- 1Graduate School, Beijing University of Chinese Medicine, Beijing, China
- 2International Medical Services, China-Japan Friendship Hospital, Beijing, China
- 3Department of Pediatrics, China-Japan Friendship Hospital, Beijing, China
- 4Institute of Clinical Medical Sciences, China-Japan Friendship Hospital, Beijing, China
Background and Objectives: As the worldwide secular trends are toward earlier puberty, identification of contributing factors for precocious puberty is critical. We aimed to identify and optimize contributing factors responsible for onset of precocious puberty via machine learning/deep learning algorithms in girls.
Methods: A cross-sectional study was performed among girls aged 6-16 years from 26 schools in Beijing based on a cluster sampling method. Information was gleaned online via questionnaires. Machine/deep learning algorithms were performed using Python language (v3.7.6) on PyCharm platform.
Results: Of 11308 students enrolled, there are 5527 girls, and 408 of them had experienced precocious puberty. Training 13 machine learning algorithms revealed that gradient boosting machine (GBM) performed best in predicting precocious puberty. By comparison, six top factors including maternal age at menarche, paternal body mass index (BMI), waist-to-height ratio, maternal BMI, screen time, and physical activity were sufficient in prediction performance, with accuracy of 0.9530, precision of 0.9818, and area under the receiver operating characteristic curve (AUROC) of 0.7861. The performance of the top six factors was further validated by deep learning sequential model, with accuracy reaching 92.9%.
Conclusions: We identified six important factors from both parents and girls that can help predict the onset of precocious puberty among Chinese girls.
Introduction
The incidence and prevalence of precocious puberty are rising rapidly during recent decades, and differ greatly between genders around the global (1, 2). In Denmark, there was a 33-fold increase in annual incidence of premature thelarche from 1998 at 0.07 per 10,000 girls to 2007 at 2.24 per 10 000 girls, and contrastingly in boys a 15-fold increase in diagnosis of central precocious puberty was seen during this period (from 0.1 per 10 000 to 2.1 per 10 000) (3). In a separate survey from China, the adjusted prevalence of precocious puberty was estimated to be 6.19% (girls: 11.47% and boys: 3.26%) in 2019 (2). One major reason behind these alarming numbers is the worldwide secular trends toward earlier puberty. Evidence from a comprehensive meta-analysis showed that age at thelarche decreased 3 months per decade from 1977 to 2013 (4). With a great improvement in living standards and nutritional status in school-aged children, the issue of precocious puberty has received scientific attention, and we must expand this attention to develop preventive strategies to curb this global issue.
Observational studies focusing on the risk profiles of precocious puberty in school-aged children are very limited in the literature (5–8). For instance, obesity and central obesity in children were identified as promising risk factors for the development of earlier pubertal (8). In a separate study, vitamin D deficiency was associated with over 3-fold increased risk of precocious puberty in girls (6). The development of precocious puberty is a complex process, and its manifestations often involve concurrence of multiple factors, with each factor bearing a small contribution. In this context, identifying potential contributing factors and characterizing their internal relationship represents a practical strategy to inform parents and public health practitioners to delay or prevent early onset of precocious puberty. To this end, predictive models or tools are recommended to risk-stratify precocious puberty among children. However, the majority of published models or tools that are developed by traditional statistical methods are based on the “relative independence” assumption of different factors. In most cases, this assumption is untenable, as predictive factors usually act in a synergistic – instead of independent – manner, leading to biased estimation between exposures and outcomes.
To solve this methodological issue and yield more information for future studies, we identified and optimized the panel of contributing factors susceptible to onset of precocious puberty in Chinese girls aged 6-16 years by using the widely-used machine learning and deep learning algorithms. To facilitate practical application, a predictive tool using the minimal number of contributing factors and the optimal learning algorithm was proposed.
Methods
Study Design
This study follows the rationales of a cross-sectional design based on a stratified cluster random sampling strategy. Online survey was conducted at a suburb district (Pinggu) of Beijing during the first month of 2022. The design and implementation of this survey, in accordance with the principles of the Declaration of Helsinki, was approved the Ethics Committees of Beijing University of Chinese Medicine. The parents and supervisors of each assessable students gave informed consent prior to participation in this survey.
Study Participants
Students from total 26 schools, which were randomly selected from Pinggu district, formed study participants of this survey. Out of 26 schools, 8 were primary schools, and 18 were middle schools. All students were initially deemed eligible for inclusion if they were not diagnosed or reported to experience severe endocrine disorders, which included but not limited to hyperthyroidism, hypothyroidism and diabetes mellitus.
Questionnaire
An electronic questionnaire was designed, and it was a priori tested to be appropriate and valid based on the reliability coefficient α exceeding 0.85. For the sake of convenience, questionnaire was generated in the form of QR code that can be easily recognized by common smart phones on the market by the “Wenjuanxing” (available at the website https://www.wenjuan.com), an online crowdsourcing platform in mainland China.
Data collection
This valid questionnaire was distributed by school teachers in charge to the parents or supervisors of each student to collect data from both students and their parents, as well as their grandparents and grandparents-in-law.
In total, parents or supervisors of 11633 students received this questionnaire, and 11308 of them responded. Given the fact that the prevalence of precocious puberty differs by gender, only 87 of 5781 boys (1.5%) were found to experience precocious puberty in this study population. To derive a reliable estimate, the present analysis was restricted to girls only. There were 5567 questionnaires filled by the parents or supervisors of girls. After excluding 40 questionnaires with missing important information, data from 5527 valid questionnaires were analyzed finally.
Quality Control
Because data in this survey were gleaned from online questionnaires, it is essential to appraise the quality. Before circulating our questionnaires, each item was explained in details to teachers in charge by trained staff. The questionnaire was circulated by school teachers to the parents or supervisors of students under survey. In the case of any uncertainty when filling in the questionnaire, trained staff were contacted by teachers for confirmation. Labeled data were downloaded from the “Wenjuanxing” platform into the Microsoft Office Excel™ file. All data were double checked by trained staff to ensure accuracy. In the case of missing data or data with extreme values, teachers in charge were contacted by re-inviting the parents or Supervisors to provide or validate these data.
Survey Data From Students
Data on birth year and month, sex, nationality, birth weight, birth body length, gestational week, time spent on physical activity and screen, daily sitting time, time to night sleep, sleep duration and eating speed were collected from students under study. In addition, dietary preferences including frequency of eating fiber-rich, out-of-season, meat, plant protein, tonic or sweet foods, snacks, milk products, foods with preservatives or fast foods were recorded. Lifestyle habits that may affect puberty onset were also recorded, including consuming night meal, sleeping with the lights on, monophagia, frequent use of plastic tableware, and exposures to adult cosmetics. Body height (to the nearest 0.1 cm), body weight (to the nearest 0.1 kg), and waist circumference (to the nearest 0.1 cm) were measured by trained physicians. Waist circumference was measured at 2 cm above the umbilicus.
