- 1Department of Endocrine and Metabolic Diseases, Shanghai Institute of Endocrine and Metabolic Diseases, Ruijin Hospital, Shanghai Jiaotong University School of Medicine, Shanghai, China
- 2Shanghai National Clinical Research Center for Metabolic Diseases, Key Laboratory for Endocrine and Metabolic Diseases of the National Health Commission of the PR China, Shanghai National Center for Translational Medicine, Ruijin Hospital, Shanghai Jiaotong University School of Medicine, Shanghai, China
- 3Department of Endocrinology, The Third People’s Hospital of Datong, Datong, China
Aim: We aimed to detect the individual and combined effect of glucose metabolic components on cognitive function in particular domains among older adults.
Methods: Data of 2,925 adults aged over 60 years from the 2011 to 2014 National Health and Nutrition Examination Survey were analyzed. Individuals’ cognitive function was evaluated using the Digit Symbol Substitution Test (DSST), the Animal Fluency Test (AF), the Consortium to Establish a Registry for Alzheimer’s Disease Immediate Recall (CERAD-IR), and CERAD Delayed Recall (CERAD-DR). Participants’ glucose metabolic health status was determined based on fasting plasma glucose, insulin, homeostasis model assessment of insulin resistance (HOMA-IR), glycated hemoglobin (HbA1c), and 2-h postload glucose. Linear regression models were used to delineate the associations of cognitive function with individual glucose metabolic component and with metformin use. Logistic regression models were performed to evaluate the associations of cognition with the number of glucose metabolic risk components.
Results: CERAD-IR was significantly associated with HOMA-IR and insulin. HbA1c was related to all the cognitive tests except AF. Among participants without obesity, HOMA-IR and insulin were both negatively associated with CERAD-IR and CERAD-DR. Odds of scoring low in DSST increased with the number of glucose metabolic risk components (odds ratio 1.94, 95% confidence interval [CI] 1.26 to 2.98). Metformin use was associated with better performance in DSST among diabetes patients (β = 4.184, 95% CI 1.655 to 6.713).
Conclusions: Our findings support the associations of insulin resistance and glycemic level with cognitive function in key domains, especially among adults without obesity. There is a positive association between metformin use and cognition.
Introduction
The prevalence of type 2 diabetes has increased remarkably over the past decades (1), imposing huge burden on healthcare expenditure. Dementia has witnessed a similar population trend. The number of people living with dementia worldwide in 2015 was estimated at 47.47 million, and reaching 135.46 million by 2050 (2). Previous literatures demonstrated increased odds of cognitive impairment in relation to diabetes (3); the former, in turn, results in worse diabetes management, more frequent occurrence of severe hypoglycemic episodes, an increased risk of cardiovascular events, and death (4). Therefore, comprehensive understanding and management of glucose metabolic risk factors have become an imperative issue for the prevention of cognitive dysfunction.
With respect to risk factors for cognitive impairment among patients with diabetes, glucose metabolic components, including glycemic level and insulin resistance, have attracted much attention. Some studies have shown that high glycated hemoglobin (HbA1c) was detrimentally related to cognitive functions (5), whereas limited research has focused on the associations of cognition with other glucose metabolic indicators, such as fasting plasma glucose (FPG) and 2-h postload glucose (2h-PG). Regarding disorders of insulin homeostasis, converging evidence has shown that insulin resistance or consequent hyperinsulinemia is related to poor cognitive performance (6). However, most of these studies focused on the relationship of global cognitive function with glycemic level or insulin homeostasis separately, and few have systematically described individual and combined associations of glucose metabolic components with cognition in particular domains, such as learning, memory, processing speed, executive function, and language.
Additionally, given the relationship of obesity with both diabetes (7) and neurodegenerative diseases (8), it is of substantial interest to investigate whether any potential association of glucose metabolic components with domain-specific cognitive functions could be modified by obesity status. Furthermore, since diabetes medications, especially metformin, have been implicated to be associated with slowed rate of cognitive decline by previous studies (9, 10), we also tested this hypothesis in the current study population.
Several studies have investigated associations between diabetes and cognitive function using data from earlier National Health and Nutrition Examination Survey (NHANES), which lacked information on cognitive function in key domains measured comprehensively among older adults. Two studies based on 1999–2002 NHANES (11, 12) assessed the association of FPG, insulin resistance, and metabolic syndrome with cognition, but only implemented Digit Symbol Substitution Test (DSST) to evaluate cognitive function. A 1988–1994 NHANES study (13) investigated the associations between diabetes combined with hypertension and cognitive function, but the study population was restricted to individuals aged between 20 and 59, so the external validity for elder populations is uncertain. Another study (14) using NHANES 2011–2014 cycles, found lower DSST and Animal Fluency Test (AF) scores in relation to higher HbA1c. However, evidence regarding the relationship of cognitive dysfunction with a broad spectrum of glucose metabolic components, such as insulin resistance, obesity status, and metformin usage, is still limited.
Therefore, taking advantage of NHANES, a nationally representative sample of US older adults, we aimed to determine the individual and combined associations of glucose metabolic components with cognitive functions in key domains, and whether such associations were modified by obesity status. We also test the hypothesis that metformin use is associated with better cognitive function in elder adults with diabetes.
Materials and Methods
Study Population
NHANES is a nationwide continuous cross-sectional survey that assessed nutritional and health status of civilians in the United States. The survey was performed by the National Center for Health Statistics (https://www.cdc.gov/nchs/nhanes/index.htm) and had a complex, multistage, stratified sampling design in order to provide nationally representative data. We conducted an analysis using the data collected from the adults aged over 60 years who participated in the NHANES 2011 to 2014 cycles. In the NHANES 2011–2012 survey, the unweighted response rate for participants aged 60–70, 70–80, and over 80 were 62.7%, 56.1%, and 46.4% respectively. In the NHANES 2013-2014 survey, the unweighted response rate for participants aged 60–70, 70–80, and over 80 were 62.9%, 59.8%, and 45.2% (https://wwwn.cdc.gov/nchs/nhanes/responserates.aspx). Participants without response to cognitive functioning tests (n = 286), without data of glucose metabolic components (n = 247), and those who were taking prescribed medications to treat obesity (n = 14) were excluded, yielding a primary analytical sample of 2,925, including 511 patients with diabetes who were using oral hypoglycemic agents or insulin (Figure 1). The survey collected data first through interviews at participants’ home by experienced interviewers using Computer-Assisted Personal Interviewing system, with a subsequent visit to a mobile examination center (MEC) where some clinical assessments and collection of biological specimens were carried out. The National Center for Health Statistics ethics review board reviewed and approved the survey and participants of NHANES gave informed consent prior to participation.
