- 1National Chinmedomics Research Center and National Traditional Chinese Medicine (TCM) Key Laboratory of Serum Pharmacochemistry, Department of Pharmaceutical Analysis, Heilongjiang University of Chinese Medicine, Harbin, China
- 2State Key Laboratory of Quality Research in Chinese Medicine, Macau University of Science and Technology, Macau, Macau SAR, China
- 3National Engineering Laboratory for the Development of Southwestern Endangered Medicinal Materials, Guangxi Botanical Garden of Medicinal Plant, Nanning, China
Diabetic retinopathy is one of the serious complications of diabetes, which the leading causes of blindness worldwide, and its irreversibility renders the existing treatment methods unsatisfactory. Early detection and timely intervention can effectively reduce the damage caused by diabetic retinopathy. Metabolomics is a branch of systems biology and a powerful tool for studying pathophysiological processes, which can help identify the characteristic metabolic changes marking the progression of diabetic retinopathy, discover potential biomarkers to inform clinical diagnosis and treatment. This review provides an update on the known metabolomics biomarkers of diabetic retinopathy. Through comprehensive analysis of biomarkers, we found that the arginine biosynthesis is closely related to diabetic retinopathy. Meanwhile, creatine, a metabolite with arginine as a precursor, has attracted our attention due to its important correlation with diabetic retinopathy. We discuss the possibility of the arginine-creatine metabolic pathway as a therapeutic strategy for diabetic retinopathy.
Introduction
Diabetic retinopathy (DR) has been recognized as the main cause of blindness worldwide, with about one-third of all diabetes patients developing diabetic retinopathy (1). The retina is metabolically active and transmits electrochemical signals from photoreceptors to the brain via neurons, supported by glial cells and vascular tissue (2). The entire process relies on highly complex coordination between the various cell types, and the blood-vision barrier plays a key role (3, 4). The accumulation of glycation end products, oxidative stress, polyol pathway and protein kinase C (PKC) activation are the main pathogenesis of DR. This changes the normal interaction between cells and causes serious blood vessel abnormalities leading to damaging of the blood-retinal barrier and neuronal function (5–9). Diabetic retinopathy is difficult to cure, diagnosis and drug intervention in the early stages of diabetic retinopathy can effectively prevent or slow down the progression of disease. Therefore, identification of biomarkers associated with disease progression can be very helpful.
Metabolomics is the analysis of a large number of endogenous small molecules. It provides the overall metabolic profile of a biological sample as opposed to genomics and proteomics, which provide the profiles for DNA/RNA and proteins alone, respectively (10–12). The methods of analysis used in metabolomics are mostly classified into two categories: targeted metabolomics and non-targeted metabolomics (13–15). In contrast to targeted metabolomics, which focuses only on changes in specific metabolites, non-targeted metabolomics is designed to capture much more metabolite information to compare these high-throughput data under normal vs. disease states (15–17). Non-targeted metabolomics approaches can thus discover potential biomarkers of diseases and provide an effective basis for diagnosing and treating them (18–20).
Arginine, a semi-essential amino acid, involved in many biological processes such as creatine biosynthesis and the urea cycle, is one of the strongest insulin secretagogues, which induce insulin release from pancreatic β cells (21). Additionally, arginine is a substrate for nitric oxide synthase (NOS) and can produce NO, which exerts a significant influence on the health of the vascular endothelial cells as well as the kidneys (22, 23). Creatine (Cr) can be either be synthesized endogenously within the body or extrinsically derived from foods like meat, fish, etc. (24). Cr, phosphocreatine(PCr), and creatine kinase (CK) isoenzymes are responsible for maintaining the ATP pool (25). Therefore, creatine is one of the leading sports supplements (26). As research continues, Creatine has been found to have multiple physiological effects, including anti-inflammatory (27–29), antioxidant (30–33), neuroprotective (34), reduce homocysteine(Hcy) (35–37), and anti-diabetic (34).
This review aims to summarize the progress of metabolomics studies in diabetic retinopathy and to explore common research platforms for metabolomics. We also summarize the current knowledge of known metabolomics biomarkers of diabetic retinopathy based on literature and analyze the metabolic pathways involving those biomarkers. In addition, we discuss the creatine-arginine metabolic network as a potential area for finding new treatment strategies.
Metabolomics Analysis Platform
Metabolomics analysis platform can be divided into two main types, nuclear magnetic resonance (NMR) spectroscopy (38) and mass spectrometry (MS) (39, 40). Using different instruments and platforms, typically 50 to as many as 5000 different metabolites can be identified at any given time. No technique so far has been successful in identifying all metabolites in a single run or analysis, and most metabolomics studies use only one platform or multiple tandems. Due to the complementarity between NMR (41) and MS (42), researchers often use combinations of NMR and MS as well as employ the current method to enhance research quality and expand the metabolome coverage (43–46).
Nuclear Magnetic Resonance (NMR) Spectroscopy
NMR spectroscopy can measure the behavior of an atom’s nucleus when subjected to a magnetic field (47, 48). Currently, instruments that use 500 and 600 MHz frequencies are the most widely used instruments to detect these signals and are the optimal choice for their sensitivity and manufacturing cost. It is worth noting that the resolution of these signals increases when the magnetic field strength is higher (49).
NMR spectroscopy applies to both liquid/gas phase samples as well as tissue samples (50, 51). It carries several advantages, for example, it requires less sample preparation and the detection process is non-destructive to the sample, so it can be reused for other studies. Moreover, NMR has high reproducibility and good quantitative performance, allowing the measurement of the number of protons under a given condition which allows for direct comparison with spectral data (52). However, the primary disadvantage of NMR is its lower sensitivity compared with MS. NMR can identify nearly 50 metabolites in serum/plasma samples and approximately 200 in urine (53).
Mass Spectrometry (MS)
Mass spectrometry is an analytical method that measures the ion-to-mass ratio based on the ionization of components in the samples by an ion source, and is widely used in the detection of metabolites (54–56). The sample can be directly analyzed by mass spectrometry, or in tandem with other separation methods to obtain mass spectra, such as liquid chromatography (LC) (57–59), gas chromatography (GC) (60, 61), hydrophilic interaction chromatography-mass spectrometry (HILIC-MS) (62), Flow-injection analysis-mass spectrometry(FIA–MS) (63), or capillary electrophoresis (CE) (64, 65). It should be noted that no single method can separate all metabolites simultaneously, as some metabolites are difficult to ionize, and in some cases, mass number limitations prevent mass spectrometry techniques from measuring all metabolites (66). LC has been most widely used because of its better separation. Especially, high-performance liquid chromatography (HPLC) and ultra-high performance liquid chromatography (UPLC) have become increasingly popular (67–69). GC also offers high separation, but it is unable to measure metabolites with poor thermal stability (70). Capillary electrophoresis (CE) has a long history of use. Its application is mainly limited by its poor sensitivity, which has been greatly improved by the introduction of the CE-ESI interface (71, 72).
Compared to NMR, MS has a much higher sensitivity and is therefore able to measure a wider range of metabolites (40, 43, 73, 74). In particular, UPLC offers excellent chromatographic separation, high speed, and high sensitivity, allowing the detection of thousands of metabolites within a short time (75–78). HPLC tandem MS plays a huge contribution in research that requires high throughput, such as natural drug development and disease biomarker identification (79–83).
Biomarkers for Diabetic Retinopathy
Vitreous Humor Biomarkers
Tomita et al. (84) analyzed the metabolites of vitreous humor in 43 proliferative diabetic retinopathy (PDR) patients, and 21 controls using ultra-performance liquid chromatography-mass spectrometry (UPLC-MS) with significant differences in creatine. The authors found that patients with PDR had lower levels of creatine and higher levels of glycine in the vitreous humor than controls. They also verified in an oxygen induced ischemic retinopathy (OIR) model that reduced creatine levels correlate with retinal vascular proliferation and demonstrated that oral creatine caused a significant reduction in retinal vascular proliferation (p=0.0024), opening the possibility for a new therapeutic strategy for diabetic retinopathy. Wang et al. (85) identified potential DR biomarkers in vitreous humor using gas chromatography mass spectrometry (GC-MS). Vitreous humor samples were gathered from 28 type-2 diabetes patients with PDR as well as 22 non-diabetic patients with macular fissure. They found 15 potential biomarkers in the vitreous humor, namely pyruvate, ornithine, uric acid, pyroglutamic acid, creatinine, L-leucine, L-alanine, L-threonine, L lysine, L-valine, L-phenylalanine, L-isoleucine, L-glutamine, inositol, and hydroxylamine. These are mainly involved in various metabolic pathways such as gluconeogenesis, ascorbate-aldose metabolism, valine-leucine-isoleucine biosynthesis, and arginine-proline metabolism.
A non-targeted metabolomics study on vitreous humor from patients with DR showed changes in glucose metabolism as well as activation of the pentose phosphate pathway. Glass fluid samples from PDR patients (n=9) and normal subjects were kept as controls (n=8) and were analyzed by ultra-performance liquid chromatography-mass spectrometry (UPLC-MS). A variety of metabolites were found to be potential biomarkers, including xanthine, pyruvate, proline, and guanine (86). Paris et al. (62) used liquid chromatography-mass spectrometry (LC-MS) and hydrophilic interaction liquid chromatography (HILIC)-mass spectrometry to analyze the vitreous humor of PDR patients (n=9), non-diabetes control patients (n=11), and OIR mouse model. They found significant changes in the levels of octanoyl carnitine, propionyl carnitine, hexanoyl carnitine, acetylcarnitine, palmitoylcarnitine, elaidic/vaccenylcarnitine, allantoin, glutamate, lysine, and arginine. Barba et al. (87) analyzed the vitreous humor of a total of 22 patients suffering from PDR and 22 non-diabetic patients and found that the content of lactate and glucose among the PDR patients was higher than that in non-diabetic patients, while that of galactitol and ascorbic acid was lower when compared with that in non-diabetic patients. The reduced galactitol level was attributed to activation of the polyol pathway.
