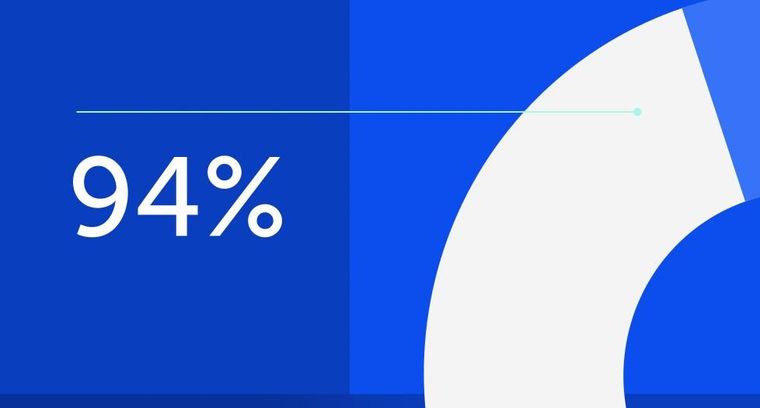
94% of researchers rate our articles as excellent or good
Learn more about the work of our research integrity team to safeguard the quality of each article we publish.
Find out more
ORIGINAL RESEARCH article
Front. Endocrinol., 28 April 2022
Sec. Translational and Clinical Endocrinology
Volume 13 - 2022 | https://doi.org/10.3389/fendo.2022.804455
Objective: An altered gut microbiota has been associated with insulin resistance, a metabolic dysfunction consisting of cellular insulin signaling impairment. The aim of the present study is to determine the taxonomic and functional fecal microbiota signatures associated with HOMA-IR index in a population with high cardiovascular risk.
Methods: A total of 279 non-diabetic individuals (55–75 years aged) with overweight/obesity and metabolic syndrome were stratified according to tertiles of HOMA-IR index. Blood biochemical parameters, anthropometric measurements and fecal samples were collected at baseline. Fecal microbial DNA extraction, 16S amplicon sequencing and bioinformatics analysis were performed.
Results: Desulfovibrio, Odoribacter and Oscillospiraceae UCG-002 were negatively associated with HOMA-IR index, whereas predicted total functional abundances revealed gut metabolic modules mainly linked to amino acid degradation. Butyricicoccus, Erysipelotrichaceae UCG-003, Faecalibacterium were positively associated with HOMA-IR index, whereas predicted total functional abundances revealed gut metabolic modules mainly linked to saccharide degradation. These bacteria contribute differentially to the gut metabolic modules, being the degree of contribution dependent on insulin resistance. Both taxa and gut metabolic modules negatively associated to HOMA-IR index were linked to mechanisms involving sulfate reducing bacteria, improvement of intestinal gluconeogenesis and production of acetate. Furthermore, both taxa and gut metabolic modules positively associated to HOMA-IR index were linked to production and mechanisms of action of butyrate.
Conclusions: Specific taxonomic and functional fecal microbiota signatures associated with insulin resistance were identified in a non-diabetic population with overweight/obesity at high cardiovascular risk. These findings suggest that tailoring therapies based on specific fecal microbiota profiles could be a potential strategy to improve insulin sensitivity.
Insulin is a peptide hormone that plays an essential role in the process of glucose metabolism regulation. Insulin resistance (IR) can be defined as a pathological condition of cellular insulin signaling impairment with consequent disturbance of intracellular signaling transduction, which affects several organs and tissues (1, 2). Visceral adiposity and increased body fat induce the release of relatively high levels of free fatty acids and pro-inflammatory cytokines into circulation, causing the development of hepatic and muscle IR and type 2 diabetes (T2D) (3).
An altered gut microbiota composition has been associated with the pathophysiology of obesity and IR (4). First evidence was reported in 2004 by Bäckhed et al., who has observed an increase in body fat and IR in germ-free mice transplanted with gut microbiota from conventionally raised mice donors (5). A few years later, Vrieze et al., shown that insulin sensitivity improved in a cohort of human participants with metabolic syndrome (MetS) six weeks after receiving microbiota from lean healthy human donors (6).
The gut microbiome can induce IR through different mechanisms. Cani et al., were the first to identify bacterial lipopolysaccharide (LPS) as a causative inflammatory factor of IR (7). LPS increases intestinal permeability with its consequent translocation from the intestinal lumen to circulation (8, 9). At the cell membrane level, LPS binds and activates the toll-like receptor 4 (TLR4), with consequent downstream cell signaling pathway activation which lead to inflammatory response and cytokine expression and secretion (10). A reduction of bacterial species able to produce short chain fatty acids (SCFAs), with well-established anti-inflammatory effects (11–13), also induces IR (4). However, these findings are still not totally clear due to some controversial results, as those described by Perry and colleagues, who observed increased production of acetate associated with IR and obesity in rodents exposed to high caloric diet (14). In addition, the bioconversion of bile acids carried out by bacteria from human gut, including members of Lactobacilli, Bifidobacteria, Clostridium and Bacteroides, has been shown contributing to glucose homeostasis (15, 16). A study conducted by Pedersen et al., aiming to identify the gut microbial profile of non-diabetic individuals with IR, demonstrated a positive association between IR and branched-chain amino acids (BCAA) levels (15). Taken together, these studies suggest that gut microbiota may play an important role in modulating IR, though it remains unclear which aspects of the microbiota contribute to this. Therefore, the identification of potential fecal microbiota profiles and related metabolic pathways linked to the development of IR is crucial, as the characterization of the intestinal bacterial community related to glucose homeostasis would be a useful strategy to ameliorate IR and related disorders (17). Accordingly, the aim of the present study was to determine the taxonomic and functional fecal microbiota signatures associated with IR in a population of 279 non-diabetic elderly individuals with overweight/obesity and MetS.
This cross-sectional study was conducted with baseline data of participants recruited for the PREDIMED-Plus study (18, 19), an ongoing parallel-group, randomized and controlled clinical trial conducted in 23 Spanish centers, which aims to evaluate the effect of an intensive weight loss intervention, based on an hypocaloric Mediterranean diet (MedDiet), physical activity promotion and behavioral support, on cardiovascular disease (CVD) events, compared to a control group receiving usual care advice. The trial was registered at the International Standard Randomized Controlled Trial (http://www.isrctn.com/ISRCTN89898870) and approved by the institutional review board of all participating institutions. The full detailed PREDIMED-Plus protocol is available at http://www.predimedplus.com.
