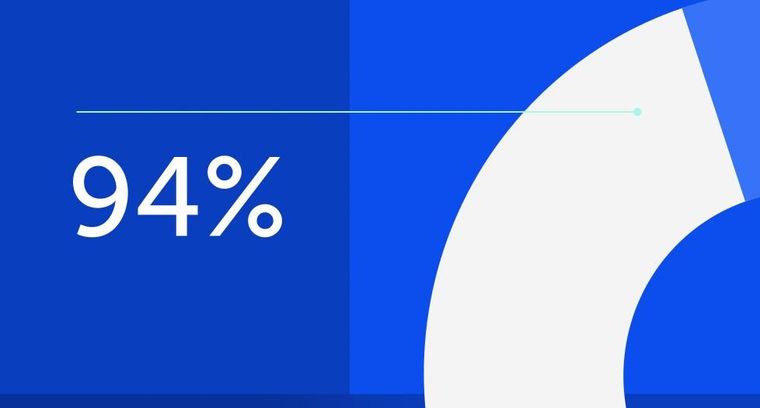
94% of researchers rate our articles as excellent or good
Learn more about the work of our research integrity team to safeguard the quality of each article we publish.
Find out more
ORIGINAL RESEARCH article
Front. Endocrinol., 03 February 2022
Sec. Adrenal Endocrinology
Volume 13 - 2022 | https://doi.org/10.3389/fendo.2022.808331
This article is part of the Research TopicInsights in Adrenal Endocrinology: 2021View all 10 articles
Whole transcriptome profiling is a promising technique in adrenal studies; however, whole transcriptome profiling of adrenal disease using formalin-fixed paraffin-embedded (FFPE) samples has to be further explored. The aim of this study was to evaluate the utility of transcriptome data from FFPE samples of adrenocortical tumors. We performed whole transcriptome profiling of FFPE and fresh frozen samples of adrenocortical carcinoma (ACC, n = 3), aldosterone-producing adenoma (APA, n = 3), and cortisol-producing adenoma (CPA, n = 3), and examined the similarity between the transcriptome data. We further examined whether the transcriptome data of FFPE samples could be used to distinguish tumor types and detect marker genes. The number of read counts was smaller in FFPE samples than in fresh frozen samples (P < 0.01), while the number of genes detected was similar (P = 0.39). The gene expression profiles of FFPE and fresh frozen samples were highly correlated (r = 0.93, P < 0.01). Tumor types could be distinguished by consensus clustering and principal component analysis using transcriptome data from FFPE samples. In the differential expression analysis between ACC and APA-CPA, known marker genes of ACC (e.g., CCNB2, TOP2A, and MAD2L1) were detected in FFPE samples of ACC. In the differential expression analysis between APA and CPA, known marker genes of APA (e.g., CYP11B2, VSNL1, and KCNJ5) were detected in the APA of FFPE samples. The results suggest that FFPE samples may be a reliable alternative to fresh frozen samples for whole transcriptome profiling of adrenocortical tumors.
The adrenal cortex produces a variety of steroid hormones and maintains metabolic homeostasis (1). Aldosterone, produced in the zona glomerulosa, is involved in the regulation of both the Na/K balance and blood pressure, while cortisol, produced in the zona fasciculata, plays an important role in the regulation of glucose metabolism and the immune response. Some adrenocortical adenomas produce these steroid hormones inappropriately and cause a variety of clinical syndromes. Aldosterone-producing adenoma (APA) causes primary aldosteronism, and cortisol-producing adenoma (CPA) causes Cushing’s syndrome (2, 3). Adrenocortical carcinoma (ACC) is a rare malignant tumor that arises in the adrenal cortex and has a very poor prognosis (4). In the clinical management of adrenocortical tumors, it is important to distinguish between these tumors, especially ACC and adenoma, although they can have overlapping clinical and pathologic findings that can be difficult to distinguish. Previous transcriptome studies have shown that APA, CPA, and ACC have distinct gene expression profiles, suggesting that transcriptome profiling can contribute to the accurate diagnosis of adrenocortical tumors (5, 6). Furthermore, transcriptome profiling is also a promising technique for elucidating the pathogenesis of adrenal diseases in terms of endocrine function and adrenal differentiation (7, 8).