Survey Data From Parents and Grandparents
Data collected from parents and grandparents included birth year, sex, body height and weight, education, family income, maternal age at menarche, baring age of both parents, pregnancy order, delivery order, history of using assisted reproductive technology, delivery mode, breastfeeding duration, infancy feeding ways, and age of adding complementary food. The number of two parents and four grandparent who were diagnosed to have hypertension or diabetes mellitus was summed meanwhile.
Precocious Puberty Definition
Precocious puberty was defined according to the guidelines recommended by the Chinese Medical Association Pediatrics Branch in 2015 (9) and the Ministry of Health of the People’s Republic of China in 2011 (10). Girls with breast development before 8 years of age and menarche before 10 years of age are considered to experience precocious puberty.
As this survey was undertaken in schools and due to the strict prevention and control for COVID-19 in China, it is difficult to examine the physical status of students on spot. Self-observation and visual assessment methods suitable for survey were adopted to determine whether girls had precocious puberty (11). In detail, the standards for breast development are that the breasts are hill-shaped and palpated with nodules inside. To reduce or avoid information bias, the methods were explained in detail by trained staff to teachers in charge, as well as the parents and grandparents of each girl in the survey.
Definitions of Survey Data
Body mass index (BMI) was calculated as follows: weight (kg)/height (m2). Waist-to-height ratio (WHtR) was calculated as waist (cm) divided by height (cm). Central obesity was defined as WHtR ≥0.47 in girls (12). Duration of sleeping, physical activity, sitting, and screening was recorded in hours and separately calculated as the sum of time on working days × 5 and time on weekends × 2 divided by 7. Eating speed was recorded in minutes and calculated as the average time of breakfast, lunch and supper within a day. All types of dietary preferences and habits that may affect puberty onset were categorized into 4 groups: every day, 3-5 times weekly, 1-2 times weekly and none or occasionally. Fiber-rich foods referred to grains, seasonal vegetables and fruits. Night meal was defined as eating foods within 2 hours before bedtime. Screen time was defined as sum of time spent on watching television, using computers and mobile phones, and the other kinds of electronic devices with screens.
Maternal BMI and paternal BMI were calculated from self-reported weight and height. Education was classified into Bachelor’s (or equivalent) degree or above, high (or equivalent) school degree, and junior high school degree or below. Household income was categorized as <100,000, 100,000-300,000 and >300,000 RMB per year. Delivery mode included natural labor and cesarean section. Breastfeeding duration and age of adding complementary foods were recorded in months. Infancy feeding ways included exclusive breastfeeding, mixed feeding, and artificial feeding.
Statistical Analyses
Data were analyzed using the PyCharm (Edition 2018.1 x64) embedded by the Python (Python Software Foundation) software (Version 3.7.6) under the Windows 10 system. Variables were retained for analysis if the percentage of missing rows is less than 30%. Missing data were filled in by multiple imputation method using the “mice” package in the R environment (Version 4.1.1).
As this survey was performed at 26 schools, the degree of difference between schools was appraised by the intraclass correlation coefficient (ICC) (13). The ICC statistic ranges from zero to unity, and if this statistic equals to zero, the variance in questionnaire is not due to variation between schools.
All study girls were grouped according to the absence or presence of precocious puberty features. Descriptive statistics were expressed as mean (standard deviation) or median (interquartile range) for continuous variables, and as number (percent) for categorical variables. Deviation from normal distribution was tested from skewness and kurtosis statistics. Differences in these variables between girls with and without precocious puberty were quantified by using the t test, rank-sum test or χ2, when appropriate. Two-sided probability was deemed statistically significant if it is less than 0.05.
All valid variables were fed to machine learning algorithms. To minimize overfitting, data from 5527 girls were randomized into the training set and the validation set at a ratio of 6:4. Due to the low ratio of precocious puberty, the purpose of this classification choice is to ensure that there are sufficient number of cases with precocious puberty in both training and validation sets.
In this study, besides the traditional logistic regression algorithm, additional 12 different machine learning algorithms were employed, including decision tree, Adaboost decision tree, support vector machine (SVM), random forest, K-nearest neighbor (KNN), gradient boosting machine (GBM), extreme gradient boosting (Xgbc), light gradient boosting machine (LGBM), Gaussian naive Bayes (gNB), multinomial naive Bayes (mNB), Bernoulli naive Bayes (bMB) and multi-layer perceptron (MLP). The machine learning algorithms performed supervised learning in given data, adjusting for the magnitude and direction of model error to improve prediction accuracy. Data from the training set were first fed to each machine learning algorithm, and data from the validation set were used to test prediction performance of these algorithms. Prediction performance was assessed from five diverse aspects, namely, accuracy, precision, recall, F1 score, and the area under the receiver operating characteristic curve (AUROC). Based on the five aspects, the optimal machine learning algorithm was selected. By definition, accuracy refers to the rate of correct prediction, and precision measures the ability to target actual positive observations. Recall reflects the capability to predict actual positivity correctly. F1 score, calculated as the harmonic mean between precision and recall, takes both false positives and false negatives into account. AUROC is proposed as a summarized accuracy index, with a higher value indicating a higher probability of having the characteristic under study.
With the use of optimal machine learning algorithm, the minimum number of top variables were determined by prediction capability. The prediction capability of cumulative variables was reflected by AUROC, accuracy, and precision, upon which, the top variables was ranked in an ascending order. For clinical application, the minimal number of top variables that can achieve maximum predictive performance was ascertained. Performance of this minimal number of top variables in predicting onset of precocious puberty in girls was further appraised by accuracy and loss using the deep learning sequential model. For comparison, deep learning sequential model was performed using 3 different optimizers [adaptive moment estimation (Adam), root mean square prop (RMSprop), and stochastic gradient descent (SGD)].
To reflect the degree of prediction directly, the top variables identified above were incorporated into the Logistic regression model. The effect-size estimates are expressed as odds ratio (OR) with 95% confidence interval (CI).
Results
Between-School Variation Appraisal
The ICC statistic for all items in our questionnaire was extremely low (less than 0.044), meaning that there was low likelihood of clustering within schools, as well as low likelihood of difference in items between schools.
Baseline Characteristics
Table 1 shows the distributions and comparisons of 5527 girls (mean age: 10.88 years, range: 6-16 years) stratified by the presence and absence of precocious puberty. 408 girls were defined to experience precocious puberty, with the prevalence rate of 7.4% in this girl population.
