- 1Department of Epidemiology and Health Statistics, Guilin Medical University, Guilin, China
- 2Fujian Key Laboratory of Women and Children’s Critical Diseases Research, Fujian Maternity and Child Health Hospital, Fuzhou, China
Objectives: The CDK5 regulatory subunit-associated protein 1-like 1 (CDKAL1) contributes to islet β-cell function and insulin secretion by inhibiting the activation of CDK5. The current studies on the relationship between CDKAL1 polymorphisms rs7756992 A>G and rs7754840 C>G and the risk of gestational diabetes mellitus (GDM) have drawn contradictory conclusions.
Materials and Methods: A meta-analysis with a fixed- or random-effects model was conducted to estimate the correlation between studied CDKAL1 polymorphisms and GDM risk with the summary odds ratio (OR) and 95% confidence interval (CI). In addition, trial sequential analysis (TSA) and false-positive report probability (FPRP) analysis were performed to confirm the study findings.
Results: A total of 13,306 subjects were included in the present study. Meta-analysis results showed that the variant heterozygous and homozygous genotypes of the two polymorphisms were associated with increased GDM risk in comparison with the wild-type AA genotype (AG vs. AA: OR = 1.23, 95% CI = 1.08, 1.41, p = 0.002; GG vs. AA: OR = 1.47, 95% CI = 1.05, 2.05, p = 0.024 for rs7756992; and CG vs. GG: OR = 1.36, 95% CI = 1.13, 1.65, p = 0.002; CC vs. GG: OR = 1.76, 95% CI = 1.37, 2.26, p < 0.001 for rs7754840). The TSA confirmed a significant association between rs7754840 and the susceptibility to GDM because the cumulative Z-curve crossed both the conventional cutoff value and the TSA boundaries under the heterozygote and homozygote models.
Conclusions: This study supported the finding that rs7756992 and rs7754840 are associated with susceptibility to GDM. However, further functional studies are warranted to clarify the mechanism.
Introduction
Gestational diabetes mellitus (GDM), defined as an abnormal glucose tolerance onset or first recognition in pregnancy (1), is approximately 1% to 22% of all pregnancies (2, 3). Clinical and epidemiological studies show that GDM can lead to short- and long-term adverse consequences for both mothers and their offspring, including increases in the incidence of gestational hypertension, spontaneous abortion, respiratory distress syndrome of newborns, or the development of complex diseases such as type 2 diabetes mellitus (T2DM) and cardiovascular disease (4–7). GDM has become a major challenge in the field of public health.
It is known that pregnancy at an older age, obesity, immune status, individual nutrition, behavior, etc., are factors associated with GDM (8–11). However, these factors do not completely explain the pathogenesis of GDM. Now, there is new evidence that the risk of GDM in individuals with family history of T2DM is significantly higher and that the risk of T2DM will increase in GDM patients in the future (10, 11). It suggests that inherited genetic factors also contribute to the genesis of GDM. The single-nucleotide polymorphism (SNP) is one of the main forms of heritable variation in the human genome DNA sequence, which determines the basis of genetic susceptibility to human disease. At present, SNPs have become the best genetic marker to interpret genetic susceptibility and predict disease risk. Replication of a recent genome-wide association study (GWAS) confirmed that T2DM-associated genetic variants (TCF7L2 rs7903146, CDKAL1 rs7756992 and rs7754840, MTNR1B rs10830962, and FTO rs8050136) are associated with the risk of GDM (12). Functional studies show that these diabetogenic genes and polymorphisms might participate in abnormal glucose metabolism, insulin resistance, and impaired β-cell function, etc., which could lead to the occurrence and development of GDM.
The CDK5 regulatory subunit-associated protein 1-like 1 (CDKAL1) is located at the human chromosome 6p22.3, encoding a 65-Ku CDKAL1 protein. CDKAL1 is mainly expressed in human pancreatic islet cells and shows considerable homology with CDK5RAP1, a well-known inhibitor of CDK5 activation. CDK5 has been suggested to downregulate insulin secretion through the formation of p35/CDK5 complexes (13, 14). In addition, CDK5 transduces glucose toxicity signals in pancreatic β-cells (13). Given that GDM has some common risk factors and genetic susceptibilities with T2DM (15, 16), a series of studies have investigated the relationship between CDKAL1 polymorphisms rs7756992 and rs7754840 and the risk of GDM. However, because of the limitations of the sample size and statistical efficiency of a single study, the conclusions are still inconsistent. In addition, to our knowledge, if the number of participants is less than required, under a realistic intervention effect, the constant application of a 5% statistical significance threshold would lead to too many false-positive and/or false-negative conclusions. Thus, a single meta-analysis usually does not reflect whether it has enough power to detect or refute the effect of an intervention. To get a clearer picture of the relationship between polymorphisms rs7756992 and rs7754840 of CDKAL1 and GDM risk, we therefore performed a trial sequential analysis (TSA) and false-positive report probability (FPRP) analysis in this meta-analysis.