Plasma Biomarkers
Plasma metabolomics of 124 DR patients and 32 controls were explored using GC–MS, and UPLC–MS. They identified glutamine and glutamic acid as new biomarkers for the prediction of DR (88). A plasma metabolomics analysis based on GC–MS demonstrated that 2,4-dihydroxybutyric acid (DHBA), 3,4-DHBA, ribonic acid, and ribitol are risk markers for DR progression as these metabolites are associated (P <0.042) with DR (89). Another plasma metabolomics study using GC-MS identified 11 potential biomarkers of diabetic retinopathy, namely 1,5-gluconolactone, 1,5-anhydroglucitol, gluconic acid, lactose/cellobiose, maltose/trehalose, 2-deoxyribonic acid, 3,4-dihydroxybutyric acid, erythritol, mannose, ribose, and urea. The samples for this study were acquired from 40 patients undergoing non-proliferative diabetic retinopathy (NPDR) and 40 patients suffering from T2DM without retinopathy. Metabolic pathway analysis indicated a remarkable enrichment of the pentose phosphate pathway, which could explain the NADPH production against oxidative stress (49). Sumarriva et al. performed plasma metabolomics research showed that compared to diabetes controls, the metabolism of multiple amino acids, such as leukotrienes, niacin, pyrimidine, and purine, changed in DR patients. Arginine, citrulline, glutamic γ-semialdehyde, and de-hydroxy carnitine were critical members in the above pathways differences (90). Li et al. (91) employed GC-MS in the study of plasma metabolomics in 25 patients with PDR, 39 patients with NPDR, and 24 patients with NDR, and found 10 metabolites with significant differences: β-hydroxybutyrate, methylmalonic acid, citric acid, pyruvate, glucose, stearic acid trans-oleic acid, L-aspartate, linoleic acid, and arachidonic acid.
Serum Biomarkers
Xuan et al. (92) studied 43 patients with diabetic retinopathy and 44 normally controlled serum lipomics using UPLC-MS. Significant differences were found in the following 14 lipid metabolites: Lysophosphatidylcholine(LPC)(14:0) LPC (14:0), LPC (16:0) LPC (14:0), LPC (16:0), LPC (16:1), LPC (18:0), LPC (18:1), LPC (18:2), LPC (18:3), LPC (18:4), LPC (20:0), LPC (20:3), LPC (20:4), LPC (20:5), LPC (22:3), and LPC (22:6). These provide a basis for the discovery of lipid biomarkers in diabetic retinopathy. Xuan et al. (93) in their study used multi-platform techniques to analyze serum samples from 111 diabetic patients without retinopathy (NDR=111) and 350 diabetic patients with retinopathy (n=350). The DR-induced metabolic changes were usually linked to glycolytic metabolism, tricarboxylic acid cycle (TCA) metabolism, urea cycle metabolism, polyol metabolism, amino acid metabolism, and lipid metabolism. Following a systematic screening using univariate analysis, 2-piperidone and 12-HETE were recognized as potential biomarkers for DR. 12-HETE, an eicosanoid-like acid, is the leading product of human 12-lipoxygenase (LOX), inducing endoplasmic reticulum stress in human retinal endothelial cells. Studies show that 12-LOX is involved in retinal microvascular disorders of DR (94–96). A study based on widely targeted metabolomics evaluated serum metabolites from 69 type 2 diabetes mellitus (T2DM) patients with DR and 69 T2DM patients without DR. The biomarkers of diabetic retinopathy identified using a UPLC-MS system were linoleic acid, nicotinuric acid, ornithine, and phenylacetylglutamine. In particular, this research developed a new multidimensional network of biomarker systems and the area under the curve (95% CI) of this system is an exploration of the biomarker determination method (97).
Zhu et al. (98) studied the serum metabolomics of 21 PDR patients and 21 diabetic patients without retinopathy (NDR) patients. A total of 63 significant changes in metabolites were found using LC-MS. Fumaric acid, uridine, acetic acid, and cytidine (area under curve 0.96, 0.95, 1.0, and 0.95, respectively) are considered potential biomarkers of PDR. A serum metabolomics study of 24 patients with PDR, 22 patients with NPDR, and 35 healthy human control groups demonstrated that compared with the control group, indolamine-2,3-dioxygenase (IDO) expression was enhanced among patients with NPDR, while the levels of kynurenine, kynurenic acid, and 3-hydroxy kynurenine were higher in PDR patients. The authors speculated that diabetic retinopathy might be related to IDO and tryptophan metabolites (99). Serum samples from patients with NPDR (n=123), PDR (n=51), and NDR (n=143) were profiled by targeted mass-spectrometry-based metabolomics. After multivariate analyses, 16 metabolites were found to show profound changes, including tetradecenoylcarnitine (C14:1), hexadecanoylcarnitine (C16), lysine, methionine, tryptophan, tyrosine, total dimethyarginine, phosphatidylcholine diacyl C32:2, phosphatidylcholine diacyl C34:2, phosphatidylcholine diacyl C36:2, phosphatidylcholine diacyl C38:6, phosphatidylcholine diacyl C40:6, phosphatidylcholine acyl-alkyl C36:5, phosphatidylcholine acyl-alkyl C42:3, hydroxysphingomyeline C22:1 and sphingomyeline C24:0 (63).
Aqueous Humor Biomarkers
Wang et al. (85) analyzed and identified potential DR biomarkers in aqueous humor of 23 patients suffering from PDR and 25 patients with non-diabetic cataracts. Eight metabolites, namely D-glyceric acid, isocitric acid, threonine, d-glucose, inositol, L-lactic acid, citrulline, and fructose 6-phosphate, were found to be significantly different in the aqueous humor by comparative analysis.
A metabolomics study based on NMR was carried out on the aqueous humor samples from diabetic patients with cataracts (n=13), DR patients with cataracts (n=14), and elderly cataracts (n=7). Metabolites such as lactate, succinate, 2-hydroxybutyrate, aspartamide, dimethylamine, histidine, threonine, and glutamine showed significant changes. Pathway analysis showed that DR might be related to alanine, aspartic acid, and glutamate metabolic pathways (100). The information of DR biomarker was listed in Table 1.
KEGG Enrichment Analysis
We enriched the above potential biomarkers according to the types of biological fluids, intending to comprehend the relationship between biomarkers and diseases. Enrichment analysis by metaPA and Kyoto Encyclopedia of Genes and Genomes (KEGG) showed that metabolic pathways enriched in the different biological fluids are unique (Figure 1). It is worth mentioning that arginine-related metabolism was both enriched in vitreous humor, plasma, serum, and aqueous humor. This suggests that arginine has a critical effect on diabetic retinopathy.
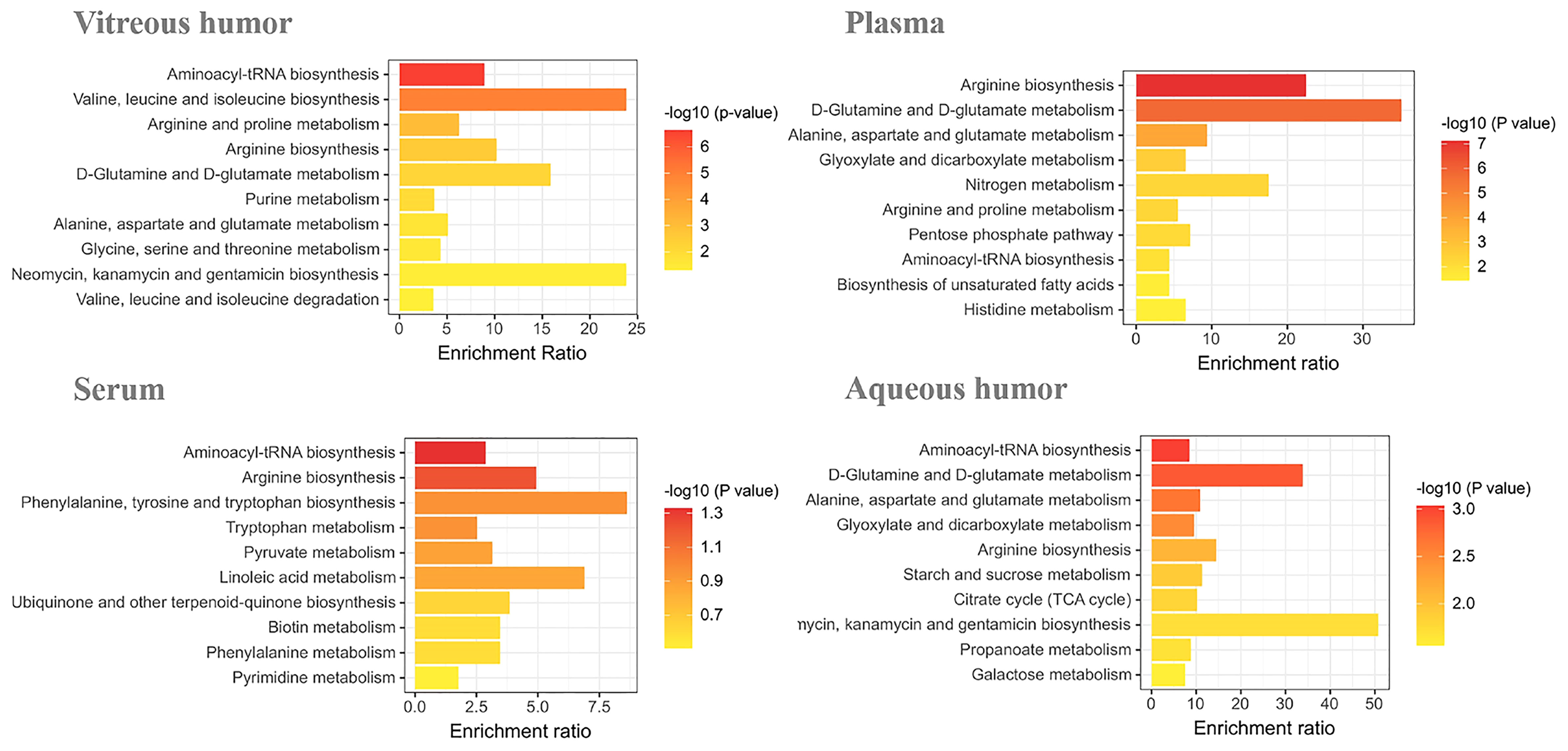
Figure 1 Enrichment analysis of DR potential biomarkers in vitreous humor, plasma, serum, and aqueous humor.
Discussion
Biomarkers can provide early warning signs in patients with serious diseases. Therefore, they help in the early diagnosis of the disease so that effective treatment can be made available to the patient at the earliest. In this review, we have summarized the known potential biomarkers for DR, in a variety of biological samples, including vitreous humor, plasma, serum, and aqueous humor, from research done in recent years. Through enrichment analysis, we found that arginine-related metabolic pathways were abnormal in a variety of biological fluids.
