- 1Department of Spinal Surgery, The Affiliated Hospital of Qingdao University, Qingdao, China
- 2The First Clinical College, Wenzhou Medical University, Wenzhou, China
- 3Department of Surgery, The People’s Hospital of Yunhe, Lishui, China
Background: Osteosarcoma is the most common bone cancer, mainly occurring in children and adolescents, among which distant metastasis (DM) still leads to a poor prognosis. Although nomogram has recently been used in tumor areas, there are no studies focused on diagnostic and prognostic evaluation of DM in primary osteosarcoma patients.
Methods: The data of osteosarcoma patients diagnosed between 2004 and 2015 were extracted from the Surveillance, Epidemiology, and End Results (SEER) database. Univariate and multivariate logistic regression analyses were used to identify independent risk factors for DM in osteosarcoma patients, and univariate and multivariate Cox proportional hazards regression analyses were used to determine independent prognostic factors of osteosarcoma patients with DM. We then established two novel nomograms and the results were evaluated by receiver operating characteristic (ROC) curves, calibration curves, and decision curve analysis (DCA).
Result: A total of 1,657 patients with osteosarcoma were included, and 267 patients (16.11%) had DM at the time of diagnosis. The independent risk factors for DM in patients with osteosarcoma include age, grade, T stage, and N stage. The independent prognostic factors for osteosarcoma patients with DM are age, chemotherapy and surgery. The results of ROC curves, calibration, DCA, and Kaplan–Meier (K-M) survival curves in the training, validation, and expanded testing sets, confirmed that two nomograms can precisely predict occurrence and prognosis of DM in osteosarcoma patients.
Conclusion: Two nomograms are expected to be effective tools for predicting the risk of DM for osteosarcoma patients and personalized prognosis prediction for patients with DM, which may benefit clinical decision-making.
Introduction
Osteosarcoma is the most prevalent form of bone cancer and mainly occurs in children and adolescents (1), which is predominantly derived from the terminus of the long bones, including distal femur (43%), proximal tibias (23%), and proximal humor (10%) (2). Recent reports suggested that the incidence and mortality rates of osteosarcoma have been annually growing by 0.3 and 1.4%, respectively (3, 4). Currently, systemic chemotherapy combined with extensive surgical resection is recognized as the most effective treatment method for osteosarcoma (5–7), and the 5-year survival rate of non-metastatic osteosarcoma patients has been improved to 60–70% with multimodal therapy (8). However, osteosarcoma with distant metastasis (DM) still results in poor prognosis, and only 11–30% of patients can survive with a multimodal combination of surgical resection and chemotherapy (9, 10).
Approximately 20–30% of osteosarcoma patients presented clinical DM (most commonly in the lung) at the time of the first diagnosis (10, 11), and about 25–35% of patients with initially non-metastatic osteosarcoma subsequently develop metastatic diseases (12, 13). Of note, osteosarcoma patients with DM promptly develop more lesions and become resistant to chemotherapy (14), with dismal 5-year overall survival (OS) time less than 20% (15). Therefore, it is imperative to construct exact models to assess the risk of DM of osteosarcoma patients and evaluate the prognosis of patients with DM. Previous studies have revealed that age, M stage, grade, primary tumor site, tumor size, surgery, radiotherapy, and extent of disease were the independent prognostic factors for osteosarcoma (16–18). However, to the best of our knowledge, there are a limited number of studies focusing reliable data on the relationship between clinicopathological features and metastatic pattern of osteosarcoma, and no predictive model for predicting the DM in osteosarcoma or the prognosis of osteosarcoma with DM were established.
Nomogram has been diffusely generated to evaluate the prognosis of cancer patients recently owing to its convenience and precision, which is a good choice for our purpose (19, 20). Thus, we identified a representative cohort from the Surveillance, Epidemiology, and End Results (SEER) database to evaluate incidence, risk factors, and prognosis of de-novo metastatic osteosarcoma, and develop two nomograms for predicting the DM in osteosarcoma patients and OS of osteosarcoma patients with DM, respectively.
Patients and Methods
Patients
The current study data of osteosarcoma patients were extracted from the SEER database from 2004 to 2015. Inclusion criteria were as follows: (1) Patients were diagnosed with osteosarcoma that was occurring in the bone and joints; (2) Demographic variables, including age, sex, and race were available; (3) Clinical pathological information, including primary tumor site, grade, histological type, TNM, and tumor size were available. Besides, patients diagnosed with autopsy or death certificate were excluded from the study. Finally, 1,657 patients diagnosed with osteosarcoma were included in the present study, including 267 patients who had DM. All patients were used to form a diagnostic cohort to explore the risk factors of DM and develop a predictive nomogram. Moreover, out of 267 osteosarcoma patients with DM, 260 patients with survival time of ≥1 month, and available specific treatment information, including surgery, chemotherapy and radiotherapy, were used to form a prognostic cohort to study the prognostic factors for patients with DM and develop a novel prognostic nomogram.
In the diagnostic cohort, patients were randomly divided into the training (70%), and validation sets (30%) with a ratio of 7:3. As for the prognostic cohort, patients in the training and validation sets were composed of the patients who had DM from corresponding sets in the diagnostic cohort. For each cohort, patients in the training set were used to construct the nomogram, and corresponding patients in the validation set were used to validate it.