Materials and Methods
Literature Search Strategy
Published studies of CDKAL1 rs7756992 and/or rs7754840 and the risk of GDM were searched in PubMed, Web of Science, the China National Knowledge Infrastructure (CNKI), and Wanfang databases, with languages limited to English and Chinese. The latest search was performed on March 31, 2021. The search terms “CDK5 regulatory subunit associated protein 1-like 1,” “CDKAL1,” “GDM,” “gestational diabetes mellitus,” “variation,” “polymorphism,” and “SNP” were used as retrieval words. Studies were also identified by a manual search of the references cited in the retrieved studies.
Inclusion and Exclusion Criteria
Inclusion criteria were as follows: 1) original study on the association between rs7756992 and/or rs7754840 polymorphisms and the risk of GDM and 2) case–control study or GWAS with independent data, 3) with genotype and/or allele distribution information, and 4) with sufficient data for calculating an OR with its corresponding 95% CI.
Exclusion criteria were as follows: 1) duplicate data, 2) no specific genotype or allele data, and 3) family-based study, review, systematic review or meta-analysis, case report, comment, and editorial.
Data Extraction and Literature Evaluation
Two professional investigators (X-YY and L-PS) independently searched articles and extracted target data from the included studies. If there were any dispute, it was settled by X-YY and L-PS. Data on the first author, year of publication, country, diagnostic criteria, source of controls, number of cases and controls, mean age, mean body mass index (BMI), genotype, and/or allele distribution were extracted.
In the present study, the Newcastle-Ottawa Scale (NOS) was used to assess the quality of target studies (17). The score range of the scale was from 0 (the lowest) to 9 (the highest), studies with a score <5 were considered to be of low quality, and those ≥5 to be of high quality. If a study is considered to be of low quality, it will not be included in the subsequent meta-analysis.
Statistical Analysis
The ORs with their corresponding 95% CIs were used to assess the strength of association between the polymorphism and GDM risk. The I2 index was calculated to quantify the degree of heterogeneity across studies, and a corresponding p less than 0.1 was considered significant (18, 19). The potential source of heterogeneity across studies was explored by stratification and meta-regression analysis. Begg’s tests (20) were used for testing the publication bias, and p < 0.05 was considered statistically significant. Sensitivity analyses were also done to assess a single study on the pooled OR. All analyses were performed by using Stata software, version 12.0 (Stata Corp LP, College Station, TX, USA).
In addition, FPRP was estimated to assess the robustness of findings of statistically significant associations. The FPRP threshold and the prior probability were set to 0.2 and 0.1, respectively, to detect the noteworthiness for OR of 1.5 (0.67 for an OR less than 1.0), with an alpha level equal to the observed p-value. An FPRP less than 0.2 was considered as a noteworthy association (21, 22).
Trial Sequential Analysis
Meta-analysis might be affected by the increased risk of random errors and repeated significance testing (23). TSA can increase the robustness of the conclusions by estimating the amount of the required information size (RIS) and the threshold for statistical significance (24). During the analysis, the significance levels for type I and type II errors were set to 5% and 20%, respectively, and relative risk reduction (RRR) was set at 20%. When the cumulative Z-curve crosses the TSA boundary or enters the insignificance area, it demonstrates a sufficient level of evidence, and no further study is necessary (25). The TSA software (version 0.9.5.10 beta) was used for data processing.
Results
Characteristics of the Included Studies
The literature search and selection are shown in Figure 1. A total of 159 studies were initially identified, and 95 articles retrieved from different databases were excluded. Subsequently, an additional 51 studies were excluded for the following reasons: 1) 22 studies were not about CDKAL1 gene and GDM; 2) six articles concerned a non-human model of GDM; 3) 15 were not case–control or GWASs; and 4) eight studies did not focus on the topic of CDKAL1 rs7756992/rs7754840 and the risk of GDM. According to the study selection strategy, 13 studies (nine in English and four in Chinese) met the research theme, of which 10 were considered to be of high quality (score ≥5) and the other three [Hu et al. (26), Wu et al. (27) and Noury et al. (28)] were of low quality (score <5), as shown in Figure 1 and Table 1. Finally, 10 studies of high quality with 5,094 GDM patients and 8,212 controls were ultimately recruited.
Association Between rs7756992 and Gestational Diabetes Mellitus Risk
In total, six high-quality studies containing 2,376 GDM cases and 4,458 controls explored the relationship between rs7756992 and the risk of GDM (29–34). The results of meta-analysis showed that compared with the wild-type homozygous AA genotype, the variant AG heterozygous and GG homozygous genotypes were significantly associated with increased risks of GDM (AG vs. AA: OR = 1.23, 95% CI = 1.08, 1.41, p = 0.002; GG vs. AA: OR = 1.47, 95% CI = 1.05, 2.05, p = 0.024).
In the stratified analysis by ethnicity and sample size (≥200 or <200) of the cases, positive results were found by comparing the variant AG heterozygous and GG homozygous genotypes with the wild-type homozygous AA genotype (AG vs. AA: OR = 1.20, 95% CI = 1.03, 1.39, p = 0.022 in Asians, OR = 1.35, 95% CI = 1.04, 1.76, p = 0.023 in Caucasians; and AG vs. AA: OR = 1.22, 95% CI = 1.06, 1.41, p = 0.005 and GG vs. AA: OR = 1.49, 95% CI = 1.01, 2.19, p = 0.044 in the sample size ≥200 group). These results are shown in Figures 2, 3 and Table 2.
