- 1Department of Ultrasound, Jiangsu Hospital of Integrated Traditional Chinese and Western Medicine, Nanjing, China
- 2Department of Ultrasound, Affiliated People’s Hospital of Jiangsu University, Zhenjiang, China
- 3Department of Pediatrics, Affiliated Hospital of Jiangsu University, Zhenjiang, China
- 4Department of Radiotherapy, Affiliated People’s Hospital of Jiangsu University, Zhenjiang, China
- 5Department of Pathology, Affiliated People’s Hospital of Jiangsu University, Zhenjiang, China
- 6Department of General Surgery, Affiliated People’s Hospital of Jiangsu University, Zhenjiang, China
- 7Department of Artificial Intelligence, Julei Technology, Company, Wuhan, China
- 8Department of Radiology, Affiliated Hospital of Jiangnan University, Wuxi, China
BRAFV600E is the most common mutated gene in thyroid cancer and is most closely related to papillary thyroid carcinoma(PTC). We investigated the value of elasticity and grayscale ultrasonography for predicting BRAFV600E mutations in PTC.
Methods: 138 patients with PTC who underwent preoperative ultrasound between January 2014 and 2021 were retrospectively examined. Patients were divided into BRAFV600E mutation-free group (n=75) and BRAFV600E mutation group (n=63). Patients were randomly divided into training (n=96) and test (n=42) groups. A total of 479 radiomic features were extracted from the grayscale and elasticity ultra-sonograms. Regression analysis was done to select the features that provided the most information. Then, 10-fold cross-validation was used to compare the performance of different classification algorithms. Logistic regression was used to predict BRAFV600E mutations.
Results: Eight radiomics features were extracted from the grayscale ultrasonogram, and five radiomics features were extracted from the elasticity ultrasonogram. Three models were developed using these radiomic features. The models were derived from elasticity ultrasound, grayscale ultrasound, and a combination of grayscale and elasticity ultrasound, with areas under the curve (AUC) 0.952 [95% confidence interval (CI), 0.914−0.990], AUC 0.792 [95% CI, 0.703−0.882], and AUC 0.985 [95% CI, 0.965−1.000] in the training dataset, AUC 0.931 [95% CI, 0.841−1.000], AUC 0. 725 [95% CI, 0.569−0.880], and AUC 0.938 [95% CI, 0.851−1.000] in the test dataset, respectively.
Conclusion: The radiomic model based on grayscale and elasticity ultrasound had a good predictive value for BRAFV600E gene mutations in patients with PTC.
Introduction
Papillary thyroid carcinoma (PTC) is the most common type of pathological thyroid cancer, accounting for approximately 80–90% of all thyroid cancers and with an annually increasing incidence (1, 2). Most patients have a low death rate, with a 10-year survival rate of > 90%; however, some histological subtypes are prone to extraglandular thyroid invasion, vascular invasion, a high postoperative recurrence rate, and distant metastasis rate. The BRAFV600E gene encodes a protein-dependent kinase, an essential component of the mitogen-activated protein kinase pathway that plays a crucial role in the regulation of cell proliferation, differentiation, and apoptosis (3, 4). The BRAFV600E gene has the highest mutation rate in thyroid cancer and is closely associated with PTC. The mutation of BRAFV600E is an important factor in the PTC phenotype, which contributes to the diagnosis and differential diagnoses of PTC; additionally, the detection rate of PTC is 30–84% (4, 5). The BRAFV600E mutation is closely related to PTC extrathyroid extension invasion and cervical lymph node metastasis, suggesting an invasion of patients with PTC (6, 7). The BRAFV600E mutation was also included in the risk stratification of PTC in the 2015 American Thyroid Association Guidelines; therefore, the preoperative diagnosis of the BRAFV600E mutation is closely related to the development of an appropriate surgical treatment plan to prevent the recurrence of PTC.
While recent studies have shown that grayscale ultrasound can predict the mutation of BRAFV600E in PTC, the results are highly controversial (8, 9). Kabaker et al. reported that vertical and horizontal diameter ratios >1, an unclear boundary, low echo, calcification, and no halo were all associated with the BRAFV600E mutation on ultrasound examination (10). Similarly, Hahn et al. reported that vertical and horizontal diameter ratios >1 were associated with BRAFV600E gene mutations (11); however, some related scholars simultaneously reported that there were no ultrasonic characteristics related to the BRAFV600E mutation (12, 13). Ultrasound radiomics (USR) is a branch of radiomics that is based on ultrasound examination, combined with genetic and clinical data, and employs artificial intelligence as a tool for high-throughput extraction and analysis of relevant tumor information, from structure to molecular cell function analysis, identify tissue and cell level characteristics that cannot be detected by visual reading or conventional quantitative technology, and provide auxiliary decision support for clinical diagnosis and prognosis (14, 15). With high throughput, it can transform the image data from the region of interest (ROI) of lesions into quantitative data with feature space, performing precise quantitative analysis. Previous studies have shown that ultrasonic histogram and texture analysis can help distinguish between benign and malignant thyroid nodules (15, 16); however, there are few reports regarding the ultrasonic image features of the BRAFV600E mutation in PTC using the USR method (8, 9), and there is no further application of multimodal USR.
In this study, the USR features of PTC were analyzed based on grayscale and elasticity ultrasound to predict the risk of the BRAFV600E mutation.
Materials and Methods
Clinical Data
This study was approved by the Ethics Committee of Jiangsu University Affiliated People’s Hospital, and all patients provided written informed consent. PTC patients who underwent a preoperative thyroid ultrasound examination, BRAFV600E mutation detection, and surgery at Jiangsu University Affiliated People’s Hospital between January 2014 and 2021, were retrospectively examined. Figure 1 shows the enrolment procedure. The inclusion criteria were having undergone preoperative grayscale and elasticity ultrasound examination of the thyroid, with related ultrasound images and diagnostic results obtained; a maximum nodule diameter > 5 mm; a maximum nodule diameter > 5 mm, < 5 cm; postoperative pathology-confirmed PTC; and unilateral and single focal lesion. The exclusion criteria were unclear ultrasound images of nodules owing to artifacts and a maximum nodule diameter > 5 cm. Finally, 138 PTCs of 138 patients (mean age, 41.63 ± 11.36 [range, 25–65] years) were analyzed in this study. One hundred thirty-eight patients were divided into BRAFV600E mutation-free group (n=75) and BRAFV600E mutation group (n=63). The clinical information of the enrolled patients, including age, sex, nodule diameter, nodule location, nodule echo, nodule boundary, nodule internal and peripheral blood flow, nodule elastic grading, calcification, cervical lymph node metastasis, and BRAFV600E mutation results were recorded.