Arginine Biosynthesis-Related Metabolites Are Significantly Elevated in DR Patients
The urea cycle is a part of the arginine biosynthesis pathway, and the arginase enzyme can cleave arginine to generate urea and ornithine. Ornithine can be converted into citrulline, and then citrulline is produced through a series of reactions to arginine (101). The metabolites of the urea cycle seem to have some association with DR. The metabolites of the urea cycle seem to have some association with DR.The levels of ornithine (85, 97, 102), arginine (62, 90, 102), citrulline (85, 90, 102), proline (86), and argininosuccinate (102) were significantly elevated in DR patients (Figure 2) (73). The above content expands our understanding of the pathogenesis of DR. The changes in the metabolites of the urea cycle, especially arginine, are significantly associated with DR.
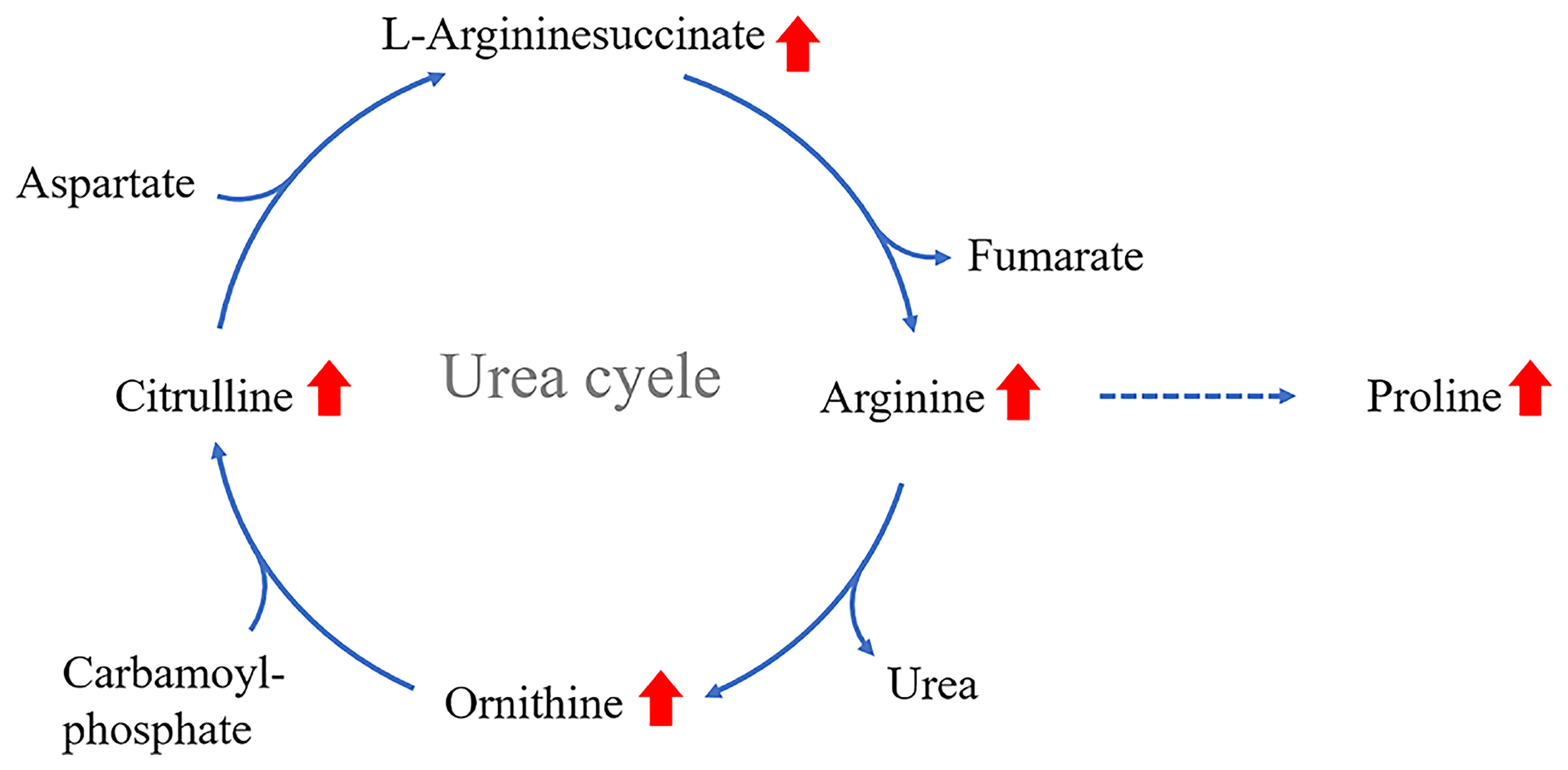
Figure 2 Increased levels of proline, ornithine and arginine in the vitreous humor of PDR patients; arginine levels are elevated in the serum of severe DR patients; citrulline levels are elevated in the aqueous humor of DR patients.
Arginine is involved in many biological processes and is also the substrate of nitric oxide synthase (NOS) and arginase, producing nitric oxide (NO) and urea, respectively (103). NO is a vasodilator that exerts a significant influence on vascular endothelial health, while arginine induces the release of insulin in pancreatic β cells (Figure 3) (104). In addition, animal experiments using DR mouse models and bovine retinal endothelial cells cultivated by high glucose revealed the role of arginine metabolism as a mediator for DR (105, 106).
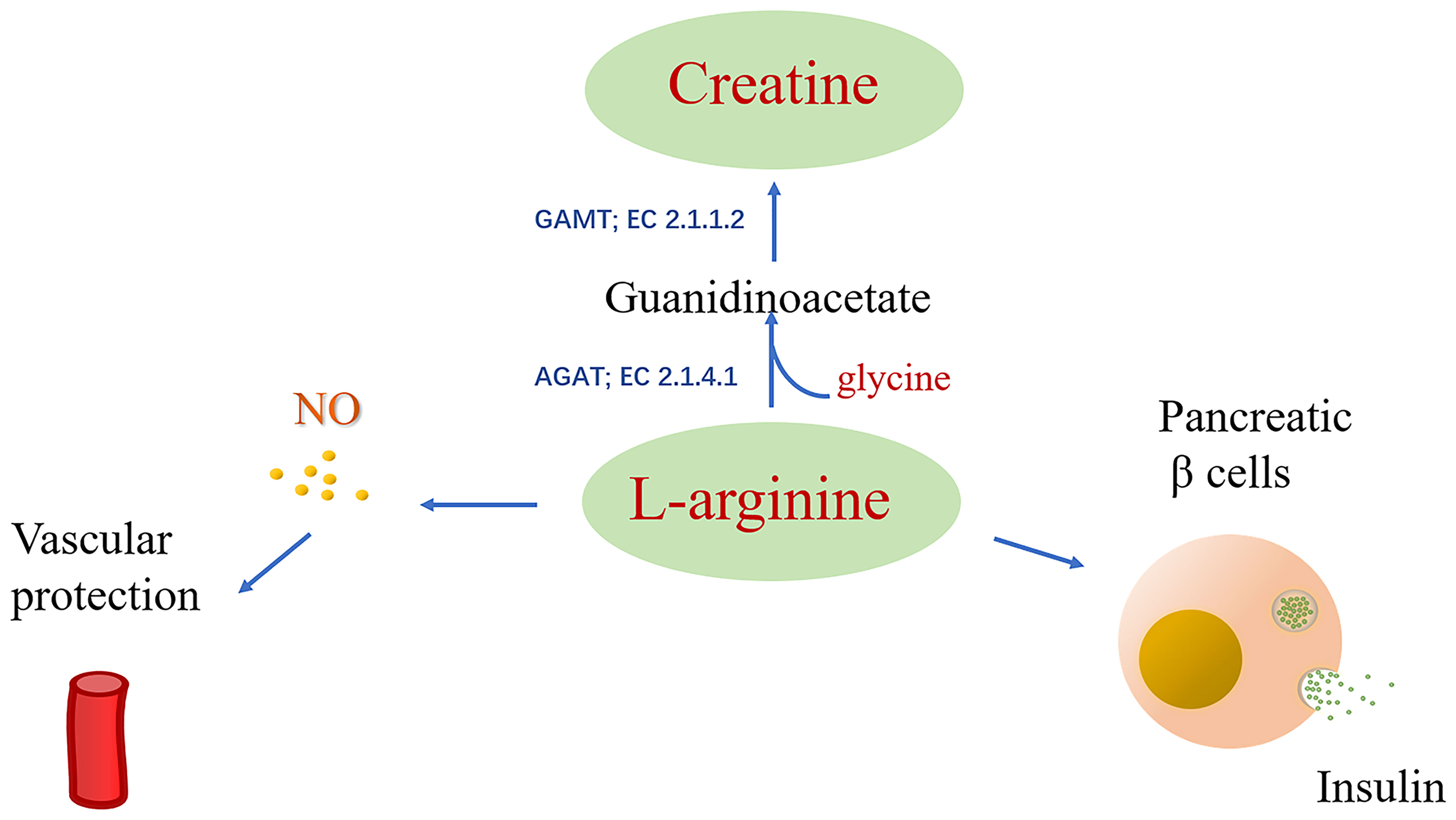
Figure 3 Arginine is catalyzed by the substrate of nitric oxide synthase (NOS) to produce NO, and arginine can induce the release of insulin from pancreatic β cells.
Arginine-Creatine Metabolic Pathway May Be a New Therapeutic Strategy for DR
Meanwhile, another biomarker that caught our attention, creatine, a product of arginine metabolism. Unlike the elevated levels of arginine, creatine levels were significantly lower in patients with DR (84, 85). Thereby, we put forward a hypothesis that the reduced conversion of arginine to creatine leads to metabolic changes in DR patients with increased arginine levels and decreased creatine levels. Callback of this metabolic change, may be a new treatment strategy for DR. There is no strong evidence for this hypothesis, but there is substantial research supporting the positive effects of creatine supplementation on DR.
Creatine can be either be synthesized endogenously within the body or extrinsically derived from foods like meat, fish, etc. (24). There are two steps in creatine biosynthesis. The first step is to catalyze arginine and glycine with L-arginine glycine amidinotransferase (AGAT; EC 2.1.4.1) to produce ornithine and guanidinoacetate (GAA). This step mainly occurs in the kidney and is mostly distributed in the mitochondrial intermembrane space (107). The second step is the methylation of GAA in the amidino group for producing Cr through the action of S-adenosyl-l-methionine: N-guanidinoacetate methyltransferase (GAMT; EC 2.1.1.2) (108), the liver is possible to be the principal organ contributing this reaction (109, 110). Approximately two-thirds of Cr is phosphorylated to form PCr, a key agents of cellular energy regeneration (111, 112). Cr, PCr, and creatine kinase (CK) isoenzymes are responsible for maintaining the ATP pool (25). This is critical for some organs with high energy demands, like retina, skeletal or cardiac muscle, retina, spermatozoa, and brain (113).
