Moon JS, Cho NH, Han E, et al. The Clinical Characteristics and Outcomes of Patients With Moderate-To-Severe Coronavirus Disease 2019 Infection and Diabetes in Daegu, South Korea. Diabetes Metab J (2020) 44(4):602–13. doi: 10.4093/dmj.2020.0146
42. Saygili ES, Karakiliç E, Mert E, Şener A, Mirci A. Preadmission Usage of Metformin and Mortality in Covid-19 Patients Including the Post-Discharge Period. Irish J Med Sci (2022) 191(2):1–7. doi: 10.1007/s11845-021-02823-9
43. Wander PL, Lowy E, Beste LA, Tulloch-Palomino L, Korpak A, Peterson AC, et al. Prior Glucose-Lowering Medication Use and 30-Day Outcomes Among 64,892 Veterans With Diabetes and Covid-19. Diabetes Care (2021) 44(12):2708–13. doi: 10.2337/dc21-1351
44. Tamura RE, Said SM, de Freitas LM, Rubio IGS. Outcome and Death Risk of Diabetes Patients With Covid-19 Receiving Pre-Hospital and in-Hospital Metformin Therapies. Diabetol Metab Syndrome (2021) 13(1):76. doi: 10.1186/s13098-021-00695-8
45. Dave JA, Tamuhla T, Tiffin N, Levitt NS, Ross IL, Toet W, et al. Risk Factors for Covid-19 Hospitalisation and Death in People Living With Diabetes: A Virtual Cohort Study From the Western Cape Province, South Africa. Diabetes Res Clin Pract (2021) 177:108925. doi: 10.1016/j.diabres.2021.108925
46. Cariou B, Hadjadj S, Wargny M, Pichelin M, Al-Salameh A, Allix I, et al. Phenotypic Characteristics and Prognosis of Inpatients With Covid-19 and Diabetes: The Coronado Study. Diabetologia (2020) 63(8):1500–15. doi: 10.1007/s00125-020-05180-x
47. Fadini GP, Morieri ML, Longato E, Bonora BM, Pinelli S, Selmin E, et al. Exposure to Dipeptidyl-Peptidase-4 Inhibitors and Covid-19 Among People With Type 2 Diabetes: A Case-Control Study. Diabetes Obes Metab (2020) 22(10):1946–50. doi: 10.1111/dom.14097
48. Rhee SY, Lee J, Nam H, Kyoung DS, Shin DW, Kim DJ. Effects of a Dpp-4 Inhibitor and Ras Blockade on Clinical Outcomes of Patients With Diabetes and Covid-19. Diabetes Metab J (2021) 45(2):251–9. doi: 10.4093/dmj.2020.0206
49. Dalan R, Ang LW, Tan WYT, Fong SW, Tay WC, Chan YH, et al. The Association of Hypertension and Diabetes Pharmacotherapy With Covid-19 Severity and Immune Signatures: An Observational Study. Eur Heart J Cardiovasc Pharmacother (2021) 7(3):e48–51. doi: 10.1093/ehjcvp/pvaa098
50. Noh Y, Oh IS, Jeong HE, Filion KB, Yu OHY, Shin JY. Association Between Dpp-4 Inhibitors and Covid-19-Related Outcomes Among Patients With Type 2 Diabetes. Diabetes Care (2021) 44(4):e64–e6. doi: 10.2337/dc20-1824
51. Roussel R, Darmon P, Pichelin M, Goronflot T, Abouleka Y, Ait Bachir L, et al. Use of Dipeptidyl Peptidase-4 Inhibitors and Prognosis of Covid-19 in Hospitalized Patients With Type 2 Diabetes: A Propensity Score Analysis From the Coronado Study. Diabetes Obes Metab (2021) 23(5):1162–72. doi: 10.1111/dom.14324
52. Zhou JH, Wu B, Wang WX, Lei F, Cheng X, Qin JJ, et al. No Significant Association Between Dipeptidyl Peptidase-4 Inhibitors and Adverse Outcomes of Covid-19. World J Clin Cases (2020) 8(22):5576–88. doi: 10.12998/wjcc.v8.i22.5576