Molecular profiling, including transcriptomics, usually requires fresh frozen tissue samples. However, these are difficult to obtain for most adrenocortical tumors because fresh frozen tissue is rarely preserved in routine clinical practice. This is a major obstacle to transcriptome studies of adrenocortical tumors. However, formalin-fixed paraffin-embedded (FFPE) samples are relatively easy to obtain, but the nucleic acids are degraded and less high-quality DNA and RNA can be extracted compared with fresh frozen samples (9, 10). In recent years, advances in sequencing technology have made it possible to perform molecular profiling using nucleic acids extracted from FFPE samples, and techniques for the genomic profiling of tumors using DNA extracted from FFPE samples have been established and are being used clinically (11). Whole transcriptome profiling using FFPE samples has been reported to be useful in malignant tumors such as hepatocellular carcinoma and breast cancer (12, 13). However, it remains unknown whether whole transcriptome profiling of adrenal disease using FFPE samples can be successfully performed.
In this study, we performed whole transcriptome profiling using FFPE samples of adrenocortical tumors (ACC, APA, and CPA) and compared the results with those of fresh frozen samples. We demonstrated the utility of FFPE samples in the whole transcriptome profiling of adrenocortical tumors.
We used ACC (n = 3), APA (n = 3), and CPA (n = 3) tumor tissues obtained from adrenocortical tumor patients operated at Kyushu University Hospital from 2017 to 2020. Diagnosis was made based on clinical symptoms, imaging features, and postoperative histopathological data. Each tumor was stored by both FFPE and fresh frozen after surgery. A portion of each tumor was collected in microtubes immediately after surgical removal and stored in a deep freezer (-80°C) (fresh frozen sample). The rest of the tumor was formalin-fixed and paraffin-embedded (FFPE sample). FFPE samples were stored at room temperature and fresh frozen samples were stored at -80°C for 1-4 years until RNA extraction. The detailed characteristics of each tumor sample were summarized in Supplementary Table 1.
FFPE samples were sectioned into 10 μm slices and only the tumor area was collected under visual observation. Total RNA was extracted from the collected tumor using the Maxwell RSC RNA FFPE Kit (Promega) according to the manufacturer’s instructions. Total RNA from fresh frozen samples was extracted using TRIzol reagent (Thermo Fisher) according to the manufacturer’s instructions. The RNA yield and quality were determined by UV absorption using a NanoDrop 2000 spectrophotometer. The RNA fragment size of FFPE samples was analyzed using the RNA 6000 Pico Kit (Agilent Technologies) running on the 2100 Bioanalyzer. Similarly, the RNA fragment size of fresh frozen samples was analyzed using the RNA 6000 Nano Kit. RNA integrity number (RIN) values and DV200 (the percentage of RNA fragments above 200 nucleotides in length) were obtained by running Bioanalyzer according to the manufacturer’s instructions. RNA quality was assessed using RIN and DV200 values.
Ribosomal RNA was removed from 100 ng of total RNA of each sample using the NEBNext rRNA Depletion Kit (Human/Mouse/Rat) (NEB, E6310). Libraries were then prepared using the NEBNext Ultra II Directional RNA Library Prep Kit for Illumina (NEB, E7760). Libraries were quantified using the Bioanalyzer DNA-sensitivity kit (Agilent, 5067-4626) and sequenced on the NextSeq 500 (Illumina) using a 36 cycle, paired-end protocol providing approximately 33 million reads per sample. Base call files were converted to the FASTQ format using Bcl2Fastq (Illumina). Using the CLC genomics workbench (v10.1.1), all reads were aligned to the reference genome (Human, hg19), and gene expression was quantified.
We used R (version 4.1.1) to perform the data analysis. Gene expression data were used as inputs for the R package edgeR (14). Genes with low expression were excluded using the “filterByExpr” function of edgeR. The gene counts were normalized by applying the trimmed mean of M-values (TMM) normalization method using the “calcNormFactors” and “cpm” functions of edgeR. The resulting log2 counts per million (logCPM) were used as inputs for downstream analyses (e.g., sample-to-sample correlation and consensus clustering). Consensus clustering (with the number of clusters “k” evaluated for k = 2–6) was performed using the R package ConsensusClusterPlus (15). Fifty iterations were performed with a sample inclusion probability of 0.8 and an item inclusion probability of 1. The number of clusters was selected based on the inspection of the delta area plot. Principal component analysis was performed using the “prcomp” function of R package stats (16–18). Differentially expressed genes (DEGs) between adrenocortical tumors (ACC and the other types, or APA and CPA) were detected using the “lmfit” and “eBayes” functions of the R package limma (19, 20). The Benjamini-Hochberg method was used to correct for multiple comparisons. DEGs were defined as genes with an absolute value of log2 fold-change (logFC) greater than 2 and an adjusted P value (adj. P) of less than 0.05. KEGG pathway analysis was performed using the R package pathfindR (21). The “run_pathfindR” function was used with default parameters to identify the enriched KEGG pathways. The “score_terms” function was then used to calculate the agglomerated z score of each enriched pathway per sample.