AGAT is the rate-limiting enzyme in creatine biosynthesis, simultaneous reduction in mRNA content, enzyme levels, and AGAT enzyme activity when endogenous sources or dietary Cr supplementation (114). This feedback inhibition of AGAT by Cr is most pronounced in the kidney and pancreas, which are the major tissues for GAA production (115). Research shows that ingestion of creatine supplements reduces the rate of creatine biosynthesis (116).GAA, catalyzed by GAMT to generate creatine, is an important intermediate in creatine biosynthesis. Deficiency of GAMT will cause GAA accumulation and lead to axonal hypersprouting and apoptosis (117). There are no reports of abnormal GAA levels in DR patients.
Studies have shown that creatine supplementation can help improve hyperglycemia (34) and improve glycemic control in patients with type 2 diabetes (118). In mice, lower creatine levels could be ascribed to the vascular proliferation of the retina under the OIR model (p=0.027) with the use of retinal metabolomics. Moreover, it was seen that this vascular proliferation could be reversed after the administration of oral creatine via anti-VEGF (84). Tomita et al. found that a decrease in creatine was accompanied by an increase in glycine levels in OIR mice, this results consistent with the vitreous humor of PDR patients (84). Glycine is involved in the biosynthesis of creatine, the amidine group of arginine is transferred to glycine to generate ornithine and GAA, and then GAA is catalyzed by GAMT to generate creatine. Increased glycine appears to be protective for DR, and glycine has proven anti-glycation and anti-diabetic properties (119, 120). Moreover, glycine significantly upregulated the mRNA expression of PEDF (an angiogenesis inhibitor) (121). However, in the study by Tomita et al., arginine was not significantly different in the vitreous humor of PDR patients and the retina of OIR mice. In previous studies, arginine was reported to be significantly elevated in plasma and vitreous humour (62, 90, 102).
Mitochondria are the primary site of production ATP and the main source of cellular energy. The number of mitochondria in a cell depends on its energy demand (122). Mitochondrial dysfunction due to overproduced of ROS in hyperglycemic states (122, 123), and make a major impact on tissues with high energy demands, such as the retina (111). Study shows persistent hyperglycemia leads to reduced mitochondrial respiration (124), Cr-Pcr system is essential for energy-demanding tissues and cells due to the maintenance of adequate ATP pools (111).
Another study showed that creatine enhanced the functional capillary density in skin and recruitment in post-occlusive reactive hyperemia (35, 125). The author speculates that creatine may help increase the bioavailability of epoxyeicosatrienoic acid (EET), thereby improving endothelium-derived hyperpolarizing factor (EDHF) stimulation and microvascular dilation (125). Apart from this, the potential therapeutic effect of creatine on the nervous system also deserves attention. It has been reported that creatine protects against neurotoxicity and oxidative stress (30, 31). Oxidative stress is one of the biggest risk factors for diabetic retinopathy. An animal experiment demonstrated that creatine has a significant antioxidant effect and indicated that creatine supplementation may become a treatment strategy for neurodegenerative diseases caused by oxidative stress (34, 126). Besides, creatine administration significantly attenuated abnormal glucose tolerance, and is considered to delayed the onset of diabetes (34). Studies have shown that creatine exhibits resistance to oxidation, which is effective in protecting mtDNAs from oxidative stress-elicited cytotoxicity (127, 128). Suggestively, creatine could provide a way for the effective management of diseases involving oxidative stress (126–128).
Synthesis of creatine yields homocysteine as a byproduct, which is an amino acid that contains sulfhydryl groups. S-adenosylmethionine (SAM) is demethylated to generate creatine as well as S-adenosyl homocysteine (SAH). SAH hydrolase (SAHH) enzyme then hydrolyzes SAH to Hcy. A correlation has been reported between the increase in Hcy expression and an aggravated risk for diverse DR, including blood retinal barrier dysfunction, inflammation, and mitochondria dysfunction (129–131). Replenishment of creatine has been demonstrated to save the SAM input (132–134) given about 40–70% expenditure of entire methyl groups by the creatine synthesis (134), which can diminish the Hcy formation (133) and may help reduce the possibility of developing DR.
In addition, creatine can reduce acute inflammation induced by carrageenan, whose action is identical to that of butazepine, a non-steroidal anti-inflammatory drug (27). Research done by Nomura et al. on pulmonary endothelial cells (ECs) revealed that after the administration of 0.5 mM creatine, the endothelial cell (EC) expressions of E-selectin and Intercellular Adhesion Molecule-1 were suppressed. Moreover, the serotonin-and H2O2-elicited permeability of endothelium was also prominently reduced upon creatine (5 mM) replenishment. These observations suggested that the administration of creatine makes the membranes more stable, and the ECs less leaky (28). Associations between DR and increased intercellular cell adhesion molecule-1 (ICAM-1), E-selectin expressions, and enhanced permeability “leakiness” of the endothelium have been reported several times (135). It shows that creatine has the potential to act as a protector of the vascular system and as an inflammation inhibitor (Figure 4).
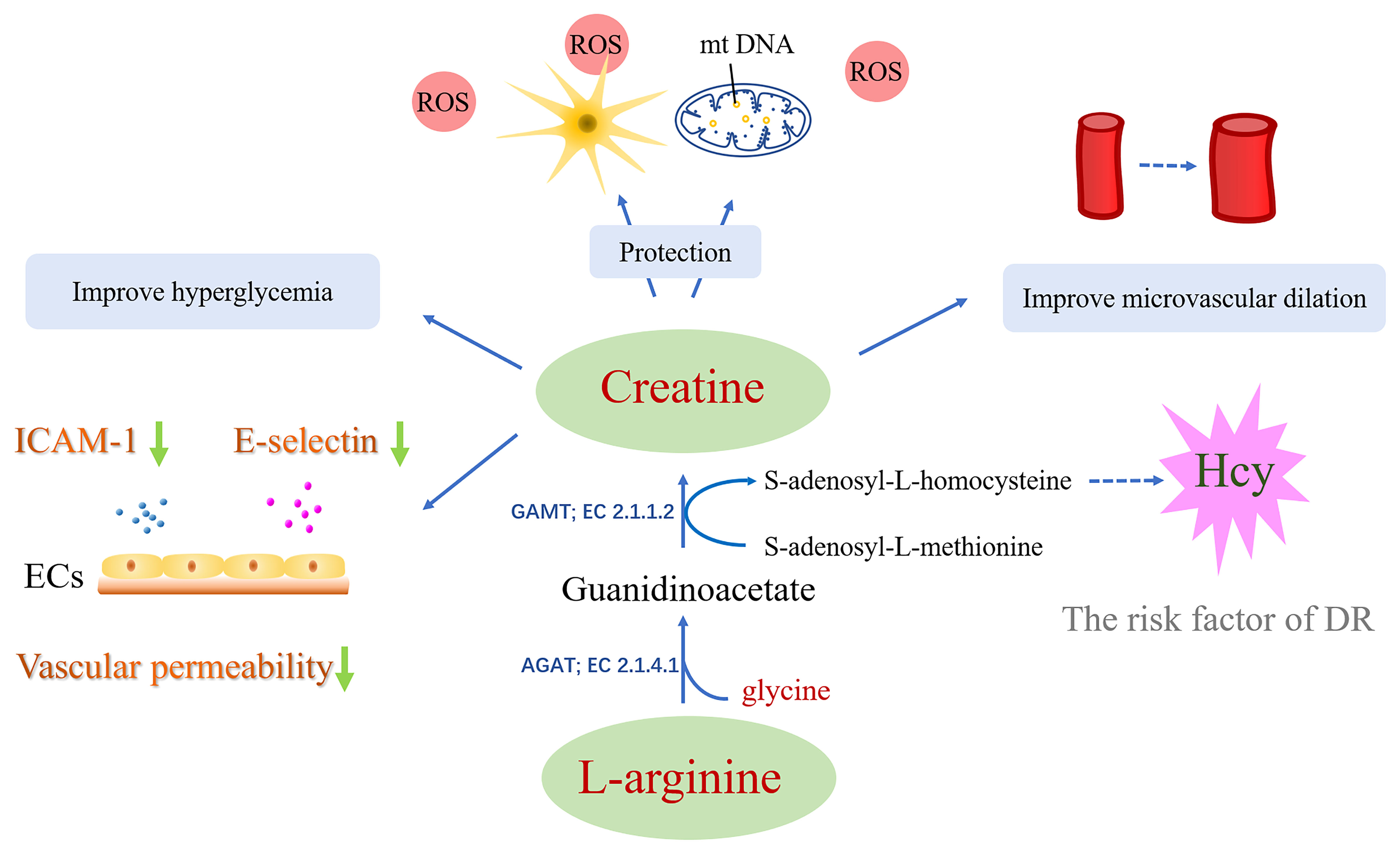
Figure 4 The approach of creatine in treating diabetic retinopathy: i) Creatine has the potential to act as an anti-inflammatory aid and provide vascular protection. ii) Creatine has a significant antioxidant effect and protects mtDNA and nerve cells from cytotoxicity induced by oxidative stress. iii) Creatine may help increase the bioavailability of epoxyeicosatrienoic acid, thereby improving microvascular dilation. iv) Creatine may reduce the formation of Hcy. v) Creatine supplementation can help improve hyperglycemia.
Conclusion
In recent years, researchers have identified many potential DR biomarkers, which are not yet used for clinical diagnosis. Further research is required to clarify their molecular mechanisms in DR. In this review, we have discussed the known biomarkers of diabetic retinopathy, which can help in predicting and preventing DR in the future. Furthermore, we suggest that the arginine-creatine metabolic pathway may be a new strategy for the treatment of diabetic retinopathy.
Author Contributions
YS, LK, A-HZ, YH, HS, and G-LY analyzed the data. YS wrote the paper. A-HZ and X-JW revised the paper. All the authors read and approved the final manuscript.
Funding
This work was supported by grants from the Key Program of Natural Science Foundation of State (Grant No. 81973745, 81830110), Natural Science Foundation of Heilongjiang Province (YQ2019H030), Heilongjiang Touyan Innovation Team Program.
Conflict of Interest
The authors declare that the research was conducted in the absence of any commercial or financial relationships that could be construed as a potential conflict of interest.
Publisher’s Note
All claims expressed in this article are solely those of the authors and do not necessarily represent those of their affiliated organizations, or those of the publisher, the editors and the reviewers. Any product that may be evaluated in this article, or claim that may be made by its manufacturer, is not guaranteed or endorsed by the publisher.