Ultrasound and Elastic Ultrasound Examination
The Philips Q5 (both Healthcare, Eindhoven, Netherlands) and the GE LOGIC E20 (GE Medical Systems, American General) ultrasonic instrument (L12-5 linear array probe, frequency: 10–14 MHz) were used. The patients were placed in the supine position, and longitudinal and transverse continuous scanning were performed to obtain longitudinal and transverse images of the thyroid nodules. A coexisting diagram including the nodule diameter, nodule location, nodule echo, nodule boundary, blood flow in and around the nodule, elastic grading of the nodule, calcification, and cervical lymph node metastasis was observed. The elastic imaging mode was enabled, and the position and size of the sampling frame were adjusted on the cross-sectional image. The nodules were positioned at the center of the elastic imaging region, with the ROI more than four times the size of the nodules. The probe was perpendicular to the nodule, and pressure was applied in a steady manner (range 1–2 mm, 1–2 times/s). When the linear strain hint graph (green spring) indicated stability, the freeze key was pressed to obtain an elastic image; the color change in the ROI was observed (green indicated soft; red indicated hard), and the hardness of the nodule was scored based on elasticity. The grading standard of the elastic image was as follows: 1 point, nodules, and surrounding tissues were completely green; 2 points, nodules were red and green (mostly green); 3 points, mixed red and green distribution (mainly red); 4 points, nodules were completely red; and 5 points, the red coverage was greater than that of the nodule.
ROI Segmentation and Normalization
Thyroid ultrasound examinations were performed within one week before surgery. Two radiologists (with 9 and 10 years of experience in the diagnosis of thyroid diseases, respectively) blinded to the pathological and surgical records, completed the manual ultrasonic image segmentation independently. The ROIs were placed on the tumor mass without the surrounding tissue, the ROIs were manually sketched on each image using the ITK-SNAP software (http://www.itksnap.org). The tumor regions in the elasticity ultrasound images were sketched according to the grayscale images. Following the manual ROI segmentation, the z-score normalization method was used to standardize the grayscale and elasticity ultrasound images to obtain a standard normal distribution of image intensities.
Radiomic Feature Extraction and Feature Selection
The PyRadiomics platform from the medical image data was used to extract the standardized set of radiology characteristics (https://github.com/Radiomics/pyradiomics). In total, 479 radiomics features were extracted from the ultrasound images of each ROI. The grayscale features were extracted from the ROI of the grayscale images, and elastic features were extracted from the ROI of the elastic images. The interclass correlation coefficient (ICC) was used to evaluate the inter-observer and intra-observer consistency of feature extraction. ICC > 0.80 was considered excellent. Spearman’s correlation analysis was used to select radiometric that were highly correlated with BRAFV600E mutations. The redundant features identified by Spearman’s correlation coefficient ≥0.8 were eliminated; the minimum redundancy maximum relevance (mRMR) algorithm was subsequently used to select the top 10 features with high relevance and low redundancy for the following analyses. After mRMR, the least absolute shrinkage and selection operator (LASSO) logistic regression method, using 10-fold cross-validation, was used to select the most useful BRAFV600E mutation-related predictive features in the training dataset (Figure 2).
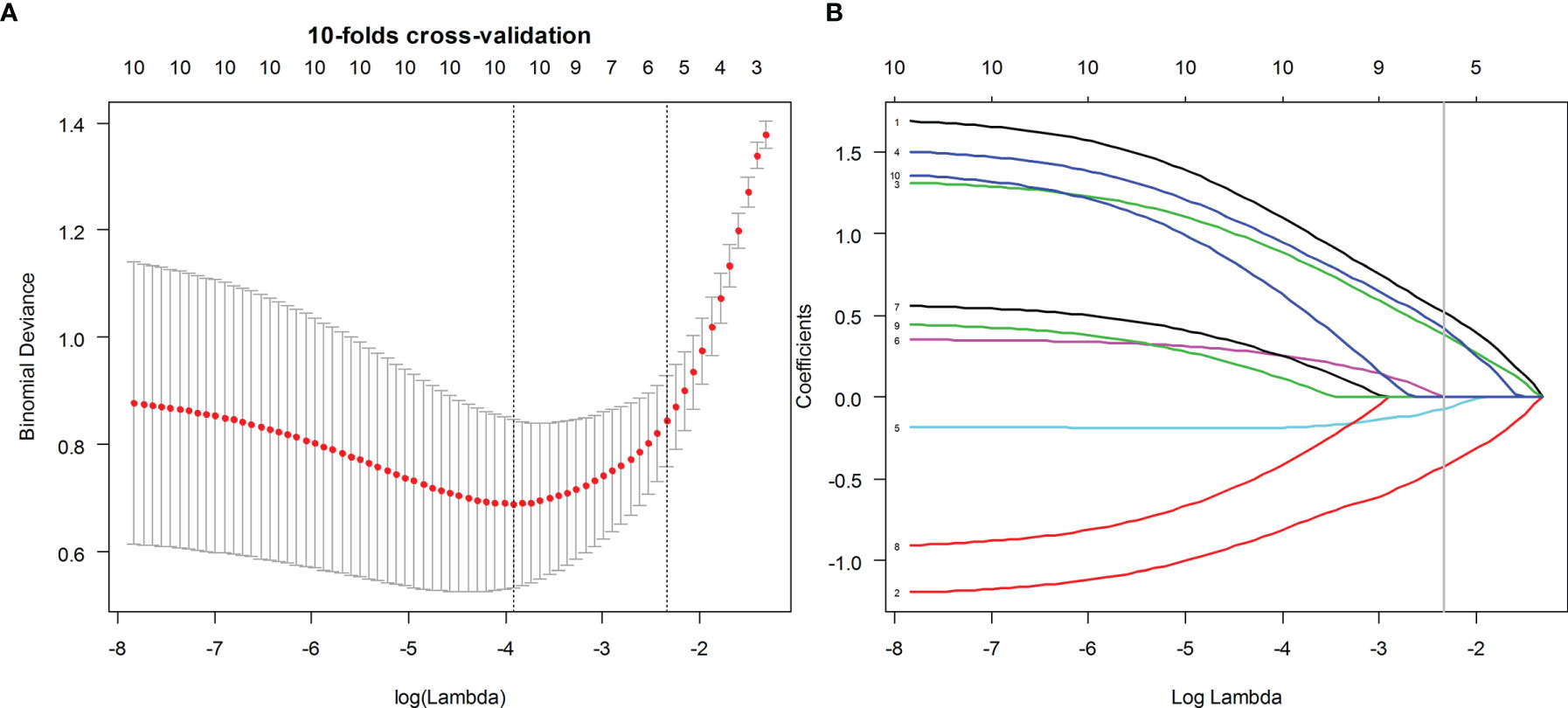
Figure 2 Ultrasound image feature selection using the least absolute shrinkage and selection operator (LASSO) logistic regression model in the training dataset. (A) The 10-fold cross-validation and the minimal criteria process were used to generate the optimal penalization coefficient lambda in the LASSO model. (B) LASSO coefficient profiles of the BMUS and ES features. The dotted vertical line was drawn at the value selected by 10-fold cross-validation when the optimal λ resulted in eight (BMUS) and five (ES) nonzero coefficients, respectively.