Data Collection
In this study, variables selected to identify the risk factors of DM in osteosarcoma patients are as follows: age, sex, race, primary site, grade, histology type, T stage, N stage, and tumor size. Besides, our research also conducted survival analyses to investigate prognostic factors of osteosarcoma patients with DM. On the basis of the above factors, three treatment variables were included, namely, surgery, radiotherapy, and chemotherapy. In this part, OS was the primary outcome, which was defined as the time interval between the day of diagnosis and the day of death for any reason.
Statistical Analysis
In the present study, all statistical analysis was performed with SPSS 24.0 and R software (version 3.6.1), and a P value <0.05 (two side) was considered as statistical significance. All osteosarcoma patients were randomly divided into the training and validation sets in R software, and the Chi-square test or Fisher’s exact test was used to compare the distribution of variables between the two sets.
In the diagnostic cohort, the univariate logistic analysis was performed to identify DM-related risk factors. The variables with P <0.05 in the univariate analysis were incorporated into the multivariate logistic analysis with “Forward LR” in SPSS 24.0, to determine independent risk factors of DM in osteosarcoma patients (21). In addition, a novel diagnostic nomogram was built using the “rms” package based on independent risk factors. The receiver operating characteristic (ROC) curve of nomogram and all independent variables were generated, and the corresponding area under the curve (AUC) was calculated to assess the discrimination. Moreover, the calibration curves and decision curve analysis (DCA) were used to evaluate the performance of the nomogram.
For prognostic factors, the univariate Cox regression analysis was applied to determine OS-related factors for osteosarcoma patients with DM. Then, significant variables with P <0.05 were incorporated into the multivariate Cox analysis with “Forward LR” in SPSS 24.0 to further determine the independent prognostic factors. A prognostic nomogram based on the independent prognostic predictors was developed to predict the OS of osteosarcoma patients with DM, and the individual risk score was calculated using the formula of nomogram. In addition, time-dependent ROC curves of nomogram and all independent prognostic variables at 12, 24, and 36 months were generated, and the corresponding time-dependent AUCs were applied to show the discrimination. Calibration curves and DCA of 12, 24, and 36 months were plotted to evaluate the nomogram. According to the median risk score, all osteosarcoma patients with DM were divided into high- and low-risk groups. Kaplan–Meier (K–M) survival curves with the log-rank test were performed to show the difference OS status between the two groups.
Results
Baseline Characteristics of the Study Population
A total of 1,657 patients with osteosarcoma were enrolled, and 996 and 661 patients were stratified into the training and validation sets. The mean age of the training and validation sets were 26.69 years old (ranging from 3 to 94) and 26.86 years old (ranging from 3 to 89), respectively. As shown in Table 1, the most common primary site location was limb (82.13% in the training set and 79.58% in the validation set), and the most common tumor grades of differentiation were grades III–IV (87.15% in the training set and 86.99% in the validation set). The most common T and N stages were T2 (56.33% in the training set and 53.10% in the validation set) and N0 (97.59% in the training set, and 98.18% in the validation set). Regarding the histological type of osteosarcoma patients, osteosarcoma, NOS accounted for 62.55% in the training set and 63.39% in the validation set. Meanwhile, the Chi-square test proved that the deviation was completely randomized (Table 1).
Incidence and Risk Factors of Distant Metastasis in Osteosarcoma Patients
A total of 267 cases (16.11%) confirmed as DM at initial diagnosis and 1,390 cases (83.89%) without it. As shown in Table 2, nine potential factors were analyzed by the univariate logistic analysis, and the result revealed six DM-related variables, including age, primary site, grade, T stage, N stage, and tumor size. Additionally, the multivariate logistic regression analysis determined that patients younger than 18 or older than 50, higher T stage, higher N stage, and higher grade were independent risk predictors of DM in primary osteosarcoma patients (Table 2).