PREDIMED-Plus eligible participants were men and women aged 55–75 years, with overweight or obesity (body mass index (BMI) 27–40 kg/m2), who satisfied at least three criteria for the MetS (waist circumference > 102 cm in men and > 88 cm in women; serum triglyceride ≥ 150 mg/dL or drug treatment for elevated triglycerides; high-density lipoprotein (HDL) cholesterol < 40 mg/dL in men and < 50 mg/dL in women or drug use for low HDL cholesterol; blood pressure ≥ 130/85 mmHg or antihypertensive drug treatment; and fasting plasma glucose level ≥ 100 mg/dL or hypoglycemic treatment) and were free of CVD.
A subsample of 400 participants from the PREDIMED-Plus recruiting centers of Reus and Malaga (Spain) were randomized and matched for age, sex and BMI. For the present cross-sectional study, we selected 279 individuals with available stool samples at baseline and without T2D.
Body weight was measured using high-quality electronic calibrated scales, height was measured using a wall-mounted stadiometer, waist circumference was measured midway between the lowest rib and the iliac crest using an anthropometric tape, blood pressure was measured using a validated semiautomatic oscillometer (Omron HEM-705CP, Kyoto, Japan).
Blood samples were collected after overnight fasting. Plasma levels of glucose, insulin, total cholesterol, HDL cholesterol and triglycerides were measured using standard enzymatic methods, low-density lipoprotein (LDL) cholesterol was calculated with the Friedewald formula (whenever triglycerides were less than 300 mg/dL), glycated hemoglobin was measured by a chromatographic method. Insulin resistance was estimated by the homeostatic model assessment of insulin resistance (HOMA-IR index) (20), using the following formula:
In addition, information regarding lifestyle, education level, disease prevalence and current medication use was collected, whereas a validated 17-item questionnaire (21) was used to assess the adherence to the MedDiet.
A complete workflow including the analytic steps is shown in Figure 1.
Figure 1 Workflow showing the fecal samples analytic process and bioinformatics pipeline. Bacterial DNA was extracted from frozen fecal and 16S amplicon sequencing performed. Resulting raw sequences in fastq format were imported into R environment and processed with DADA2 package. Output files followed 3 different pipelines: (1) processed in order to obtain information about alpha and beta diversity and differential abundant taxa; (2) processed with PICRUSt2 in order to obtain the predicted total functional abundances, then gut metabolic modules (GMM) were computed and the association with HOMA-IR index determined; (3) processed with PICRUSt2 in order to obtain the predicted contribution per genus of each GMM previously computed.
Stool samples were collected at home by participants and kept frozen. In case of antibiotic use or prebiotics supplementation, samples were collected 15 days after completion of treatment. A time range of 15 days was considered wide enough to gut microbiota recovery after therapeutic antibiotic usage, taking into account the exposure to short-term treatment with amoxicillin (22). Once delivered to the laboratory, stool samples were separated into 250 mg aliquots and stored at −80°C until analysis.
Microbial DNA was extracted with the QIAmp PowerFecal DNA kit (Qiagen, Hilden, Germany), following the manufacturer’s instructions, and DNA concentration and purity assessed with the Qubit 2.0 Fluorometer dsDNA Broad Range Assay Kit (Thermo Fisher Scientific, Waltham, MA, USA). Targeted 16S sequencing libraries were created with the 16S Metagenomics kit (Thermo Fisher Scientific, Waltham, MA, USA), using a pool of primers to amplify the regions V2–V9 of the 16S rRNA gene, and barcoded adapters ligated using the Ion Plus Fragment Library Kit (Thermo Fisher Scientific, Waltham, MA, USA). Synthesized libraries were pooled and templated on the automated Ion Chef system (Thermo Fisher Scientific, Waltham, MA, USA) and sequenced on the Ion S5 (Thermo Fisher Scientific, Waltham, MA, USA). Reads generated were subsequently converted in fastq format using the File Explorer plugin of the Torrent Suite Server software (Thermo Fisher Scientific, Waltham, MA, USA), interfaced with the Ion S5. The quality control of fastq files was assessed with FastQC using a quality score of 20 as a threshold (https://www.bioinformatics.babraham.ac.uk/projects/fastqc/).
Demultiplexed sequence reads, were imported into R (version 4.0.5), quality filtered, chimera-checked and clustered in amplicon sequence variants (ASVs) using the R package DADA2 (v 1.16.0) (23) whereas taxonomy was assigned using the SILVA reference database release 138 (24). Obtained counts were filtered and transformed using a centered log-ratio (CLR) transformation (25). Principal component analysis (PCA) was performed to evaluate the distribution of the study population. Alpha diversity indices, Chao1 (26), Shannon (27) and Simpson (28) were calculated and differences between tertiles of HOMA-IR index tested. Beta diversity was computed in terms of Aitchison distance, or Euclidean distance over CLR-transformed counts (25). Permutational multivariate analysis of variance (PERMANOVA) was performed using the adonis function (“vegan” package, version 2.5-6) to test differences between groups composition. The association between taxa and tertiles of HOMA-IR index was tested using generalized linear modelling (GLM), adjusted for 17-item MedDiet adherence score and recruiting center as covariates. Multiple testing correction was performed using Storey’s q-value procedure, and q < 0.1 deemed as significant (29).
In order to perform an inferential analysis of the functional potential of the microbiome, DADA2 output files were parsed and used as input for PICRUSt2 in order to generate a table of inferred per-sample abundances of KEGG genome orthologs (KO) (30). To assess the diversity in metabolic potential and anaerobic fermentation capacity encoded in (meta-) genomic sequences, we used a manually curated database of human gut metabolic modules (GMM), that describe enzymatic processes annotated using exclusively prokaryotic and archaeal KO (31). GMM counts were filtered, converted to CLR and the association with HOMA-IR index tested with the same GLM setup used for the analysis of taxa.
In addition to the predicted total functional abundances, PICRUSt2 also includes the predicted functional contribution per taxa (using the –stratified flag). This information was used to study the contribution of each taxon of interest to the GMM of interest.
The clinical characteristics of the study population were described using IBM SPSS Statistics version 23 (SPSS Inc., Chicago, Illinois, USA). Data normality was assessed with Shapiro-Wilk test. Normally distributed variables were described by means and standard deviations, non-normally distribute variables by median and interquartile range, whereas categorical variables were described as number and percentages. Differences across tertiles were evaluated through one-way analysis of variance (ANOVA) parametric test or Kruskal-Wallis non-parametric test for numerical variables, and with Pearson’s chi-square test for categorical variables. Student’s t parametric test or Mann-Whitney non-parametric test was used to calculate differences between tertiles for numerical variables. All statistical tests were 2-sided and p < 0.05 was deemed statistically significant.