Abbreviation
DR, Diabetic retinopathy; T2DM, type 2 diabetes mellitus; PKC, protein kinase C; Cr, creatine; PCr, phosphocreatine; CK, creatine kinase; NMR, nuclear magnetic resonance; MS, mass spectrometry; LC, liquid chromatography; GC, gas chromatography; HILIC-MS, hydrophilic interaction chromatography-mass spectrometry; FIA–MS, Flow-injection analysis-mass spectrometry; CE, capillary electrophoresis; HPLC, high-performance liquid chromatography; UPLC, ultra-high performance liquid chromatography; CE, Capillary electrophoresis; PDR, proliferative diabetic retinopathy; OIR, oxygen induced ischemic retinopathy; GC-MS, gas chromatography mass spectrometry; UPLC-MS, ultra-performance liquid chromatography-mass spectrometry; TCA, tricarboxylic acid cycle; LC-MS, liquid chromatography-mass spectrometry; HILIC-MS Hydrophilic interaction chromatography-mass spectrometry; UPLC-Q-Axis Orbiter-MS, ultra-performance liquid chromatography-quadrupole-Exactive Orbitrap-mass spectrometry; IDO, indolamine-2,3-dioxygenase; UACR, albumin/creatinine; KEGG, Kyoto Encyclopedia of Genes and Genomes; NOS, nitric oxide synthase; NO, nitric oxide; EDHF, endothelium-derived hyperpolarizing factor; GAA, guanidinoacetate; Hcy, homocysteine; ECs, endothelial cells; EC, endothelial cell; LPC, Lysophosphatidylcholine; ICAM-1, increased intercellular cell adhesion molecule-1.
References
1. Cheung N, Mitchell P, Wong TY. Diabetic Retinopathy. Lancet (2010) 376(9735):124–36. doi: 10.1016/S0140-6736(09)62124-3
2. Rasmussen RN, Matsumoto A, Arvin S, Yonehara K. Binocular Integration of Retinal Motion Information Underlies Optic Flow Processing by the Cortex. Curr Biol (2021) 31(6):1165–74.e6. doi: 10.1016/j.cub.2020.12.034
3. Huang H. Pericyte-Endothelial Interactions in the Retinal Microvasculature. Int J Mol Sci (2020) 21(19):7413. doi: 10.3390/ijms21197413
4. Nakamachi T, Matkovits A, Seki T, Shioda S. Distribution and Protective Function of Pituitary Adenylate Cyclase-Activating Polypeptide in the Retina. Front Endocrinol (2012) 3:145. doi: 10.3389/fendo.2012.00145
5. Klaassen I, Van Noorden CJ, Schlingemann RO. Molecular Basis of the Inner Blood-Retinal Barrier and Its Breakdown in Diabetic Macular Edema and Other Pathological Conditions. Prog Retin Eye Res (2013) 34:19–48. doi: 10.1016/j.preteyeres.2013.02.001
6. Antonetti DA, Silva PS, Stitt AW. Current Understanding of the Molecular and Cellular Pathology of Diabetic Retinopathy. Nat Rev Endocrinol (2021) 17(4):195–206. doi: 10.1038/s41574-020-00451-4
7. Rudraraju M, Narayanan SP, Somanath PR. Regulation of Blood-Retinal Barrier Cell-Junctions in Diabetic Retinopathy. Pharmacol Res (2020) 161:105115. doi: 10.1016/j.phrs.2020.105115
8. Gui F, You Z, Fu S, Wu H, Zhang Y. Endothelial Dysfunction in Diabetic Retinopathy. Front Endocrinol (2020) 11:591. doi: 10.3389/fendo.2020.00591
9. Augustine J, Troendle EP, Barabas P, McAleese CA, Friedel T, Stitt AW, et al. The Role of Lipoxidation in the Pathogenesis of Diabetic Retinopathy. Front Endocrinol (2021) 11:621938. doi: 10.3389/fendo.2020.621938
10. Jang C, Chen L, Rabinowitz JD. Metabolomics and Isotope Tracing. Cell (2018) 173(4):822–37. doi: 10.1016/j.cell.2018.03.055
11. Rinschen MM, Ivanisevic J, Giera M, Siuzdak G. Identification of Bioactive Metabolites Using Activity Metabolomics. Nat Rev Mol Cell Biol (2019) 20(6):353–67. doi: 10.1038/s41580-019-0108-4
12. Wang X-q, Zhang A-h, Miao J-h, Sun H, Yan G-l, Wu F-f, et al. Gut Microbiota as Important Modulator of Metabolism in Health and Disease. RSC Adv (2018) 8:42380–9. doi: 10.1039/C8RA08094A
13. Schrimpe-Rutledge AC, Codreanu SG, Sherrod SD, McLean JA. Untargeted Metabolomics Strategies-Challenges and Emerging Directions. J Am Soc Mass Spectrom (2016) 27(12):1897–905. doi: 10.1007/s13361-016-1469-y
14. Liu C, Zong WJ, Zhang AH, Zhang HM, Luan YH, Sun H, et al. Lipidomic Characterisation Discovery for Coronary Heart Disease Diagnosis Based on High-Throughput Ultra-Performance Liquid Chromatography and Mass Spectrometry. RSC Adv (2018) 8(2):647–54. doi: 10.1039/C7RA09353E
15. Yan Y, Zhang A, Hui D, Yan G, Wang X. Toxicity and Detoxification Effects of Herbal Caowu via Ultra Performance Liquid Chromatography/Mass Spectrometry Metabolomics Analyzed Using Pattern Recognition Method. Pharmacognosy Magazine (2017) 13(52):683–92. doi: 10.4103/pm.pm_475_16
16. Zhang A, Fang H, Wang Y, Yan G, Sun H, Zhou X, et al. Discovery and Verification of the Potential Targets From Bioactive Molecules by Network Pharmacology-Based Target Prediction Combined With High-Throughput Metabolomics. RSC Adv (2017) 7(81):51069–78. doi: 10.1039/C7RA09522H
17. Zhao Q, Zhang A, Zong W, Na A, Zhang H, Luan Y, et al. Exploring Potential Biomarkers and Determining the Metabolic Mechanism of Type 2 Diabetes Mellitus Using Liquid Chromatography Coupled to High-Resolution Mass Spectrometry. RSC Adv (2017) 7(70):44186–98. doi: 10.1039/C7RA05722A
18. Bujak R, Struck-Lewicka W, Markuszewski MJ, Kaliszan R. Metabolomics for Laboratory Diagnostics. J Pharm BioMed Anal (2015) 113:108–20. doi: 10.1016/j.jpba.2014.12.017
19. Johnson CH, Ivanisevic J, Siuzdak G. Metabolomics: Beyond Biomarkers and Towards Mechanisms. Nat Rev Mol Cell Biol (2016) 17(7):451–9. doi: 10.1038/nrm.2016.25
20. Zhang HL, Zhang AH, Zhou XH, Sun H, Wang XQ, Liang L, et al. High-Throughput Lipidomics Reveal Mirabilite Regulating Lipid Metabolism as Anticancer Therapeutics. RSC Adv (2018) 8(62):35600–10. doi: 10.1039/C8RA06190D
21. Umeda M, Hiramoto M, Watanabe A, Tsunoda N, Imai T. Arginine-Induced Insulin Secretion in Endoplasmic Reticulum. Biochem Biophys Res Commun (2015) 466(4):717–22. doi: 10.1016/j.bbrc.2015.09.006
22. Rajendran S, Shen X, Glawe J, Kolluru GK, Kevil CG. Nitric Oxide and Hydrogen Sulfide Regulation of Ischemic Vascular Growth and Remodeling. Compr Physiol (2019) 9(3):1213–47. doi: 10.1002/cphy.c180026
23. Lowenstein CJ. Metabolism Reprogrammed by the Nitric Oxide Signalling Molecule. Nature (2019) 565(7737):33–4. doi: 10.1038/d41586-018-07457-z
24. Clarke H, Kim D-H, Meza CA, Ormsbee MJ, Hickner RC. The Evolving Applications of Creatine Supplementation: Could Creatine Improve Vascular Health? Nutrients (2020) 12(9):2834. doi: 10.3390/nu12092834
25. Roberts R, Sobel BE, Parker CW. Radioimmunoassay for Creatine Kinase Isoenzymes. Science (1976) 194(4267):855–7. doi: 10.1126/science.982049
26. Butts J, Jacobs B, Silvis M. Creatine Use in Sports. Sports Health (2018) 10(1):31–4. doi: 10.1177/1941738117737248
27. Yeh HI, Chen IJ, Teng CM. Cyclooxygenase–Independent Pathway of Phospholipase Activation in Carrageenan–Induced Platelet Aggregation. Thromb Res (1987) 45(1):39–49. doi: 10.1016/0049-3848(87)90255-6
28. Nomura A, Zhang M, Sakamoto T, Ishii Y, Morishima Y, Mochizuki M, et al. Anti-Inflammatory Activity of Creatine Supplementation in Endothelial Cells In Vitro. Br J Pharmacol (2003) 139(4):715–20. doi: 10.1038/sj.bjp.0705316
29. Candow DG, Forbes SC, Chilibeck PD, Cornish SM, Antonio J, Kreider RB. Effectiveness of Creatine Supplementation on Aging Muscle and Bone: Focus on Falls Prevention and Inflammation. J Clin Med (2019) 8(4):488. doi: 10.3390/jcm8040488
30. Béard E, Braissant O. Synthesis and Transport of Creatine in the CNS: Importance for Cerebral Functions. J Neurochem (2010) 115(2):297–313. doi: 10.1111/j.1471-4159.2010.06935.x
31. Marques EP, Ferreira FS, Santos TM, Prezzi CA, Martins LAM, Bobermin LD, et al. Cross-Talk Between Guanidinoacetate Neurotoxicity, Memory and Possible Neuroprotective Role of Creatine. Biochim Biophys Acta Mol Basis Dis (2019) 1865(11):165529. doi: 10.1016/j.bbadis.2019.08.005
32. Lawler JM, Barnes WS, Wu G, Song W, Demaree S. Direct Antioxidant Properties of Creatine. Biochem Biophys Res Commun (2002) 290(1):47–52. doi: 10.1006/bbrc.2001.6164
33. Sestili P, Martinelli C, Colombo E, Barbieri E, Potenza L, Sartini S, et al. Creatine as an Antioxidant. Amino Acids (2011) 40(5):1385–96. doi: 10.1007/s00726-011-0875-5
34. Ferrante RJ, Andreassen OA, Jenkins BG, Dedeoglu A, Kuemmerle S, Kubilus JK, et al. Neuroprotective Effects of Creatine in a Transgenic Mouse Model of Huntington’s Disease. J neuroscience: Off J Soc Neurosci (2000) 20(12):4389–97. doi: 10.1523/JNEUROSCI.20-12-04389.2000
35. Van Bavel D, de Moraes R, Tibirica E. Effects of Dietary Supplementation With Creatine on Homocysteinemia and Systemic Microvascular Endothelial Function in Individuals Adhering to Vegan Diets. Fundam Clin Pharmacol (2019) 33(4):428–40. doi: 10.1111/fcp.12442
36. Korzun WJ. Oral Creatine Supplements Lower Plasma Homocysteine Concentrations in Humans. Clin Lab Sci (2004) 17(2):102–6.