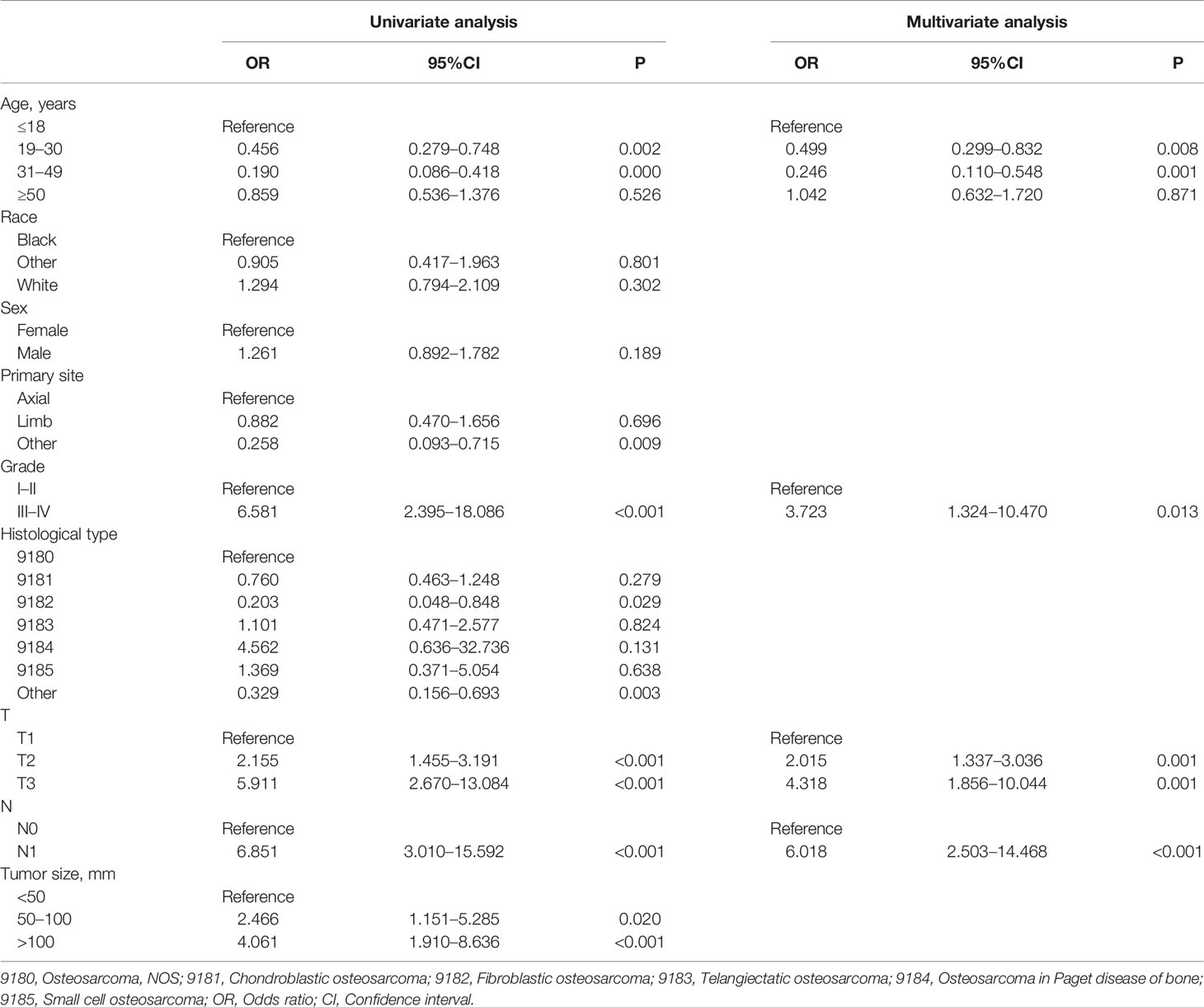
Table 2 Univariate and multivariate logistic analyses of distant metastasis in osteosarcoma patients.
Diagnostic Nomogram Development and Validation
A novel nomogram for predicting the risk of DM in osteosarcoma patients was established based on the four independent predictors (Figure 1A). Then, we established the ROC curves of the training and validation sets, and the AUCs of the nomogram were 0.693 and 0.700 in the training and validation set, respectively (Figures 1B, E). Meanwhile, the ROC curves of all independent predictors were also generated (Figures 2A, B), demonstrating a better discriminative ability than the other individual factors, both in the training and validation sets. More importantly, the calibration curves of the nomogram illustrated excellent consistency between the observed and predicted results (Figures 1C, F). As shown in Figures 1D, G, DCA curves indicated that the diagnostic nomogram can serve as a precise tool for DM assessment. In addition, to further verify the applicability of the model in the absence of external data, we went back to the SEER database and re-screened suitable patients with complete age, T stage, N stage, grade stage, and M stage. Totally, 1,667 patients were obtained to form an expanded testing set. Meanwhile, the ROC curve showed that the AUC of the nomogram was 0.696 in the expanded testing set (Supplementary Figure 1A), and calibration, DCA, and ROC curves of all independent factors (Supplementary Figures 1B–D) also proved the good performance of the diagnostic nomogram.