Clinical characteristics of the study population categorized by tertiles of HOMA-IR index are shown in Table 1. Body weight and waist circumference were significantly lower in those participants in tertile 1 (T1) of HOMA-IR index than those in tertile 2 (T2) or tertile 3 (T3); BMI, triglycerides, glucose, insulin and glycated hemoglobin were significantly lower in those participants in T1 compared to T2 and T3 and participants in T2 versus those in T3; HDL-cholesterol was significantly higher in T1 versus T2 and T3, and in T2 versus T3; MedDiet adherence score was significantly higher in T2 vs T3.
PCA analysis over the CLR-transformed microbiome count data revealed that the first two components account approximately 12.22% and 8.46% respectively. These figures are approximately in line with literature (32). PCA plots shown that the microbiome sample distribution did not cluster based on tertiles of HOMA-IR index (Supplementary Figure 1). There were no statistically significant differences across tertiles of HOMA-IR index in the alpha diversity indices Chao1, Shannon and Simpson (Supplementary Figure 2 and Supplementary Table 1). The results of the PERMANOVA analysis and the permutation test for homogeneity of multivariate dispersions using Aitchison distance were also not statistically significant across tertiles of HOMA-IR index (Supplementary Table 2).
Taxa and GMM significantly associated with HOMA-IR index are summarized in Table 2. Genera Desulfovibrio, Odoribacter and Oscillospiraceae UCG-002 were negatively associated with the highest tertile of HOMA-IR index, whereas genera Butyricicoccus, Erysipelotrichaceae UCG-003 and Faecalibacterium were positively associated with the highest tertile of HOMA-IR index. In addition, 21 GMM generated from the predicted total functional abundances were associated with the highest tertile of HOMA-IR index, 8 of these GMM were negatively associated with the highest tertile of HOMA-IR index, whereas 14 GMM were positively associated.
The contribution of each taxon to the GMM of interest has been displayed at family level (at genus level in case of taxa of interest) per sample (Supplementary Figures 3, 4) and per tertile of HOMA-IR index (Supplementary Figures 5, 6). For the generation of stacked barplots, just the main contributors are represented with a cutoff of 0.3, it means that if a bacterium never contributes more than 30% to any function is not considered for the plot and is categorized as “other taxa”. However, the “other taxa” were not excluded from the statistical analysis.
Just those taxa significantly contributing (q < 0.1) to those GMM associated with the highest tertile of HOMA-IR index in from the predicted total functional abundances were depicted.
Taxa contribute differentially to the GMM of interest depending on IR: in case of negative association with HOMA-IR index, Desulfovibrio, Odoribacter, Oscillospiraceae UCG-002, significantly contributes to aspartate degradation and glycine degradation (Table 3); in case of positive association with HOMA-IR index, Butyricicoccus, Erysipelotrichaceae UCG-003, Faecalibacterium, significantly contribute to glutamine degradation II, lactaldehyde degradation, lactate production and fructan degradation (Table 4).
In the current study, we identified 6 different taxa consistently associated with IR. In addition, exploring the total predicted metagenome we found 21 GMM associated with IR. Finally, we explored the predicted taxa contribution to the GMM of interest, showing that bacteria contribute differentially to the function and that the degree of contribution is also dependent on IR. Therefore, we identified taxonomic signatures and potential metabolic pathways related to IR in a non-diabetic population at high cardiovascular risk. The relatively large number of altered GMM found may be understood in light of the altered gut metabolic environment one would expect to typically accompany a higher IR, thus drastically altering the potential substrates for the microbiome. Potential mechanisms explaining the association between fecal microbiota and IR are resumed in Figure 2. Microbial genera Desulfovibrio, Odoribacter and Oscillospiraceae UCG-002, were found negatively associated with IR. Notably, Desulfovibrio, a genus that is typically associated with worsened host health (33), was negatively associated with IR. Bacteria of the genus Desulfovibrio are the most represented sulfate-reducing bacteria (SRB) residing in the human gut. The excessive presence of SRB in the gut, has been associated with the development of disorders, such as inflammatory bowel disease (34) and ulcerative colitis (33). Also, 16S pyrosequencing results shown that members of Desulfovibrionaceae family were found significantly abundant in murine models of MetS and in animals with diet-induced obesity (35). In contrast, a significant increase of Desulfovibrio was described in the fecal microbiota of healthy subjects compared to individuals with obesity analysed with 16S rRNA sequencing (36), and reduced Desulfovibrio was also found in the gut microbiota assessed with quantitative PCR of preschool children with obesity compared to healthy controls, echoing our findings (37). In a study conducted to assess the nutritional effects as well as the adherence to the MedDiet on the gut microbiota of healthy adults analyzed with 16S rRNA gene sequencing, Desulfovibrio was found more abundant in lean individuals (38). Accordingly, we found Desulfovibrio negatively associated with the highest tertile of HOMA-IR index, who includes those subjects with a significantly higher BMI. Hydrogen sulfide (H2S) is a gas metabolite produced by SRB in the gut that directly activates the secretion of glucagon-like peptide-1 (GLP-1), a peptide hormone involved in glucose homeostasis and appetite regulation. Mice treated with a specific SRB supplemented prebiotic diet has elevated levels of Desulfovibrio piger, detected with targeted real-time PCR, and increased concentrations of H2S in feces and colon, with consequent stimulation of GLP-1 and enhancement of insulin secretion, improved oral glucose tolerance, and reduced food intake (39). Mice treated with liraglutide, an injectable GLP-1 receptor agonist (GLP-1 RA) used in the treatment of T2D, with demonstrated efficacy in improving glycemic control, has been shown to produce substantial changes in the gut microbiota assessed with pyrosequencing of 16S rRNA with enrichment of several genera, of whom Desulfovibrio (40). These findings support the potential involvement of Desulfovibrio in glucose homeostasis through the production of H2S and activation of GLP-1 and can partially explain the negative association between this genus and IR observed in our study.
Figure 2 Potential mechanisms explaining the association between fecal microbiome and IR. The negative association with IR seems to be linked with glucose homeostasis, induced by an increase in amino acids breakdown and by an increase in sulfate-reducing bacteria, with consequent promotion of intestinal gluconeogenesis, acetate synthesis and H2S production, in addition to an improved succinate metabolism. The positive association with IR seems to be linked to an increase in saccharides degradation that can induce the growth of butyrate-producing bacteria and bring to a disproportion in butyrate synthesis and an impairment in glycolipid metabolism.