37. Bereket-Yücel S. Creatine Supplementation Alters Homocysteine Level in Resistance Trained Men. J Sports Med Phys Fitness (2015) 55(4):313–9.
38. Emwas AH. The Strengths and Weaknesses of NMR Spectroscopy and Mass Spectrometry With Particular Focus on Metabolomics Research. Methods Mol Biol (2015) 1277:161–93. doi: 10.1007/978-1-4939-2377-9_13
39. Zhang AH, Sun H, Yan GL, Han Y, Zhao QQ, Wang XJ. Chinmedomics: A Powerful Approach Integrating Metabolomics With Serum Pharmacochemistry to Evaluate the Efficacy of Traditional Chinese Medicine. Engineering (2019) 5(1):60–8. doi: 10.1016/j.eng.2018.11.008
40. Zhang A, Sun H, Wang X. Mass Spectrometry-Driven Drug Discovery for Development of Herbal Medicine. Mass Spectrom Rev (2018) 37(3):307–20. doi: 10.1002/mas.21529
41. Xie J, Zhang A, Wang X. Metabolomic Applications in Hepatocellular Carcinoma: Toward the Exploration of Therapeutics and Diagnosis Through Small Molecules. RSC Adv (2017) 7(28):17217–26. doi: 10.1039/C7RA00698E
42. Marshall DD, Powers R. Beyond the Paradigm: Combining Mass Spectrometry and Nuclear Magnetic Resonance for Metabolomics. Prog Nucl Magn Reson Spectrosc (2017) 100:1–16. doi: 10.1016/j.pnmrs.2017.01.001
43. Swain D, Samanthula G. Study on the Forced Degradation Behaviour of Ledipasvir: Identification of Major Degradation Products Using LC-QTOF-MS/MS and NMR. J Pharm BioMed Anal (2017) 138:29–42. doi: 10.1016/j.jpba.2017.01.033
44. Ha TJ, Park JE, Lee KS, Seo WD, Song SB, Lee MH, et al. Identification of Anthocyanin Compositions in Black Seed Coated Korean Adzuki Bean (Vigna Angularis) by NMR and UPLC-Q-Orbitrap-MS/MS and Screening for Their Antioxidant Properties Using Different Solvent Systems. Food Chem (2021) 346:128882. doi: 10.1016/j.foodchem.2020.128882
45. Farag MA, Shakour ZT, Lübken T, Frolov A, Wessjohann LA, Mahrous E. Unraveling the Metabolome Composition and Its Implication for Salvadora Persica L. Use as Dental Brush via a Multiplex Approach of NMR and LC-MS Metabolomics. J Pharm BioMed Anal (2021) 193:113727. doi: 10.1016/j.jpba.2020.113727
46. Duley G, Dujourdy L, Klein S, Werwein A, Spartz C, Gougeon RD, et al. Regionality in Australian Pinot Noir Wines: A Study on the Use of NMR and ICP-MS on Commercial Wines. Food Chem (2021) 340:127906. doi: 10.1016/j.foodchem.2020.127906
47. Barnes S, Benton HP, Casazza K, Cooper SJ, Cui X, Du X, et al. Training in Metabolomics Research. I. Designing the Experiment, Collecting and Extracting Samples and Generating Metabolomics Data. J Mass Spectrom (2016) 51(7):ii–iii. doi: 10.1002/jms.3672
48. Grootveld M, Percival B, Gibson M, Osman Y, Edgar M, Molinari M, et al. Progress in Low-Field Benchtop NMR Spectroscopy in Chemical and Biochemical Analysis. Anal Chim Acta (2019) 1067:11–30. doi: 10.1016/j.aca.2019.02.026
49. Chen L, Cheng C-Y, Choi H, Ikram MK, Sabanayagam C, Tan GSW, et al. Plasma Metabonomic Profiling of Diabetic Retinopathy. Diabetes (2016) 65(4):1099–108. doi: 10.2337/db15-0661
50. Broft P, Dzatko S, Krafcikova M, Wacker A, Hänsel-Hertsch R, Dötsch V, et al. In-Cell NMR Spectroscopy of Functional Riboswitch Aptamers in Eukaryotic Cells. Angew Chem Int Ed Engl (2021) 60(2):865–72. doi: 10.1002/anie.202007184
51. Singh A. Atomic-Level in-Cell Protein NMR. Nat Methods (2019) 16(8):676. doi: 10.1038/s41592-019-0525-5
52. Holmes E, Wijeyesekera A, Taylor-Robinson SD, Nicholson JK. The Promise of Metabolic Phenotyping in Gastroenterology and Hepatology. Nat Rev Gastroenterol Hepatol (2015) 12(8):458–71. doi: 10.1038/nrgastro.2015.114
53. Kohler I, Verhoeven A, Derks RJ, Giera M. Analytical Pitfalls and Challenges in Clinical Metabolomics. Bioanalysis (2016) 8(14):1509–32. doi: 10.4155/bio-2016-0090
54. Sun H, Zhang A-H, Liu S-B, Qiu S, Li XN, Zhang TL, et al. Cell Metabolomics Identify Regulatory Pathways and Targets of Magnoline Against Prostate Cancer. J Chromatogr B Analytical Technol Biomed Life Sci (2018) 1102-1103:143–51. doi: 10.1016/j.jchromb.2018.10.017
55. Song Q, Zhang AH, Yan GL, Liu L, Wang XJ. Technological Advances in Current Metabolomics and Its Application in Tradition Chinese Medicine. RSC Adv (2017) 7(84):53516–24. doi: 10.1039/C7RA02056B
56. Li XN, Zhang A, Wang M, Sun H, Liu Z, Qiu S, et al. Screening the Active Compounds of Phellodendri Amurensis Cortex for Treating Prostate Cancer by High-Throughput Chinmedomics. Sci Rep (2017) 7:46234. doi: 10.1038/srep46234
57. Wang XJ, Zhang AH, Kong L, Yu JB, Gao HL, Liu ZD, et al. Rapid Discovery of Quality-Markers From Kaixin San Using Chinmedomics Analysis Approach. Phytomedicine (2019) 54:371–81. doi: 10.1016/j.phymed.2017.12.014
58. Ren JL, Dong H, Han Y, Yang L, Zhang AH, Sun H, et al. Network Pharmacology Combined With Metabolomics Approach to Investigate the Protective Role and Detoxification Mechanism of Yunnan Baiyao Formulation. Phytomedicine (2020) 77:153266. doi: 10.1016/j.phymed.2020.153266
59. Shi Q, Zhang A, Zhang T, Hui S, Wang X. Dissect New Mechanistic Insights for Geniposide Efficacy on the Hepatoprotection Using Multiomics Approach. Oncotarget (2017) 8(65):108760–70. doi: 10.18632/oncotarget.21897
60. Zhou Y, Qin Q, Zhang PW, Chen XT, Liu BJ, Cheng DM, et al. Integrated LC-MS and GC-MS-Based Untargeted Metabolomics Studies of the Effect of Azadirachtin on Bactrocera Dorsalis Larvae. Sci Rep (2020) 10(1):2306. doi: 10.1038/s41598-020-58796-9
61. Wu H, Xu C, Gu Y, Yang S, Wang Y, Wang C. An Improved Pseudotargeted GC-MS/MS-Based Metabolomics Method and Its Application in Radiation-Induced Hepatic Injury in a Rat Model. J Chromatogr B Analyt Technol BioMed Life Sci (2020) 1152:122250. doi: 10.1016/j.jchromb.2020.122250
62. Paris LP, Johnson CH, Aguilar E, Usui Y, Cho K, Hoang LT, et al. Global Metabolomics Reveals Metabolic Dysregulation in Ischemic Retinopathy. Metabolomics (2016) 12:15–. doi: 10.1007/s11306-015-0877-5
63. Yun JH, Kim JM, Jeon HJ, Oh T, Choi HJ, Kim BJ. Metabolomics Profiles Associated With Diabetic Retinopathy in Type 2 Diabetes Patients. PloS One (2020) 15(10):e0241365. doi: 10.1371/journal.pone.0241365
64. Zhang W, Ramautar R. CE-MS for Metabolomics: Developments and Applications in the Period 2018-2020. Electrophoresis (2021) 42(4):381–401. doi: 10.1002/elps.202000203
65. Wells SS, Dawod M, Kennedy RT. CE-MS With Electrokinetic Supercharging and Application to Determination of Neurotransmitters. Electrophoresis (2019) 40(22):2946–53. doi: 10.1002/elps.201900203
66. Khamis MM, Adamko DJ, El-Aneed A. Mass Spectrometric Based Approaches in Urine Metabolomics and Biomarker Discovery. Mass Spectrom Rev (2017) 36(2):115–34. doi: 10.1002/mas.21455
67. Liu SB, Lu SW, Sun H, Zhang AH, Wang H, Wei WF, et al. Deciphering the Q-Markers of Nourishing Kidney-Yin of Cortex Phellodendri Amurense From Zhibaidihuang Pill Based on Chinmedomics Strategy. Phytomedicine (2021) 91:153690. doi: 10.1016/j.phymed.2021.153690
68. Hui S, Zhang HL, Zhang AH, Zhou XH, Wang XQ, Ying H, et al. Network Pharmacology Combined With Functional Metabolomics Discover Bile Acid Metabolism as a Promising Target for Mirabilite Against Colorectal Cancer. RSC Adv (2018) 8(53):30061–70. doi: 10.1039/C8RA04886J
69. Liu XY, Zhang AH, Fang H, Li MX, Song Q, Su J, et al. Serum Metabolomics Strategy for Understanding the Therapeutic Effects of Yin-Chen-Hao-Tang Against Yanghuang Syndrome. RSC Adv (2018) 8(14):7403–13. doi: 10.1039/C7RA11048K
70. Korban A, Charapitsa S, Čabala R, Sobolenko L, Egorov V, Sytova S. Advanced GC-MS Method for Quality and Safety Control of Alcoholic Products. Food Chem (2021) 338:128107. doi: 10.1016/j.foodchem.2020.128107
71. Konášová R, Koval D, Hošek J, Kašička V. Investigating the Position of the Separation Capillary and Emitter Tube Tips in a Nanoflow Sheath-Liquid CE-ESI-MS Interface to Decouple the ESI Potential. Talanta (2021) 228:122212. doi: 10.1016/j.talanta.2021.122212
72. Höcker O, Knierman M, Meixner J, Neusüß C. Two Capillary Approach for a Multifunctional Nanoflow Sheath Liquid Interface for Capillary Electrophoresis-Mass Spectrometry. Electrophoresis (2021) 42(4):369–73. doi: 10.1002/elps.202000169
73. Gao HL, Zhang AH, Yu JB, Sun H, Kong L, Wang XQ, et al. High-Throughput Lipidomics Characterize Key Lipid Molecules as Potential Therapeutic Targets of Kaixinsan Protects Against Alzheimer’s Disease in APP/PS1 Transgenic Mice. J Chromatogr B Analyt Technol BioMed Life Sci (2018) 1092:286–95. doi: 10.1016/j.jchromb.2018.06.032
74. Sun H, Zhang AH, Yang L, Li MX, Fang H, Xie J, et al. High-Throughput Chinmedomics Strategy for Discovering the Quality-Markers and Potential Targets for Yinchenhao Decoction. Phytomedicine (2019) 54:328–38. doi: 10.1016/j.phymed.2018.04.015