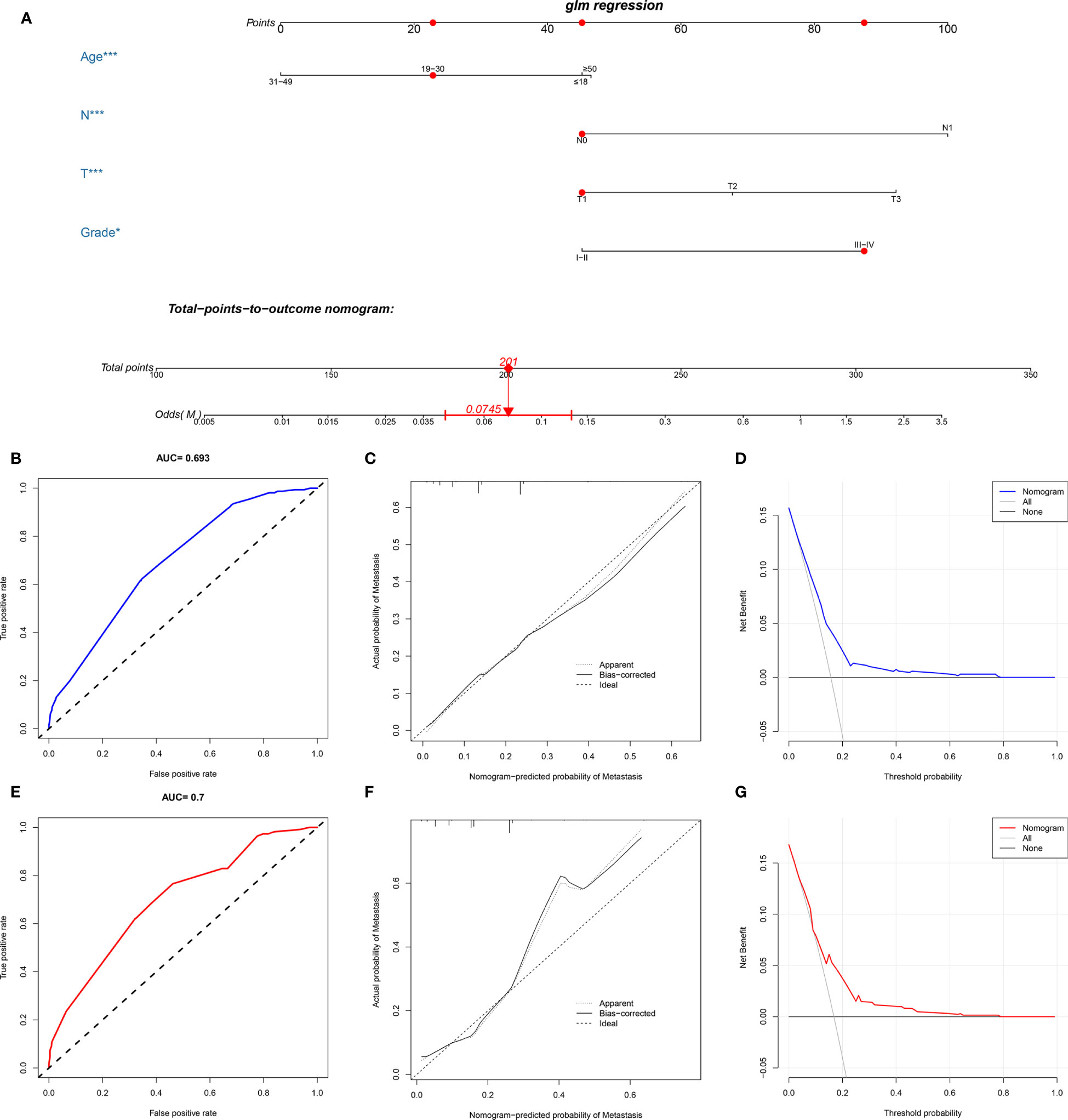
Figure 1 Construction and validation of a diagnostic nomogram. A nomogram to estimate the risk of DM in osteosarcoma patients (A). The receiver operating characteristic curve (B), calibration curve (C), and decision curve analysis (D) of the training set, and the receiver operating characteristic curve (E), calibration curve (F), and decision curve analysis (G) of the validation set.
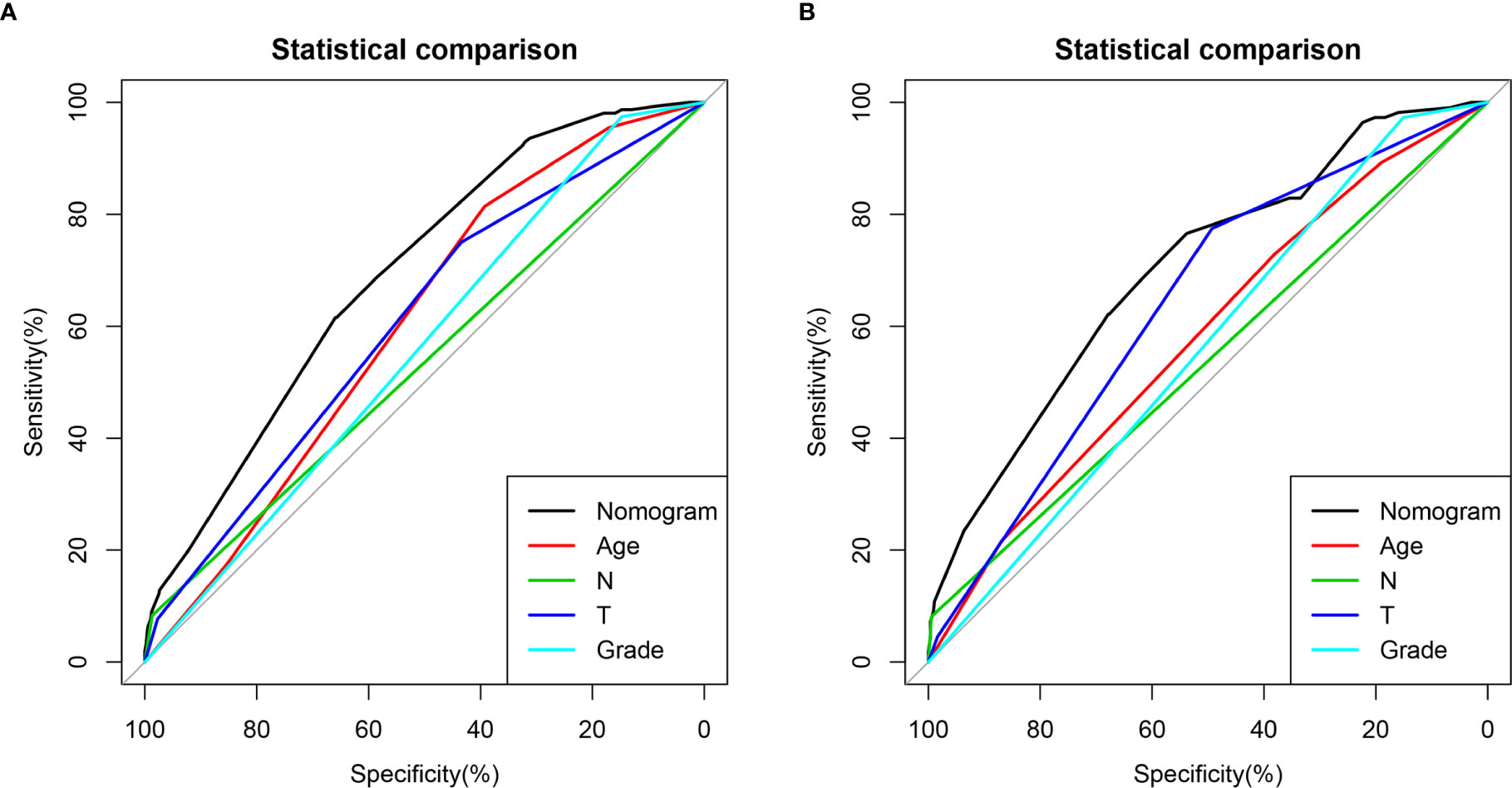
Figure 2 Comparison of area under the receiver operating characteristic curves between nomogram and all independent factors, including Grade, T stage, N stage, and Age in the training set (A) and Validation set (B).
Prognostic Factors for Osteosarcoma Patients With DM
In the present study, 260 eligible osteosarcoma patients with DM were used to explore prognostic factors. As shown in Table 3, 207 (79.61%) patients underwent surgery, 48 (18.46%) underwent radiotherapy, and 240 (92.31%) underwent chemotherapy. The Chi-square test and Fisher’s exact test indicated that the differences of all variables were not significant between the training set and the validation set. Then, the univariate and multivariate Cox regression analyses were used to screen robust prognostic factors, which revealed that the higher age (P <0.001), absence of surgery (P <0.001) and absence of chemotherapy (P = 0.001) were independent prognostic factors for osteosarcoma patients with DM (Table 4).
Prognostic Nomogram Development and Validation
Based on the three prognostic factors, a nomogram was established to predict the OS of osteosarcoma patients with DM (Figure 3). The calibration curves of the nomogram for the probability of 12, 24, and 36 months OS exhibited a strong agreement between nomogram-predicted OS and the actual outcome in the training set (Figures 4A–C) and validation set (Figures 