In the present study HOMA-IR index was negatively associated with GMM linked to amino acid breakdown. The fraction of amino acids that are not absorbed by the colonic mucosa are metabolized by the large intestinal microbiota, resulting in the generation of metabolites and end-products such as SCFAs, and hydrogen sulfate ion (HO4S−) (41). Accordingly, we observed that HOMA-IR index was negatively associated to functions linked to cysteine degradation, resulting in the production of H2S. Glycine and glutamine are intestinal gluconeogenesis substrates, and it has been observed that intestinal gluconeogenesis protects against diabetes and obesity by suppressing hepatic gluconeogenesis and positively regulating glucose homeostasis (42). Furthermore, glutamine immunomodulatory effects have been described (43). In addition, we observed that in case of functions linked to aspartate degradation I and glycine degradation II, taxa of interest contribute significantly to the negative association with HOMA-IR index. Aspartate and glycine are precursors for the synthesis of acetate (44), who has been demonstrated to play a regulatory role in body weight control, and insulin sensitivity through effects on lipid metabolism and glucose homeostasis (45).
Odoribacter is an anaerobic nonmotile Gram-negative, succinate-consuming and SCFA-producing rod-shaped bacteria. This genus has been linked to a decrease in clinical parameters associated with CVD risk (46). The results of a cross-sectional study conducted in a cohort of Spanish subjects shown that obesity was associated with elevated levels of circulating succinate concomitant with impaired glucose metabolism. This increase was associated with specific changes in gut microbiota, assessed with 16S sequencing, including lower relative abundance of succinate-consuming Odoribacteraceae family in obese individuals (47). Odoribacter spp. were found to be associated with a healthy fasting serum lipid profile in women with obesity (48). Wang et al. shown that a high-fat diet induces reduction in the relative abundance of Odoribacter in mice fecal microbiota analysed with 16S rRNA gene sequencing, then suppressed by the use of capsaicin, suggesting that changes in the abundance of Odoribacter may play an important role in the effects of treatments for glucose intolerance and obesity (49). Our findings are in agreement with the beneficial characteristics described for this genus in relation to IR, as we observed a negative association with high HOMA-IR index in our study population.
Oscillospiraceae family negatively correlates with BMI and anti-insulin autoantibodies in type 1 diabetes in children (50). In addition, members of the family Oscillospiraceae were found depleted in obese children (51). Accordingly, we find UCG-002 from Oscillospiaraceae family negatively associated with the highest tertile of HOMA-IR index, that includes those subjects with significantly higher BMI, and other variables related to obesity and IR.
Therefore, our results suggest that genera negatively associated with IR, may be involved in mechanisms improving insulin sensitivity in our study population.
On the other hand, in our study we also find some genera positively associated with IR: Faecalibacterium, Butyricicoccus and Erysipelotricaceae UCG-003. Some Faecalibacterium strains, like F. prausnitzii, are butyrate producers used as health biomarkers in humans for its immunomodulatory properties and more specifically for its anti-inflammatory effects (52). Hippe et al., analyzed through molecular experiments based on qPCR, the different F. prausnitzii phylotypes and their butyrate producing capacity in non-diabetic individuals with obesity, to determine whether an excess of butyrate production can be a risk factor for the development T2D. Results shown that the overproduction of butyrate induced by different F. prausnitzii phylotypes discriminates between obese developing T2D or not, suggesting possible different inflammatory genesis patterns in the host (53). Other studies have suggested that the disruption in the relative proportions of butyrate-producing bacteria, such as F. prausnitzi, in gut microbial populations may contribute to weight gain and IR (54).
A similar argument can be extended to another genus found positively associated with HOMA-IR index, Butyricicoccus, which includes butyrate-producing strains considered beneficial for gut health (55). Despite the large evidence supporting the beneficial effects of butyrate on gut health, its effect on obesity is still a debated topic. Some studies shown that butyrate induces obesity providing the substrate for energy expenditure but also by engaging in signaling pathways involved in glycolipid metabolism (56). This last observation supports our findings showing butyrate-producing taxa such as Faecalibacterium and Butyricicoccus positively associated with impaired metabolism and IR.
In accordance, our findings also reveal that HOMA-IR index was positively associated with functions linked to mono-, di-, and polysaccharides degradation. Non-digestible carbohydrates are fermented by colonic microbes, leading to the production SCFAs such as butyrate (57).
We observed that taxa of interest contribute significantly to the GMM positively associated with HOMA-IR index linked to fructan degradation, glutamine degradation II, lactaldehyde degradation and lactate production. Indeed, dietary fibers are the main source of oligosaccharides such as fructan, which can promote the growth of butyrate-producing bacteria (58). In addition, lactate and lactaldehyde are fermentation products intermediary in the production of butyrate from carbohydrates (59, 60). Accordingly, it seems that the action of some taxa of interest is mediated by functions mainly linked to butyrate production, and that this metabolite is playing a role in the association observed with IR.
Erysipelotrichaceae have been found enriched in case of inflammatory diseases and obesity, probably because of its involvement in host lipid metabolism (61). In addition, it was found associated with impaired glucose metabolism (62). Accordingly, we observed a positive association between this genus and high levels of IR. Bacteria belonging to Erysipelotrichaceae family use proteins and saccharides as main substrates for lactate production, one of the functional metabolic pathways involved in our study (60).
Unsurprisingly, we discovered that the taxa associated with IR were inferred to contribute to several of the GMM also associated with IR. However, we also found that the degree to which these taxa contributed to the respective functions was dependent on IR as well. This may have major implications for our understanding of the role of the microbiome in IR and indeed other conditions altering host gut metabolism. The altered gut metabolic environment promotes the relative abundance of different microbes that perform the same functions, in a manner similar to that described by Zhang and colleagues in their work on ecological guilds (63).
However, both in the taxonomic and functional aspect, previous studies shown discrepancies, perhaps partially explained by the inherent biases in metagenomic sequencing which make it problematic to estimate bacterial abundance from sequencing data (64). It is also important to mention that to overpass such bias we used a compositional approach for the microbiome analysis (65). Furthermore, is also worth to mention that the sequencing approach conducted in this study is also prone to bias, as described by Barb and colleagues (66). More complete and complex studies, including deepen microbial community profiling together with biochemical characterization of the environment, are needed in order to better understand the taxonomic and functional signatures related to IR.
There were several limitations in this study. First, due to the nature of 16S sequencing, we were limited to a genus-level resolution. Similarly, though PICRUSt2 has been shown to be reasonably reliable, especially in humans, we were restricted by the number and quality of the genomes present in the database used to infer the functional potential of the microbiome. For the same reasons, we were unable to assess the non-bacterial fraction of the microbiome. Whole genome shotgun metagenomics addresses both of these limitations, as it enables the detection and relative quantification of non-bacterial microbes as well as the bacteriome, and also enables analysis at a higher resolution and should be considered in the future. Another important point to consider is the large inherent variance in the microbiome, which is often confounded by host variables (67). The PREDIMED-Plus cohort by its nature consists late-middle aged and overweight participants. It is likely that some of the findings cannot be generalized to all ages and weights. Furthermore, this study only had a single microbiome measurement per participant, thus rendering stability and volatility analysis of the microbiome beyond our reach (68, 69). We eagerly await future studies and subsequent meta-analyses that will shed further light on this. Furthermore, given the observational nature of this study, it was not possible to conclude on causality or directionality. Future experiments using the fecal microbiota transplantation (FMT) technique could be considered to elucidate this last aspect.