Association Between rs7754840 and Gestational Diabetes Mellitus Risk
The association between rs7754840 and the risk of GDM has been explored in 10 high-quality studies, including 4,819 GDM patients and 5,873 controls (12, 29–37). The results of the meta-analysis showed that compared with the wild-type GG genotype, the variant CG heterozygous and CC homozygous genotypes were significantly associated with increased risks of GDM (CG vs. GG: OR = 1.36, 95% CI = 1.13, 1.65, p = 0.002; CC vs. GG: OR = 1.76, 95% CI = 1.37, 2.26, p < 0.001).
The stratified analysis suggested a significant association between rs7754840 and GDM risk (CG vs. GG: OR = 1.45, 95% CI = 1.15, 1.83, p = 0.002 in Asians, OR = 1.21, 95% CI = 1.07,1.37, p = 0.003 in sample sizes ≥200 and OR = 3.32, 95% CI = 1.40, 7.90, p = 0.007 in smaller sample size groups; CC vs. GG: OR = 1.82, 95% CI = 1.35, 2.44, p < 0.001 in Asians and OR = 1.74, 95% CI = 1.33, 2.28, p < 0.001 in sample sizes ≥200 group). These results are shown in Figures 4, 5 and Table 2.
Evaluation of Heterogeneity
Meta-regression analysis was performed to explore the source of heterogeneity by ethnicity and the sample size of the case group (≥200). In the homozygous genotype comparison of rs7756992, meta-regression analysis showed that heterogeneity was not significantly associated with ethnicity (t = 0.27, p = 0.797) or sample size (t = 0.12, p = 0.910). The subsequent leave-one-out analysis showed that after removing the report by Zhang et al. (31), the overall heterogeneity (pheterogeneity and I2) changed from 0.012% and 66% to 0.135% and 43%.
For the observed significant heterogeneity of rs7754840 and the susceptibility to GDM, meta-regression analyses showed that the sample size (t = −2.45, p = 0.034) might be the main heterogeneity source in the heterozygote genotype comparison. However, in the homozygous genotype comparison, we did not find ethnicity (t = 0.46, p = 0.657) or sample size (t = 0.21, p = 0.836) to be a significant cause of the heterogeneity between studies. In addition, leave-one-out analysis showed that no single factor had a significant association with the observed heterogeneity.
Publication Bias and Sensitivity Analysis
Begg’s tests were performed to identify the publication bias of the included studies, and the shape of the funnel plots did not reveal any evidence of obvious asymmetry (Figures 6 and 7). Further statistical analysis suggested no significant statistical findings for the publication bias of rs7756992 (AG vs. AA: z = −0.19, pBegg’s = 0.851; GG vs. AA: z = 0.19, pBegg’s = 0.851) and rs7754840 (CG vs. GG: z = 1.61, pBegg’s = 0.107; CC vs. GG: z = 0.31, pBegg’s = 0.754) in the present study. Sensitivity analyses suggesting no single study significantly changed the pooled ORs (Figures 8 and 9).
False-Positive Report Probability Analysis
The FPRP was adopted to assess the noteworthiness of the significant associations between the studied rs7756992 and rs7754840 polymorphisms and the risk of GDM. At the prior probability of 0.1 and a relatively stringent FPRP cutoff value of 0.2, the FPRP values calculated for the positive findings were 0.018 (overall), 0.043 (sample size ≥200), and 0.165 (Asian group) for rs7756992 A>G, and 0.021 (overall), 0.026 (sample size ≥200), and 0.028 (Asian group) for rs7754840 C>G in heterozygous genotype comparisons; in addition, FPRP values were 0.013 (overall), 0.017 (sample size ≥200), and 0.022 (Asian group) for rs7754840 C>G in homozygous genotype comparisons. These FPRP values suggested that the above positive findings were probability correct and reliable (Table 3).