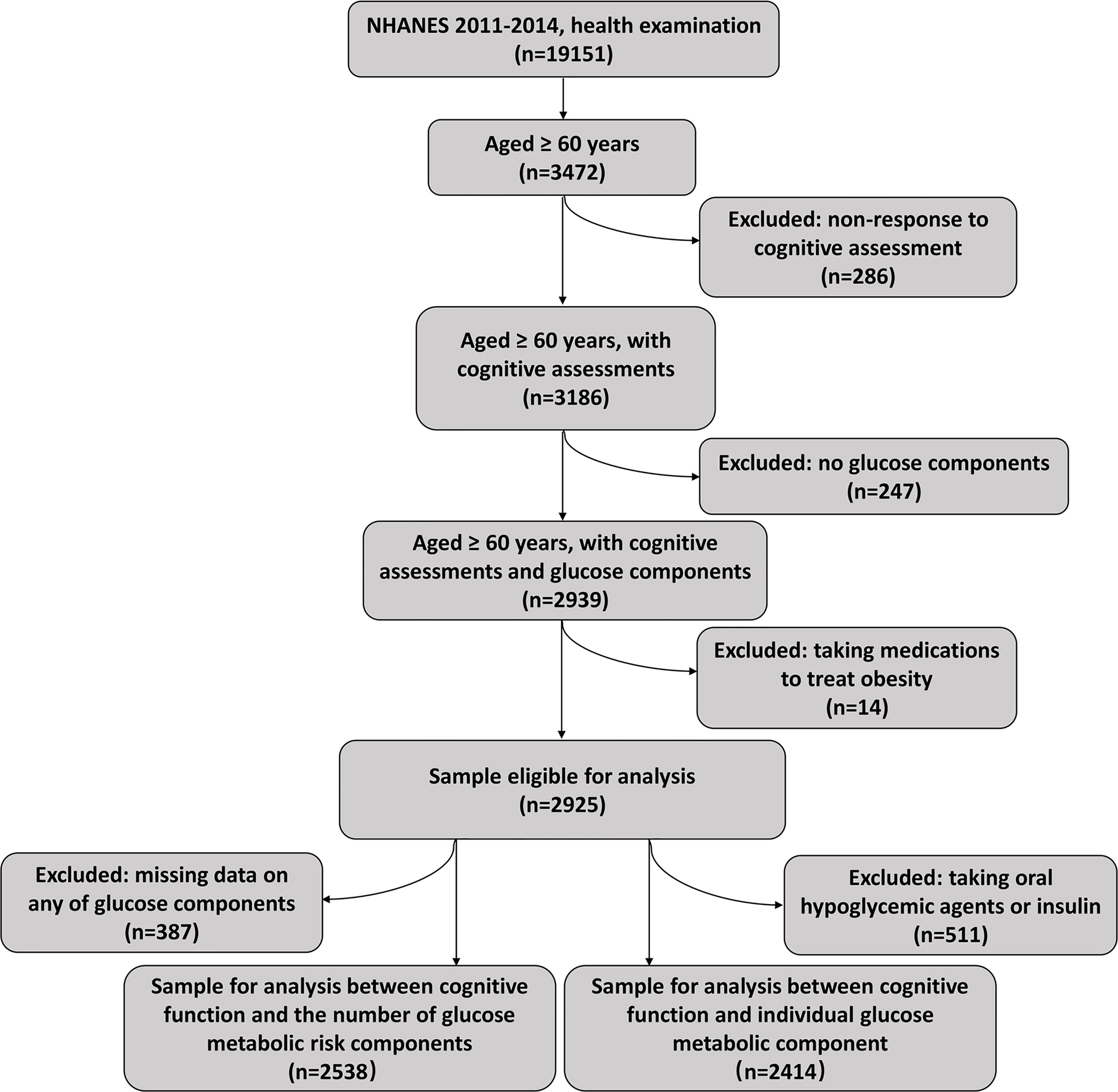
Figure 1 Flow chart showing pipeline of selecting subjects using NHANES 2011–2014. NHANES, National Health and Nutrition Examination Survey.
Cognitive Functioning Assessment
Cognitive function was evaluated during the in-home interview using four separate tests that have been validated in American communities (15–17), including the Consortium to Establish a Registry for Alzheimer’s Disease Immediate Recall (CERAD-IR), the Consortium to Establish a Registry for Alzheimer’s Disease Delayed Recall (CERAD-DR), the AF, and the DSST.
CERAD-IR and CERAD-DR comprehensively measure both learning ability and memory (18). The CERAD-IR test consists of three consecutive learning trials with a maximum total score of 30. Participants were asked to read 10 unrelated words at the beginning and repeat the words as many as possible in each learning trial. The CERAD-DR occurred after the other cognitive tests (AF and DSST) were completed, in which the participants recalled the words presented in the CERAD-IR test. The AF evaluates categorical verbal fluency by counting the number of animals named by the participant in 1 min (19). The DSST is a widely used evaluation tool assessing processing speed, sustained attention, and working memory (20). The test was conducted using a paper form containing nine digit–symbol pairs. For this test, the participants had to fill in the blank boxes next to the digit with corresponding symbols within 2 min. In line with previous literature, we used the criteria of <17 for CERAD-IR (21), <5 for CERAD-DR (21), <14 for AF (22), and <34 for DSST (23) to identify potential cognitive impairment.
Glucose Metabolic Components
HbA1c measures were available for the entire sample and were conducted through a high-performance liquid chromatography method. Participants who were randomly assigned to the morning session were eligible for FPG and insulin measures, whereas only part of the morning session participants were suitable for a subsequent oral glucose tolerance test (OGTT). The exclusion criteria for OGTT included hemophilia or chemotherapy patients, fasting less than 9 h, refusing phlebotomy, failing to drink entire glucose solution, or currently receiving diabetes treatment. After the FPG test, participants were asked to drink a solution containing approximately 75 g of glucose and have a second venipuncture 2 h later. AIA-PACK IRI, a two-site immunoenzymometric assay, was performed to quantitively measure insulin in serum specimens; plasma glucose was measured using hexokinase assay. The homeostatic model assessment of insulin resistance (HOMA-IR) was calculated as [fasting glucose(mmol/L)] × [plasma insulin (μIU/ml)]/22.5 (24) and used as surrogate measurement of insulin resistance. Details of lab method, lab equipment, and lab site were described elsewhere (25, 26). According to the American Diabetes Association 2014 criteria (27), diabetes was defined as FPG ≥ 7.0 mmol/L, 2h-PG ≥ 11.1 mmol/L, or HbA1c ≥ 6.5%. In the current study, the presence of diabetes was defined as FPG ≥ 7.0 mmol/L, 2h-PG ≥ 11.1 mmol/L, HbA1c ≥ 6.5%, and receiving oral hypoglycemic agent treatment or insulin treatment. We calculated the number of glucose metabolic risk factors according to the presence of five glucose metabolic risk factors (1): FPG ≥ 7.0 mmol/L (2); HbA1c ≥ 6.5% (3); HOMA-IR ≥ 4.11 (highest quartile) (4); receive hypoglycemic agent treatment; and (5) receive insulin treatment. The glucose metabolic health status was defined based on the number of glucose metabolic risk factors and thus classified into three categories: the healthiest glucose metabolism (without any risk factor), moderately healthy glucose metabolism (1–3 risk factors), and the least healthy glucose metabolism (4–5 risk factors).
Covariates
Demographic statistics, sleep disorder, history of stroke, history of hyperlipidemia, smoking status, alcohol consumption, prescription medication, and mental health data were collected in the in-home interviews. In terms of age, responses of participants who were more than 80 years old were coded as “80” in order to protect participants’ privacy. Depressive symptoms were evaluated through Patient Health Questionnaire (PHQ-9), a nine-item depression screener that asked questions on the frequency of depression symptoms in the past 2 weeks. Each item was scored on a scale of 1–3 with a maximum total score of 27; higher scores represent more severe depression (28). Currently smoking was defined as having smoked at least 100 cigarettes in life, and current alcohol consumption was defined as having at least 12 alcohol drinks in the past year. Information on health status (history of stroke, history of hyperlipidemia, and sleep disorder) was collected by asking the questions “have you ever been told by a doctor or other health professional that you have stroke/hyperlipidemia/sleep disorder?”
Body and blood pressure (BP) measurements were taken at MEC by trained technicians. The BP examiners asked the participants to sit quietly for 5 min and subsequently obtained three consecutive systolic blood pressure (SBP) readings. Participants without suitable BP cuff, or with rashes, gauze dressings, casts, edema, paralysis, tubes, open sores or wounds, withered arms, a-v shunts, and radical mastectomy on both arms were excluded. Body mass index (BMI) was calculated as the weight in kilograms divided by height in meters squared. We applied BMI ≥ 30 kg/m2, a cutoff recommended for US adults (29), to identify obesity.
Statistical Analysis
All statistical analyses were conducted using Stata version 11.0 and R version 4.0.3, accounting for the complex sampling design in NHANES. Each person in the survey was assigned a sample weight that was created by three steps (1): calculation of the base weight according to the possibility that his/her county, city block, household, and then herself/himself is selected (2); adjustment for nonresponse; and (3) post stratification adjustment to match the US Census population. Weights of the 2011–2012 cycle and 2013–2014 cycle were combined to create 4-year survey weights to adjust for unequal selection probability and non-response bias (30). Weights of the variable that were collected on the smallest number of respondents were recommended to be applied during analysis.