In the current study we were able to identify taxa and functions associated with IR and to suggest that bacteria contribute differentially to these functions, also depending on IR. We observed genera Desulfovibrio, Odoribacter and Oscillospiraceae UCG-002 negatively associated with IR through mechanism of amino acid degradation that involves H2S, activation of gluconeogenesis, immunomodulation and beneficial effects of acetate. On the other hand, we observed that the positive association with IR involves genera such as Feacalibaterium and Butyricicoccus and GMM linked with the production of butyrate. To conclude, these findings are promising especially for the perspective of tailoring therapeutic strategies based on the identification of specific signatures of the gut bacterial community and using this information to improve the treatments for IR and other metabolic impairments related to obesity. However, some further investigation, especially under the metabolic point of view, are needed in order to strengthen these findings.
The datasets generated and analyzed during the current study are not publicly available due to data regulations and for ethical reasons, considering that this information might compromise research participants’ consent because our participants only gave their consent for the use of their data by the original team of investigators. However, collaboration for data analyses can be requested by sending a letter to the PREDIMED-Plus steering Committee (cHJlZGltZWRfcGx1c19zY29tbWl0dGVlQGdvb2dsZWdyb3Vwcy5jb20=). The request will then be passed to all the members of the PREDIMED-Plus Steering Committee for deliberation.
The study was conducted according to the guidelines of the Declaration of Helsinki, and approved by the Hospital Universitari Sant Joan de Reus Ethics Committee (protocol code 13-07-25/7proj2, date of approval: 25/07/2013), and the Comité de Ética de la Investigación Provincial de Málaga (protocol code Predimed+DM/01, date of approval: 27/11/2014). The patients/participants provided their written informed consent to participate in this study.
Conceptualization, AA, FJT, JeV, DC, JoV, MF, MB, and JSS. Formal analysis, AA and TFSB. Funding acquisition, FJT, JeV, DC, JoV, MF, and JSS. Writing—original draft preparation, AA. Writing—review and editing, TFSB, MB, and JSS. Supervision, MB and JSS. All authors have read and agreed to the published version of the manuscript.
This research was funded by the European Union’s Horizon 2020 research and innovation programme under the Marie Skłodowska-Curie grant agreement No. 713679 and from the Universitat Rovira i Virgili (URV). This work was supported by the official Spanish Institutions for funding scientific biomedical research, CIBER Fisiopatología de la Obesidad y Nutrición (CIBEROBN) and Instituto de Salud Carlos III (ISCIII), through the Fondo de Investigación para la Salud (FIS), which is co-funded by the European Regional Development Fund (three coordinated FIS projects led by JJS, including the following projects: PI13/00462, PI16/00501 and PI19/00576); the Especial Action Project entitled: Implementación y evaluación de una intervención intensiva sobre la actividad física Cohorte PREDIMED-Plus grant to JSS; the Recercaixa (number 2013ACUP00194) grant to JSS; The Fondo de Investigaciones Sanitarias of the Instituto de Salut Carlos III PI17/00215; The Generalitat Valenciana PROMETEO 17/2017 and PROMETEO 21/2021. None of the funding sources took part in the design, collection, analysis, interpretation of the data, or writing the report, or in the decision to submit the manuscript for publication. JSS, senior author of this study gratefully acknowledges the financial support by ICREA under the ICREA Academia programme. Food companies Hojiblanca (Lucena, Spain) and Patrimonio Comunal Olivarero (Madrid, Spain) donated extra virgin olive oil; and the Almond Board of California (Modesto, CA), American Pistachio Growers (Fresno, CA), and Paramount Farms (Wonderful Company, LLC, Los Angeles, CA) donated nuts for the PREDIMED- Pilot.
The authors declare that the research was conducted in the absence of any commercial or financial relationships that could be construed as a potential conflict of interest.
All claims expressed in this article are solely those of the authors and do not necessarily represent those of their affiliated organizations, or those of the publisher, the editors and the reviewers. Any product that may be evaluated in this article, or claim that may be made by its manufacturer, is not guaranteed or endorsed by the publisher.
We thank all the volunteers for the participation and personnel for the contribution in the PREDIMED-Plus trial. We also thank all the investigators of the PREDIMED-Plus study. CIBEROBN (Centros de Investigación Biomédica en Red: Obesidad y Nutrición) is an initiative of ISCIII, Madrid, Spain. The authors also thank the PREDIMED-Plus Biobank Network as a part of the National Biobank Platform of the ISCIII for storing and managing the PREDIMED-Plus biological samples. JSS, senior author of this study gratefully acknowledges the financial support by ICREA under the ICREA Academia programme. The research groups thanks for its support to the CIBER-IBIMA-Metagenomics platform, especially to Pablo Rodríguez.