According to the result of the LASSO regression analysis, the mathematical expression of the BMUS and ES radiomic signatures are given as follows:
Radiomic Model Construction and Validation
A single radiomics model using grayscale and elasticity ultrasound was generated using a linear combination of the selected features, weighted by the LASSO algorithm with non-zero coefficients. The combined grayscale and elasticity radiomics model was built based on multivariate analysis of the training dataset. Differences in the radiomics model between patients with and without the BRAFV600E mutation were compared using the Mann–Whitney U test. The predictive performance of the radiomics model was evaluated using a receiver operating characteristic (ROC) curve, and the area under the curve (AUC), sensitivity, and specificity were calculated for both the training and test datasets.
Detection Method of BRAF Gene
More than two needles were used to puncture tissue, and DNA was extracted using the QIAamp Human Genome DNA Extraction Kit (QIAGEN). The NanoDrop 1000 (Thermo Fisher, USA) was used to measure DNA concentrations, which were ≥50ng/l. The PCR reaction system was set up in accordance with the PCR kit’s specifications, which included a special probe, reaction solution, and pure water. The processed nucleic acid to be tested, as well as the positive and negative quality controls, were then added to the reaction system, centrifuged at 2000 rpm for 15 seconds to the bottom of the tube, and the PCR amplification reaction was carried out. The reaction conditions were set in accordance with the kit’s specifications. Following the completion of the reaction, the negative quality control should have no S-type amplification curve and the positive quality control should have an S-type amplification curve and a Ct value of 30. The results of the BRAF gene to be tested are finally read after the above two conditions are met.
Statistical Analysis
Statistical analyses were performed using R software (version 3.6.1, https://www.r-project.org) and SPSS 20.0 software (SPSS,Inc., Chicago,IL). Descriptive statistics of continuous variables were expressed as mean ± standard deviation, and the age difference between the two groups was tested using the Student’s t-test. The χ2 test showed that the gender difference between the two groups was statistically significant (P<0.05). To evaluate the best predictive models, AUCs in the ROC analysis were calculated.
Results
Clinical Characteristics
In 138 patients with PTC, 78 cases were classical type, 49 cases were follicular type, and 6 cases were diffuse sclerosis type. The mutation rate of BRAF gene was 45.6% (63/138), 62.8% (49/78) and 16.3% (8/49). The mutation rate of diffuse sclerosing type was 33.3% (2/6) and that of hypercellular subtype was 80% (4/5). A total of 138 PTC patients, including 87 women (mean age, 41.81 ± 11.23 [range, 25–57] years) and 51 men (mean age, 43.82 ± 12.18 [range, 28–65] years). All patients were randomly divided into either the training group (n=96) or the test group (n=42) using a stratified sampling method, and the mutation and non-mutation groups were randomly divided at 7:3, respectively. A comparison of the clinical data and imaging comparisons of the 138 patients between the training and test groups is shown in Table 1. There were no significant differences in clinical characteristics between the two groups, including age and sex (P>0.05). There were also no significant differences in the mean nodule size (BRAFV600E mutant group: 24.12 ± 8.6 mm; without BRAFV600E mutant group: 23.98 ± 11.01 mm, P=0.928) or cervical lymph node metastasis (P=0.102) between the two groups. The relationship between the BRAFV600E mutation and ultrasonic imaging characteristics is shown in Table 2.