5A–C). Additionally, the DCA curves also determined that the nomogram had good performance in clinical practice (Figures 4D–F, 5D–F). Moreover, ROC analysis showed that the AUCs of the nomogram in the training set for the 12, 24, and 36 months reached 0.835, 0.747, and 0.758 (Figure 6A), and 0.792, 0.831, and 0.786, respectively, in the validation set (Figure 6B), which also showed good discrimination in predicting the OS of osteosarcoma patients with DM. The K–M curves indicated that the patients in the high-risk group had significantly worse OS than the patients in the low-risk group (Figures 6C, D). Furthermore, we further compared the discrimination between nomogram and each independent prognostic factor, and the results indicated that the discrimination of nomogram was better than all independent prognostic factors at 12, 24, and 36 months (Figures 7A–F). Meanwhile, although histology type was not an independent prognostic factor for osteosarcoma patients with DM, considering histologically different osteosarcomas arising from different cells may affect the predictive ability of the nomogram, the stratification analysis was implemented to evaluated this. Due to the limitation of the study sample, we only divided the patients into two subgroups (9180: osteosarcoma, Nos Vs others). As shown in Supplementary Figure 2, the AUCs of patients with 9,180 for predicting the 12, 24, and 36 months OS were 0.849, 0.755, and 0.756 in the training set and 0.786, 0.855, and 0.810 in the validation set (Supplementary Figures 2A, C), and ROC analysis showed the AUCs of patients with other histology type reached 0.821, 0.732, and 0.761 in the training set and 0.817, 0.706, and 0.640 in the validation set (Supplementary Figures 2B, D), which implied the prognostic nomogram could serve a rigorous tool for patients with different histology type.