We extracted RNA from a total of nine FFPE and nine fresh frozen samples from three ACC, three APA, and three CPA cases. Total RNA extracted from FFPE samples had lower RNA yield and quality parameters compared to those from fresh frozen samples (RNA concentration [mean 36.0 vs 464.5 μL, Wilcoxon rank sum test: P value < 0.01], RIN [mean 2.2 vs 7.9, P value < 0.01], DV200 [mean 47.6 vs 71.0, P value < 0.01]). To examine the effect of storage period on RNA yield and quality for each storage method, we divided the samples into two groups by the median storage period from surgery to RNA extraction and compared the two groups. There was no significant difference in the RNA yield and quality (Supplementary Figure 1).
Next, we performed RNA sequencing (RNA-seq) using the extracted RNA. The number of raw reads obtained from FFPE samples was similar to those from frozen samples (mean 3.30E7 vs 3.29E7, P value = 0.86), but the percentage of mapped reads of FFPE samples was less than those of fresh frozen samples (mean 82.9% vs 87.7%, P value = 0.02) (Supplementary Table 1). Consequently, the read counts obtained from FFPE samples were less than those from the fresh frozen samples (mean 3.58E6 vs 5.13E6, P value < 0.01) (Figure 1A). In contrast, the number of unique genes detected in FFPE samples was similar to those in fresh frozen samples (mean 18160 vs 18001, P value = 0.39).
Figure 1 Comparison of transcriptome data obtained from FFPE and fresh frozen samples. (A) Distributions of the number of read counts (nCount) and the number of genes detected (nGene) were compared between FFPE and fresh frozen samples. (B) Results of correlation analysis between FFPE and fresh frozen samples for each patient. r; Pearson’s correlation coefficient.
Using normalized gene expression data, we examined the correlation between samples. In each case, the FFPE and fresh frozen samples were highly correlated (mean Pearson’s correlation coefficient [r] = 0.93) (Figure 1B). Therefore, we found that similar gene expression profiles could be obtained using FFPE samples, although the amount of detectable transcriptome was lower than that of fresh frozen samples.
We examined whether transcriptome data from FFPE samples could be used to distinguish between ACC, APA, and CPA. First, we validated whether the adrenocortical tumor types (ACC, APA, and CPA) could be separated at the transcriptome level using fresh frozen samples. Using consensus clustering, fresh frozen samples were classified into five clusters. APA and CPA were each classified into one cluster, and ACC was classified into one cluster per case (Figure 2A). Principal component analysis of fresh frozen samples was performed and visualized on two dimensions, with principal components 1 and 2 as the axes. APA and CPA each formed one cluster, whereas ACC was scattered in each case (Figure 2B). These results confirmed that the three types of tumors used in this study (ACC, APA, and CPA) had distinct gene expression profiles. In addition, ACC was considered to have a large difference in gene expression profiles among the cases.
Figure 2 Results of consensus clustering and principal component analysis. (A) Fresh frozen samples were divided into five clusters by consensus clustering (Cluster 1-3: ACC, Cluster 4: APA, Cluster 5: CPA samples). (B) Principal component analysis of fresh frozen samples confirmed separation based on tumor types. (C) By consensus clustering, FFPE samples were also divided into five clusters (Cluster 1-3: ACC, Cluster 4: APA, Cluster 5: CPA samples) similar to fresh frozen samples. (D) Principal component analysis of FFPE samples confirmed separation based on tumor types.
Further, we performed consensus clustering and principal component analysis of the transcriptome data of FFPE samples. The FFPE samples were classified into five clusters by consensus clustering. APA and CPA were each classified into one cluster, and ACC was classified into one cluster per case (Figure 2C). Principal component analysis of FFPE samples was performed and visualized on two dimensions with principal components 1 and 2 as the axes. APA and CPA each formed one cluster, whereas ACC was scattered in each case (Figure 2D). The results of consensus clustering and principal component analysis of FFPE samples were both similar to those of fresh frozen samples. Therefore, we found that it was possible to distinguish between ACC, APA, and CPA using transcriptome data from FFPE samples.