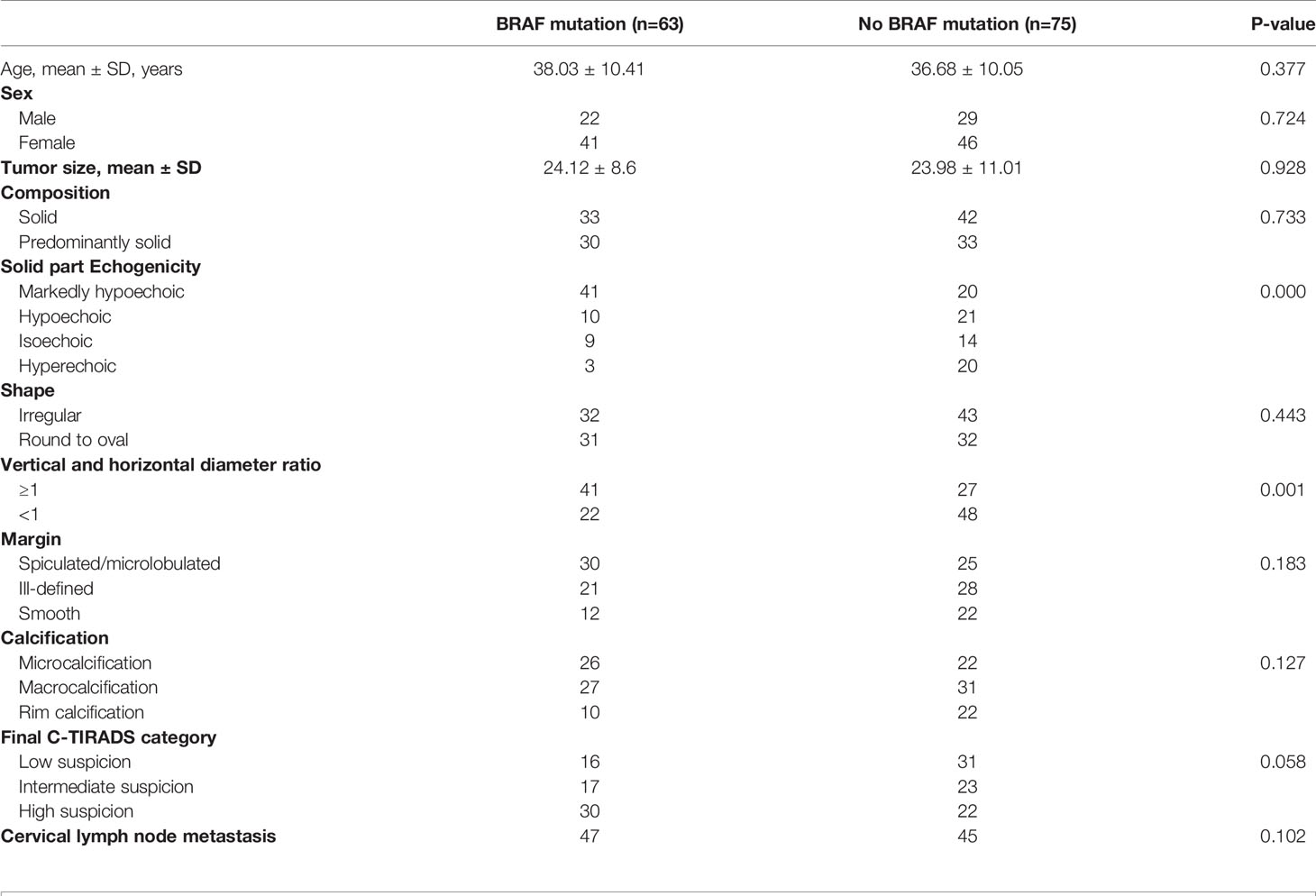
Table 2 Relationship between BRAF mutations and US imaging characteristics through visual assessment of papillary thyroid carcinomas.
Feature Selection
In total, 479 radiomic features were extracted from the grayscale and elasticity ultra-sonograms of each patient. The grayscale features were reduced to eight BRAFV600E mutation-related features after Spearman’s correlation analysis, mRMR, and LASSO algorithms were applied in the training cohort (Figure 3). Likewise, the elasticity features were reduced to five risk predictors in the training cohort (Figure 3). Favorable inter-and intra-observer reproducibility of feature extraction was achieved, with intra-observer ICCs of 0.487–0.965 and inter-observer ICCs of 0.543–0.982.
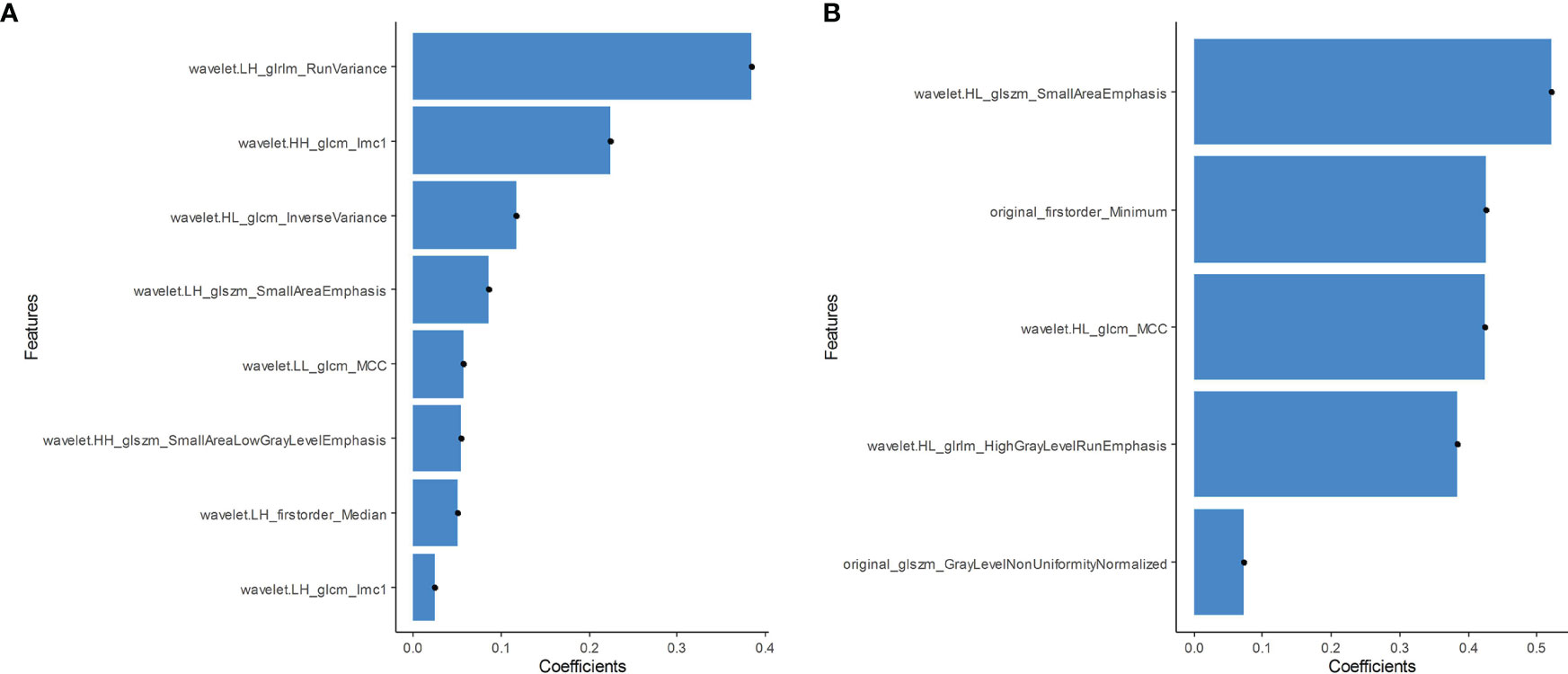
Figure 3 (A) Grayscale features were reduced to eight BRAFV600E mutation-related features in the training cohort. (B) The elasticity features were reduced to five risk predictors in the training cohort.