53. Kosiborod MN, Esterline R, Furtado RHM, Oscarsson J, Gasparyan SB, Koch GG, et al. Dapagliflozin in Patients With Cardiometabolic Risk Factors Hospitalised With Covid-19 (Dare-19): A Randomised, Double-Blind, Placebo-Controlled, Phase 3 Trial. Lancet Diabetes Endocrinol (2021) 9(9):586–94. doi: 10.1016/s2213-8587(21)00180-7
54. Katsanos K, Spiliopoulos S, Karunanithy N, Krokidis M, Sabharwal T, Taylor P. Bayesian Network Meta-Analysis of Nitinol Stents, Covered Stents, Drug-Eluting Stents, and Drug-Coated Balloons in the Femoropopliteal Artery. J Vasc Surg (2014) 59(4):1123–33.e8. doi: 10.1016/j.jvs.2014.01.041
55. Zangiabadian M, Nejadghaderi SA, Zahmatkesh MM, Hajikhani B, Mirsaeidi M, Nasiri MJ. The Efficacy and Potential Mechanisms of Metformin in the Treatment of Covid-19 in the Diabetics: A Systematic Review. Front Endocrinol (Lausanne) (2021) 12:645194. doi: 10.3389/fendo.2021.645194
56. Bonora BM, Avogaro A, Fadini GP. Disentangling Conflicting Evidence on Dpp-4 Inhibitors and Outcomes of Covid-19: Narrative Review and Meta-Analysis. J Endocrinol Invest (2021) 44(7):1379–86. doi: 10.1007/s40618-021-01515-6
57. Dalan R. Metformin, Neutrophils and Covid-19 Infection. Diabetes Res Clin Pract (2020) 164:108230–. doi: 10.1016/j.diabres.2020.108230
58. Yang W, Sun X, Zhang J, Zhang K. The Effect of Metformin on Mortality and Severity in Covid-19 Patients With Diabetes Mellitus. Diabetes Res Clin Pract (2021) 178:108977–. doi: 10.1016/j.diabres.2021.108977
59. Yang Y, Cai Z, Zhang J. Dpp-4 Inhibitors May Improve the Mortality of Coronavirus Disease 2019: A Meta-Analysis. PloS One (2021) 16(5):e0251916. doi: 10.1371/journal.pone.0251916
60. Rakhmat II, Kusmala YY, Handayani DR, Juliastuti H, Nawangsih EN, Wibowo A, et al. Dipeptidyl Peptidase-4 (Dpp-4) Inhibitor and Mortality in Coronavirus Disease 2019 (Covid-19) - a Systematic Review, Meta-Analysis, and Meta-Regression. Diabetes Metab Syndrome (2021) 15(3):777–82. doi: 10.1016/j.dsx.2021.03.027
61. Patoulias D, Doumas M. Dipeptidyl Peptidase-4 Inhibitors and Covid-19-Related Deaths Among Patients With Type 2 Diabetes Mellitus: A Meta-Analysis of Observational Studies. Endocrinol Metab (Seoul Korea) (2021) 36(4):904–8. doi: 10.3803/EnM.2021.1048
62. Liu X, Mei T, Chen W, Ye S. Comparison of Antidiabetic Medications During the Treatment of Atherosclerosis in T2dm Patients. Mediat Inflamm (2017) 2017:5032708. doi: 10.1155/2017/5032708
63. Nomoto H, Kimachi K, Miyoshi H, Kameda H, Cho KY, Nakamura A, et al. Effects of 50 Mg Vildagliptin Twice Daily Vs. 50 Mg Sitagliptin Once Daily on Blood Glucose Fluctuations Evaluated by Long-Term Self-Monitoring of Blood Glucose. Endocr J (2017) 64(4):417–24. doi: 10.1507/endocrj.EJ16-0546
64. Fadini GP, Albiero M, Avogaro A. Direct Effects of Dpp-4 Inhibition on the Vasculature. Reconciling Basic Evidence With Lack of Clinical Evidence. Vascul Pharmacol (2015) 73:1–3. doi: 10.1016/j.vph.2015.08.004