Weighted means with standard deviations for normally distributed data, medians with 25th–75th percentiles for skewed distributed variables, and percentages of categorical data were calculated. We performed t-tests for continuous data and design-based χ2 tests for categorical data to determine statistical difference between characteristics of participants with normal and low scores in each cognitive test. Insulin and HOMA-IR were log-transformed before statistical comparisons owing to their nonnormal, positively skewed distributions. First, we used multivariable linear regression models to delineate the individual association of glucose metabolic components with cognitive function among participants without using oral hypoglycemic agents and insulin. To estimate whether the associations of individual glucose metabolic component with cognitive functions were modified by obesity status, we replicated linear regression analyses in both BMI stratification categories (<30 kg/m2 and ≥ 30 kg/m2). Design-based t-tests were used to determine difference between cognitive scores between patients using metformin and using other anti-diabetic medications. Linear regression analysis was performed to evaluate relationship between metformin use and cognitive function among 511 patients who were taking medications used to treat diabetes. Multiple logistic regression models and 95% confidence intervals (CIs) were used to estimate the odds of scoring low in different cognitive tests that are associated with the number of glucose metabolic risk components. Non-response to cognitive testing and measurement of glucose components for any reason was treated as missing data and not included in the analyses.
The covariates adjusted in the models are presented as follows: sex (male and female), race (Hispanic, White, Black, and Asian), education (<9th grade, 9–11th grade, high school, some college, and ≥college), sleep disorder (yes and no), history of stroke (yes and no), smoking (yes and no), alcohol consumption status (yes and no), history of hyperlipidemia (yes and no), BMI (continuous data), SBP (continuous data), and PHQ-9 scores (continuous data). Three models were generated for the analysis. Model 1 was univariate, and Model 2 was adjusted for age, sex, race, education level, PHQ-9 scores, sleep disorder, history of stroke, history of hyperlipidemia, BMI, and SBP. Model 3 was additionally adjusted for alcohol consumption and smoking. We selected Model 3 as the main adjustment model.
We used the software of Power Analysis & Sample Size 11.0 to conduct the power calculation (31). According to a previous published study that had evaluated the association between HbA1c and cognitive assessment score (14), we calculated the statistical power of the present study by using the following parameters (1): a two-sided test at the 5% level (2); the null hypothesis of β of linear regression is 0 (3); the standard deviations of the HbA1c in our study was 0.54 (4); an estimation of the correlation between HbA1c and cognitive assessment score was 0.1 (5); the sample size is 2,414 (6); the β of linear regression between HbA1c and CERAD-IR, CERAD-DR, DSST, and AF was −0.19, −0.51, −2.55, and −0.64 reported by the previous published study, respectively (14). The calculated statistical powers were all over 90%.
Results
Description of Population Characteristics
Table 1 presents the characteristics of 2,925 participants. Participants scoring low in cognitive tests were significantly older and had higher SBP and higher 2h-PG than those with normal cognitive function. Differences were also observed in race and education constituent ratios between participants with normal and low cognitive scores. There was higher prevalence of impaired reaction on CERAD-IR and CERAD-DR among women compared with men, whereas gender difference was not significant regarding DSST and AF.
As shown in Figure 2, diabetes patients accounted for about 30% of participants who scored low in CERAD-IR, CERAD-DR, and AF. It is notable that more than 36% of individuals who scored low in DSST were patients with diabetes. By contrast, diabetes patients only accounted for less than a quarter of those with normal cognitive scores. The differences between participants with normal and low cognitive scores were statically significant according to design-based χ2 tests (p = 0.047 for CERAD-IR; p = 0.020 for CERAD-DR; p = 0.010 for AF; p < 0.001 for DSST).
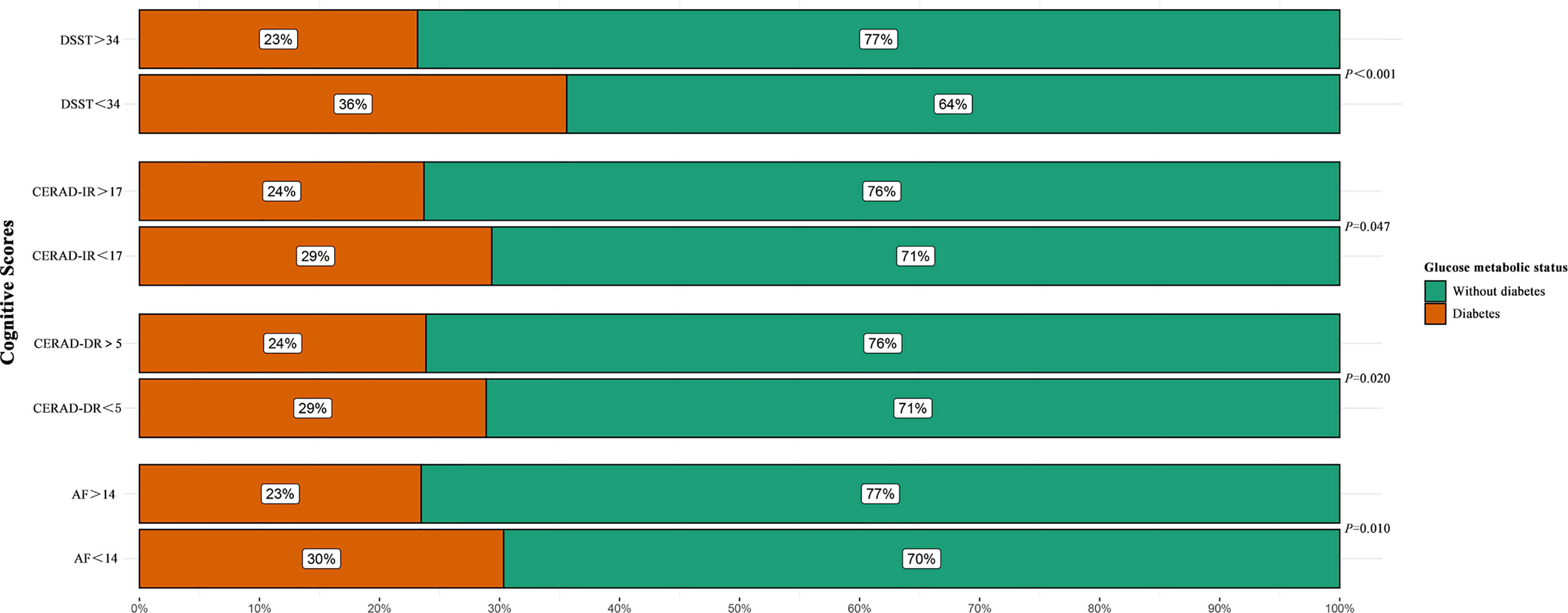
Figure 2 Percentages of patients with diabetes among participants with normal and low scores in each cognitive test. Design-based χ2 test was performed to determine the difference between the constituent ratios of diabetes among participants with normal and low scores in each cognitive test. AF, Animal Fluency Test; CERAD-IR, Consortium to Establish a Registry for Alzheimer’s Disease Immediate Recall; CERAD-DR, Consortium to Establish a Registry for Alzheimer’s Disease Delayed Recall; DSST, Digit Symbol Substitution Test.