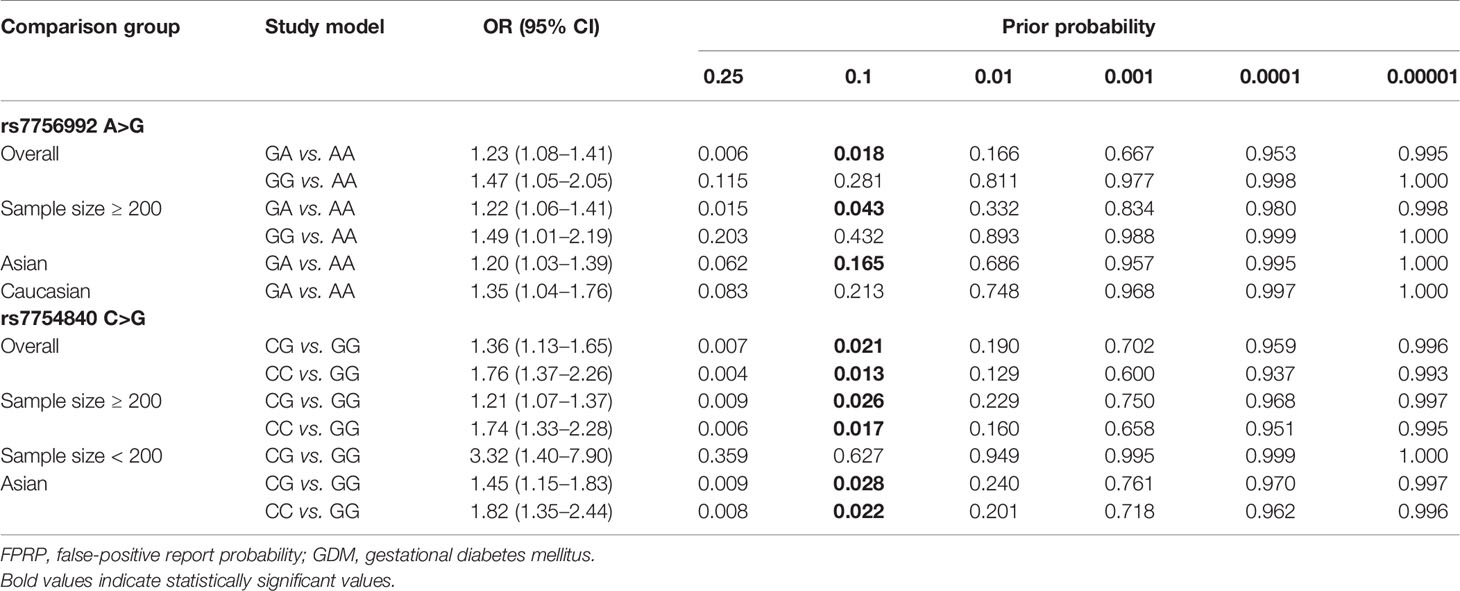
Table 3 FPRP analysis for the significant associations of the CDKAL1 genetic variations and GDM risk.
Trial Sequential Analysis
For reducing the random errors and increasing the credibility of the conclusions, TSA was performed. It showed that the cumulative Z-curve crossed both the conventional cutoff value and the TSA boundaries, suggesting that the accumulated amount information was sufficient and that no more study evidence was needed for the significant association of CDKAL1 rs7756992 and the risk of GDM under the heterozygote model (AG vs. AA). However, in the homozygote model (GG vs. AA), the cumulative Z-curve crossed only the conventional boundary but did not cross the TSA boundary, indicating that additional studies were necessary (Figure 10). Meanwhile, the TSA confirmed a significant association between rs7754840 and the susceptibility to GDM under the heterozygote model (CG vs. GG) and homozygote models (CC vs. GG) because the cumulative Z-curve crossed both the conventional cutoff value and TSA boundaries (Figure 11). Even if the cumulative amount information did not reach the RIS, no additional study evidence was needed to verify the conclusions.
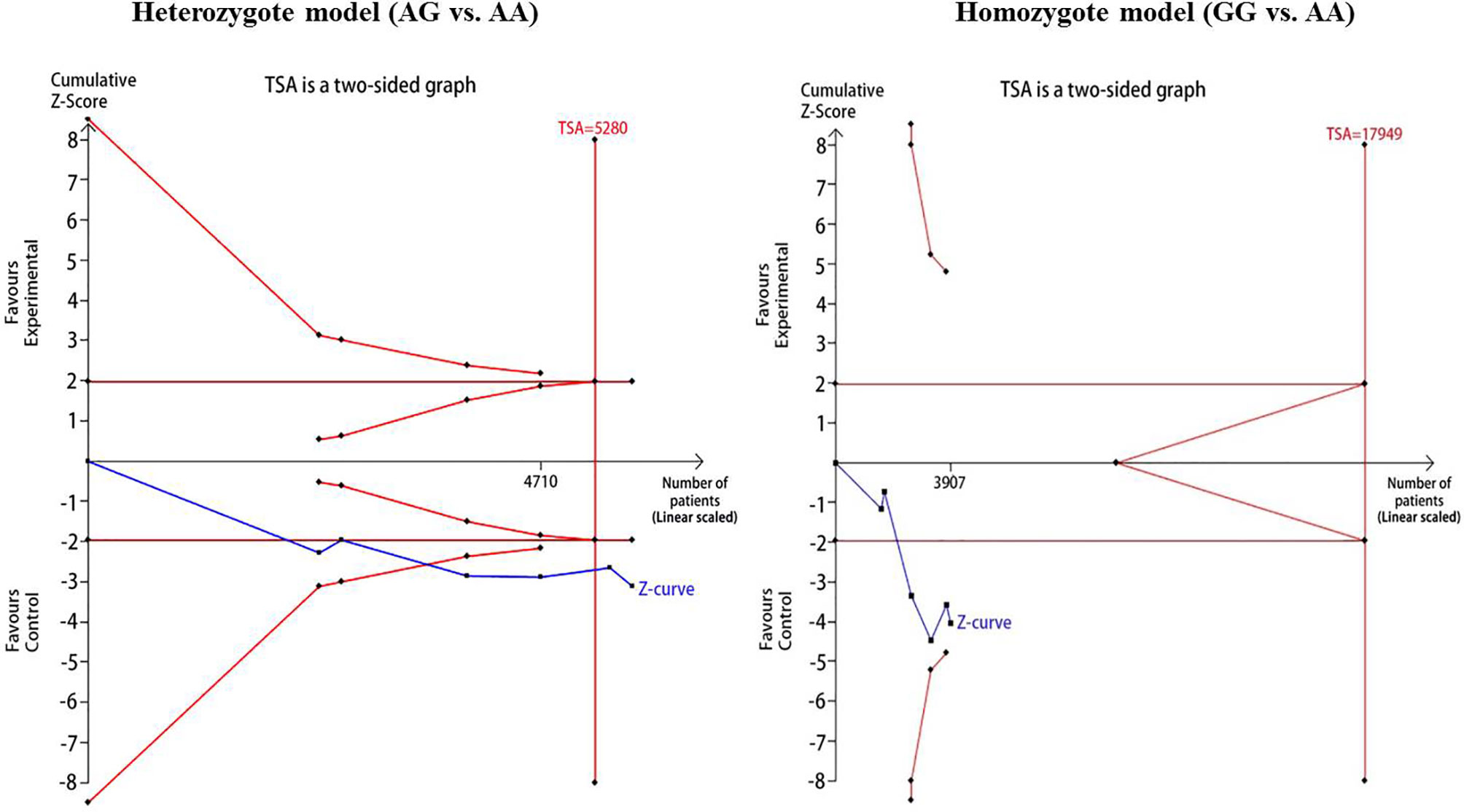
Figure 10 TSA for CDKAL1 rs7756992 A>G. We calculated α-spending adjusted required information size (RIS) by using α = 0.05 (two-sided), power = 80%. The cumulative Z-curve (Blue); Conventional boundary (Deep red); TSA boundary (red).