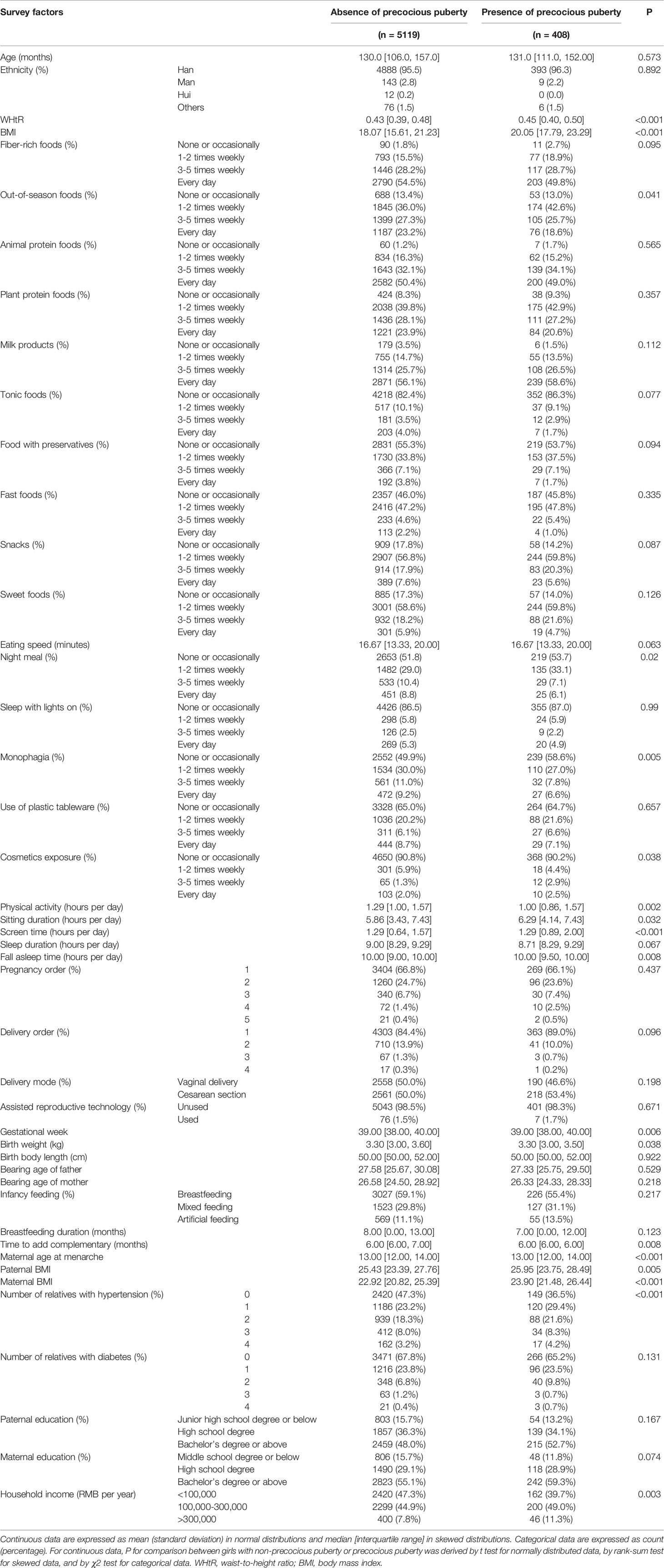
Table 1 The baseline characteristics of school girls stratified by the presence of precocious puberty.
Selecting the Optimal Machine Learning Algorithm
Figure 1 shows the prediction accuracy of 13 different machine learning algorithms evaluated in this study. Table 2 compares the performance of these algorithms from five aspects. After comparison, the algorithm GBM performed the best, with the AUROC of 0.784 (Figure 2), the accuracy of 0.952, the precision of 0.932, the recall of 0.350, and the F1 score of 0.509. The prediction model for precocious puberty was therefore constructed using the GBM algorithm.
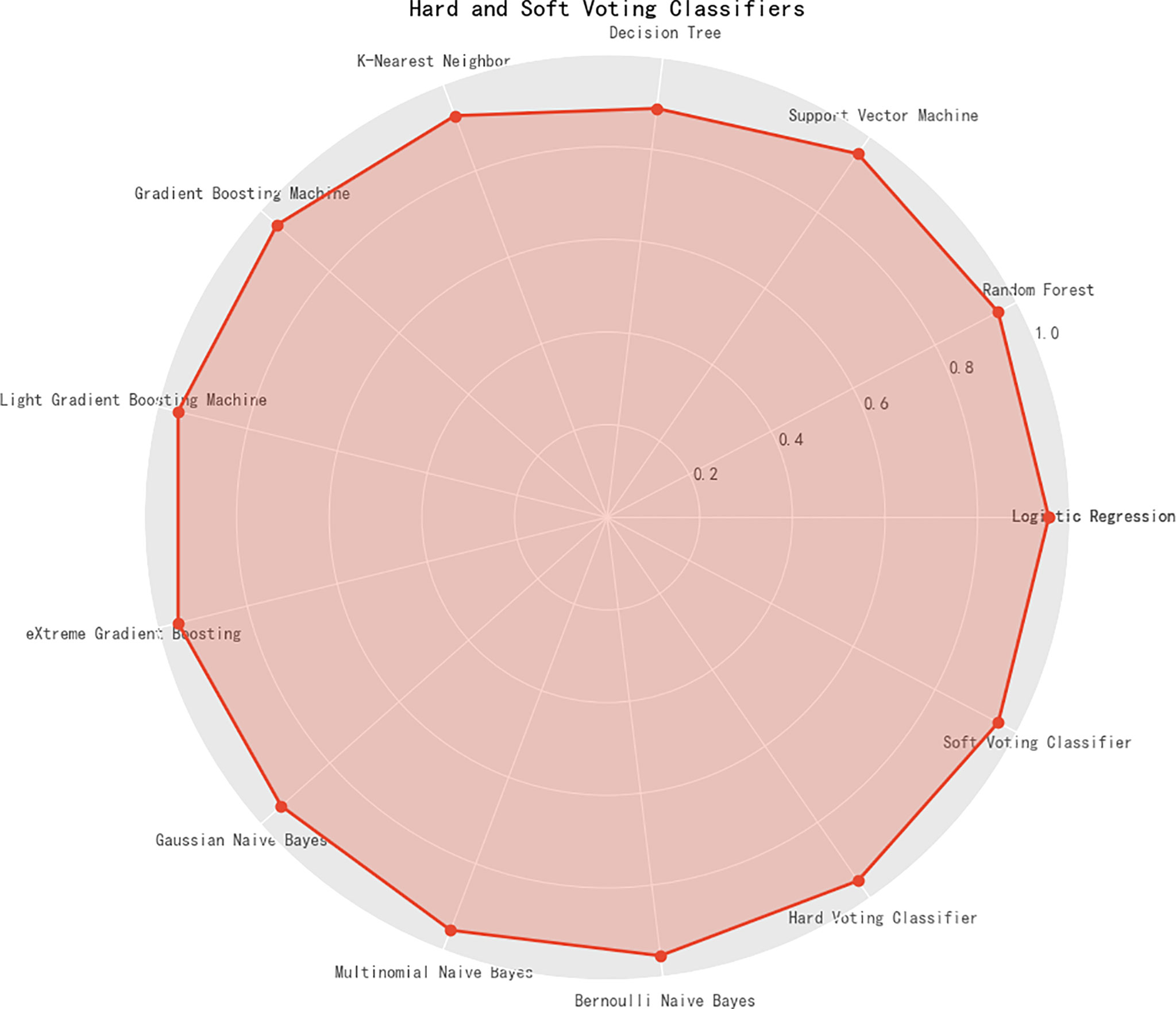
Figure 1 The prediction accuracy of 13 machine learning algorithms, along with hard and soft voting classifiers.