75. Buziau AM, Scheijen J, Stehouwer CDA, Simons N, Brouwers M, Schalkwijk CG. Development and Validation of a UPLC-MS/MS Method to Quantify Fructose in Serum and Urine. J Chromatogr B Analyt Technol BioMed Life Sci (2020) 1155:122299. doi: 10.1016/j.jchromb.2020.122299
76. Fang H, Zhang AH, Sun H, Yu JB, Wang L, Wang XJ. High-Throughput Metabolomics Screen Coupled With Multivariate Statistical Analysis Identifies Therapeutic Targets in Alcoholic Liver Disease Rats Using Liquid Chromatography-Mass Spectrometry. J Chromatogr B Analyt Technol BioMed Life Sci (2019) 1109:112–20. doi: 10.1016/j.jchromb.2019.01.017
77. Ren JL, Zhang AH, Ling K, Wang XJ. Advances in Mass Spectrometry-Based Metabolomics for Investigation of Metabolites. Rsc Adv (2018) 8(40):22335–50. doi: 10.1039/C8RA01574K
78. Lu S, Han Y, Chu H, Kong L, Zhang A, Yan G, et al. Characterizing Serum Metabolic Alterations of Alzheimer’s Disease and Intervention of Shengmai-San by Ultra-Performance Liquid Chromatography/Electrospray Ionization Quadruple Time-of-Flight Mass Spectrometry. Food Funct (2017) 8(4):1660–71. doi: 10.1039/C7FO00154A
79. Wang XJ, Ren JL, Zhang AH, Sun H, Yan GL, Han Y, et al. Novel Applications of Mass Spectrometry-Based Metabolomics in Herbal Medicines and Its Active Ingredients: Current Evidence. Mass Spectrom Rev (2019) 38(4-5):380–402. doi: 10.1002/mas.21589
80. Zhang AH, Ma ZM, Sun H, Zhang Y, Liu JH, Wu FF, et al. High-Throughput Metabolomics Evaluate the Efficacy of Total Lignans From Acanthophanax Senticosus Stem Against Ovariectomized Osteoporosis Rat. Front Pharmacol (2019) 10:553. doi: 10.3389/fphar.2019.00553
81. Zhang AH, Yu JB, Sun H, Kong L, Wang XQ, Zhang QY, et al. Identifying Quality-Markers From Shengmai San Protects Against Transgenic Mouse Model of Alzheimer’s Disease Using Chinmedomics Approach. Phytomedicine (2018) 45:84–92. doi: 10.1016/j.phymed.2018.04.004
82. Sun H, Yang L, Li MX, Fang H, Zhang AH, Song Q, et al. UPLC-G2Si-HDMS Untargeted Metabolomics for Identification of Metabolic Targets of Yin-Chen-Hao-Tang Used as a Therapeutic Agent of Dampness-Heat Jaundice Syndrome. J Chromatogr B Analyt Technol BioMed Life Sci (2018) 1081-1082:41–50. doi: 10.1016/j.jchromb.2018.02.035
83. Han Y, Zhang AH, Zhang YZ, Sun H, Meng XC, Wang XJ. Chemical Metabolomics for Investigating the Protective Effectiveness of Acanthopanax Senticosus Harms Leaf Against Acute Promyelocytic Leukemia. Rsc Adv (2018) 8(22):11983–90. doi: 10.1039/C8RA01029C
84. Tomita Y, Cagnone G, Fu Z, Cakir B, Kotoda Y, Asakage M, et al. Vitreous Metabolomics Profiling of Proliferative Diabetic Retinopathy. Diabetologia (2021) 64(1):70–82. doi: 10.1007/s00125-020-05309-y
85. Wang H, Fang J, Chen F, Sun Q, Xu X, Lin SH, et al. Metabolomic Profile of Diabetic Retinopathy: A GC-TOFMS-Based Approach Using Vitreous and Aqueous Humor. Acta Diabetol (2020) 57(1):41–51. doi: 10.1007/s00592-019-01363-0
86. Haines NR, Manoharan N, Olson JL, D’Alessandro A, Reisz JA. Metabolomics Analysis of Human Vitreous in Diabetic Retinopathy and Rhegmatogenous Retinal Detachment. J Proteome Res (2018) 17(7):2421–7. doi: 10.1021/acs.jproteome.8b00169
87. Barba I, Garcia-Ramírez M, Hernández C, Alonso MA, Masmiquel L, García-Dorado D, et al. Metabolic Fingerprints of Proliferative Diabetic Retinopathy: An 1H-NMR-Based Metabonomic Approach Using Vitreous Humor. Invest Ophthalmol Vis Sci (2010) 51(9):4416–21. doi: 10.1167/iovs.10-5348
88. Rhee SY, Jung ES, Park HM, Jeong SJ, Kim K, Chon S, et al. Plasma Glutamine and Glutamic Acid Are Potential Biomarkers for Predicting Diabetic Retinopathy. Metabolomics (2018) 14(7):89. doi: 10.1007/s11306-018-1383-3
89. Curovic VR, Suvitaival T, Mattila I, Ahonen L, Trošt K, Theilade S, et al. Circulating Metabolites and Lipids Are Associated to Diabetic Retinopathy in Individuals With Type 1 Diabetes. Diabetes (2020) 69(10):2217–26. doi: 10.2337/db20-0104
90. Sumarriva K, Uppal K, Ma C, Herren DJ, Wang Y, Chocron IM, et al. Arginine and Carnitine Metabolites Are Altered in Diabetic Retinopathy. Invest Ophthalmol Vis Sci (2019) 60(8):3119–26. doi: 10.1167/iovs.19-27321
91. Li X, Luo X, Lu X, Duan J, Xu G. Metabolomics Study of Diabetic Retinopathy Using Gas Chromatography-Mass Spectrometry: A Comparison of Stages and Subtypes Diagnosed by Western and Chinese Medicine. Mol Biosyst (2011) 7(7):2228–37. doi: 10.1039/c0mb00341g
92. Xuan Q, Zheng F, Yu D, Ouyang Y, Zhao X, Hu C, et al. Rapid Lipidomic Profiling Based on Ultra-High Performance Liquid Chromatography-Mass Spectrometry and Its Application in Diabetic Retinopathy. Anal Bioanal Chem (2020) 412(15):3585–94. doi: 10.1007/s00216-020-02632-6
93. Xuan Q, Ouyang Y, Wang Y, Wu L, Li H, Luo Y, et al. Multiplatform Metabolomics Reveals Novel Serum Metabolite Biomarkers in Diabetic Retinopathy Subjects. Adv Sci (Weinh) (2020) 7(22):2001714–. doi: 10.1002/advs.202001714
94. Elmasry K, Ibrahim AS, Saleh H, Elsherbiny N, Elshafey S, Hussein KA, et al. Role of Endoplasmic Reticulum Stress in 12/15-Lipoxygenase-Induced Retinal Microvascular Dysfunction in a Mouse Model of Diabetic Retinopathy. Diabetologia (2018) 61(5):1220–32. doi: 10.1007/s00125-018-4560-z
95. Ibrahim AS, Elshafey S, Sellak H, Hussein KA, El-Sherbiny M, Abdelsaid M, et al. A Lipidomic Screen of Hyperglycemia-Treated Hrecs Links 12/15-Lipoxygenase to Microvascular Dysfunction During Diabetic Retinopathy via NADPH Oxidase. J Lipid Res (2015) 56(3):599–611. doi: 10.1194/jlr.M056069
96. Othman A, Ahmad S, Megyerdi S, Mussell R, Choksi K, Maddipati KR, et al. 12/15-Lipoxygenase-Derived Lipid Metabolites Induce Retinal Endothelial Cell Barrier Dysfunction: Contribution of NADPH Oxidase. PloS One (2013) 8(2):e57254–e. doi: 10.1371/journal.pone.0057254
97. Zuo J, Lan Y, Hu H, Hou X, Li J, Wang T, et al. Metabolomics-Based Multidimensional Network Biomarkers for Diabetic Retinopathy Identification in Patients With Type 2 Diabetes Mellitus. BMJ Open Diabetes Res Care (2021) 9(1):e001443. doi: 10.1136/bmjdrc-2020-001443
98. Zhu X-R, Yang F-Y, Lu J, Zhang H-R, Sun R, Zhou J-B, et al. Plasma Metabolomic Profiling of Proliferative Diabetic Retinopathy. Nutr Metab (Lond) (2019) 16:37–. doi: 10.1186/s12986-019-0358-3
99. Munipally PK, Agraharm SG, Valavala VK, Gundae S, Turlapati NR. Evaluation of Indoleamine 2,3-Dioxygenase Expression and Kynurenine Pathway Metabolites Levels in Serum Samples of Diabetic Retinopathy Patients. Arch Physiol Biochem (2011) 117(5):254–8. doi: 10.3109/13813455.2011.623705
100. Jin H, Zhu B, Liu X, Jin J, Zou H. Metabolic Characterization of Diabetic Retinopathy: An (1)H-NMR-Based Metabolomic Approach Using Human Aqueous Humor. J Pharm BioMed Anal (2019) 174:414–21. doi: 10.1016/j.jpba.2019.06.013
101. Nishio A, Rehermann B. Virus-Induced Interferon Regulates the Urea Cycle. Immunity (2019) 51(6):975–7. doi: 10.1016/j.immuni.2019.11.012
102. Peters KS, Rivera E, Warden C, Harlow PA, Mitchell SL, Calcutt MW, et al. Plasma Arginine and Citrulline Are Elevated in Diabetic Retinopathy. Am J Ophthalmol (2021) 235:154–62. doi: 10.1016/j.ajo.2021.09.021
103. Tejero J, Hunt AP, Santolini J, Lehnert N, Stuehr DJ. Mechanism and Regulation of Ferrous Heme-Nitric Oxide (NO) Oxidation in NO Synthases. J Biol Chem (2019) 294(19):7904–16. doi: 10.1074/jbc.RA119.007810
104. Palmer JP, Benson JW, Walter RM, Ensinck JW. Arginine-Stimulated Acute Phase of Insulin and Glucagon Secretion in Diabetic Subjects. J Clin Invest (1976) 58(3):565–70. doi: 10.1172/JCI108502
105. Narayanan SP, Rojas M, Suwanpradid J, Toque HA, Caldwell RW, Caldwell RB. Arginase in Retinopathy. Prog Retin Eye Res (2013) 36:260–80. doi: 10.1016/j.preteyeres.2013.06.002
106. Patel C, Rojas M, Narayanan SP, Zhang W, Xu Z, Lemtalsi T, et al. Arginase as a Mediator of Diabetic Retinopathy. Front Immunol (2013) 4:173. doi: 10.3389/fimmu.2013.00173
107. Magri E, Baldoni G, Grazi E. On the Biosynthesis of Creatine. Intramitochondrial Localization of Transamidinase From Rat Kidney. FEBS Lett (1975) 55(1):91–3. doi: 10.1016/0014-5793(75)80966-5
108. Gerber GB, Koszalka TR, Gerber G, Altman KI. Biosynthesis of Creatine by the Kidney. Nature (1962) 196(4851):286–7. doi: 10.1038/196286a0
109. da Silva RP, Clow K, Brosnan JT, Brosnan ME. Synthesis of Guanidinoacetate and Creatine From Amino Acids by Rat Pancreas. Br J Nutr (2014) 111(4):571–7. doi: 10.1017/S0007114513003012
110. Sandberg AA, Hecht HH, Tyler FH. Studies in Disorders of Muscle. X. The Site of Creatine Synthesis in the Human. Metabolism (1953) 2(1):22–9.