Model Construction and Validation
According to the grayscale and elastic ultrasound images in the training dataset, logistic regression values were the best classifier. First, we evaluated the performances of the three models in the training dataset and then validated them in the test dataset. The AUCs of the grayscale, elasticity, grayscale, and elasticity combination prediction model in the training dataset was 0.792 (95% CI, 0.703−0.882), 0.952 (95% CI, 0.914−0.990), and 0.985 (95% CI, 0.965−1.000), respectively. In the test dataset, the AUCs were 0.725 (95% CI, 0.569−0.880), 0.931 (95% CI, 0.841−1.000), and 0.938 (95% CI, 0.851−1.000), respectively. The results showed that the elastic prediction model or the combination of the grayscale and elastic prediction model was better than the grayscale prediction model. The AUC, accuracy, sensitivity, and specificity of the three models are shown in Table 3. The ROC curves of the three models are shown in Figure 4. The decision curve analysis (DCA) was applied in determining the clinical usefulness of the radiomic by calculating the net benefits at different threshold values in the combined training and validation cohort. The DCA showed that radiometric provided a higher overall net benefit than the all or no treatment strategy (Figure 5).
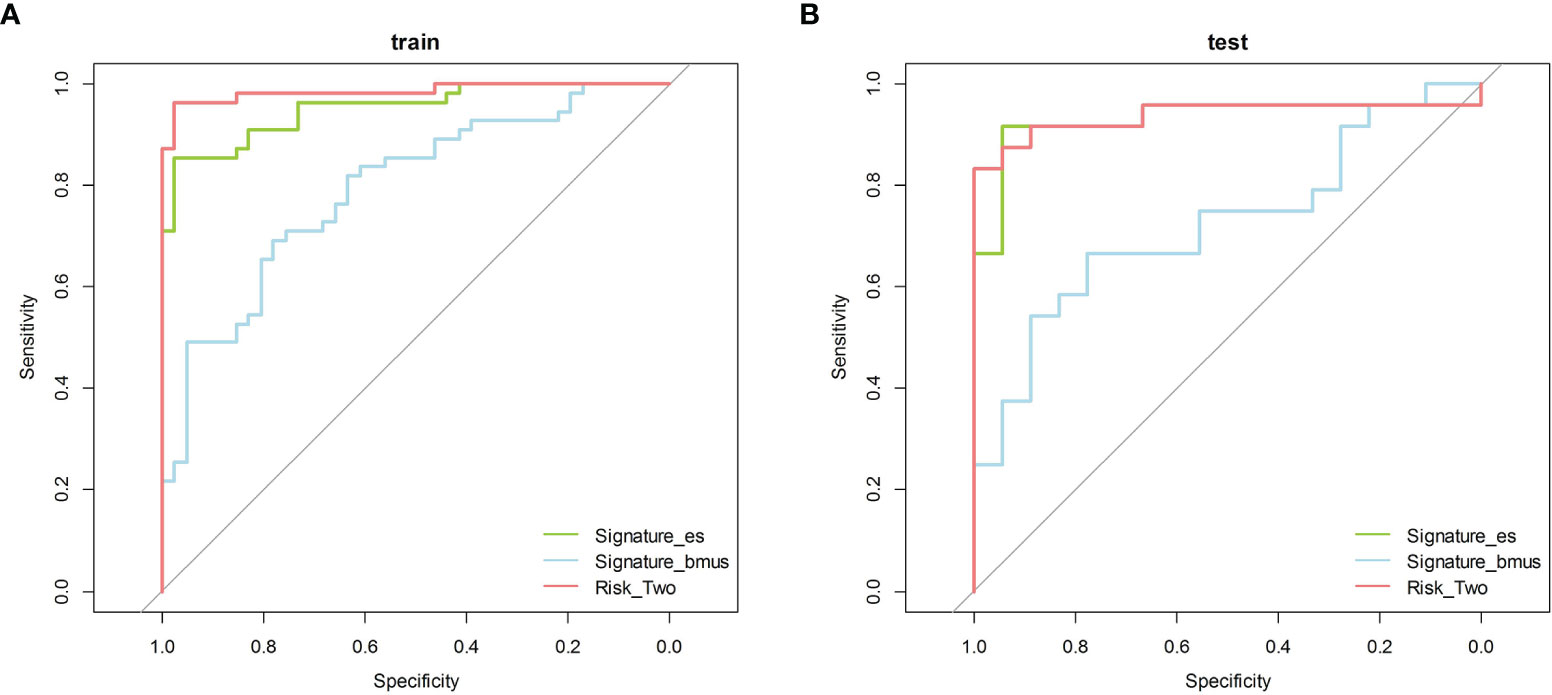
Figure 4 The ROC curves of the three models. ROC, receiver operating characteristic. (A) Training cohort (B) Test cohort.