65. Lim S, Bae JH, Kwon H-S, Nauck MA. Covid-19 and Diabetes Mellitus: From Pathophysiology to Clinical Management. Nat Rev Endocrinol (2021) 17(1):11–30. doi: 10.1038/s41574-020-00435-4
66. Yang Y, Cai Z, Zhang J. Insulin Treatment May Increase Adverse Outcomes in Patients With Covid-19 and Diabetes: A Systematic Review and Meta-Analysis. Front Endocrinol (Lausanne) (2021) 12:696087. doi: 10.3389/fendo.2021.696087
67. Koufakis T, Karras SN, Zebekakis P, Ajjan R, Kotsa K. Should the Last Be First? Questions and Dilemmas Regarding Early Short-Term Insulin Treatment in Type 2 Diabetes Mellitus. Expert Opin Biol Ther (2018) 18(11):1113–21. doi: 10.1080/14712598.2018.1526278
68. Schaschkow A, Mura C, Dal S, Langlois A, Seyfritz E, Sookhareea C, et al. Impact of the Type of Continuous Insulin Administration on Metabolism in a Diabetic Rat Model. J Diabetes Res (2016) 2016:8310516. doi: 10.1155/2016/8310516
69. Filgueiras LR, Capelozzi VL, Martins JO, Jancar S. Sepsis-Induced Lung Inflammation Is Modulated by Insulin. BMC Pulm Med (2014) 14:177. doi: 10.1186/1471-2466-14-177
70. Aroda VR. A Review of Glp-1 Receptor Agonists: Evolution and Advancement, Through the Lens of Randomised Controlled Trials. Diabetes Obes Metab (2018) 20 Suppl 1:22–33. doi: 10.1111/dom.13162
71. Rogliani P, Matera MG, Calzetta L, Hanania NA, Page C, Rossi I, et al. Long-Term Observational Study on the Impact of Glp-1r Agonists on Lung Function in Diabetic Patients. Respir Med (2019) 154:86–92. doi: 10.1016/j.rmed.2019.06.015
72. Koufakis T, Pavlidis AN, Metallidis S, Kotsa K. Sodium-Glucose Co-Transporter 2 Inhibitors in Covid-19: Meeting at the Crossroads Between Heart, Diabetes and Infectious Diseases. Int J Clin Pharm (2021) 43(3):764–7. doi: 10.1007/s11096-021-01256-9
Keywords: diabetes, antidiabetic agents, COVID-19, mortality, Bayesian network meta-analysis
Citation: Chen Y, Lv X, Lin S, Arshad M and Dai M (2022) The Association Between Antidiabetic Agents and Clinical Outcomes of COVID-19 Patients With Diabetes: A Bayesian Network Meta-Analysis. Front. Endocrinol. 13:895458. doi: 10.3389/fendo.2022.895458
Received: 13 March 2022; Accepted: 19 April 2022;
Published: 27 May 2022.
Edited by:
Mohamed Abu-Farha, Dasman Diabetes Institute, KuwaitReviewed by:
Theocharis Koufakis, University General Hospital of Thessaloniki AHEPA, GreeceJuan Zheng, Tongji Medical College, Huazhong University of Science and Technology, China
Copyright © 2022 Chen, Lv, Lin, Arshad and Dai. This is an open-access article distributed under the terms of the Creative Commons Attribution License (CC BY). The use, distribution or reproduction in other forums is permitted, provided the original author(s) and the copyright owner(s) are credited and that the original publication in this journal is cited, in accordance with accepted academic practice. No use, distribution or reproduction is permitted which does not comply with these terms.
*Correspondence: Mengjun Dai, ZGFpbWVuZ2p1bkBzanR1LmVkdS5jbg==