Individual Association of Glucose Metabolic Components With Cognitive Function
Table 2 shows the coefficients of the relationships of individual glucose metabolic component with cognitive scores. We found significant associations of 2h-PG with scores of CERAD-IR, CERAD-DR, and DSST in Model 1, but such associations were only maintained for CERAD-DR (β = −0.070, 95% CI −0.134 to −0.005) in the fully adjusted model. HbA1c was inversely associated with scores in all four cognitive tests in the unadjusted model, and the association remained robust even after adjustment for all the confounding factors for CERAD-IR (β = −0.319, 95% CI −0.585 to −0.053), CERAD-DR (β = −0.227, 95% CI −0.405 to −0.050), and DSST (β = −1.914, 95% CI −3.537 to −0.291), but not for AF. The significant negative association of CERAD-IR with HOMA-IR (β = −0.830, 95% CI −1.655 to −0.005) and insulin (β = −1.013, 95% CI −1.969 to −0.058) was detected after adjustment of covariates. We did not observe any association between FPG and cognitive function.
Individual Association of Glucose Metabolic Components With Cognitive Function Stratified by Obesity Status
As shown in Table 3, in the BMI stratified analysis, we found more prominent associations of individual glucose metabolic component with cognitive functions among adults without obesity (BMI < 30 kg/m2) after adjustment for age, gender, race, education, sleep disorder, history of stroke, history of hyperlipidemia, BMI, SBP, smoking, and alcohol consumption. Among participants with BMI < 30 kg/m2, HbA1c and HOMA-IR were negatively associated with CERAD-IR (HOMA-IR: β = −1.778, 95% CI −2.842 to −0.714; HbA1c: β = −0.469, 95% CI −0.907 to −0.031) and CERAD-DR (HOMA-IR: β = −0.842, 95% CI −1.399 to −0.284; HbA1c: β = −0.310, 95% CI −0.572 to −0.049). Insulin was reversely associated with cognitive function based on CERAD-IR (β = −2.053, 95% CI −3.283 to −0.822) and CERAD-DR (β = −1.034, 95% CI −1.680 to −0.387). It is notable that no significant association was found between FPG and any cognitive test in both BMI categories.
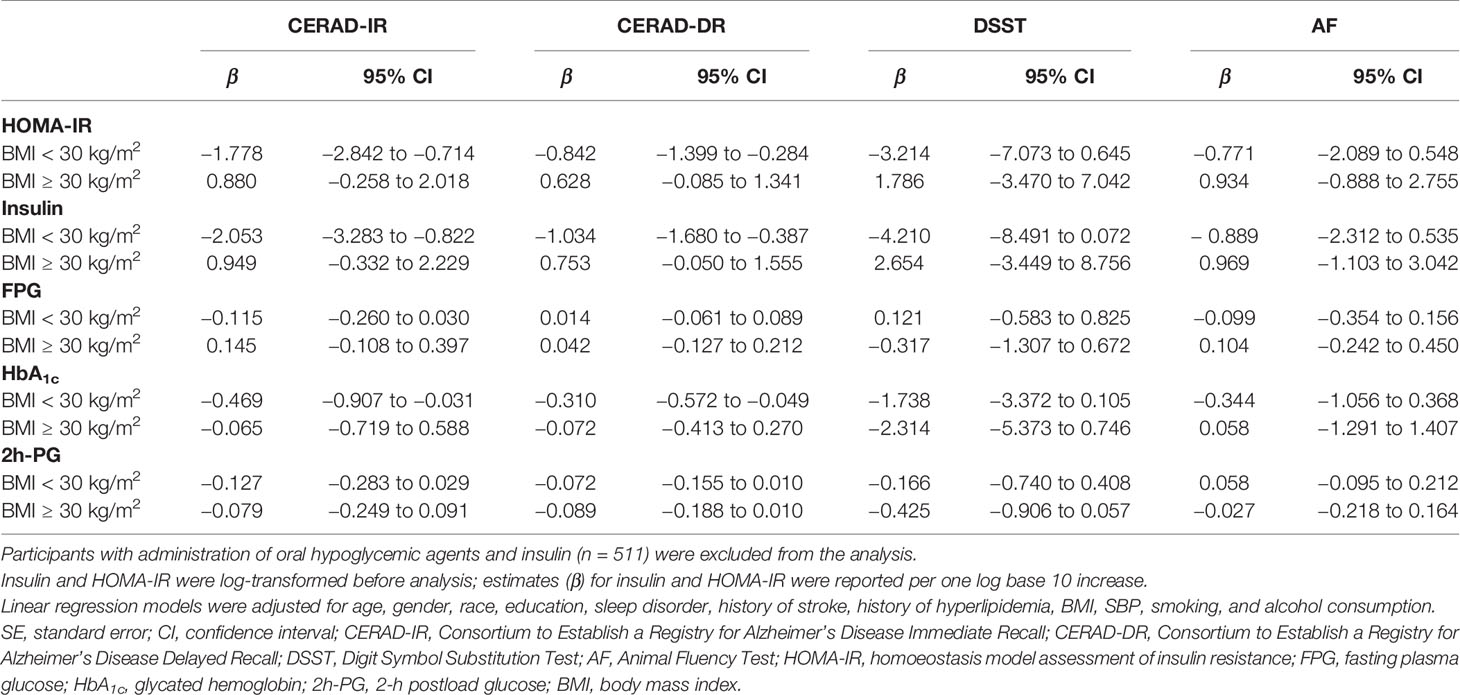
Table 3 Individual association of glucose metabolic components with cognitive function stratified by obesity status.
Combined Associations of Glucose Metabolic Components With Cognitive Function
After adjusting for covariates, ORs of cognitive impairment based on CERAD-IR, CERAD-DR, and AF tests increased with the number of glucose metabolic risk components but did not reach statistical significance. Importantly, participants with least healthy glucose metabolic status (four to five risk components) have nearly doubled OR of scoring low in DSST compared with those with the healthiest glucose metabolic status (OR 1.94, 95% CI 1.26 to 2.98; Figure 3).
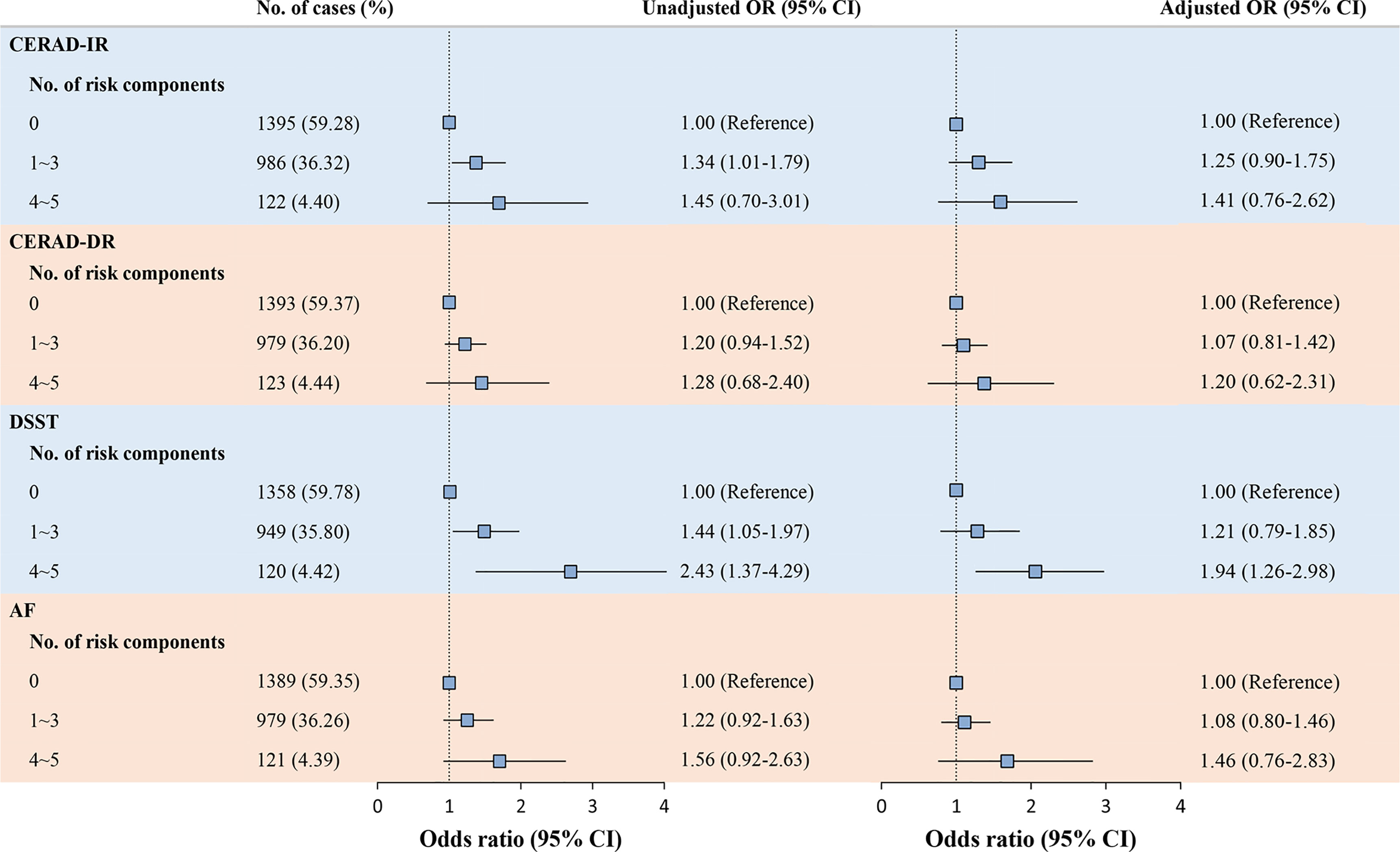
Figure 3 Associations of the number of glucose metabolic risk components with cognitive function. Participants with missing data on any of the glucose components (n = 387) were excluded from the analysis. Logistic regression models were used to generate odds ratios (ORs) and corresponding 95% confidence intervals (95% CIs) to detect the odds of cognitive impairment, adjusted for age, gender, race, education, sleep disorder, history of stroke, history of hyperlipidemia, BMI, SBP, smoking, and alcohol consumption. AF, Animal Fluency Test; BMI, body mass index; CERAD-IR, Consortium to Establish a Registry for Alzheimer’s Disease Immediate Recall; CERAD-DR, Consortium to Establish a Registry for Alzheimer’s Disease Delayed Recall; DSST, Digit Symbol Substitution Test; SBP, systolic blood pressure.