Discussion
GDM is a special form of diabetes that occurs during pregnancy (32). Current studies have demonstrated that gene polymorphisms might help to provide insight on the underlying backgrounds of complex diseases. Hence, many candidate gene polymorphisms, including CDKAL1 rs7756992 and rs7754840, have been tested for a relationship with genetic susceptibility to GDM. Some studies demonstrated a significant susceptibility to GDM associated with rs7756992 and rs7754840 in allele models (25, 29, 32). However, Zhang et al. (31) and Deng et al. (34) found negative associations between rs7756992 and the risk of GDM. Several studies concluded that there was no association between rs7754840 and the risk of GDM (28, 36, 37). The present meta-analysis summarizes the evidence to date on the association between CDKAL1 rs7756992 and rs7754840 and the risk of GDM.
The meta-analysis results indicated that the variant heterozygous and homozygous genotypes of the two polymorphisms were associated with increased GDM risk. Results of TSA suggested that the accumulated amount of information was sufficient and that no additional studies were required to demonstrate the significant association of CDKAL1 rs7756992 and the risk of GDM under the heterozygote model but not in the homozygote model. The TSA also confirmed the significant association between rs7754840 and the susceptibility to GDM under the heterozygote and homozygote models without further study evidence. The study by Zhang et al. (31) might be the main source of heterogeneity in the homozygous genotype comparison of rs7756992, and “sample size” might significantly influence the heterogeneity in the heterozygote genotype comparison of rs7754840. No significant statistical publication bias was detected for the analyses of candidate SNPs and GDM risk. The findings support that the CDKAL1 polymorphisms modify the susceptibility of pregnant women to GDM.
GDM is characterized by insulin resistance and impaired insulin secretion (38). The variants of CDKAL1 may influence CDKAL1 expression and leading to impaired β-cell function and insulin secretion. A study of Steinthorsdottir et al. (39) suggested that the CDKAL1 intron variant rs7756992 was associated with insulin response. A study by Kwak et al. (12) showed that CDKAL1 rs7754840 played a role in decreasing fasting insulin concentration and homeostasis model assessment for β-cell function (HOMA-β). One study has shown that variants located at the gene intron region mainly inactivate the splice-donor site and change the splicing pattern of pre-mRNA (40). The splicing error may lead to a wrong transcript product and ultimately influence gene function. However, to date, there are still no relevant studies interpreting how the studied two loci affect CDKAL1 function and change susceptibility to GDM. Therefore, further studies of the biological function of the two polymorphisms in the etiology of GDM are warranted.
Previous meta-analysis showed that polymorphisms located on CDKAL1 gene were significantly associated with genetic susceptibility to GDM (41, 42). Guo et al. (41) found that the variants rs7756992 and rs7754840 showed significant correlation with GDM risk under the allele, recessive, dominant, homozygote, and heterozygote models. The study of Mao et al. (42) indicated that CDKAL1 rs7754840 was associated with GDM risk among East Asians. However, if the number of participants was less than required, based on a realistic intervention effect, the constant application of a traditional 95% CI or 5% statistical significance threshold will lead to a false-positive or false-negative conclusion. Thus, in the present trial sequential meta-analysis, we confirmed the association between CDKAL1 polymorphisms rs7756992 or rs7754840 with the risk of GDM under the allele models, and no more samples are needed to further evaluate these findings.
The FPRP analysis is an effective approach to verify the noteworthiness of significant association findings. For the present study, a relatively stringent FPRP threshold of 0.2 was set, and much lower FPRP values of the observed significant associations between rs7756992 G>A and rs7754840 C>G variations, and the risk of GDM suggested that the positive findings were probability accurate and reliable. Hence, we believe that the association of the two variations and GDM risk is credible.