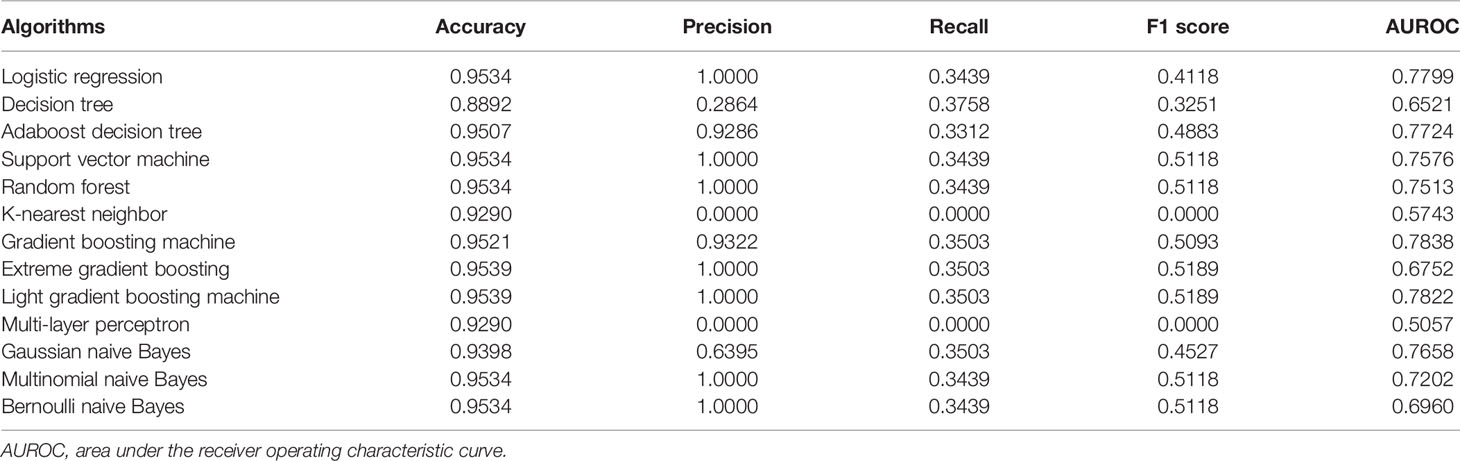
Table 2 The prediction performance of 13 machine learning algorithms from accuracy, precision, recall, F1 score and AUROC aspects for precocious puberty.
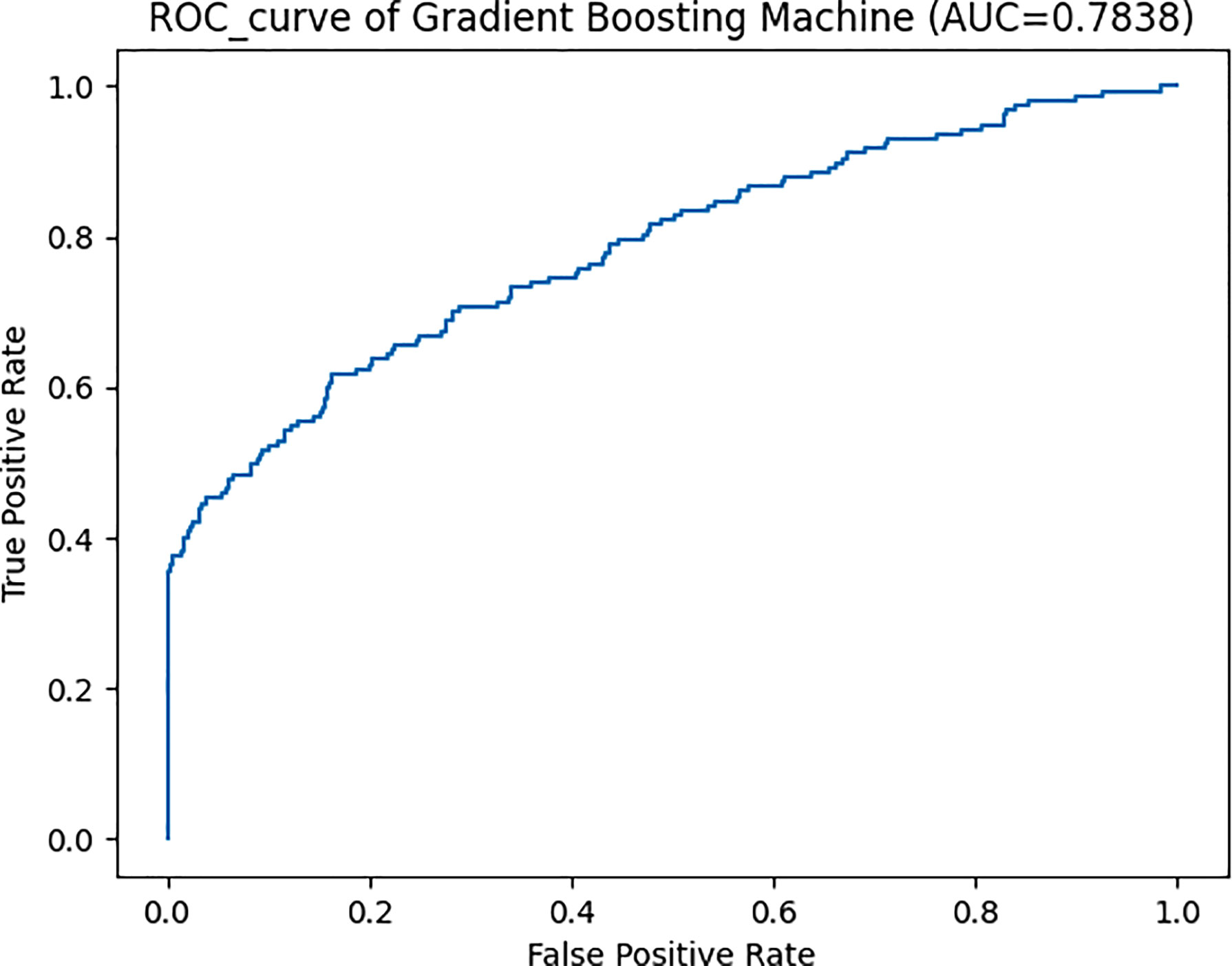
Figure 2 The area under the receiver operating characteristic curve (AUROC) of gradient boosting machine algorithm for the prediction of precocious puberty. AUC, area under the receiver operating characteristic curve; ROC, receiver operating characteristic curve.