Associations of Metformin Use With Cognitive Function
Among 511 participants who were taking oral hypoglycemic agents or using insulin to treat diabetes, 311 were using metformin. As shown in Figure 4, patients using metformin scored significantly higher in DSST and AF, compared with patients who were using other anti-diabetic medications. In the linear regression analysis (Table 4), we found significant associations of metformin use with better language fluency, as evaluated with AF (β = 1.362, 95% CI 0.270 to 2.454), and better executive function, as evaluated by DSST (β = 7.406, 95% CI 4.373 to 10.440). After adjusting for aforementioned covariates, metformin use remained significantly associated with better executive function based on DSST (β = 4.184, 95% CI 1.655 to 6.713).
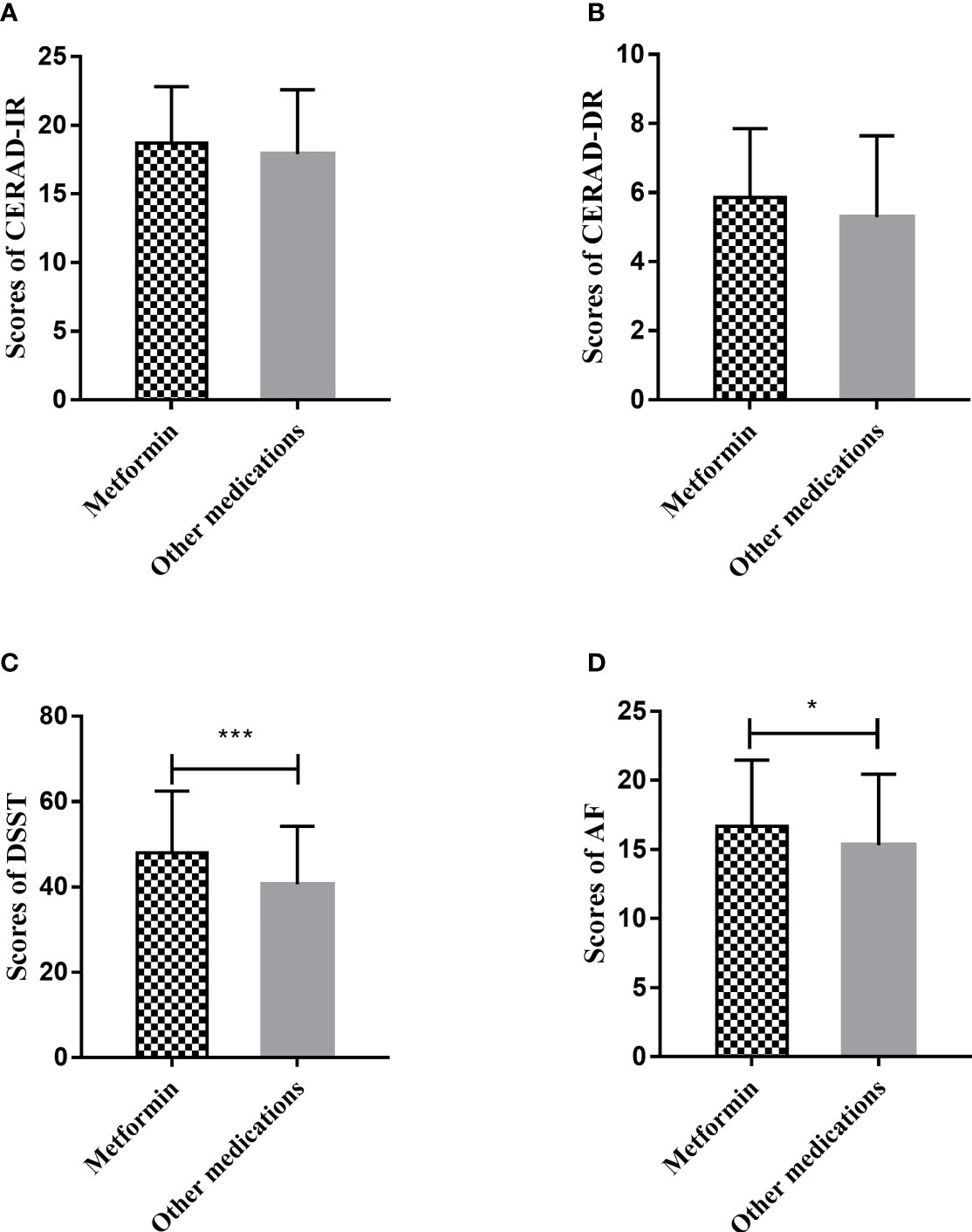
Figure 4 Cognitive scores of diabetes patients according to whether they are using metformin. (A) Comparison of CERAD-IR scores of patients using metformin and that of patients using other anti-diabetic medications. (B) Comparison of CERAD-DR scores of patients using metformin and that of patients using other anti-diabetic medications. (C) Comparison of DSST scores of patients using metformin and that of patients using other anti-diabetic medications. (D) Comparison of AF scores of patients using metformin and that of patients using other anti-diabetic medications. Design-based t-test was performed to determine the difference between cognitive scores of diabetes patients taking metformin and those taking other anti-diabetic medications. *p < 0.05; ***p < 0.001. AF, Animal Fluency Test; CERAD-IR, Consortium to Establish a Registry for Alzheimer’s Disease Immediate Recall; CERAD-DR, Consortium to Establish a Registry for Alzheimer’s Disease Delayed Recall; DSST, Digit Symbol Substitution Test.