The Supplementary Material for this article can be found online at: https://www.frontiersin.org/articles/10.3389/fendo.2022.804455/full#supplementary-material
1. Gurung M, Li Z, You H, Rodrigues R, Jump DB, Morgun A, et al. Role of Gut Microbiota in Type 2 Diabetes Pathophysiology. EBioMedicine (2020) 51:102590. doi: 10.1016/j.ebiom.2019.11.051
2. Martyn JAJ, Kaneki M, Yasuhara S, Warner DS, Warner MA. Obesity-Induced Insulin Resistance and Hyperglycemia. Anesthesiology (2008) 109:137–48. doi: 10.1097/ALN.0b013e3181799d45
3. Fahed M, Abou Jaoudeh MG, Merhi S, Mosleh JMB, Ghadieh R, Ghadieh R, et al. Evaluation of Risk Factors for Insulin Resistance: A Cross Sectional Study Among Employees at a Private University in Lebanon. BMC Endocr Disord (2020) 20:1–14. doi: 10.1186/s12902-020-00558-9
4. Saad MJA, Santos A, Prada PO. Linking Gut Microbiota and Inflammation to Obesity and Insulin Resistance. Physiology (2016) 31:283–93. doi: 10.1152/physiol.00041.2015
5. Bäckhed F, Ding H, Wang T, Hooper LV, Gou YK, Nagy A, et al. The Gut Microbiota as an Environmental Factor That Regulates Fat Storage. Proc Natl Acad Sci USA. (2004) 101:15718–23. doi: 10.1073/pnas.0407076101
6. Vrieze A, Van Nood E, Holleman F, Salojärvi J, Kootte RS, Bartelsman JFWM, et al. Transfer of Intestinal Microbiota From Lean Donors Increases Insulin Sensitivity in Individuals With Metabolic Syndrome. Gastroenterology (2012) 143:913–6.e7 doi: 10.1053/j.gastro.2012.06.031
7. Cani PD, Amar J, Iglesias MA, Poggi M, Knauf C, Bastelica D, et al. Metabolic Endotoxemia Initiates Obesity and Insulin Resistance. Diabetes (2007) 57:1761–72 doi: 10.2337/db06-1491
8. Amar J, Chabo C, Waget A, Klopp P, Vachoux C, Bermúdez-Humarán LG, et al. Intestinal Mucosal Adherence and Translocation of Commensal Bacteria at the Early Onset of Type 2 Diabetes: Molecular Mechanisms and Probiotic Treatment. EMBO Mol Med (2011) 3:559–72. doi: 10.1002/emmm.201100159
9. Caricilli AM, Picardi PK, de Abreu LL, Ueno M, Prada PO, Ropelle ER, et al. Gut Microbiota is a Key Modulator of Insulin Resistance in TLR 2 Knockout Mice. PloS Biol (2011) 14:e1002479. doi: 10.1371/journal.pbio.1001212
10. Medzhitov R, Horng T. Transcriptional Control of the Inflammatory Response. Nat Rev Immunol (2009) 9:692–703. doi: 10.1038/nri2634
11. Tan J, McKenzie C, Potamitis M, Thorburn AN, Mackay CR, Macia L. The Role of Short-Chain Fatty Acids in Health and Disease. Adv Immunol (2014) 91–119. doi: 10.1016/B978-0-12-800100-4.00003-9.
12. Li M, van Esch BCAM, Wagenaar GTM, Garssen J, Folkerts G, Henricks PAJ. Pro- and Anti-Inflammatory Effects of Short Chain Fatty Acids on Immune and Endothelial Cells. Eur J Pharmacol (2018) 831:52–9. doi: 10.1016/j.ejphar.2018.05.003
13. Ratajczak W, Rył A, Mizerski A, Walczakiewicz K, Sipak O, Laszczyńska M. Immunomodulatory Potential of Gut Microbiome-Derived Short-Chain Fatty Acids (SCFAs). Acta Biochim Pol (2019) 66:1–12. doi: 10.18388/abp.2018_2648
14. Perry RJ, Peng L, Barry NA, Cline GW, Zhang D, Cardone RL, et al. Acetate Mediates a Microbiome-Brain-β-Cell Axis to Promote Metabolic Syndrome. Nature (2016) 534:213–7. doi: 10.1038/nature18309
15. Wahlström A, Sayin SI, Marschall HU, Bäckhed F. Intestinal Crosstalk Between Bile Acids and Microbiota and Its Impact on Host Metabolism. Cell Metab (2016) 24:41–50. doi: 10.1016/j.cmet.2016.05.005
16. Gérard C, Vidal H. Impact of Gut Microbiota on Host Glycemic Control. Front Endocrinol (Lausanne) (2019) 10:29. doi: 10.3389/fendo.2019.00029
17. Pedersen HK, Gudmundsdottir V, Nielsen HB, Hyotylainen T, Nielsen T, Jensen BAH, et al. Human Gut Microbes Impact Host Serum Metabolome and Insulin Sensitivity. Nature (2016) 535:376–81. doi: 10.1038/nature18646
18. Salas-Salvadó J, Díaz-López A, Ruiz-Canela M, Basora J, Fitó M, Corella D, et al. Effect of a Lifestyle Intervention Program With Energy-Restricted Mediterranean Diet and Exercise on Weight Loss and Cardiovascular Risk Factors: One-Year Results of the PREDIMED-Plus Trial. Diabetes Care (2019) 44:777–88 doi: 10.2337/dc18-0836
19. Sayón-Orea C, Razquin C, Bulló M, Corella D, Fitó M, Romaguera D, et al. Effect of a Nutritional and Behavioral Intervention on Energy-Reduced Mediterranean Diet Adherence Among Patients With Metabolic Syndrome: Interim Analysis of the PREDIMED-Plus Randomized Clinical Trial. JAMA - J Am Med Assoc (2019) 322:1486–99. doi: 10.1001/jama.2019.14630
20. Matthews DR, Hosker JP, Rudenski AS, Naylor BA, Treacher DF, Turner RC. Homeostasis Model Assessment: Insulin Resistance and β-Cell Function From Fasting Plasma Glucose and Insulin Concentrations in Man. Diabetologia (1985) 28:412–9. doi: 10.1007/BF00280883
21. Schröder H, Zomeño MD, Martínez-González MA, Salas-Salvadó J, Corella D, Vioque J, et al. Validity of the Energy-Restricted Mediterranean Diet Adherence Screener. Clin Nutr (2021) 40:4971–9. doi: 10.1016/j.clnu.2021.06.030
22. MacPherson CW, Mathieu O, Tremblay J, Champagne J, Nantel A, Girard SA, et al. Gut Bacterial Microbiota and its Resistome Rapidly Recover to Basal State Levels After Short-Term Amoxicillin-Clavulanic Acid Treatment in Healthy Adults. Sci Rep (2018) 8:1–14. doi: 10.1038/s41598-018-29229-5
23. Callahan BJ, McMurdie PJ, Rosen MJ, Han AW, Johnson AJA, Holmes SP. DADA2: High-Resolution Sample Inference From Illumina Amplicon Data. Nat Methods (2016) 13:581–3. doi: 10.1038/nmeth.3869
24. Quast C, Pruesse E, Yilmaz P, Gerken J, Schweer T, Yarza P, et al. The SILVA Ribosomal RNA Gene Database Project: Improved Data Processing and Web-Based Tools. Nucleic Acids Res (2013) 41:D590–6. doi: 10.1093/nar/gks1219
25. Aitchison J, Barceló-Vidal C, Martín-Fernández JA, Pawlowsky-Glahn V. Logratio Analysis and Compositional Distance. Math Geol (2000) 32:271–5. doi: 10.1023/A:1007529726302
26. Chao A. Estimating the Population Size for Capture-Recapture Data With Unequal Catchability. Biometrics (1987) 43:783. doi: 10.2307/2531532
27. Shannon CE. A Mathematical Theory of Communication. Bell Syst Tech J (1948) 27:623–56. doi: 10.1002/j.1538-7305.1948.tb00917.x
29. Storey JD, Tibshirani R. Statistical Significance for Genomewide Studies. Proc Natl Acad Sci (2003) 100:9440–5. doi: 10.1073/pnas.1530509100