Selecting the Minimal Number of Contributors
The importance of each variable under study in predicting precocious puberty was estimated and ranked in an ascending order, and that of the top 20 variables is illustrated in Figure 3. The cumulative contribution of top 10 variables is assessed in Table 3, and by comparison the prediction capability of top six variables was comparable with that of all 45 variables under study (AUROC: 0.7861; accuracy: 0.9534; precision: 1.0000). The six variables included maternal age at menarche, paternal BMI, WHtR, maternal BMI, screen time, and physical activity.
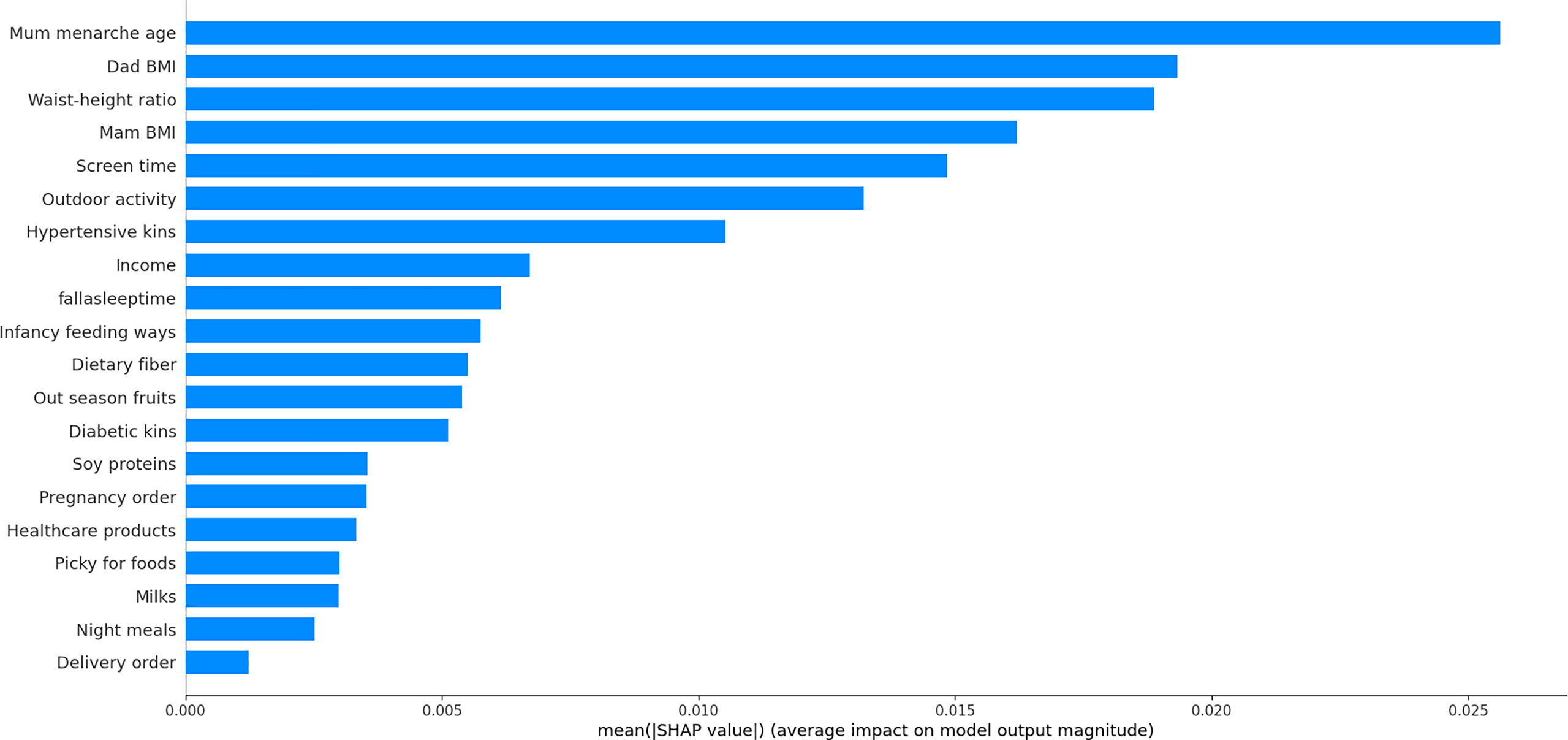
Figure 3 Top 20 factors for predicting precocious puberty in a descending order of importance. BMI, body mass index.
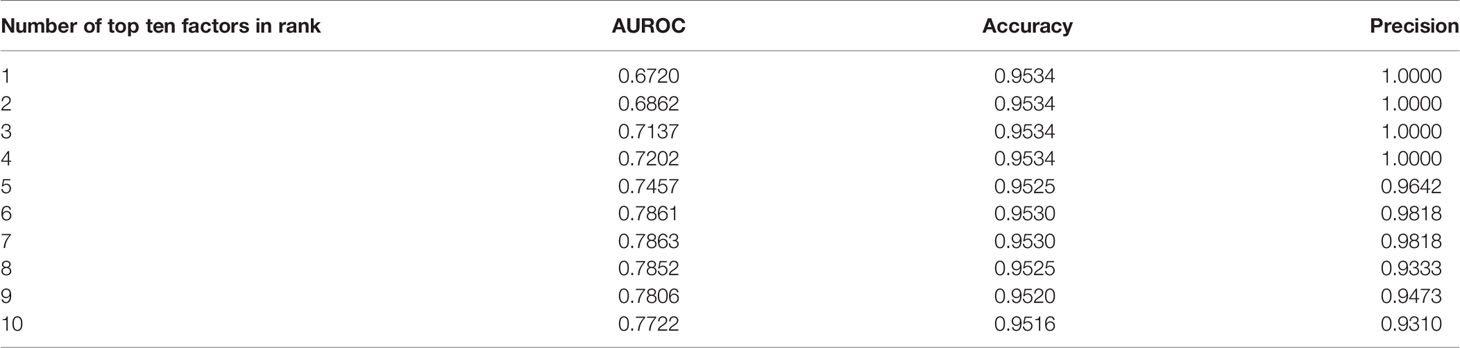
Table 3 The areas under the receiver operating curve (AUROC), accuracy and precision with the cumulating number of top ten factors in an ascending order.
Performance Assessment Using Deep Learning Model
As presented in Table 4, the prediction performance was compared between top 6 variables and all study variables under the deep learning sequential model. By using three optimizers, the performance as assessed by model accuracy and model loss was comparable between the two sets of variables (for accuracy: 94.12% using all variables and 92.90% using top six variables; for loss: 26.05% using all variables and 25.66% using top six variables in the validation set), which further reinforced the prediction capability of the top six variables selected by machine learning algorithms.