Discussion
To our knowledge, our study is the first to examine the individual and combined associations of glucose metabolic components with domain-specific cognitive function comprehensively among US older adults. Our study demonstrated that severe insulin resistance estimated using HOMA-IR and insulin concentration and high glycemic level estimated using HbA1c and 2h-PG were negatively associated with cognitive function in key domains except for verbal fluency, especially among participants without obesity. Patients with four to five glucose metabolic risk components had significantly worse performance in DSST, indicating poorer processing speed, sustained attention, and working memory in relation to worse glucose metabolism. Furthermore, metformin use was associated with executive function as evaluated by DSST among diabetes patients.
The association of HbA1c and cognitive impairment is still debated. Elevated HbA1c level has been shown to be related to increased odds of dementia in epidemiological studies (32), though not all studies found such associations (33). A recent study (14) carried out in the NHANES 2011–2014 population did find cognitive impairment in relation to elevated HbA1c. Our study, however, extended the knowledge by comprehensively taking multiple glycemic and insulin resistance indicators, obesity status, and anti-diabetic medications into account.
Studies examining other glucose metabolic components, such as FPG and 2h-PG, in relation to cognitive outcomes are more limited. Most studies reported no relation between FPG concentration and cognition (5), consistent with findings of our study. The level of 2h-PG indicates moderate to severe insulin resistance and impaired late-phase insulin secretory response to OGTT; thus, it is different from FPG with regard to pathophysiology and odds of diabetes-related clinical outcomes (34). The Hisayama Study (35) reported increased odds of all-cause dementia, Alzheimer’s disease, and vascular dementia in relation to elevated 2h-PG, rather than FPG. Given the fact that memory loss is the key symptom of Alzheimer’s disease (36), our study, which showed 2h-PG negatively associated with memory, supports results derived from the Hisayama Study.
Insulin resistance, a state of inadequate response to insulin, is considered to be a hallmark of type 2 diabetes, and it is also observed in neurodegenerative disease (37). In addition, hyperinsulinemia is a sign of insulin resistance (38). It has been documented that both hyperinsulinemia and insulin resistance are related to poorer cognitive performance and dementia among a population without diabetes (39). Regarding diabetes patients, a cross-sectional study derived from the CAROLINA trial showed no significant association of insulin resistance with cognitive outcomes (40). Some cross-sectional studies reported significant, but weak correlation between fasting insulin concentration and Mini Mental State Examination score (40, 41) or memory (42).
Over the past decade, the prevalence of obesity in the United States has increased substantially and steadily (43). Results from different studies investigating association of obesity with dementia have been conflicting. It has been documented that mid-life obesity is related to poorer cognitive function in late-life (44, 45). Nevertheless, regarding elder adults, several reports showed impaired cognitive function in relation to increased BMI in late life (46), but more studies indicated opposite results (47, 48). In the current study, we observed stronger and more pronounced associations of cognitive functions with HOMA-IR, insulin, and HbA1c among adults with BMI < 30 kg/m2 compared with those with obesity.
We conducted risk stratification of cognitive impairment based on glucose metabolic health status. Relative to the group without any risk component, the odds of poor performance in DSST increased significantly with the number of metabolic risk components. DSST evaluates important aspects of executive function, including processing speed, sustained attention, and working memory (20). Several studies have reported consistent results that cognitive impairments were more prominent in the executive domain among patients with type 2 diabetes (49, 50); this might be in part attributed to decline in motor function due to peripheral neuropathy of diabetes patients (51).
Metformin, recommended globally as first-line treatment for type 2 diabetes, could reduce advanced glycation end products (52), which promote tissue degeneration and the microvascular complications of hyperglycemia. Epidemiological studies also support the beneficial associations of metformin with cognitive function (9, 10, 53). In support, we observed better processing speed associated with metformin use among diabetes patients who were using oral hypoglycemic agents or insulin.
Glucose metabolic disorders and cognitive impairment frequently coexist because they shared pathological mechanisms. Endothelial dysfunction related to hyperglycemia increased the accumulation of toxic lipids and advanced glycation end products, leading to reduced cerebral blood flow (54). Furthermore, insulin could protect neurons from Aβ synaptotoxicity and modulates Aβ clearance through its effects on lipid metabolism and proteases, such as the insulin-degrading enzyme (39). However, peripheral insulin resistance, and subsequent chronic hyperinsulinemia, could reduce the transport of insulin through the brain–blood barrier (55), which was in line with our findings that elevated fasting insulin and insulin resistance were associated with worse cognition.
The primary strengths of this study include a nationally representative sample, comprehensive measurements of cognitive functions in multiple domains, and glucose metabolic components. Our study does have a number of limitations. Firstly, owing to the cross-sectional design of our data, a causal relation cannot be established between glucose metabolic components and cognitive functions. Furthermore, we lacked parameters evaluating chronic inflammation, which was, however, identified as a potential risk factor for cognitive dysfunction in people with diabetes (56). Finally, because only participants assigned to the morning session were available for measurements of FPG and insulin, and an even smaller part of individuals in the morning session were suitable for the oral glucose tolerance test, our sample size was limited when doing most evaluations.
Conclusions
The nationwide cross-sectional study showed that both insulin resistance and glycemic level were reversely related to cognitive function in multiple domains, including learning, memory, and executive functions, but not in language fluency. In addition, such associations seem to be more prominent among adults without obesity. Our findings add to the existing evidence supporting the beneficial associations of metformin with cognition and also underline the crosstalk between glycemic metabolism and cognitive functions in key domains, especially among older adults without obesity.
Data Availability Statement
The datasets presented in this study can be found in online repositories. The names of the repository/repositories and accession number(s) can be found at: https://www.cdc.gov/nchs/nhanes/index.htm.
Author Contributions
GN, YL, and ML conceived and designed the study. RH, YX, RZ, JLi, and ML analyzed data. YX, YB, WW, ML, YL, and GN interpreted the data. RH and RZ drafted the manuscript. QC, TH, ZZ, JLi, and MX revised it. YC, JLu, and TW collected data. All authors agreed to be accountable for all aspects of the work and approved the final version of the paper. ML, YL, and GN are the guarantors of this work and, as such, had full access to all the data in the study and take responsibility for the integrity of the data and the accuracy of the data analysis.
Funding
This work was supported by grants from the Shanghai Shenkang Hospital Development Center (SHDC12019101).
Conflict of Interest
The authors declare that the research was conducted in the absence of any commercial or financial relationships that could be construed as a potential conflict of interest.
Publisher’s Note
All claims expressed in this article are solely those of the authors and do not necessarily represent those of their affiliated organizations, or those of the publisher, the editors and the reviewers. Any product that may be evaluated in this article, or claim that may be made by its manufacturer, is not guaranteed or endorsed by the publisher.
References
1. Zheng Y, Ley SH, Hu FB. Global Aetiology and Epidemiology of Type 2 Diabetes Mellitus and Its Complications. Nat Rev Endocrinol (2018) 14:88–98. doi: 10.1038/nrendo.2017.151
2. Alzheimer’s Disease International. Policy Brief for Heads of Government: The Global Impact of Dementia 2013–2050 (2013). Available at: https://www.alzint.org/resource/policy-brief-the-global-impact-of-dementia-2013-2050 (Accessed Assessed 10 August 2021).