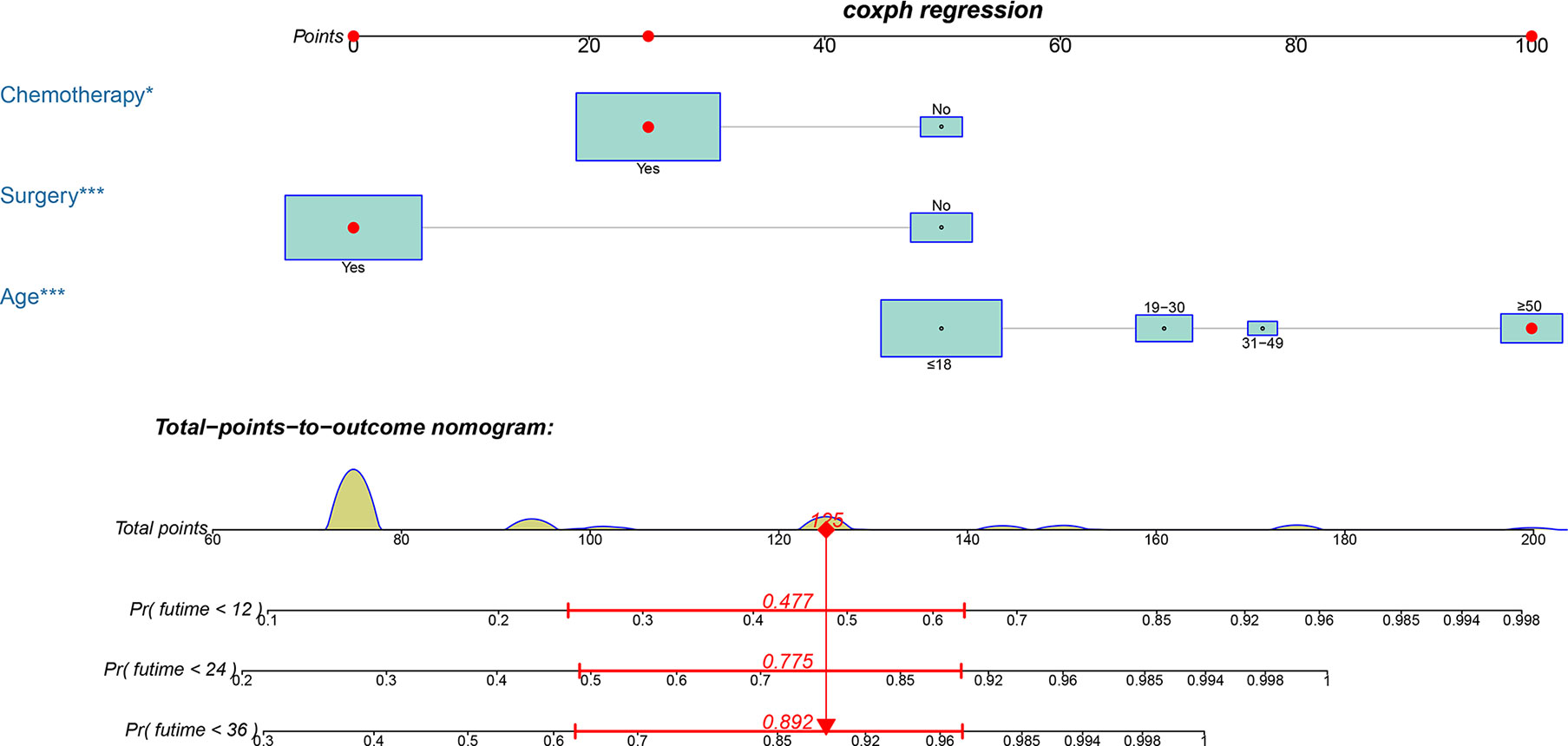
Figure 3 A prognostic nomogram for predicting the OS of osteosarcoma patients with DM for the 12, 24, and 36 months.
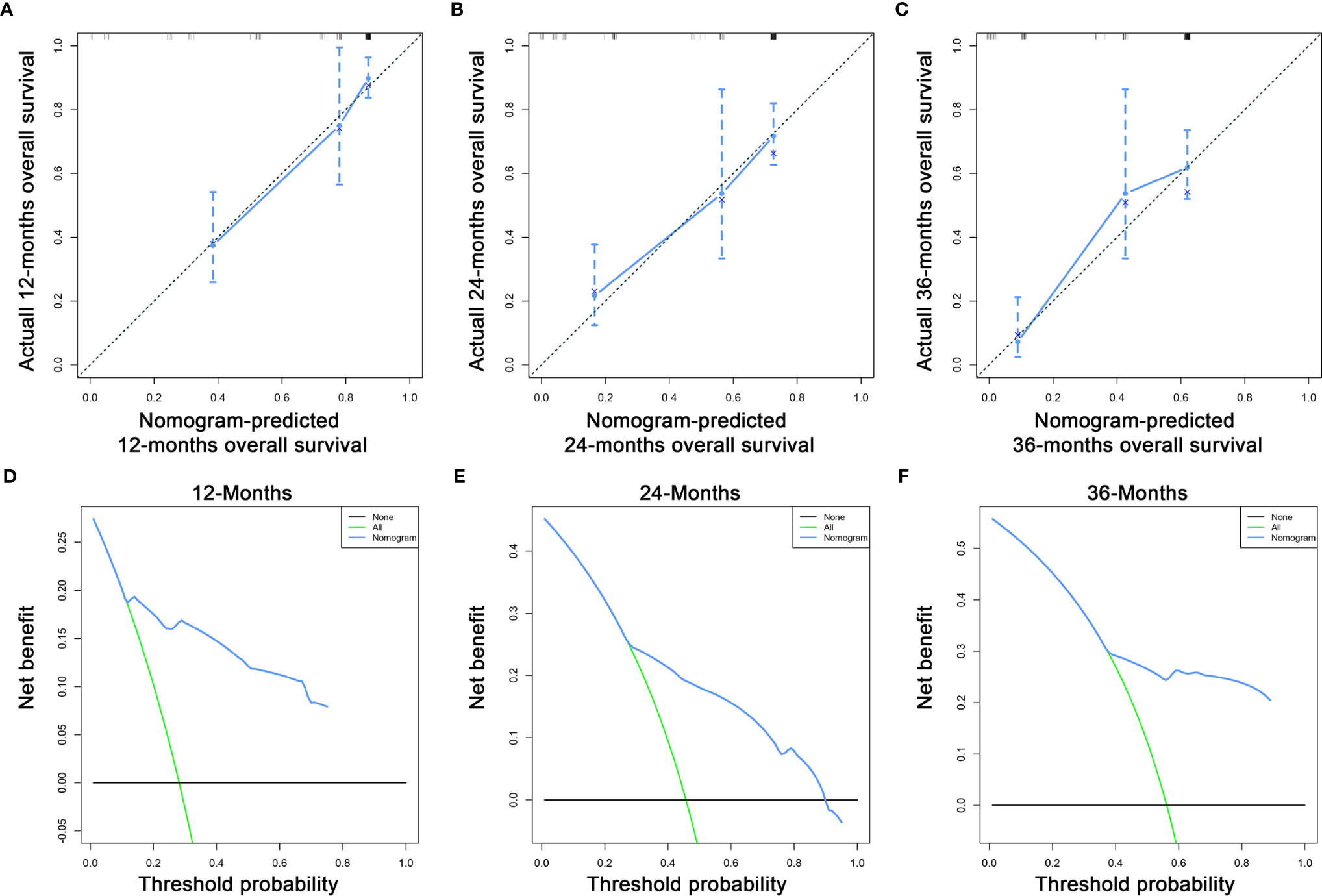
Figure 4 The calibration curves of the nomogram for the 12 (A), 24 (B), and 36 months (C) in the training set. The decision curve analysis of