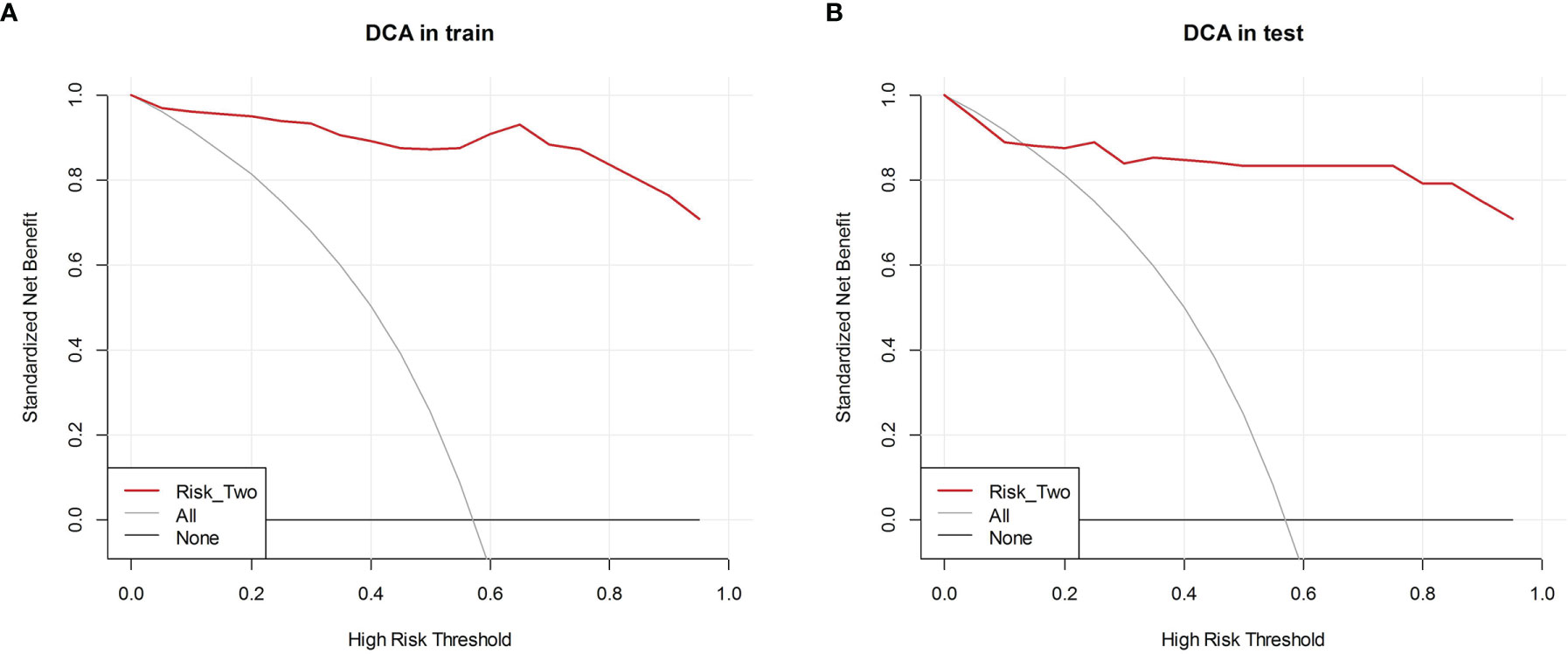
Figure 5 Decision curve analysis (DCA) of each model in predicting BRAFV600E Mutations for papillary thyroid carcinoma (PTC). The vertical axis measures standardized net benefit. The horizontal axis shows the corresponding risk threshold. The DCA showed using the risk_two radiomics (red curve) derived in the present study to predict BRAFV600E Mutations provided the greatest benefit. (A) DCA in training cohort (B) DCA in the test cohort.
Discussion
At present, mutations in the BRAFV600E gene positively correlate with high-risk clinicopathological factors of PTC such as extraglandular invasion, cervical lymph node metastasis, and tumor stage III-IV, suggesting that the BRAFV600E mutation may reflect the degree of malignancy of PTC (17, 18). It plays a key role in the development and evolution of PTC and can be used as an important indicator to objectively judge the invasiveness of PTC. We thus believe that by detecting BRAFV600E mutations and expanding the scope of surgical resection for cases with BRAFV600E mutation, active treatment can be optimized thereby enabling a better prognosis. Because of its strong association with aggressive clinic pathological outcomes and serious molecular derangements in PTC, the BRAF mutation has emerged as a unique and valuable molecular marker in the management of PTC. The preoperative prediction of BRAFV600E mutations in patients with PTC is therefore of great significance for guiding their clinical treatment.
Liu et al. predicted the BRAFV600E mutation in patients with PTC using gray-scale ultrasound radiomics, demonstrating the feasibility of using the USR method for analysis (15). When compared with a single ultrasound imaging mode, USR can delve into details behind PTC ultrasound images to determine the temporal and spatial heterogeneity of PTC, indicating the relationship between image features and BRAFV600E mutations. USR can therefore be used as a noninvasive imaging method for the preoperative evaluation of BRAFV600E mutations in patients with PTC. In our study, we developed a preoperative, radiomic ultrasound model to improve the prediction of the BRAFV600E mutation in patients with PTC. The AUC of gray-scale radiomic sequence model, elastic radiomic sequence model, and multi-sequence (grayscale combined with elastic ultrasound) radiomic model in the training dataset was 0.792, 0.952, 0.985 respectively, and in the test dataset were 0.725, 0.931, 0.938, respectively. Our study shows that both the elastic radiomic sequence model and the multi-sequence (grayscale combined with elastic ultrasound) sequence model can well predict the BRAFV600E mutation in the training dataset and test dataset. Elasticity ultrasound can estimate the corresponding conditions inside PTC tissues via an ultrasonic imaging method combined with digital image processing or digital signal processing technology. Elastography reflects the relative hardness of the lesion and its surrounding tissues, while the hardness of PTC tissues is closely related to its internal pathological structure; therefore, real-time tissue elastography can evaluate the anatomical structure and biological characteristics of PTC.