30. Douglas GM, Maffei VJ, Zaneveld JR, Yurgel SN, Brown JR, Taylor CM, et al. PICRUSt2 for Prediction of Metagenome Functions. Nat Biotechnol (2020) 38:685–8. doi: 10.1038/s41587-020-0548-6
31. Vieira-Silva S, Falony G, Darzi Y, Lima-Mendez G, Garcia Yunta R, Okuda S, et al. Species-Function Relationships Shape Ecological Properties of the Human Gut Microbiome. Nat Microbiol (2016) 1:1–8. doi: 10.1038/nmicrobiol.2016.88
32. The Human Microbiome Project Consortium. Structure, Function and Diversity of the Healthy Human Microbiome. Nature (2012) 486:207–14. doi: 10.1038/nature11234
33. Kushkevych I, Dordević D, Kollár P. Analysis of Physiological Parameters of Desulfovibrio Strains From Individuals With Colitis. Open Life Sci (2018) 13:481–8. doi: 10.1515/biol-2018-0057
34. Rowan F, Docherty NG, Murphy M, Murphy B, Coffey JC, O’Connell PR. Desulfovibrio Bacterial Species Are Increased in Ulcerative Colitis. Dis Colon Rectum (2010) 53:1530–6. doi: 10.1007/DCR.0b013e3181f1e620
35. Zhang C, Zhang M, Wang S, Han R, Cao Y, Hua W, et al. Interactions Between Gut Microbiota, Host Genetics and Diet Relevant to Development of Metabolic Syndromes in Mice. ISME J (2010) 4:232–41. doi: 10.1038/ismej.2009.112
36. Andoh A, Nishida A, Takahashi K, Inatomi O, Imaeda H, Bamba S, et al. Comparison of the Gut Microbial Community Between Obese and Lean Peoples Using 16S Gene Sequencing in a Japanese Population. J Clin Biochem Nutr (2016) 59:65–70. doi: 10.3164/jcbn.15-152
37. Karlsson CLJ, Önnerfält J, Xu J, Molin G, Ahrné S, Thorngren-Jerneck K. The Microbiota of the Gut in Preschool Children With Normal and Excessive Body Weight. Obesity (2012) 20:2257–61. doi: 10.1038/oby.2012.110
38. Garcia-Mantrana I, Selma-Royo M, Alcantara C, Collado MC. Shifts on Gut Microbiota Associated to Mediterranean Diet Adherence and Specific Dietary Intakes on General Adult Population. Front Microbiol (2018) 9:890. doi: 10.3389/fmicb.2018.00890
39. Pichette J, Fynn-Sackey N, Gagnon J. Hydrogen Sulfide and Sulfate Prebiotic Stimulates the Secretion of GLP-1 and Improves Glycemia in Male Mice. Endocrinology (2017) 158:3416–25. doi: 10.1210/en.2017-00391
40. Wang L, Li P, Tang Z, Yan X, Feng B. Structural Modulation of the Gut Microbiota and the Relationship With Body Weight: Compared Evaluation of Liraglutide and Saxagliptin Treatment. Sci Rep (2016) 6:33251. doi: 10.1038/srep33251
41. Neis EPJG, Dejong CHC, Rensen SS. The Role of Microbial Amino Acid Metabolism in Host Metabolism. Nutrients (2015) 7:2930–46 doi: 10.3390/nu7042930
42. Sircana A, Framarin L, Leone N, Berrutti M, Castellino F, Parente R, et al. Altered Gut Microbiota in Type 2 Diabetes: Just a Coincidence? Curr Diabetes Rep (2018) 18:98. doi: 10.1007/s11892-018-1057-6
43. Orsso CE, Peng Y, Deehan EC, Tan Q, Field CJ, Madsen KL, et al. Composition and Functions of the Gut Microbiome in Pediatric Obesity: Relationships With Markers of Insulin Resistance. Microorganisms (2021) 9:1–18. doi: 10.3390/microorganisms9071490
44. Zhao J, Zhang X, Liu H, Brown MA, Qiao S. Dietary Protein and Gut Microbiota Composition and Function. Curr Protein Pept Sci (2018) 20:145–54. doi: 10.2174/1389203719666180514145437
45. Hernández MAG, Canfora EE, Jocken JWE, Blaak EE. The Short-Chain Fatty Acid Acetate in Body Weight Control and Insulin Sensitivity. Nutrients (2019) 11:1943. doi: 10.3390/nu11081943
46. Gomez-Arango LF, Barrett HL, McIntyre HD, Callaway LK, Morrison M, Dekker Nitert M. Increased Systolic and Diastolic Blood Pressure is Associated With Altered Gut Microbiota Composition and Butyrate Production in Early Pregnancy. Hypertension (2016) 68:974–81. doi: 10.1161/HYPERTENSIONAHA.116.07910
47. Serena C, Ceperuelo-Mallafré V, Keiran N, Queipo-Ortuño MI, Bernal R, Gomez-Huelgas R, et al. Elevated Circulating Levels of Succinate in Human Obesity are Linked to Specific Gut Microbiota. ISME J (2018) 12:1642–57. doi: 10.1038/s41396-018-0068-2
48. Brahe LK, Le Chatelier E, Prifti E, Pons N, Kennedy S, Hansen T, et al. Specific Gut Microbiota Features and Metabolic Markers in Postmenopausal Women With Obesity. Nutr Diabetes (2015) 5:e159–7. doi: 10.1038/nutd.2015.9
49. Wang Y, Tang C, Tang Y, Yin H, Liu X. Capsaicin has an Anti-Obesity Effect Through Alterations in Gut Microbiota Populations and Short-Chain Fatty Acid Concentrations. Food Nutr Res (2020) 64:1–14. doi: 10.29219/fnr.v64.3525
50. Biassoni R, Di Marco E, Squillario M, Barla A, Piccolo G, Ugolotti E, et al. Gut Microbiota in T1DM-Onset Pediatric Patients: Machine-Learning Algorithms to Classify Microorganisms as Disease Linked. J Clin Endocrinol Metab (2020) 105:e3114–26. doi: 10.1210/clinem/dgaa407
51. Maya-Lucas O, Murugesan S, Nirmalkar K, Alcaraz LD, Hoyo-Vadillo C, Pizano-Zárate ML, et al. The Gut Microbiome of Mexican Children Affected by Obesity. Anaerobe (2019) 55:11–23. doi: 10.1016/j.anaerobe.2018.10.009
52. Lenoir M, Martín R, Torres-Maravilla E, Chadi S, González-Dávila P, Sokol H, et al. Butyrate Mediates Anti-Inflammatory Effects of Faecalibacterium Prausnitzii in Intestinal Epithelial Cells Through Dact3. Gut Microbes (2020) 12:1–16. doi: 10.1080/19490976.2020.1826748
53. Hippe B, Remely M, Aumueller E, Pointner A, Magnet U, Haslberger AG. Faecalibacterium Prausnitzii Phylotypes in Type Two Diabetic, Obese, and Lean Control Subjects. Benef Microbes (2016) 7:511–7. doi: 10.3920/BM2015.0075
54. Barlow GM, Yu A, Mathur R. Role of the Gut Microbiome in Obesity and Diabetes Mellitus. Nutr Clin Pract (2015) 30:787–97. doi: 10.1177/0884533615609896
55. Ma Q, Li Y, Wang J, Li P, Duan Y, Dai H, et al. Investigation of Gut Microbiome Changes in Type 1 Diabetic Mellitus Rats Based on High-Throughput Sequencing. BioMed Pharmacother (2020) 124:109873. doi: 10.1016/j.biopha.2020.109873
56. Liu H, Wang J, He T, Becker S, Zhang G, Li D, et al. Butyrate: A Double-Edged Sword for Health? Adv Nutr (2018) 9:21–9. doi: 10.1093/advances/nmx009
57. Blandino G, Inturri R, Lazzara F, Di Rosa M, Malaguarnera L. Impact of Gut Microbiota on Diabetes Mellitus. Diabetes Metab (2016) 42:303–15. doi: 10.1016/j.diabet.2016.04.004
58. Bibbò S, Ianiro G, Giorgio V, Scaldaferri F, Masucci L, Gasbarrini A, et al. The Role of Diet on Gut Microbiota Composition. Eur Rev Med Pharmacol Sci (2016) 20:4742–9.