111. Wyss M, Braissant O, Pischel I, Salomons GS, Schulze A, Stockler S, et al. Creatine and Creatine Kinase in Health and Disease–a Bright Future Ahead? Subcell Biochem (2007) 46:309–34. doi: 10.1007/978-1-4020-6486-9_16.
112. Wallimann T, Tokarska-Schlattner M, Schlattner U. The Creatine Kinase System and Pleiotropic Effects of Creatine. Amino Acids (2011) 40(5):1271–96. doi: 10.1007/s00726-011-0877-3
113. Markus W, Rima KD. Creatine and Creatinine Metabolism. Physiol Rev (2000) 80(3):1107–213. doi: 10.1152/physrev.2000.80.3.1107
114. Guthmiller P, Pilsum J, Boen JR, Mcguire DM. Cloning and Sequencing of Rat Kidney L-Arginine:Glycine Amidinotransferase. Studies on the Mechanism of Regulation by Growth Hormone and Creatine. J Biol Chem (1994) 269(26):17556–60. doi: 10.1016/S0021-9258(17)32477-8
115. Walker JB. Creatine: Biosynthesis, Regulation, and Function. Adv Enzymol Relat Areas Mol Biol (1979) 50:177–242. doi: 10.1002/9780470122952.ch4
116. Takeda M, Kiyatake I, Koide H, Jung KY, Endou H. Biosynthesis of Guanidinoacetic Acid in Isolated Renal Tubules. Eur J Clin Chem Clin Biochem (1992) 30(6):325–31. doi: 10.1515/cclm.1992.30.6.325
117. Hanna-El-Daher L, Béard E, Henry H, Tenenbaum L, Braissant O. Mild Guanidinoacetate Increase Under Partial Guanidinoacetate Methyltransferase Deficiency Strongly Affects Brain Cell Development. Neurobiol Dis (2015) 79:14–27. doi: 10.1016/j.nbd.2015.03.029
118. Gualano B, Painelli V, Roschel H, Lugaresi R, Dorea E, Artioli GG, et al. Creatine Supplementation Does Not Impair Kidney Function in Type 2 Diabetic Patients: A Randomized, Double-Blind, Placebo-Controlled, Clinical Trial. Eur J Appl Physiol (2011) 111(5):749–56. doi: 10.1007/s00421-010-1676-3
119. Ramakrishnan S, Sulochana KN. Decrease in Glycation of Lens Proteins by Lysine and Glycine by Scavenging of Glucose and Possible Mitigation of Cataractogenesis. Exp Eye Res (1993) 57(5):623–8. doi: 10.1006/exer.1993.1167
120. Ramakrishnan S, Sulochana KN, Punitham R. Free Lysine, Glycine, Alanine, Glutamic Acid and Aspartic Acid Reduce the Glycation of Human Lens Proteins by Galactose. Indian J Biochem Biophys (1997) 34(6):518–23. doi: 10.1007/BF00731438
121. Vidhya S, Ramya R, Coral K, Sulochana KN, Bharathidevi SR. Free Amino Acids Hydroxyproline, Lysine, and Glycine Promote Differentiation of Retinal Pericytes to Adipocytes: A Protective Role Against Proliferative Diabetic Retinopathy. Exp Eye Res (2018) 173:179–87. doi: 10.1016/j.exer.2018.05.004
122. Kowluru RA, Mishra M. Therapeutic Targets for Altering Mitochondrial Dysfunction Associated With Diabetic Retinopathy. Expert Opin Ther Targets (2018) 22(3):233–45. doi: 10.1080/14728222.2018.1439921
123. Miller DJ, Cascio MA, Rosca MG. Diabetic Retinopathy: The Role of Mitochondria in the Neural Retina and Microvascular Disease. Antioxidants (Basel) (2020) 9(10):905. doi: 10.3390/antiox9100905
124. Han WH, Gotzmann J, Kuny S, Huang H, Chan CB, Lemieux H, et al. Modifications in Retinal Mitochondrial Respiration Precede Type 2 Diabetes and Protracted Microvascular Retinopathy. Invest Ophthalmol Vis Sci (2017) 58(10):3826–39. doi: 10.1167/iovs.17-21929
125. Moraes R, Van Bavel D, Moraes BS, Tibiriçá E. Effects of Dietary Creatine Supplementation on Systemic Microvascular Density and Reactivity in Healthy Young Adults. Nutr J (2014) 13(1):115. doi: 10.1186/1475-2891-13-115
126. Matthews RT, Yang L, Jenkins BG, Ferrante RJ, Rosen BR, Kaddurah-Daouk R, et al. Neuroprotective Effects of Creatine and Cyclocreatine in Animal Models of Huntington’s Disease. J Neurosci (1998) 18(1):156–63. doi: 10.1523/JNEUROSCI.18-01-00156.1998
127. Kazak L, Chouchani ET, Jedrychowski MP, Erickson BK, Shinoda K, Cohen P, et al. A Creatine-Driven Substrate Cycle Enhances Energy Expenditure and Thermogenesis in Beige Fat. Cell (2015) 163(3):643–55. doi: 10.1016/j.cell.2015.09.035
128. Maresca A, Del Dotto V, Romagnoli M, La Morgia C, Di Vito L, Capristo M, et al. Expanding and Validating the Biomarkers for Mitochondrial Diseases. J Mol Med (Berl) (2020) 98(10):1467–78. doi: 10.1007/s00109-020-01967-y
129. Tawfik A, Samra YA, Elsherbiny NM, Al-Shabrawey M. Implication of Hyperhomocysteinemia in Blood Retinal Barrier (BRB) Dysfunction. Biomolecules (2020) 10(8):1119. doi: 10.3390/biom10081119
130. Elsherbiny NM, Sharma I, Kira D, Alhusban S, Samra YA, Jadeja R, et al. Homocysteine Induces Inflammation in Retina and Brain. Biomolecules (2020) 10(3):393. doi: 10.3390/biom10030393
131. Kowluru RA. Diabetic Retinopathy: Mitochondria Caught in a Muddle of Homocysteine. J Clin Med (2020) 9(9):3019. doi: 10.3390/jcm9093019
132. Deminice R, Cella PS, Padilha CS, Borges FH, da Silva LE, Campos-Ferraz PL, et al. Creatine Supplementation Prevents Hyperhomocysteinemia, Oxidative Stress and Cancer-Induced Cachexia Progression in Walker-256 Tumor-Bearing Rats. Amino Acids (2016) 48(8):2015–24. doi: 10.1007/s00726-016-2172-9
133. McCarty MF. Supplemental Creatine may Decrease Serum Homocysteine and Abolish the Homocysteine ‘Gender Gap’ by Suppressing Endogenous Creatine Synthesis. Med Hypotheses (2001) 56(1):5–7. doi: 10.1054/mehy.1999.1014
134. Brosnan JT, Silva R, Brosnan ME. The Metabolic Burden of Creatine Synthesis. Amino Acids (2011) 40(5):1325–31. doi: 10.1007/s00726-011-0853-y
Keywords: diabetic retinopathy, metabolomics, biomarker, creatine, arginine, mechanism
Citation: Sun Y, Kong L, Zhang A-H, Han Y, Sun H, Yan G-L and Wang X-J (2022) A Hypothesis From Metabolomics Analysis of Diabetic Retinopathy: Arginine-Creatine Metabolic Pathway May Be a New Treatment Strategy for Diabetic Retinopathy. Front. Endocrinol. 13:858012. doi: 10.3389/fendo.2022.858012
Received: 19 January 2022; Accepted: 01 March 2022;
Published: 24 March 2022.
Edited by:
Undurti Narasimha Das, UND Life Sciences LLC, United StatesReviewed by:
Yohei Tomita, Boston Children’s Hospital and Harvard Medical School, United StatesJihong Lin, Heidelberg University, Germany
Copyright © 2022 Sun, Kong, Zhang, Han, Sun, Yan and Wang. This is an open-access article distributed under the terms of the Creative Commons Attribution License (CC BY). The use, distribution or reproduction in other forums is permitted, provided the original author(s) and the copyright owner(s) are credited and that the original publication in this journal is cited, in accordance with accepted academic practice. No use, distribution or reproduction is permitted which does not comply with these terms.
*Correspondence: Xi-Jun Wang, xijunw@sina.com