Bojunga et al. showed that elasticity ultrasound can effectively distinguish between benign and malignant thyroid nodules (19), while Moon et al. found that PTC elastography findings with a degree of high hardness were associated with extrathyroidal invasion (20). These studies provide a new perspective for the ultrasound diagnosis of BRAFV600E mutation-positive and negative PTC. Recent studies have shown that owing to the different arrangement and composition of cells, elasticity indices of parts of tissues are different (21, 22); the higher the malignancy of PTC, the greater the hardness on elasticity ultrasound imaging. There were significant differences in the elastic modulus or hardness between patients with and without BRAFV600E mutations based on the ultrasound images. Elasticity could directly and quantitatively reflect the absolute hardness of BRAFV600E mutation-positive and negative PTC and better reflect the pathological characteristics of tissues. Therefore, multimodal USR was used in this study to predict the BRAFV600E mutation in patients with PTC. A significant association was revealed between ultrasound radiomic features and the BRAFV600E mutation in PTC; thus, our analysis provides an alternative method that is noninvasive and convenient for the assessment of the BRAFV600E mutation in patients. Using LASSO feature selection, 958 quantitative imaging features were reduced to 13 potential features (8 from grayscale and 5 from elasticity ultrasound) suitable for radiomic features analysis of the ultrasound datasets. Most of the selected imaging features were microscopic structural features of the tumor, including cellularity, and compression of the normal thyroid tissues by the tumor. We analyzed the relationship between radiomic features and the BRAFV600E mutation and found 11 wavelet features, 1 first-order feature, and 1 texture feature that were significantly correlated with the BRAFV600E mutation. According to the definitions of these texture features, these radiomic features can serve as a new tool for preoperative prediction of BRAFV600E mutations in PTC patients.
There are some limitations to our study. First, this was a small sample retrospective study conducted at two institutions; thus, a selection bias may exist. In the future, we aim to conduct a multicenter study with a larger sample size. Second, only two ultrasound machines with similar parameters were used in this study to avoid the influence of equipment; however, parameter characteristics and patient-related factors may still affect the pixel intensity of the ultrasound images. Future studies should investigate several different types of ultrasonic instruments. Third, we focused on assessing the BRAFV600E mutation in patients with PTC and did not study the gene in healthy individuals, which may have led to a selection bias. Finally, the lack of external validation data is a limitation of this study.
Conclusions
Our preliminary study suggests that elasticity ultrasound, combined with gray-scale ultrasound imaging, has significant clinical value for predicting BRAFV600E mutation in patients with PTC. This can provide clinicians with a more accurate and noninvasive diagnosis of the BRAFV600E mutation before surgery thereby optimizing treatment outcomes.
Data Availability Statement
The original contributions presented in the study are included in the article/supplementary material. Further inquiries can be directed to the corresponding authors.
Ethics Statement
The studies involving human participants were reviewed and approved by Jiangsu University-affiliated peoples ethics committee. The patients/participants provided their written informed consent to participate in this study.
Author Contributions
Conceptualization, Y-gW, F-jX, EA, XW, and X-qQ. Data curation, GZ, XB, W-zL, and SH. Formal analysis, F-jX, GZ, and W-zL. Funding acquisition, Y-gW, XW, S-dH, and X-qQ. Investigation, HX, Y-dW, and JZ. Methodology, EA, HS, and W-zL. Project administration, XW, S-dH, and X-qQ. Resources, Y-gW, F-jX, and SH. Software, HS, X-sB, and W-zL. Supervision, XW, SH, and X-qQ. Validation, Y-gW, F-jX, HX, Y-dW, and JZ. Visualization, Y-gW, F-jX, and X-sB. Writing – original draft, XW and X-qQ. Writing – review and editing, EA. All authors contributed to the article and approved the submitted version.
Funding
The authors disclose the receipt of financial support for the research, authorship, and/or publication of this article from the Clinical Medicine Science and Technology Development Foundation of Jiangsu University (Project No. JLY2021001) and the Zhenjiang Commission of Science and Technology (Project No. SH2020046, Project No. SH2018050, and Project No. SH2018056). Guiding Project of Jiangsu Health Committee (Z2021071).
Conflict of Interest
The authors declare that the research was conducted in the absence of any commercial or financial relationships that could be construed as a potential conflict of interest.
Publisher’s Note
All claims expressed in this article are solely those of the authors and do not necessarily represent those of their affiliated organizations, or those of the publisher, the editors and the reviewers. Any product that may be evaluated in this article, or claim that may be made by its manufacturer, is not guaranteed or endorsed by the publisher.
References
1. Haugen BR, Alexander EK, Bible KC, Doherty GM, Mandel SJ, Nikiforov YE, et al. 2015 American Thyroid Association Management Guidelines for Adult Patients With Thyroid Nodules and Differentiated Thyroid Cancer: The American Thyroid Association Guidelines Task Force on Thyroid Nodules and Differentiated Thyroid Cancer. Thyroid (2016) 26:1–133. doi: 10.1089/thy.2015.0020
2. Tuttle RM, Haugen B, Perrier ND. Updated American Joint Committee on Cancer/Tumor-Node-Metastasis Staging System for Differentiated and Anaplastic Thyroid Cancer (Eighth Edition): What Changed and Why? Thyroid (2017) 27:751–6. doi: 10.1089/thy.2017.0102