We examined whether transcriptome data from FFPE samples could be used to detect previously reported adrenocortical tumor marker genes. First, we performed differential expression analysis between ACC and APA-CPA for each storage method (FFPE and fresh frozen). In FFPE samples of ACC, 402 DEGs were detected (161 upregulated and 241 downregulated). In fresh frozen samples of ACC, 356 DEGs were detected (148 upregulated and 208 downregulated). 193 DEGs were common between FFPE and fresh frozen samples (76 upregulated and 117 downregulated). The upregulated DEGs in FFPE samples of ACC included genes previously reported to be upregulated in ACC, such as CCNB2 (encoding cyclin B2), TOP2A (encoding DNA topoisomerase II alpha), and MAD2L1 (encoding mitotic arrest deficient 2 like 1) (Table 1 and Supplementary Table 2) (22–24). These genes were also included in the upregulated DEGs in fresh frozen samples of ACC. IGF2 (encoding insulin like growth factor 2), commonly used as a marker for ACC (25, 26), was not identified as an upregulated DEG in either FFPE or fresh frozen samples. This may be due to the low expression of IGF2 in both FFPE and fresh frozen samples of case ACC_2 (Supplementary Figure 2). KEGG pathway analysis showed that upregulated DEGs in FFPE samples of ACC were enriched in tumor-related pathways including “cell cycle” and “p53 signaling pathway” (Figure 3). The upregulated DEGs in fresh frozen samples of ACC were also enriched in similar tumor-related pathways. Therefore, it was confirmed that the transcriptome data from FFPE samples could be used to detect the genes characteristic of ACC.
Figure 3 Heatmap showing the results of KEGG pathway analysis of DEGs detected between ACC and APA-CPA by each storage type. Score; the agglomerated z score of each enriched KEGG pathway per sample.
Next, we performed differential expression analysis between APA and CPA for each storage method (FFPE and fresh frozen). In FFPE samples of APA, 135 upregulated DEGs were detected. In fresh frozen samples of APA, 106 upregulated DEGs were detected. Forty-seven upregulated DEGs were common between FFPE and fresh frozen samples. The upregulated DEGs in FFPE samples of APA included genes reported to be associated with APA, such as CYP11B2 (encoding cytochrome P450 family 11 subfamily B member 2, also known as aldosterone synthase), KCNJ5 (encoding potassium inwardly rectifying channel subfamily J member 5), VSNL1 (encoding visinin like 1), CALN1 (encoding calneuron 1), and HTR4 (encoding 5-hydroxytryptamine receptor 4) (Table 2 and Supplementary Table 3) (27–31). These genes were also included in the upregulated DEGs in fresh frozen samples of APA. While in FFPE samples of CPA, 115 upregulated DEGs were detected. In fresh frozen samples of CPA, 97 upregulated DEGs were detected. Forty-eight upregulated DEGs were common between FFPE and fresh frozen samples. The upregulated DEGs in FFPE samples of CPA included genes reported to be upregulated in CPA, such as FATE1 (fetal and adult testis expressed 1), PITX1 (encoding paired like homeodomain 1) and CXCL2 (encoding C-X-C motif chemokine ligand 2) (7, 32). KEGG pathway analysis showed that upregulated DEGs in FFPE samples of APA were enriched in pathways such as “serotonergic synapse” and “circadian entrainment”, while DEGs in fresh frozen samples of APA were enriched in pathways such as “calcium signaling pathway” and “hippo signaling pathway” (Figure 4). The common upregulated DEGs between FFPE samples and fresh frozen samples were enriched in the pathway of “aldosterone synthesis and secretion” (Supplementary Figure 3). The upregulated DEGs in FFPE samples of CPA were enriched in metabolism-related pathways such as “steroid biosynthesis” and “cholesterol metabolism” (Figure 4). The upregulated DEGs in fresh frozen samples of CPA were also enriched in similar metabolism-related pathways. Thus, it was confirmed that transcriptome data from FFPE samples could be used to detect the characteristic genes of APA and CPA.
Figure 4 Heatmap showing the results of KEGG pathway analysis of DEGs between APA and CPA by each storage type. Score; the agglomerated z score of each enriched KEGG pathway per sample.
To evaluate the utility of transcriptome data obtained from FFPE samples in adrenocortical tumors, we compared transcriptome data from FFPE samples of ACC, APA, and CPA with those from fresh frozen samples of the same tumors. Transcriptome data from FFPE and fresh frozen samples showed a high degree of similarity. Using transcriptome data from FFPE samples, we were able to distinguish between ACC, APA, and CPA and detect the marker genes. Our study is the first to show that FFPE samples may be an alternative to fresh frozen samples for whole transcriptome profiling of adrenocortical tumors.
Recently, transcriptome profiling using FFPE samples has been performed in a variety of tissues (12, 13), but few studies have validated the accuracy of gene expression profiles obtained from FFPE samples using fresh frozen samples of the same tissue. Hedegaard et al. reported a comparative study of RNA-seq using FFPE and fresh frozen samples from the same tissue of six different human tissue types (bladder, prostate, and colon carcinoma; liver and colon normal tissue; reactive tonsil) (33). Shohdy et al. reported a comparative study of RNA-seq using FFPE and fresh frozen samples from the same tumors of seven different tumors (urothelial cancer, gastroesophageal junction adenocarcinoma, oligodendroglioma, cancer of unknown primary, leiomyosarcoma, papillary thyroid cancer, and colorectal cancer) in 11 patients (34). In this study, we showed that gene expression profiles from FFPE samples were highly similar to those from fresh frozen samples of the same adrenocortical tumors (ACC, APA, and CPA).