Some limitations in the present meta-analysis should be pointed out. First, the literatures included in this meta-analysis are retrospective studies. Compared with prospective studies, the research data are vulnerable to selection bias and recall bias. Second, only published data were included, and some of the sample sizes were relatively small. These may affect the overall effect assessment between studied polymorphisms and GDM. To ensure the reliability of the results, a TSA was performed, and FPRP values of note were assessed. Third, this meta-analysis simply evaluated the association between rs7756992 or rs7754840 genotypes and GDM risk without considering the effects of other genetic markers or environmental factors (43, 44). If individual-level data were available, a much more precise analysis to identify the interactions between gene–gene and gene–environment could also have been done.
In summary, the present study supports the significant association of the CDKAL1 polymorphisms rs7756992 and rs7754840 with an increased risk of GDM. Further functional studies are warranted to clarify the potential mechanism in the pathogenesis of GDM.
Data Availability Statement
The original contributions presented in the study are included in the article/supplementary material. Further inquiries can be directed to the corresponding authors.
Author Contributions
X-YY and D-BL: protocol/project development and manuscript editing. D-BL and L-PS: data collection and analysis and manuscript writing. S-DW: data analysis and manuscript writing. X-LW: data collection and management. All authors contributed to the article and approved the submitted version.
Funding
This study was supported by Basic ability improvement project of young and Middle-aged College Students in Guangxi (2020KY2028), the National Natural Science Foundation of China (81760614), the Key R&D Program (2018AB62004), and College Students’ Innovation Project (201710601103, 201810601031).
Conflict of Interest
The authors declare that the research was conducted in the absence of any commercial or financial relationships that could be construed as a potential conflict of interest.
Publisher’s Note
All claims expressed in this article are solely those of the authors and do not necessarily represent those of their affiliated organizations, or those of the publisher, the editors and the reviewers. Any product that may be evaluated in this article, or claim that may be made by its manufacturer, is not guaranteed or endorsed by the publisher.
References
1. Metzger BE, Coustan DR. Summary and Recommendations of the Fourth International Workshop-Conference on Gestational Diabetes Mellitus. The Organizing Committee. Diabetes Care (1998) Suppl 2:B161–167.
2. Galtier F. Definition, Epidemiology, Risk Factors. Diabetes Metab (2010) 6 Pt 2:628–51. doi: 10.1016/j.diabet.2010.11.014
3. Shaat N, Groop L. Genetics of Gestational Diabetes Mellitus. Curr medicinal Chem (2007) 5:569–83. doi: 10.2174/092986707780059643
4. Beucher G, Viaris de Lesegno B, Dreyfus M. Maternal Outcome of Gestational Diabetes Mellitus. Diabetes Metab (2010) 6 Pt 2:522–37. doi: 10.1016/j.diabet.2010.11.006
5. Bellamy L, Casas JP, Hingorani AD, Williams D. Type 2 Diabetes Mellitus After Gestational Diabetes: A Systematic Review and Meta-Analysis. Lancet (2009) 9677:1773–9. doi: 10.1016/S0140-6736(09)60731-5
6. Johns K, Olynik C, Mase R, Kreisman S, Tildesley H. Gestational Diabetes Mellitus Outcome in 394 Patients. J Obstetrics Gynaecol Canada (2006) 2:122–7. doi: 10.1016/S1701-2163(16)32068-0
7. Reece EA, Leguizamon G, Wiznitzer A. Gestational Diabetes: The Need for a Common Ground. Lancet (2009) 9677:1789–97. doi: 10.1016/S0140-6736(09)60515-8
8. Leng J, Shao P, Zhang C, Tian H, Zhang F, Zhang S, et al. Prevalence of Gestational Diabetes Mellitus and Its Risk Factors in Chinese Pregnant Women: A Prospective Population-Based Study in Tianjin, China. PloS One (2015) 3:e0121029. doi: 10.1371/journal.pone.0121029
9. Chu SY, Callaghan WM, Kim SY, Schmid CH, Lau J, England LJ, et al. Maternal Obesity and Risk of Gestational Diabetes Mellitus. Diabetes Care (2007) 8:2070–6. doi: 10.2337/dc06-2559a
10. Rhee SY, Kim JY, Woo JT, Kim YS, Kim SH. Familial Clustering of Type 2 Diabetes in Korean Women With Gestational Diabetes Mellitus. Korean J Internal Med (2010) 3:269–72. doi: 10.3904/kjim.2010.25.3.269
11. Pu J, Zhao B, Wang EJ, Nimbal V, Osmundson S, Kunz L, et al. Racial/Ethnic Differences in Gestational Diabetes Prevalence and Contribution of Common Risk Factors. Paediatric Perinatal Epidemiol (2015) 5:436–43. doi: 10.1111/ppe.12209