3. Kure S, Ishino K, Kudo M, Wada R, Saito M, Nagaoka R, et al. Incidence of BRAF V600E Mutation in Patients With Papillary Thyroid Carcinoma: A Single-Institution Experience. J Int Med Res (2019) 47:5560–72. doi: 10.1177/0300060519873481
4. Czarniecka A, Oczko-Wojciechowska M, Barczyński M. BRAF V600E Mutation in Prognostication of Papillary Thy-Roid Cancer (PTC) Recurrence. Gland Surg (2016) 5:495–505. doi: 10.21037/gs.2016.09.09
5. Yan C, Huang M, Li X, Wang T, Ling R. Relationship Between BRAF V600E and Clinical Features in Papillary Thyroid Carcinoma. Endocr Connect (2019) 8:988–96. doi: 10.1530/EC-19-0246
6. Rashid FA, Munkhdelger J, Fukuoka J, Bychkov A. Prevalence of BRAFV600E Mutation in Asian Series of Papillary Thyroid Carcinoma—a Contemporary Systematic Review. Gland Surg (2020) 9:1878–900. doi: 10.21037/gs-20-430
7. Sun J, Zhang J, Lu J, Gao J, Ren X, Teng L, et al. BRAF V600E, and TERT Promoter Mutations in Papillary Thyroid Carcinoma in Chinese Patients. PloS One (2016) 11:e153319. doi: 10.1371/journal.pone.0153319
8. Yoon JH, Han K, Lee E, Lee J, Kim EK, Moon HJ, et al. Radiomics in Predicting Mutation Status for Thyroid Cancer: A Preliminary Study Using Radiomics Features for Predicting BRAFV600E Mutations in Papillary Thyroid Carcinoma. PloS One (2020) 15:e228968. doi: 10.1371/journal.pone.0228968
9. Yoon J, Lee E, Koo JS, Yoon JH, Nam KH, Lee J, et al. Artificial Intelligence to Predict the BRAFV600E Mutation in Patients With Thyroid Cancer. PloS One (2020) 15:e242806. doi: 10.1371/journal.pone.0242806
10. Kabaker AS, Tublin ME, Nikiforov YE, Armstrong MJ, Hodak SP, Stang MT, et al. Suspicious Ultrasound Characteristics Predict BRAFV600E-Positive Papillary Thyroid Carcinoma. Thyroid (2012) 22:585–9. doi: 10.1089/thy.2011.0274
11. Hahn SY, Kim TH, Ki CS, Kim SW, Ahn S, Shin JH, et al. Ultrasound and Clinicopathological Features of Pa-Pillary Thyroid Carcinomas With BRAF and TERT Promoter Mutations. Oncotarget (2017) 8:108946–57. doi: 10.18632/oncotarget.22430
12. Li Q, Yuan J, Wang Y, Zhai Y. Association Between the BRAF V600E Mutation and Ultrasound Features of the Thyroid in Thyroid Papillary Carcinoma. Oncol Lett (2017) 14:1439–44. doi: 10.3892/ol.2017.6276
13. Xu J, Chen Y, Dang Y, Dang YY, Chen M. Association Between Preoperative US, Elastography Features and Prognostic Factors of Papillary Thyroid Cancer With BRAFV600E Mutation. Front Endocrinol (Lausanne) (2020) 10:902. doi: 10.3389/fendo.2019.00902
14. Liu T, Ge X, Yu J, Guo Y, Wang Y, Wang W, et al. Comparison of the Application of B-Mode and Strain Elastography Ultrasound in the Estimation of Lymph Node Metastasis of Papillary Thyroid Carcinoma Based on a Radiomics Approach. Int J Comput Assist Radiol Surg (2018) 13:1617–27. doi: 10.1007/s11548-018-1796-5
15. Kwon MR, Shin JH, Park H, Cho H, Hahn SY, Park KW. Radiomics Study of Thyroid Ultrasound for Predicting BRAF Mutation in Papillary Thyroid Carcinoma: Preliminary Results. AJNR Am J Neuroradiol (2020) 41:700–5. doi: 10.3174/ajnr.A6505
16. Xu JM, Xu XH, Xu HX, Zhang YF, Guo LH, Liu LN, et al. Prediction of Cervical Lymph Node Metastasis in Patients With Papillary Thyroid Cancer Using Combined Conventional Ultrasound, Strain Elastography, and Acoustic Radiation Force Impulse (ARFI) Elastography. Eur Radiol (2016) 26:2611–22. doi: 10.1007/s00330-015-4088-2
17. Chen TY, Lorch JH, Wong KS, Barletta JA. Histological Features of BRAF V600E-Mutant Anaplastic Thyroid Carcinoma. Histopathology (2020) 77:314–20. doi: 10.1111/his.14144
18. Celik M, Bulbul BY, Ayturk S, Durmus Y, Gurkan H, Can N, et al. The Relation Between BRAFV600E Mutation and Clinicopathological Characteristics of Papillary Thyroid Cancer. Med Glas (Zenica) (2020) 17:30–4. doi: 10.17392/1086-20
19. Bojunga J, Mondorf A. Schilddrüsen-Elastografie [Thyroid Elastography]. Laryngorhinootologie (2019) 98:150–6. doi: 10.1055/a-0790-0885
20. Jiang M, Li C, Tang S, Lv W, Yi A, Wang B, et al. Nomogram Based on Shear-Wave Elastography Radiomics can Improve Preoperative Cervical Lymph Node Staging for Papillary Thyroid Carcinoma. Thyroid (2020) 30:885–97. doi: 10.1089/thy.2019.0780
21. Yi L, Qiong W, Yan W, Youben F, Bing H. Correlation Between Ultrasound Elastography and Histologic Characteristics of Papillary Thyroid Carcinoma. Sci Rep (2017) 7:45042. doi: 10.1038/srep45042
Keywords: ultrasonic examination, radiomic, papillary thyroid carcinoma, BRAF-V600E, model
Citation: Wang Y-g, Xu F-j, Agyekum EA, Xiang H, Wang Y-d, Zhang J, Sun H, Zhang G-l, Bo X-s, Lv W-z, Wang X, Hu S-d and Qian X-q (2022) Radiomic Model for Determining the Value of Elasticity and Grayscale Ultrasound Diagnoses for Predicting BRAFV600E Mutations in Papillary Thyroid Carcinoma. Front. Endocrinol. 13:872153. doi: 10.3389/fendo.2022.872153
Received: 09 February 2022; Accepted: 23 March 2022;
Published: 22 April 2022.
Edited by:
Akira Sugawara, Tohoku University, JapanReviewed by:
Ting Wang, Guangzhou First People’s Hospital, ChinaFumihiko Furuya, University of Yamanashi, Japan
Copyright © 2022 Wang, Xu, Agyekum, Xiang, Wang, Zhang, Sun, Zhang, Bo, Lv, Wang, Hu and Qian. This is an open-access article distributed under the terms of the Creative Commons Attribution License (CC BY). The use, distribution or reproduction in other forums is permitted, provided the original author(s) and the copyright owner(s) are credited and that the original publication in this journal is cited, in accordance with accepted academic practice. No use, distribution or reproduction is permitted which does not comply with these terms.
*Correspondence: Xian Wang, wangxian8812@126.com; Shu-dong Hu, hsd2001054@163.com; Xiao-qin Qian, yz_tyz1030@126.com
†These authors have contributed equally to this work and share the first authorship