In transcriptome profiling using FFPE samples, targeted RNA-seq is often used because of the low yield and quality of RNA extracted from such samples (35, 36). Plaska et al. reported that targeted RNA-seq (194 target genes) using FFPE samples of adrenocortical tumors (ACC, APA, and CPA) could distinguish between benign and malignant tumors (36). However, targeted RNA-seq is not suitable for comprehensive genetic analysis (e.g., detection of novel pathogenic genes) because it restricts the genes that can be analyzed. In this study, we used whole transcriptome RNA-seq rather than targeted RNA-seq to obtain expression profiles of a large number of genes in each tumor (average number of genes detected in FFPE samples: 18001). We thus demonstrated that it is possible to distinguish ACC, APA, and CPA and detect their marker genes using transcriptome data from FFPE samples. Our results support the possible utility of whole transcriptome profiling using FFPE samples of adrenocortical tumors.
In the consensus clustering and principal component analysis of this study, APA and CPA each formed one cluster, and ACC differed greatly among cases. This result was similar for both FFPE and fresh frozen samples. The variation in ACC may be due to the different tumor traits in each case. Alternatively, it may be due to technical issues such as the storage conditions of each sample and the storage period until RNA extraction. A larger number of cases would be needed to examine the differences in tumor traits in ACC.
In the differential expression analysis of this study, it was possible to detect the marker genes of each adrenocortical tumor using FFPE samples. There were also differences in the DEGs that could be detected using FFPE samples and fresh frozen samples. In FFPE samples of ACC, genes such as SPP1, PBK, and UBE2C could not be detected. In FFPE samples of APA, genes such as LGR5, HOPX, and ATP2B3 could not be detected. The read counts obtained from FFPE samples were lower than those of fresh frozen samples, which may result in the lower detection sensitivity of relatively low expression genes. When using FFPE samples for whole transcriptome profiling, a larger number of samples may be required compared to fresh frozen samples.
The use of FFPE samples for whole transcriptome profiling has advantages other than the ease of sample collection. FFPE samples are more suitable for morphological observation than fresh frozen samples, making it easier to collect transcriptomes from small regions of interest following microdissection. This may be applied, for example, to examine each layer of the adrenal cortex (which is composed of three layers) or small lesions such as aldosterone-producing cell clusters (presumed to be precursor lesions of APA) (37).
The limitation of this study is that the storage period of FFPE samples was relatively short (4 years at the longest). The longer the storage period, the lower the yield and quality of RNA, which may make it difficult to perform gene expression profiling equivalent to that using fresh frozen samples (38). Studies using FFPE samples with longer storage periods are required to validate our results. Another limitation is the lack of comparison between adrenocortical tumors and normal adrenocortical tissue adjacent to the tumors. In examining the intrinsic properties of each adrenocortical tumor, the normal adrenocortical tissue may be the ideal comparison target.
In conclusion, in this study, we demonstrated the utility of gene expression profiling of adrenocortical tumors using FFPE samples. FFPE samples are relatively easier to obtain, thus allowing large-scale adrenocortical tumor transcriptome studies.
The datasets presented in this study can be found in online repositories. The names of the repository/repositories and accession number(s) can be found below: https://www.ncbi.nlm.nih.gov/sra/PRJNA787399, PRJNA787399.
The studies involving human participants were reviewed and approved by the medical ethics committee of the Kyushu University Hospital (approval #21025-00). The patients/participants provided their written informed consent to participate in this study.
NI wrote the initial draft. HU and YO edited the manuscript. NI analyzed the results. HU and YO managed this study. HU contributed to data interpretation. MO, TF, HK, ET, SK, NU, KS, MY-U, YM, and RS curated the data and provided critical feedback and helped shape the research, analysis, and manuscript. All authors contributed to the article and approved the submitted version.
This work was supported by grant “KAKENHI 20K17493”, grant “The Uehara Memorial Foundation”, grant “Secom Science and Technology Foundation”, grant “Kaibara Morikazu Medical Science Promotion Foundation”, grant “Takeda Science Foundation”, grant “KAKENHI 20K16525”, grant “KAKENHI 20K17514”, grant “KAKENHI 20K21604”, and “The Mitsubishi Foundation”.
KS was employed by Kyushu Pro Search Limited Liability Partnership.