59. Louis P, Flint HJ. Formation of Propionate and Butyrate by the Human Colonic Microbiota. Environ Microbiol (2017) 19:29–41. doi: 10.1111/1462-2920.13589
60. Oliphant K, Allen-Vercoe E. Macronutrient Metabolism by the Human Gut Microbiome: Major Fermentation by-Products and Their Impact on Host Health. Microbiome (2019) 7:1–15. doi: 10.1186/s40168-019-0704-8
61. Kaakoush NO. Insights Into the Role of Erysipelotrichaceae in the Human Host. Front Cell Infect Microbiol (2015) 5:84. doi: 10.3389/fcimb.2015.00084
62. Lippert K, Kedenko L, Antonielli L, Kedenko I, Gemeier C, Leitner M, et al. Gut Microbiota Dysbiosis Associated With Glucose Metabolism Disorders and the Metabolic Syndrome in Older Adults. Benef Microbes (2017) 8:545–56. doi: 10.3920/BM2016.0184
63. Zhang C, Yin A, Li H, Wang R, Wu G, Shen J, et al. Dietary Modulation of Gut Microbiota Contributes to Alleviation of Both Genetic and Simple Obesity in Children. EBioMedicine (2015) 2:968–84. doi: 10.1016/j.ebiom.2015.07.007
64. McLaren MR, Willis AD, Callahan BJ. Consistent and Correctable Bias in Metagenomic Sequencing Experiments. Elife (2019) 8:1–31. doi: 10.7554/eLife.46923
65. Gloor GB, Macklaim JM, Pawlowsky-Glahn V, Egozcue JJ. Microbiome Datasets are Compositional: And This is Not Optional. Front Microbiol (2017) 8:2224. doi: 10.3389/fmicb.2017.02224
66. Barb JJ, Oler AJ, Kim H-S, Chalmers N, Wallen GR, Cashion A, et al. Development of an Analysis Pipeline Characterizing Multiple Hypervariable Regions of 16S rRNA Using Mock Samples. PloS One (2016) 11:e0148047. doi: 10.1371/journal.pone.0148047
67. Vujkovic-Cvijin I, Sklar J, Jiang L, Natarajan L, Knight R, Belkaid Y. Host Variables Confound Gut Microbiota Studies of Human Disease. Nature (2020) 587:448–54. doi: 10.1038/s41586-020-2881-9
68. Vandeputte D, De Commer L, Tito RY, Kathagen G, Sabino J, Vermeire S, et al. Temporal Variability in Quantitative Human Gut Microbiome Profiles and Implications for Clinical Research. Nat Commun (2021) 12:6740. doi: 10.1038/s41467-021-27098-7
Keywords: fecal microbiota, insulin resisitance, HOMA-IR, 16S sequencing, gut metabolic modules
Citation: Atzeni A, Bastiaanssen TFS, Cryan JF, Tinahones FJ, Vioque J, Corella D, Fitó M, Vidal J, Moreno-Indias I, Gómez-Pérez AM, Torres-Collado L, Coltell O, Castañer O, Bulló M and Salas-Salvadó J (2022) Taxonomic and Functional Fecal Microbiota Signatures Associated With Insulin Resistance in Non-Diabetic Subjects With Overweight/Obesity Within the Frame of the PREDIMED-Plus Study. Front. Endocrinol. 13:804455. doi: 10.3389/fendo.2022.804455
Received: 29 October 2021; Accepted: 21 March 2022;
Published: 28 April 2022.
Edited by:
Matthias Johannes Betz, University Hospital of Basel, SwitzerlandReviewed by:
Elena Rampanelli, Amsterdam University Medical Center, NetherlandsCopyright © 2022 Atzeni, Bastiaanssen, Cryan, Tinahones, Vioque, Corella, Fitó, Vidal, Moreno-Indias, Gómez-Pérez, Torres-Collado, Coltell, Castañer, Bulló and Salas-Salvadó. This is an open-access article distributed under the terms of the Creative Commons Attribution License (CC BY). The use, distribution or reproduction in other forums is permitted, provided the original author(s) and the copyright owner(s) are credited and that the original publication in this journal is cited, in accordance with accepted academic practice. No use, distribution or reproduction is permitted which does not comply with these terms.
*Correspondence: Monica Bulló, bW9uaWNhLmJ1bGxvQHVydi5jYXQ=; Jordi Salas-Salvadó, am9yZGkuc2FsYXNAdXJ2LmNhdA==
Disclaimer: All claims expressed in this article are solely those of the authors and do not necessarily represent those of their affiliated organizations, or those of the publisher, the editors and the reviewers. Any product that may be evaluated in this article or claim that may be made by its manufacturer is not guaranteed or endorsed by the publisher.
Research integrity at Frontiers
Learn more about the work of our research integrity team to safeguard the quality of each article we publish.