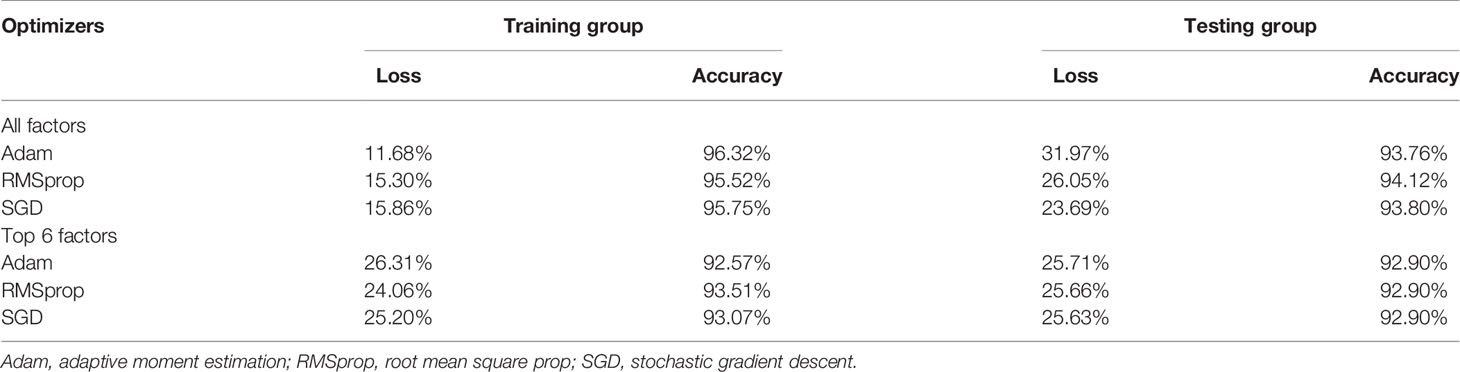
Table 4 Model loss and accuracy of deep learning sequential model in both training and testing groups.
Risk Estimates of the Top 6 Variables Using Logistic Regression Model
The top 6 variables identified above was incorporated into the Logistic regression model to estimate the effect-size of prediction (Table 5). Consistent with the above findings, the top 6 variables were significantly associated with the onset of precocious puberty.

Table 5 The risk prediction of top 6 variables for precocious puberty using the Logistic regression model.
Discussion
This is a cross-sectional, large survey in school girls aged 6-16 years from Beijing to identify and optimize contributing factors susceptible to precocious puberty. The key findings of this study are the contribution of six top factors from both parents and girls under the optimal GBM algorithm to the prediction of precocious puberty, which can not only help inform parents to prevent or postpone the earlier puberty of their daughters, but also suggest healthcare professionals to launch education reforms, such as weight control and outdoor activity recommendation. Importantly, the incorporation of the six top factors in GBM algorithm can be developed as a tool to facilitate the better identification of school girls who are at high risk for the future experience of precocious puberty and might benefit from early lifestyle or medical interventions. To the best of our knowledge, this is thus far the first attempt of using machine learning and deep learning techniques to characterize the risk profiles of precocious puberty in school girls.
Recently, the problem of precocious puberty constitutes a substantial health burden around the global (14). Several studies have shown that the incidence of precocious puberty have increased significantly and there are worldwide secular trends toward earlier puberty (2, 3, 11). Some studies have shown that early puberty is associated with cognitive function decline in children with Cushing disease (15). Another study using Mendelian randomization method provided evidence for the causal detrimental impact of early puberty on asthma (16). In a separate population study, early onset of breast development was found to be associated with high risk of the presence of depression (17). In addition, animal studies indicated puberty as an important developmental period for the establishment of adipose tissue mass and metabolic homeostasis (18). Given the high burden of precocious puberty, it is imperative to gain an understanding of its risk profiles for informing future gender-specific public health recommendations.
There is evidence that the development of precocious puberty is a multistep, multifactorial progress, to which inherited, environmental, and lifestyle-related factors contribute together (11). In most cases, the contribution of each factor is dependent on the presence of other factors, and this dependence usually follows a nonlinear manner. Despite there are good reasons to adopt traditional statistical approaches such as logistic regression, they do have limited power for modeling high-order non-linear patterns of interaction. Hence, success in characterize the risk profiles of precocious puberty will hinge on our ability to address nonlinearities and interactions between exposures and outcomes. To shed some light, besides the traditional logistic regression, we also employed 12 additional machine learning algorithms to compare their prediction performance from various aspects. These machine learning algorithms have shown great promise in application to many areas in clinical practice and health care, such as assisted disease diagnosis, clinical outcome prediction and automated image interpretation (19–22). By comparison, we found the prediction performance of GBM algorithm is best, and this algorithm focuses on improved prediction by combining information from many variables that individually may not be significant but together are very informative; of less concern is the functional form of any one variable (23). The GBM algorithm builds regression trees on all factors in parallel to take adequate utilization of all variables. On the other hand, the GBM algorithm might have the risk of overfitting. The possibility of overfitting can be checked through the accuracy of prediction in the validation set. What’s more, the six top factors, including age at menarche, paternal BMI, WHtR, maternal BMI, screen time, and physical activity were teased out from 45 potential factors in this study and their prediction performance was not inferior to all factors involved. The most important factor identified in this study, age at menarche, was supported by other studies (24, 25).
Besides, WHtR was found to be associated with the significant risk of precocious puberty in this study, consistent with the findings of previous studies (8). Relative to BMI, WHtR is an indicator of central obesity and is less correlated with age (12, 26). There is evidence that excessive visceral fat accumulation, manifested as central obesity, can impair insulin sensitivity and even result in insulin resistance (27), and reduced insulin sensitivity interferes with leptin signaling, which plays in important role in puberty initiation (28). Our findings highlight the suitability of monitoring WHtR among school girls in predicting the risk of precocious puberty. Another aspect that merits discussion is the contributory role of parental obesity in predisposition to precocious puberty in offspring, which was consolidated by other studies (29).
Moreover, it is increasingly recognized that physical activity and screen time are two factors precipitating the onset of puberty. As stated by a systematic review, menarche of athletes with high-intensity physical activity delayed 1.13 years compared to non-athlete girls (30). In a separate study, aerobic exercise was reported to delay the progression of puberty in students with central precocious puberty by increasing adiponectin levels (31). On the other hand, screen time may alter eating habits (32), and advertisements through screens would influence dietary choices (33). Notably, the incidence of precocious puberty increased significantly during the COVID-19 lockdown due to the prolonged usage of electronic devices (34). There is evidence that prolonged screen time can reduce insulin sensitivity and lead to high fasting insulin levels in girls (35). We agree that further validations of our findings in other independent groups are necessary to confirm the implication of six factors identified in the development of precocious puberty in school girls.
It is also worth noting that the contribution of these six factors to precocious puberty is not independent, and they might act in a synergistic manner. Although the GBM was selected as the best algorithm in predicting precocious puberty, the modeling process is less transparent and is harder to interpret, restricting clinical understanding and implementation in practice. As such, a high standard of model transparency is required to allow parents and students, as well as healthcare professionals to make informed decisions.