The remaining authors declare that the research was conducted in the absence of any commercial or financial relationships that could be construed as a potential conflict of interest.
All claims expressed in this article are solely those of the authors and do not necessarily represent those of their affiliated organizations, or those of the publisher, the editors and the reviewers. Any product that may be evaluated in this article, or claim that may be made by its manufacturer, is not guaranteed or endorsed by the publisher.
The Supplementary Material for this article can be found online at: https://www.frontiersin.org/articles/10.3389/fendo.2022.808331/full#supplementary-material
Supplementary Figure 1 | Comparison of RNA yield and quality parameters between two storage period groups. FFPE samples were divided into two groups by 340 days, the median storage period. Similarly, fresh-frozen samples were divided by 427 days.
Supplementary Figure 2 | Distribution of the logCPM values of IGF2. Dots; each sample.
Supplementary Figure 3 | Heatmap showing the results of KEGG pathway analysis of DEGs between APA and CPA (common to FFPE and fresh frozen samples). Score; the agglomerated z score of each enriched KEGG pathway per sample.
1. Stewart PM. Adrenal Cortex. Curr Opin Endocrinol Diabetes Obes (1999) 6:177. doi: 10.1097/00060793-199906000-00001
2. Conn JW. Presidential Address. I. Painting Background. II. Primary Aldosteronism, a New Clinical Syndrome. J Lab Clin Med (1955) 45:3–17. doi: 10.5555/uri:pii:0022214355900482
3. Cushing H. Further Notes On Pituitary Basophilism. JAMA (1932) 99:281–4. doi: 10.1001/jama.1932.02740560007003
4. Weiss LM, Medeiros LJ, Vickery AL Jr. Pathologic Features of Prognostic Significance in Adrenocortical Carcinoma. Am J Surg Pathol (1989) 13:202–6. doi: 10.1097/00000478-198903000-00004
5. Assié G, Guillaud-Bataille M, Ragazzon B, Bertagna X, Bertherat J, Clauser E. The Pathophysiology, Diagnosis and Prognosis of Adrenocortical Tumors Revisited by Transcriptome Analyses. Trends Endocrinol Metab (2010) 21:325–34. doi: 10.1016/j.tem.2009.12.009
6. Faillot S, Assie G. ENDOCRINE TUMOURS: The Genomics of Adrenocortical Tumors. Eur J Endocrinol (2016) 174:R249–65. doi: 10.1530/EJE-15-1118
7. Di Dalmazi G, Altieri B, Scholz C, Sbiera S, Luconi M, Waldman J, et al. RNA Sequencing and Somatic Mutation Status of Adrenocortical Tumors: Novel Pathogenetic Insights. J Clin Endocrinol Metab (2020) 105:e4459–79. doi: 10.1210/clinem/dgaa616
8. Lyu Q, Wang H, Kang Y, Wu X, Zheng HS, Laprocina K, et al. RNA-Seq Reveals Sub-Zones in Mouse Adrenal Zona Fasciculata and the Sexually Dimorphic Responses to Thyroid Hormone. Endocrinology (2020) 161:bqaa126. doi: 10.1210/endocr/bqaa126
9. Chaw YF, Crane LE, Lange P, Shapiro R. Isolation and Identification of Cross-Links From Formaldehyde-Treated Nucleic Acids. Biochemistry (1980) 19:5525–31. doi: 10.1021/bi00565a010
10. Penland SK, Keku TO, Torrice C, He X, Krishnamurthy J, Hoadley KA, et al. RNA Expression Analysis of Formalin-Fixed Paraffin-Embedded Tumors. Lab Invest (2007) 87:383–91. doi: 10.1038/labinvest.3700529
11. Frampton GM, Fichtenholtz A, Otto GA, Wang K, Downing SR, He J, et al. Development and Validation of a Clinical Cancer Genomic Profiling Test Based on Massively Parallel DNA Sequencing. Nat Biotechnol (2013) 31:1023–31. doi: 10.1038/nbt.2696
12. Hoshida Y, Villanueva A, Kobayashi M, Peix J, Chiang DY, Camargo A, et al. Gene Expression in Fixed Tissues and Outcome in Hepatocellular Carcinoma. N Engl J Med (2008) 359:1995–2004. doi: 10.1056/NEJMoa0804525
13. Sinicropi D, Qu K, Collin F, Crager M, Liu M-L, Pelham RJ, et al. Whole Transcriptome RNA-Seq Analysis of Breast Cancer Recurrence Risk Using Formalin-Fixed Paraffin-Embedded Tumor Tissue. PloS One (2012) 7:e40092. doi: 10.1371/journal.pone.0040092
14. Robinson MD, Oshlack A. A Scaling Normalization Method for Differential Expression Analysis of RNA-Seq Data. Genome Biol (2010) 11:R25. doi: 10.1186/gb-2010-11-3-r25
15. Wilkerson MD, Hayes DN. ConsensusClusterPlus: A Class Discovery Tool With Confidence Assessments and Item Tracking. Bioinformatics (2010) 26:1572–3. doi: 10.1093/bioinformatics/btq170
16. Becker RA, Chambers JM, Wilks AR. The New S Language: A Programming Environment for Data Analysis and Graphic. Pacific Grove, É. U: Wadsworth et Brooks/Cole (1988).