the nomogram for the 12 (D), 24 (E), and 36 months (F) in the training set.
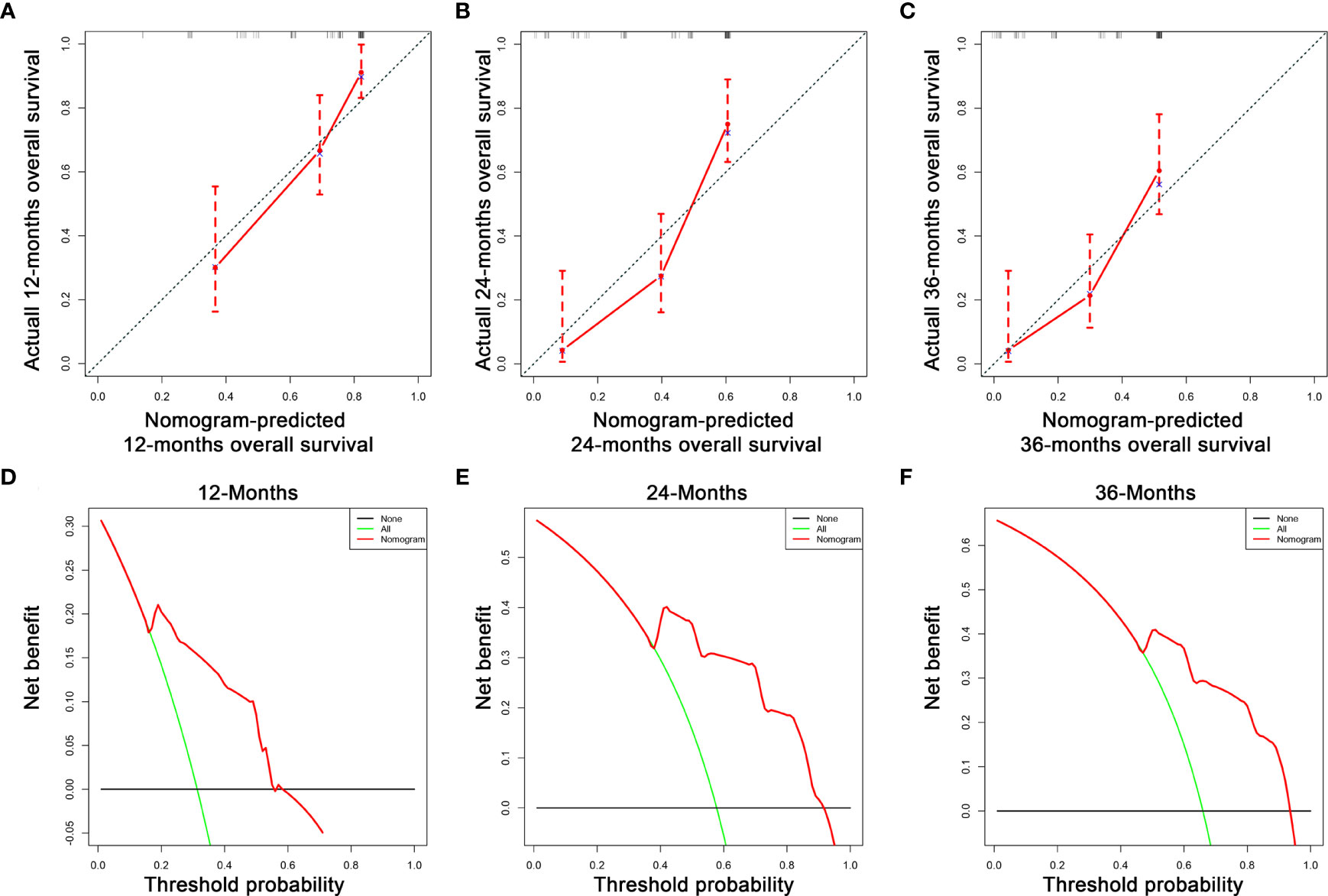
Figure 5 The calibration curves of the nomogram for the 12 (A), 24 (B), and 36 months (C) in the validation set. The decision curve analysis of the nomogram for the 12 (D), 24 (E), and 36 months (F) in the validation set.