12. Kwak SH, Kim SH, Cho YM, Go MJ, Cho YS, Choi SH, et al. A Genome-Wide Association Study of Gestational Diabetes Mellitus in Korean Women. Diabetes (2012) 2:531–41. doi: 10.2337/db11-1034
13. Ubeda M, Rukstalis JM, Habener JF. Inhibition of Cyclin-Dependent Kinase 5 Activity Protects Pancreatic Beta Cells From Glucotoxicity. J Biol Chem (2006) 39:28858–64. doi: 10.1074/jbc.M604690200
14. Wei FY, Nagashima K, Ohshima T, Saheki Y, Lu YF, Matsushita M, et al. Cdk5-Dependent Regulation of Glucose-Stimulated Insulin Secretion. Nat Med (2005) 10:1104–8. doi: 10.1038/nm1299
15. Angueira AR, Ludvik AE, Reddy TE, Wicksteed B, Lowe WL Jr, Layden BT. New Insights Into Gestational Glucose Metabolism: Lessons Learned From 21st Century Approaches. Diabetes (2015) 2:327–34. doi: 10.2337/db14-0877
16. Kawai VK, Levinson RT, Adefurin A, Kurnik D, Collier SP, Conway D, et al. A Genetic Risk Score That Includes Common Type 2 Diabetes Risk Variants Is Associated With Gestational Diabetes. Clin Endocrinol (2017) 2:149–55. doi: 10.1111/cen.13356
17. Stang A. Critical Evaluation of the Newcastle-Ottawa Scale for the Assessment of the Quality of Nonrandomized Studies in Meta-Analyses. Eur J Epidemiol (2010) 9:603–5. doi: 10.1007/s10654-010-9491-z
18. Jackson D, White IR, Riley RD. Quantifying the Impact of Between-Study Heterogeneity in Multivariate Meta-Analyses. Stat Med (2012) 29:3805–20. doi: 10.1002/sim.5453
19. Higgins JP, Thompson SG, Deeks JJ, Altman DG. Measuring Inconsistency in Meta-Analyses. BMJ (2003) 7414:557–60. doi: 10.1136/bmj.327.7414.557
20. Begg CB, Mazumdar M. Operating Characteristics of a Rank Correlation Test for Publication Bias. Biometrics (1994) 4:1088–101. doi: 10.2307/2533446
21. Wacholder S, Chanock S, Garcia-Closas M, El Ghormli L, Rothman N. Assessing the Probability That a Positive Report Is False: An Approach for Molecular Epidemiology Studies. J Natl Cancer Institute (2004) 6:434–42. doi: 10.1093/jnci/djh075
22. He J, Wang MY, Qiu LX, Zhu ML, Shi TY, Zhou XY, et al. Genetic Variations of Mtorc1 Genes and Risk of Gastric Cancer in an Eastern Chinese Population. Mol Carcinogenesis (2013) Suppl 1:E70–9. doi: 10.1002/mc.22013
23. Brok J, Thorlund K, Gluud C, Wetterslev J. Trial Sequential Analysis Reveals Insufficient Information Size and Potentially False Positive Results in Many Meta-Analyses. J Clin Epidemiol (2008) 8:763–9. doi: 10.1016/j.jclinepi.2007.10.007
24. Wetterslev J, Jakobsen JC, Gluud C. Trial Sequential Analysis in Systematic Reviews With Meta-Analysis. BMC Med Res Method (2017) 1:39. doi: 10.1186/s12874-017-0315-7
25. Thorlund K, Imberger G, Wetterslev J, Brok J, Gluud C. Comments on ’Sequential Meta-Analysis: An Efficient Decision-Making Tool’ by I Van Der Tweel and C Bollen. Clin Trials (2010) 6:752–3; author reply 754. doi: 10.1177/1740774510386255
26. Hu XH, Zheng J, Zhang K. A Research on Relationship Between Single Nucleotide Polymorphism of Susceptibility Genes of Type 2 Diabetes Mellitus and Gestational Diabetes in Jiangsu. Int J Lab Med (2014) 10:1245–7. doi: 10.3969/j.issn.1673-4130.2014.10.006
27. Wu YL, Li SP, Zhang Z. Association Between Gene Polymorphism of CDKAL1 and Gestational Diabetes Mellitus. Chin J Diabets (2015) 6:501–4. doi: 10.3969/j.issn.1006-6187.2015.06.006
28. Noury AE, Azmy O, Alsharnoubi J, Salama S, Okasha A, Gouda W. Variants of CDKAL1 Rs7754840 (G/C) and CDKN2A/2B Rs10811661 (C/T) With Gestational Diabetes: Insignificant Association. BMC Res Notes (2018) 1:181. doi: 10.1186/s13104-018-3288-7
29. Cho YM, Kim TH, Lim S, Choi SH, Shin HD, Lee HK, et al. Type 2 Diabetes-Associated Genetic Variants Discovered in the Recent Genome-Wide Association Studies Are Related to Gestational Diabetes Mellitus in the Korean Population. Diabetologia (2009) 2:253–61. doi: 10.1007/s00125-008-1196-4
30. Lauenborg J, Grarup N, Damm P, Borch-Johnsen K, Jorgensen T, Pedersen O, et al. Common Type 2 Diabetes Risk Gene Variants Associate With Gestational Diabetes. J Clin Endocrinol Metab (2009) 1:145–50. doi: 10.1210/jc.2008-1336
31. Zhang LH. Correlation Between SLC30A8, CDKAL1 Gene and Gestational Diabetes Mellitus. Tsinghua University: Beijing Concord Medical College, Chinese Academy of Medical Sciences (2009).
32. Aris NKM, Ismai NAM, Mahdy ZA. An Analysis of Targeted Single Nucleotide Polymorphisms for the Risk Prediction of Gestational Diabetes Mellitus in a Cohort of Malaysian Patients. Asia Pac J Mol Medi (2011) 1:1–8.