Limitations
Several limitations should be acknowledged for the present study. Firstly, the cross-sectional nature of this survey limited further interpretation of possible causality between important factors identified and precocious puberty. Secondly, despite much efforts are made to ensure data quality, information bias cannot be fully ruled out in our questionnaires. Thirdly, puberty is a complex biological process of sexual progression affected by a dynamic panel of risk factors, and incorporation of more lifestyle-relevant factors of students is of added interest. Fourthly, all students enrolled are school-aged girls living in Beijing, and extrapolation of our findings to other populations should be made with caution. Fifthly, by means of questionnaire, it is only possible to appraise the presence of precocious puberty, rather than to distinguish the types of precocious puberty, such as central precocious puberty. We agree that further studies are warranted to identify and optimize the contributing factors susceptible to the onset of central precocious puberty.
Conclusions
Via a comprehensive analysis, we have identified six important factors from both parents and students under the optimal GBM algorithm that can help predict the risk of precocious puberty in Chinese girls. Further explorations on other potential factors, especially for school boys and possible molecular mechanisms of precocious puberty are encouraging and essential.
Data Availability Statement
The raw data supporting the conclusions of this article will be made available by the authors, without undue reservation.
Ethics Statement
The studies involving human participants were reviewed and approved by Ethics Committees of Beijing University of Chinese Medicine. Written informed consent to participate in this study was provided by the participants’ legal guardian/next of kin.
Author Contributions
ZZ planned and designed the study, as well as directed its implementation. ZZ and WN drafted the protocol. QW, BP, MY, MX, YZ, and XD obtained statutory and ethics approvals. QW and BP contributed to data acquisition. BP and WN conducted statistical analyses. QW, BP, MY and MX did the data preparation and quality control. BP and WN wrote the manuscript. All authors read and approved the final manuscript prior to submission. All authors contributed to the article and approved the submitted version.
Conflict of Interest
The authors declare that the research was conducted in the absence of any commercial or financial relationships that could be construed as a potential conflict of interest.
Publisher’s Note
All claims expressed in this article are solely those of the authors and do not necessarily represent those of their affiliated organizations, or those of the publisher, the editors and the reviewers. Any product that may be evaluated in this article, or claim that may be made by its manufacturer, is not guaranteed or endorsed by the publisher.
Acknowledgments
We are grateful to all participating students, and their parents or guardians for their kind cooperation and willingness, as well as school teachers in charge, and health physicians for their generous help. Without their help, the conduct of this survey would not be possible.
References
1. Phillip M, Lazar L. Precocious Puberty: Growth and Genetics. Horm Res (2005) 64(Suppl 2):56–61. doi: 10.1159/000087760
2. Liu Y, Yu T, Li X, Pan D, Lai X, Chen Y, et al. Prevalence of Precocious Puberty Among Chinese Children: A School Population-Based Study. Endocrine (2021) 72(2):573–81. doi: 10.1007/s12020-021-02630-3
3. Brauner EV, Busch AS, Eckert-Lind C, Koch T, Hickey M, Juul A. Trends in the Incidence of Central Precocious Puberty and Normal Variant Puberty Among Children in Denmark, 1998 to 2017. JAMA Netw Open (2020) 3(10):e2015665. doi: 10.1001/jamanetworkopen.2020.15665
4. Eckert-Lind C, Busch AS, Petersen JH, Biro FM, Butler G, Brauner EV, et al. Worldwide Secular Trends in Age at Pubertal Onset Assessed by Breast Development Among Girls: A Systematic Review and Meta-Analysis. JAMA Pediatr (2020) 174(4):e195881. doi: 10.1001/jamapediatrics.2019.5881
5. Kim Y, Lee NK, Kim JH, Ku JK, Lee BK, Jung HI, et al. Association of Maxillary Dental Developmental Abnormality With Precocious Puberty: A Case-Control Study. Maxillofac Plast Reconstr Surg (2020) 42(1):30. doi: 10.1186/s40902-020-00274-3
6. Lee HS, Kim YJ, Shim YS, Jeong HR, Kwon E, Hwang JS. Associations Between Serum Vitamin D Levels and Precocious Puberty in Girls. Ann Pediatr Endocrinol Metab (2014) 19(2):91–5. doi: 10.6065/apem.2014.19.2.91
7. Wen Y, Liu SD, Lei X, Ling YS, Luo Y, Liu Q. Association of Paes With Precocious Puberty in Children: A Systematic Review and Meta-Analysis. Int J Environ Res Public Health (2015) 12(12):15254–68. doi: 10.3390/ijerph121214974
8. Chen C, Zhang Y, Sun W, Chen Y, Jiang Y, Song Y, et al. Investigating the Relationship Between Precocious Puberty and Obesity: A Cross-Sectional Study in Shanghai, China. BMJ Open (2017) 7(4):e014004. doi: 10.1136/bmjopen-2016-014004
9. Subspecialty Group of Endocrinologic, Hereditary and Metabolic Diseases, the Society of Pediatrics, Chinese Medical Association, Editorial Board, Chinese Journal of Pediatrics. Consensus on Diagnosis and Treatment of Central Precocious Puberty (2015). Chin J Pediatr (2015) 53(6):7. doi: 10.3760/cma.j.issn.0578-1310.2015.06.004
10. China MoHotPsRo. Guidelines for the Diagnosis and Treatment of Precocious Puberty (Trial). Chin J Child Health Care (2011) 19(4):390–92. doi: CNKI:SUN:ERTO.0.2011-04-038
11. Abreu AP, Kaiser UB. Pubertal Development and Regulation. Lancet Diabetes Endocrinol (2016) 4(3):254–64. doi: 10.1016/S2213-8587(15)00418-0
12. Weili Y, He B, Yao H, Dai J, Cui J, Ge D, et al. Waist-To-Height Ratio Is an Accurate and Easier Index for Evaluating Obesity in Children and Adolescents. Obes (Silver Spring) (2007) 15(3):748–52. doi: 10.1038/oby.2007.601
13. Donner A. A Review of Inference Procedures for the Intraclass Correlation Coefficient in the One-Way Random Effects Model. Int Stat Rev (1986) 54:67–82. doi: 10.2307/1403259
14. Chen SY, Fuldeore M, Boulanger L, Fraser KA, Chwalisz K, Marx SE. Medical Resource Use and Costs Related to Central Precocious Puberty: A Retrospective Cohort Study. Endocr Pract (2012) 18(4):519–28. doi: 10.4158/EP11293.OR