3. Biessels GJ, Staekenborg S, Brunner E, Brayne C, Scheltens P. Risk of Dementia in Diabetes Mellitus: A Systematic Review. Lancet Neurol (2006) 5:64–74. doi: 10.1016/s1474-4422(05)70284-2
4. Biessels GJ, Nobili F, Teunissen CE, Simó R, Scheltens P. Understanding Multifactorial Brain Changes in Type 2 Diabetes: A Biomarker Perspective. Lancet Neurol (2020) 19:699–710. doi: 10.1016/s1474-4422(20)30139-3
5. Geijselaers SLC, Sep SJS, Stehouwer CDA, Biessels GJ. Glucose Regulation, Cognition, and Brain Mri in Type 2 Diabetes: A Systematic Review. Lancet Diabetes Endocrinol (2015) 3:75–89. doi: 10.1016/s2213-8587(14)70148-2
6. Kellar D, Craft S. Brain Insulin Resistance in Alzheimer’s Disease and Related Disorders: Mechanisms and Therapeutic Approaches. Lancet Neurol (2020) 19:758–66. doi: 10.1016/s1474-4422(20)30231-3
7. Li M, Xu Y, Wan Q, Shen F, Xu M, Zhao Z, et al. Individual and Combined Associations of Modifiable Lifestyle and Metabolic Health Status With New-Onset Diabetes and Major Cardiovascular Events: The China Cardiometabolic Disease and Cancer Cohort (4c) Study. Diabetes Care (2020) 43:1929–36. doi: 10.2337/dc20-0256
8. Anstey KJ, Cherbuin N, Budge M, Young J. Body Mass Index in Midlife and Late-Life as a Risk Factor for Dementia: A Meta-Analysis of Prospective Studies. Obes Rev (2011) 12:e426–37. doi: 10.1111/j.1467-789X.2010.00825.x
9. Samaras K, Makkar S, Crawford JD, Kochan NA, Wen W, Draper B, et al. Metformin Use Is Associated With Slowed Cognitive Decline and Reduced Incident Dementia in Older Adults With Type 2 Diabetes: The Sydney Memory and Ageing Study. Diabetes Care (2020) 43(11):2691–701. doi: 10.2337/dc20-0892
10. Wium-Andersen IK, Osler M, Jørgensen MB, Rungby J, Wium-Andersen MK. Antidiabetic Medication and Risk of Dementia in Patients With Type 2 Diabetes: A Nested Case-Control Study. Eur J Endocrinol (2019) 181(5):499–507. doi: 10.1530/eje-19-0259
11. Tsai CK, Kao TW, Lee JT, Wu CJ, Hueng DY, Liang CS, et al. Increased Risk of Cognitive Impairment in Patients With Components of Metabolic Syndrome. Med (Baltimore) (2016) 95(36):e4791. doi: 10.1097/md.0000000000004791
12. Sherzai AZ, Shaheen M, Yu JJ, Talbot K, Sherzai D. Insulin Resistance and Cognitive Test Performance in Elderly Adults: National Health and Nutrition Examination Survey (NHANES). J Neurol Sci (2018) 388:97–102. doi: 10.1016/j.jns.2017.11.031
13. Pavlik VN, Hyman DJ, Doody R. Cardiovascular Risk Factors and Cognitive Function in Adults 30-59 Years of Age (NHANES III). Neuroepidemiol (2005) 24(1-2):42–50. doi: 10.1159/000081049
14. Casagrande SS, Lee C, Stoeckel LE, Menke A, Cowie CC. Cognitive Function Among Older Adults With Diabetes and Prediabetes, NHANES 2011-2014. Diabetes Res Clin Pract (2021) 178:108939. doi: 10.1016/j.diabres.2021.108939
15. Fillenbaum GG, van Belle G, Morris JC, Mohs RC, Mirra SS, Davis PC, et al. Consortium to Establish a Registry for Alzheimer’s Disease (CERAD): The First Twenty Years. Alzheimers Dement (2008) 4:96–109. doi: 10.1016/j.jalz.2007.08.005
16. Jaeger J. Digit Symbol Substitution Test: The Case for Sensitivity Over Specificity in Neuropsychological Testing. J Clin Psychopharmacol (2018) 38:513–9. doi: 10.1097/jcp.0000000000000941
17. Henry JD, Crawford JR, Phillips LH. Verbal Fluency Performance in Dementia of the Alzheimer’s Type: A Meta-Analysis. Neuropsychologia (2004) 42:1212–22. doi: 10.1016/j.neuropsychologia.2004.02.001
18. Morris JC, Heyman A, Mohs RC, Hughes JP, van Belle G, Fillenbaum G, et al. The Consortium to Establish a Registry for Alzheimer’s Disease (CERAD). Part I. Clinical and Neuropsychological Assessment of Alzheimer’s Disease. Neurol (1989) 39:1159–65. doi: 10.1212/wnl.39.9.1159
19. Strauss SE, Spreen O. A Compendium of Neuropathology Tests: Administration, Norms and Commentary. 3rd ed. New York: Oxford University Press (2006).
21. Sotaniemi M, Pulliainen V, Hokkanen L, Soininen H, Hänninen T. CERAD-Neuropsychological Battery in Screening Mild Alzheimer’s Disease. Acta Neurol Scand (2012) 125:16–23. doi: 10.1111/j.1600-0404.2010.01459.x
22. Sager MA, Hermann BP, La Rue A, Woodard JL. Screening for Dementia in Community-Based Memory Clinics. WMJ (2006) 105:25–9.
23. Morris MS, Jacques PF, Rosenberg IH, Selhub J. Circulating Unmetabolized Folic Acid and 5-Methyltetrahydrofolate in Relation to Anemia, Macrocytosis, and Cognitive Test Performance in American Seniors. Am J Clin Nutr (2010) 91:1733–44. doi: 10.3945/ajcn.2009.28671
24. Matthews DR, Hosker JP, Rudenski AS, Naylor BA, Treacher DF, Turner RC. Homeostasis Model Assessment: Insulin Resistance and Beta-Cell Function From Fasting Plasma Glucose and Insulin Concentrations in Man. Diabetologia (1985) 28:412–9. doi: 10.1007/bf00280883
25. NHANES 2011–2012 Laboratory Methods. (2019). Available at: https://wwwn.cdc.gov/nchs/nhanes/continuousnhanes/labmethods.aspx (Accessed Assessed 10 August 2021).
26. NHANES 2013-2014 Laboratory Methods. (2020). Available at: https://wwwn.cdc.gov/nchs/nhanes/continuousnhanes/labmethods.aspx (Accessed Assessed 10 August 2021).
27. American Diabetes Association. Diagnosis and Classification of Diabetes Mellitus. Diabetes Care (2014) 37(Suppl 1):S81–90. doi: 10.2337/dc14-S081
28. Kroenke K, Spitzer RL, Williams JBW. The PHQ-9. J Gen Internal Med (2001) 16:606–13. doi: 10.1046/j.1525-1497.2001.016009606.x
29. Ward ZJ, Bleich SN, Cradock AL, Barrett JL, Giles CM, Flax C, et al. Projected U.S. State-Level Prevalence of Adult Obesity and Severe Obesity. N Engl J Med (2019) 381:2440–50. doi: 10.1056/NEJMsa1909301
30. National Center for Health Statistics (U.S.). National Health and Nutrition Examination Survey.Module 3: Weighting. Available at: https://wwwn.cdc.gov/nchs/nhanes/tutorials/module3.aspx (Accessed Assessed October 2021).
31. Neter J, Wasserman W, Kutner M. Applied Linear Regression Models. Richard D, editor. Chicago: Illinois: Irwin, Inc (2004).