18. Venables WN, Ripley BD. Modern Applied Statistics With s. 4 edn. New York: Springer-Verlag (2002).
19. Ritchie ME, Phipson B, Wu D, Hu Y, Law CW, Shi W, et al. Limma Powers Differential Expression Analyses for RNA-Sequencing and Microarray Studies. Nucleic Acids Res (2015) 43:e47–7. doi: 10.1093/nar/gkv007
20. Phipson B, Lee S, Majewski IJ, Alexander WS, Smyth GK. Robust Hyperparameter Estimation Protects Against Hypervariable Genes and Improves Power to Detect Differential Expression. Ann Appl Stat (2016) 10:946–63. doi: 10.1214/16-AOAS920
21. Ulgen E, Ozisik O, Sezerman OU. Pathfindr: An R Package for Comprehensive Identification of Enriched Pathways in Omics Data Through Active Subnetworks. Front Genet (2019) 10:858. doi: 10.3389/fgene.2019.00858
22. de Reyniès A, Assié G, Rickman DS, Tissier F, Groussin L, René-Corail F, et al. Gene Expression Profiling Reveals a New Classification of Adrenocortical Tumors and Identifies Molecular Predictors of Malignancy and Survival. J Clin Oncol (2009) 27:1108–15. doi: 10.1200/JCO.2008.18.5678
23. Giordano TJ, Thomas DG, Kuick R, Lizyness M, Misek DE, Smith AL, et al. Distinct Transcriptional Profiles of Adrenocortical Tumors Uncovered by DNA Microarray Analysis. Am J Pathol (2003) 162:521–31. doi: 10.1016/S0002-9440(10)63846-1
24. Giordano TJ, Kuick R, Else T, Gauger PG, Vinco M, Bauersfeld J, et al. Molecular Classification and Prognostication of Adrenocortical Tumors by Transcriptome Profiling. Clin Cancer Res (2009) 15:668–76. doi: 10.1158/1078-0432.CCR-08-1067
25. Boulle N. Increased Levels of Insulin-Like Growth Factor II (IGF-II) and IGF-Binding Protein-2 Are Associated With Malignancy in Sporadic Adrenocortical Tumors. J Clin Endocrinol Metab (1998) 83:1713–20. doi: 10.1210/jcem.83.5.4816
26. Almeida MQ, Fragoso MCBV, Lotfi CFP, Santos MG, Nishi MY, Costa MHS, et al. Expression of Insulin-Like Growth Factor-II and its Receptor in Pediatric and Adult Adrenocortical Tumors. J Clin Endocrinol Metab (2008) 93:3524–31. doi: 10.1210/jc.2008-0065
27. Bassett MH, Mayhew B, Rehman K, White PC, Mantero F, Arnaldi G, et al. Expression Profiles for Steroidogenic Enzymes in Adrenocortical Disease. J Clin Endocrinol Metab (2005) 90:5446–55. doi: 10.1210/jc.2005-0836
28. Williams TA, Monticone S, Crudo V, Warth R, Veglio F, Mulatero P. Visinin-Like 1 is Upregulated in Aldosterone-Producing Adenomas With KCNJ5 Mutations and Protects From Calcium-Induced Apoptosis. Hypertension (2012) 59:833–9. doi: 10.1161/HYPERTENSIONAHA.111.188532
29. Kobuke K, Oki K, Gomez-Sanchez CE, Gomez-Sanchez EP, Ohno H, Itcho K, et al. Calneuron 1 Increased Ca2+ in the Endoplasmic Reticulum and Aldosterone Production in Aldosterone-Producing Adenoma. Hypertension (2018) 71:125–33. doi: 10.1161/HYPERTENSIONAHA.117.10205
30. Ye P, Mariniello B, Mantero F, Shibata H, Rainey WE. G-Protein-Coupled Receptors in Aldosterone-Producing Adenomas: A Potential Cause of Hyperaldosteronism. J Endocrinol (2007) 195:39–48. doi: 10.1677/JOE-07-0037
31. Choi M, Scholl UI, Yue P, Björklund P, Zhao B, Nelson-Williams C, et al. K+ Channel Mutations in Adrenal Aldosterone-Producing Adenomas and Hereditary Hypertension. Science (2011) 331:768–72. doi: 10.1126/science.1198785
32. Kitawaki Y, Nakamura Y, Kubota-Nakayama F, Yamazaki Y, Miki Y, Hata S, et al. Tumor Microenvironment in Functional Adrenocortical Adenomas: Immune Cell Infiltration in Cortisol-Producing Adrenocortical Adenoma. Hum Pathol (2018) 77:88–97. doi: 10.1016/j.humpath.2018.03.016