15. Keil MF, Kang JY, Liu A, Wiggs EA, Merke D, Stratakis CA. Younger Age and Early Puberty Are Associated With Cognitive Function Decline in Children With Cushing Disease. Clin Endocrinol (Oxf) (2022) 96(4):569–77. doi: 10.1111/cen.14611
16. Minelli C, van der Plaat DA, Leynaert B, Granell R, Amaral AFS, Pereira M, et al. Age at Puberty and Risk of Asthma: A Mendelian Randomisation Study. PloS Med (2018) 15(8):e1002634. doi: 10.1371/journal.pmed.1002634
17. Wang H, Lin SL, Leung GM, Schooling CM. Age at Onset of Puberty and Adolescent Depression: "Children of 1997" Birth Cohort. Pediatrics (2016) 137(6):e20153231. doi: 10.1542/peds.2015-3231
18. Holtrup B, Church CD, Berry R, Colman L, Jeffery E, Bober J, et al. Puberty Is an Important Developmental Period for the Establishment of Adipose Tissue Mass and Metabolic Homeostasis. Adipocyte (2017) 6(3):224–33. doi: 10.1080/21623945.2017.1349042
19. Flores AM, Demsas F, Leeper NJ, Ross EG. Leveraging Machine Learning and Artificial Intelligence to Improve Peripheral Artery Disease Detection, Treatment, and Outcomes. Circ Res (2021) 128(12):1833–50. doi: 10.1161/CIRCRESAHA.121.318224
20. Trujillo Rivera EA, Chamberlain JM, Patel AK, Morizono H, Heneghan JA, Pollack MM. Dynamic Mortality Risk Predictions for Children in Icus: Development and Validation of Machine Learning Models. Pediatr Crit Care Med (2022) 23(5):344–52. doi: 10.1097/PCC.0000000000002910
21. McLaughlin M, Pelle KG, Scarpino SV, Giwa A, Mount-Finette E, Haidar N, et al. Development and Validation of Manually Modified and Supervised Machine Learning Clinical Assessment Algorithms for Malaria in Nigerian Children. Front Artif Intell (2021) 4:554017. doi: 10.3389/frai.2021.554017
22. Malizia V, Cilluffo G, Fasola S, Ferrante G, Landi M, Montalbano L, et al. Endotyping Allergic Rhinitis in Children: A Machine Learning Approach. Pediatr Allergy Immunol (2022) 33 Suppl 27:18–21. doi: 10.1111/pai.13620
23. Just AC, Liu Y, Sorek-Hamer M, Rush J, Dorman M, Chatfield R, et al. Gradient Boosting Machine Learning to Improve Satellite-Derived Column Water Vapor Measurement Error. Atmos Meas Tech (2020) 13(9):4669–81. doi: 10.5194/amt-13-4669-2020
24. Sorensen S, Brix N, Ernst A, Lauridsen LLB, Ramlau-Hansen CH. Maternal Age at Menarche and Pubertal Development in Sons and Daughters: A Nationwide Cohort Study. Hum Reprod (2018) 33(11):2043–50. doi: 10.1093/humrep/dey287
25. Yang B, Ostbye T, Huang X, Li Y, Fang B, Wang H, et al. Maternal Age at Menarche and Pubertal Timing in Boys and Girls: A Cohort Study From Chongqing, China. J Adolesc Health (2021) 68(3):508–16. doi: 10.1016/j.jadohealth.2020.06.036
26. Bacopoulou F, Efthymiou V, Landis G, Rentoumis A, Chrousos GP. Waist Circumference, Waist-To-Hip Ratio and Waist-To-Height Ratio Reference Percentiles for Abdominal Obesity Among Greek Adolescents. BMC Pediatr (2015) 15:50. doi: 10.1186/s12887-015-0366-z
27. Wang T, Ma X, Tang T, Jin L, Peng D, Zhang R, et al. Overall and Central Obesity With Insulin Sensitivity and Secretion in a Han Chinese Population: A Mendelian Randomization Analysis. Int J Obes (Lond) (2016) 40(11):1736–41. doi: 10.1038/ijo.2016.155
28. Roemmich JN, Rogol AD. Role of Leptin During Childhood Growth and Development. Endocrinol Metab Clin North Am (1999) 28(4):749–64. doi: 10.1016/s0889-8529(05)70100-6
29. Liu G, Guo J, Zhang X, Lu Y, Miao J, Xue H. Obesity Is a Risk Factor for Central Precocious Puberty: A Case-Control Study. BMC Pediatr (2021) 21(1):509. doi: 10.1186/s12887-021-02936-1
30. Calthorpe L, Brage S, Ong KK. Systematic Review and Meta-Analysis of the Association Between Childhood Physical Activity and Age at Menarche. Acta Paediatr (2019) 108(6):1008–15. doi: 10.1111/apa.14711
31. Shokri E, Heidarianpour A, Razavi Z. Positive Effect of Combined Exercise on Adipokines Levels and Pubertal Signs in Overweight and Obese Girls With Central Precocious Puberty. Lipids Health Dis (2021) 20(1):152. doi: 10.1186/s12944-021-01588-5
32. Mihrshahi S, Drayton BA, Bauman AE, Hardy LL. Associations Between Childhood Overweight, Obesity, Abdominal Obesity and Obesogenic Behaviors and Practices in Australian Homes. BMC Public Health (2017) 18(1):44. doi: 10.1186/s12889-017-4595-y
33. Perez-Farinos N, Villar-Villalba C, Lopez Sobaler AM, Dal Re Saavedra MA, Aparicio A, Santos Sanz S, et al. The Relationship Between Hours of Sleep, Screen Time and Frequency of Food and Drink Consumption in Spain in the 2011 and 2013 Aladino: A Cross-Sectional Study. BMC Public Health (2017) 17(1):33. doi: 10.1186/s12889-016-3962-4
34. Stagi S, De Masi S, Bencini E, Losi S, Paci S, Parpagnoli M, et al. Increased Incidence of Precocious and Accelerated Puberty in Females During and After the Italian Lockdown for the Coronavirus 2019 (Covid-19) Pandemic. Ital J Pediatr (2020) 46(1):165. doi: 10.1186/s13052-020-00931-3
Keywords: precocious puberty, school children, machine learning and deep learning, prediction performance, top factor
Citation: Pang B, Wang Q, Yang M, Xue M, Zhang Y, Deng X, Zhang Z and Niu W (2022) Identification and Optimization of Contributing Factors for Precocious Puberty by Machine/Deep Learning Methods in Chinese Girls. Front. Endocrinol. 13:892005. doi: 10.3389/fendo.2022.892005
Received: 08 March 2022; Accepted: 27 May 2022;
Published: 30 June 2022.
Edited by:
Regis Coutant, Centre Hospitalier Universitaire d’Angers, FranceReviewed by:
Jian Gu, University of Texas MD Anderson Cancer Center, United StatesYue Qi, Capital Medical University, China
Xiaoqun Dong, Brown University, United States
Copyright © 2022 Pang, Wang, Yang, Xue, Zhang, Deng, Zhang and Niu. This is an open-access article distributed under the terms of the Creative Commons Attribution License (CC BY). The use, distribution or reproduction in other forums is permitted, provided the original author(s) and the copyright owner(s) are credited and that the original publication in this journal is cited, in accordance with accepted academic practice. No use, distribution or reproduction is permitted which does not comply with these terms.
*Correspondence: Wenquan Niu, bml1d2VucXVhbl9zaGNuQDE2My5jb20=; Zhixin Zhang, emhhbmd6aGl4aW4wMzJAMTYzLmNvbQ==