32. Crane PK, Walker R, Hubbard RA, Li G, Nathan DM, Zheng H, et al. Glucose Levels and Risk of Dementia. N Engl J Med (2013) 369:540–8. doi: 10.1056/NEJMoa1215740
33. Mukai N, Ohara T, Hata J, Hirakawa Y, Yoshida D, Kishimoto H, et al. Alternative Measures of Hyperglycemia and Risk of Alzheimer’s Disease in the Community: The Hisayama Study. J Clin Endocrinol Metab (2017) 102:3002–10. doi: 10.1210/jc.2017-00439
34. Lu J, He J, Li M, Tang X, Hu R, Shi L, et al. Predictive Value of Fasting Glucose, Postload Glucose, and Hemoglobin A(1c) on Risk of Diabetes and Complications in Chinese Adults. Diabetes Care (2019) 42:1539–48. doi: 10.2337/dc18-1390
35. Ohara T, Doi Y, Ninomiya T, Hirakawa Y, Hata J, Iwaki T, et al. Glucose Tolerance Status and Risk of Dementia in the Community: The Hisayama Study. Neurol (2011) 77:1126–34. doi: 10.1212/WNL.0b013e31822f0435
36. Zhou LT, Zhang J, Tan L, Huang HZ, Zhou Y, Liu ZQ, et al. Elevated Levels of miR-144-3p Induce Cholinergic Degeneration by Impairing the Maturation of NGF in Alzheimer’s Disease. Front Cell Dev Biol (2021) 9:667412. doi: 10.3389/fcell.2021.667412
37. Kullmann S, Kleinridders A, Small DM, Fritsche A, Häring HU, Preissl H, et al. Central Nervous Pathways of Insulin Action in the Control of Metabolism and Food Intake. Lancet Diabetes Endocrinol (2020) 8:524–34. doi: 10.1016/s2213-8587(20)30113-3
38. Muscogiuri G, Barrea L, Campolo F, Sbardella E, Sciammarella C, Tarsitano MG, et al. Ketogenic Diet: A Tool for the Management of Neuroendocrine Neoplasms? Crit Rev Food Sci Nutr (2020) 60:1–11. doi: 10.1080/10408398.2020.1832955
39. Arnold SE, Arvanitakis Z, Macauley-Rambach SL, Koenig AM, Wang HY, Ahima RS, et al. Brain Insulin Resistance in Type 2 Diabetes and Alzheimer Disease: Concepts and Conundrums. Nat Rev Neurol (2018) 14:168–81. doi: 10.1038/nrneurol.2017.185
40. Janssen J, van den Berg E, Zinman B, Espeland MA, Geijselaers SLC, Mattheus M, et al. HbA(1c), Insulin Resistance, and β-Cell Function in Relation to Cognitive Function in Type 2 Diabetes: The CAROLINA Cognition Substudy. Diabetes Care (2019) 42:e1–3. doi: 10.2337/dc18-0914
41. Umegaki H, Kawamura T, Mogi N, Umemura T, Kanai A, Sano T. Glucose Control Levels, Ischaemic Brain Lesions, and Hyperinsulinaemia Were Associated With Cognitive Dysfunction in Diabetic Elderly. Age Ageing (2008) 37:458–61. doi: 10.1093/ageing/afn051
42. Abbatecola AM, Lattanzio F, Molinari AM, Cioffi M, Mansi L, Rambaldi P, et al. Rosiglitazone and Cognitive Stability in Older Individuals With Type 2 Diabetes and Mild Cognitive Impairment. Diabetes Care (2010) 33:1706–11. doi: 10.2337/dc09-2030
43. Liu B, Du Y, Wu Y, Snetselaar LG, Wallace RB, Bao W. Trends in Obesity and Adiposity Measures by Race or Ethnicity Among Adults in the United States 2011-2018: Population Based Study. BMJ (2021) 372:n365. doi: 10.1136/bmj.n365
44. Brenowitz WD, Zimmerman SC, Filshtein TJ, Yaffe K, Walter S, Hoffmann TJ, et al. Extension of Mendelian Randomization to Identify Earliest Manifestations of Alzheimer’s Disease: Genetic Risk Score for Alzheimer’s Disease Reduces BMI by Age 50. Am J Epidemiol (2021) 190(10):2163–71. doi: 10.1093/aje/kwab103
45. Morys F, Dadar M, Dagher A. Association Between Mid-Life Obesity, Its Metabolic Consequences, Cerebrovascular Disease and Cognitive Decline. J Clin Endocrinol Metab (2021) 106(10):e4260–74. doi: 10.1210/clinem/dgab135
46. Livingston G, Sommerlad A, Orgeta V, Costafreda SG, Huntley J, Ames D, et al. Dementia Prevention, Intervention, and Care. Lancet (2017) 390:2673–734. doi: 10.1016/s0140-6736(17)31363-6
47. Qizilbash N, Gregson J, Johnson ME, Pearce N, Douglas I, Wing K, et al. Bmi and Risk of Dementia in Two Million People Over Two Decades: A Retrospective Cohort Study. Lancet Diabetes Endocrinol (2015) 3:431–6. doi: 10.1016/s2213-8587(15)00033-9
48. Hayden KM, Zandi PP, Lyketsos CG, Khachaturian AS, Bastian LA, Charoonruk G, et al. Vascular Risk Factors for Incident Alzheimer Disease and Vascular Dementia: The Cache County Study. Alzheimer Dis Assoc Disord (2006) 20:93–100. doi: 10.1097/01.wad.0000213814.43047.86
49. Vincent C, Hall PA. Executive Function in Adults With Type 2 Diabetes: A Meta-Analytic Review. Psychosom Med (2015) 77:631–42. doi: 10.1097/psy.0000000000000103
50. Peña-González P, Mondragón-Maya A, Silva-Pereyra J, Roa-Rojas P. Cognitive Reserve and Executive Functions in Adults With Type 2 Diabetes. J Diabetes Res (2020) 2020:7941543. doi: 10.1155/2020/7941543
51. Palta P, Schneider AL, Biessels GJ, Touradji P, Hill-Briggs F. Magnitude of Cognitive Dysfunction in Adults With Type 2 Diabetes: A Meta-Analysis of Six Cognitive Domains and the Most Frequently Reported Neuropsychological Tests Within Domains. J Int Neuropsychol Soc (2014) 20:278–91. doi: 10.1017/s1355617713001483
52. Kickstein E, Krauss S, Thornhill P, Rutschow D, Zeller R, Sharkey J, et al. Biguanide Metformin Acts on Tau Phosphorylation via mTOR/protein Phosphatase 2A (PP2A) Signaling. Proc Natl Acad Sci USA (2010) 107(50):21830–5. doi: 10.1073/pnas.0912793107
53. Orkaby AR, Cho K, Cormack J, Gagnon DR, Driver JA. Metformin vs Sulfonylurea Use and Risk of Dementia in US Veterans Aged ≥65 Years With Diabetes. Neurol (2017) 89(18):1877–85. doi: 10.1212/wnl.0000000000004586
54. Biessels GJ, Despa F. Cognitive Decline and Dementia in Diabetes Mellitus: Mechanisms and Clinical Implications. Nat Rev Endocrinol (2018) 14(10):591–604. doi: 10.1038/s41574-018-0048-7
55. Rhea EM, Banks WA. Role of the Blood-Brain Barrier in Central Nervous System Insulin Resistance. Front Neurosci (2019) 13:521. doi: 10.3389/fnins.2019.00521
Keywords: glucose metabolism, insulin resistance, glycemic level, cognitive function, obesity, metformin
Citation: He R, Zheng R, Li J, Cao Q, Hou T, Zhao Z, Xu M, Chen Y, Lu J, Wang T, Xu Y, Bi Y, Wang W, Li M, Liu Y and Ning G (2021) Individual and Combined Associations of Glucose Metabolic Components With Cognitive Function Modified by Obesity. Front. Endocrinol. 12:769120. doi: 10.3389/fendo.2021.769120
Received: 02 September 2021; Accepted: 08 November 2021;
Published: 13 December 2021.
Edited by:
Giovanna Muscogiuri, University of Naples Federico II, ItalyReviewed by:
Dongfeng Zhang, Qingdao University, ChinaChun-Xia Yi, Amsterdam University Medical Center, Netherlands
Magda Shaheen, Charles R. Drew University of Medicine and Science, United States
Copyright © 2021 He, Zheng, Li, Cao, Hou, Zhao, Xu, Chen, Lu, Wang, Xu, Bi, Wang, Li, Liu and Ning. This is an open-access article distributed under the terms of the Creative Commons Attribution License (CC BY). The use, distribution or reproduction in other forums is permitted, provided the original author(s) and the copyright owner(s) are credited and that the original publication in this journal is cited, in accordance with accepted academic practice. No use, distribution or reproduction is permitted which does not comply with these terms.
*Correspondence: Guang Ning, Z25pbmdAc2licy5hYy5jbg==; Yan Liu, bGl1eTA1MjlAMTI2LmNvbQ==; Mian Li, bGltaWFuMzlAYWxpeXVuLmNvbQ==
†These authors have contributed equally to this work and share first authorship