33. Hedegaard J, Thorsen K, Lund MK, Hein A-MK, Hamilton-Dutoit SJ, Vang S, et al. Next-Generation Sequencing of RNA and DNA Isolated From Paired Fresh-Frozen and Formalin-Fixed Paraffin-Embedded Samples of Human Cancer and Normal Tissue. PloS One (2014) 9:e98187. doi: 10.1371/journal.pone.0098187
34. Shohdy KS, Bareja R, Sigouros M, Wilkes DC, Dorsaint P, Manohar J, et al. Functional Comparison of Exome Capture-Based Methods for Transcriptomic Profiling of Formalin-Fixed Paraffin-Embedded Tumors. NPJ Genom Med (2021) 6:66. doi: 10.1038/s41525-021-00231-7
35. Hovelson DH, Udager AM, McDaniel AS, Grivas P, Palmbos P, Tamura S, et al. Targeted DNA and RNA Sequencing of Paired Urothelial and Squamous Bladder Cancers Reveals Discordant Genomic and Transcriptomic Events and Unique Therapeutic Implications. Eur Urol (2018) 74:741–53. doi: 10.1016/j.eururo.2018.06.047
36. Plaska SW, Liu C-J, Lim JS, Rege J, Bick NR, Lerario AM, et al. Targeted RNAseq of Formalin-Fixed Paraffin-Embedded Tissue to Differentiate Among Benign and Malignant Adrenal Cortical Tumors. Horm Metab Res (2020) 52:607–13. doi: 10.1055/a-1212-8803
37. Nishimoto K, Tomlins SA, Kuick R, Cani AK, Giordano TJ, Hovelson DH, et al. Aldosterone-Stimulating Somatic Gene Mutations are Common in Normal Adrenal Glands. Proc Natl Acad Sci USA (2015) 112:E4591–9. doi: 10.1073/pnas.1505529112
Keywords: whole transcriptome profiling, RNA sequencing (RNAseq), formalin-fixed paraffin-embedded samples (FFPE samples), adrenal diseases, adrenocortical tumors
Citation: Iwahashi N, Umakoshi H, Ogata M, Fukumoto T, Kaneko H, Terada E, Katsuhara S, Uchida N, Sasaki K, Yokomoto-Umakoshi M, Matsuda Y, Sakamoto R and Ogawa Y (2022) Whole Transcriptome Profiling of Adrenocortical Tumors Using Formalin-Fixed Paraffin-Embedded Samples. Front. Endocrinol. 13:808331. doi: 10.3389/fendo.2022.808331
Received: 03 November 2021; Accepted: 11 January 2022;
Published: 03 February 2022.
Edited by:
Valentina Morelli, Istituto Auxologico Italiano, ItalyReviewed by:
Matthias Kroiß, Ludwig Maximilian University of Munich, GermanyCopyright © 2022 Iwahashi, Umakoshi, Ogata, Fukumoto, Kaneko, Terada, Katsuhara, Uchida, Sasaki, Yokomoto-Umakoshi, Matsuda, Sakamoto and Ogawa. This is an open-access article distributed under the terms of the Creative Commons Attribution License (CC BY). The use, distribution or reproduction in other forums is permitted, provided the original author(s) and the copyright owner(s) are credited and that the original publication in this journal is cited, in accordance with accepted academic practice. No use, distribution or reproduction is permitted which does not comply with these terms.
*Correspondence: Hironobu Umakoshi, dW1ha29zaGlAbWVkLmt5dXNodS11LmFjLmpw; Yoshihiro Ogawa, eW9nYXdhQG1lZC5reXVzaHUtdS5hYy5qcA==
Disclaimer: All claims expressed in this article are solely those of the authors and do not necessarily represent those of their affiliated organizations, or those of the publisher, the editors and the reviewers. Any product that may be evaluated in this article or claim that may be made by its manufacturer is not guaranteed or endorsed by the publisher.
Research integrity at Frontiers
Learn more about the work of our research integrity team to safeguard the quality of each article we publish.