33. Wang Y, Nie M, Li W. Association of Six Single Nucleotide Polymorphisms With Gestational Diabetes Mellitus in a Chinese Population. PloS One (2011) 11:e26953. doi: 10.1371/journal.pone.0026953
34. Deng ZF, Ye JL, Shu QQ. Genetic Correlation of Gestational Diabetes Mellitus in Chinese Han Women. Chin Rural Health Serv Admin (2013) 7:812–4.
35. Kanthimathi S, Chidambaram M, Liju S, Bhavadharini B, Bodhini D, Prakash VG, et al. Identification of Genetic Variants of Gestational Diabetes in South Indians. Diabetes Technol Ther (2015) 7:462–7. doi: 10.1089/dia.2014.0349
36. Popova PV, Klyushina AA, Vasilyeva LB, Tkachuk AS, Bolotko YA, Gerasimov AS, et al. Effect of Gene-Lifestyle Interaction on Gestational Diabetes Risk. Oncotarget (2017) 67:112024–35. doi: 10.18632/oncotarget.22999
37. Rosta K, Al-Aissa Z, Hadarits O, Harreiter J, Nadasdi A, Kelemen F, et al. Association Study With 77 SNPs Confirms the Robust Role for the Rs10830963/G of MTNR1B Variant and Identifies Two Novel Associations in Gestational Diabetes Mellitus Development. PloS One (2017) 1:e0169781. doi: 10.1371/journal.pone.0169781
38. Metzger BE, Buchanan TA, Coustan DR, de Leiva A, Dunger DB, Hadden DR, et al. Summary and Recommendations of the Fifth International Workshop-Conference on Gestational Diabetes Mellitus. Diabetes Care (2007) Suppl 2:S251–60. doi: 10.2337/dc07-s225
39. Steinthorsdottir V, Thorleifsson G, Reynisdottir I, Benediktsson R, Jonsdottir T, Walters GB, et al. A Variant in CDKAL1 Influences Insulin Response and Risk of Type 2 Diabetes. Nat Genet (2007) 6:770–5. doi: 10.1038/ng2043
40. Thi Tran HT, Takeshima Y, Surono A, Yagi M, Wada H, Matsuo M. A G-To-A Transition at the Fifth Position of Intron-32 of the Dystrophin Gene Inactivates a Splice-Donor Site Both In Vivo and In Vitro. Mol Genet Metab (2005) 3:213–9. doi: 10.1016/j.ymgme.2005.03.006
41. Guo F, Long W, Zhou W, Zhang B, Liu J, Yu B. FTO, GCKR, CDKAL1 and CDKN2A/B Gene Polymorphisms and the Risk of Gestational Diabetes Mellitus: A Meta-Analysis. Arch Gynecology Obstetrics (2018) 4:705–15. doi: 10.1007/s00404-018-4857-7
42. Mao H, Li Q, Gao S. Meta-Analysis of the Relationship Between Common Type 2 Diabetes Risk Gene Variants With Gestational Diabetes Mellitus. PloS One (2012) 9:e45882. doi: 10.1371/journal.pone.0045882
43. Feng Y, Jiang CD, Chang AM, Shi Y, Gao J, Zhu L, et al. Interactions Among Insulin Resistance, Inflammation Factors, Obesity-Related Gene Polymorphisms, Environmental Risk Factors, and Diet in the Development of Gestational Diabetes Mellitus. J Matern Fetal Neonatal Med (2019) 2:339–47. doi: 10.1080/14767058.2018.1446207
44. Franzago M, Fraticelli F, Nicolucci A, Celentano C, Liberati M, Stuppia L, et al. Molecular Analysis of a Genetic Variants Panel Related to Nutrients and Metabolism: Association with Susceptibility to Gestational Diabetes and Cardiometabolic Risk in Affected Women. J Diabetes Res (2017) 2017:4612623. doi: 10.1155/2017/4612623
Keywords: gestational diabetes mellitus (GDM), polymorphism, meta-analysis, trial sequential analysis (TSA), false-positive report probability
Citation: Yu X-y, Song L-p, Wei S-d, Wen X-l and Liu D-b (2021) CDK5 Regulatory Subunit-Associated Protein 1-Like 1 Gene Polymorphisms and Gestational Diabetes Mellitus Risk: A Trial Sequential Meta-Analysis of 13,306 Subjects. Front. Endocrinol. 12:722674. doi: 10.3389/fendo.2021.722674
Received: 06 July 2021; Accepted: 20 September 2021;
Published: 14 October 2021.
Edited by:
Antonio Brunetti, University of Catanzaro, ItalyReviewed by:
Sinan Tanyolac, Istanbul University, TurkeyChristopher Gerner, University of Vienna, Austria
Copyright © 2021 Yu, Song, Wei, Wen and Liu. This is an open-access article distributed under the terms of the Creative Commons Attribution License (CC BY). The use, distribution or reproduction in other forums is permitted, provided the original author(s) and the copyright owner(s) are credited and that the original publication in this journal is cited, in accordance with accepted academic practice. No use, distribution or reproduction is permitted which does not comply with these terms.
*Correspondence: Xiang-yuan Yu, Guilinxiangyuan123@163.com; Da-bin Liu, 13489997701@139.com
†These authors have contributed equally to this work