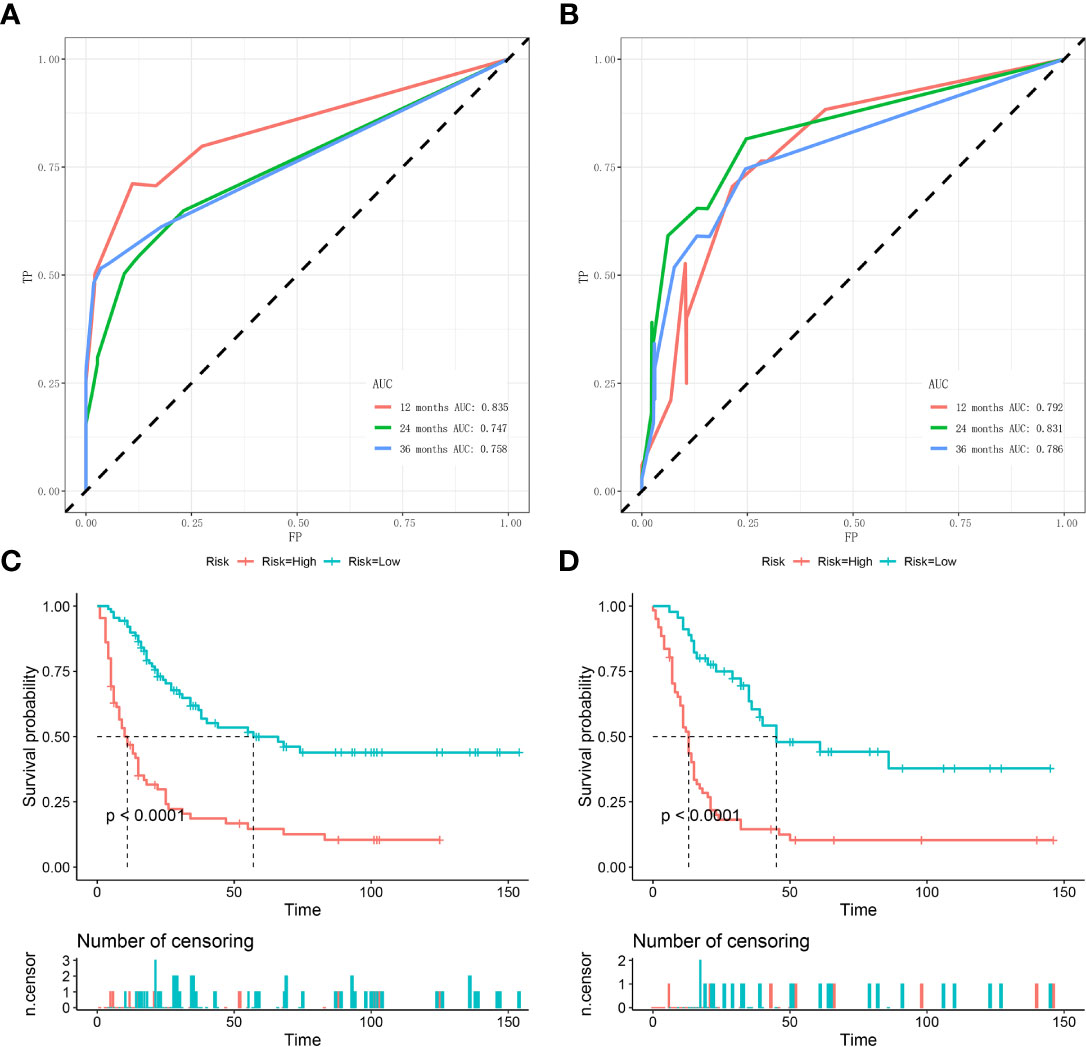
Figure 6 Time-dependent ROC curve analysis of the nomogram for the 12, 24, and 36 months in the training set (A) and the validation set (B). The Kaplan–Meier survival curves of the patients in the training set (C) and in the validation set (D).
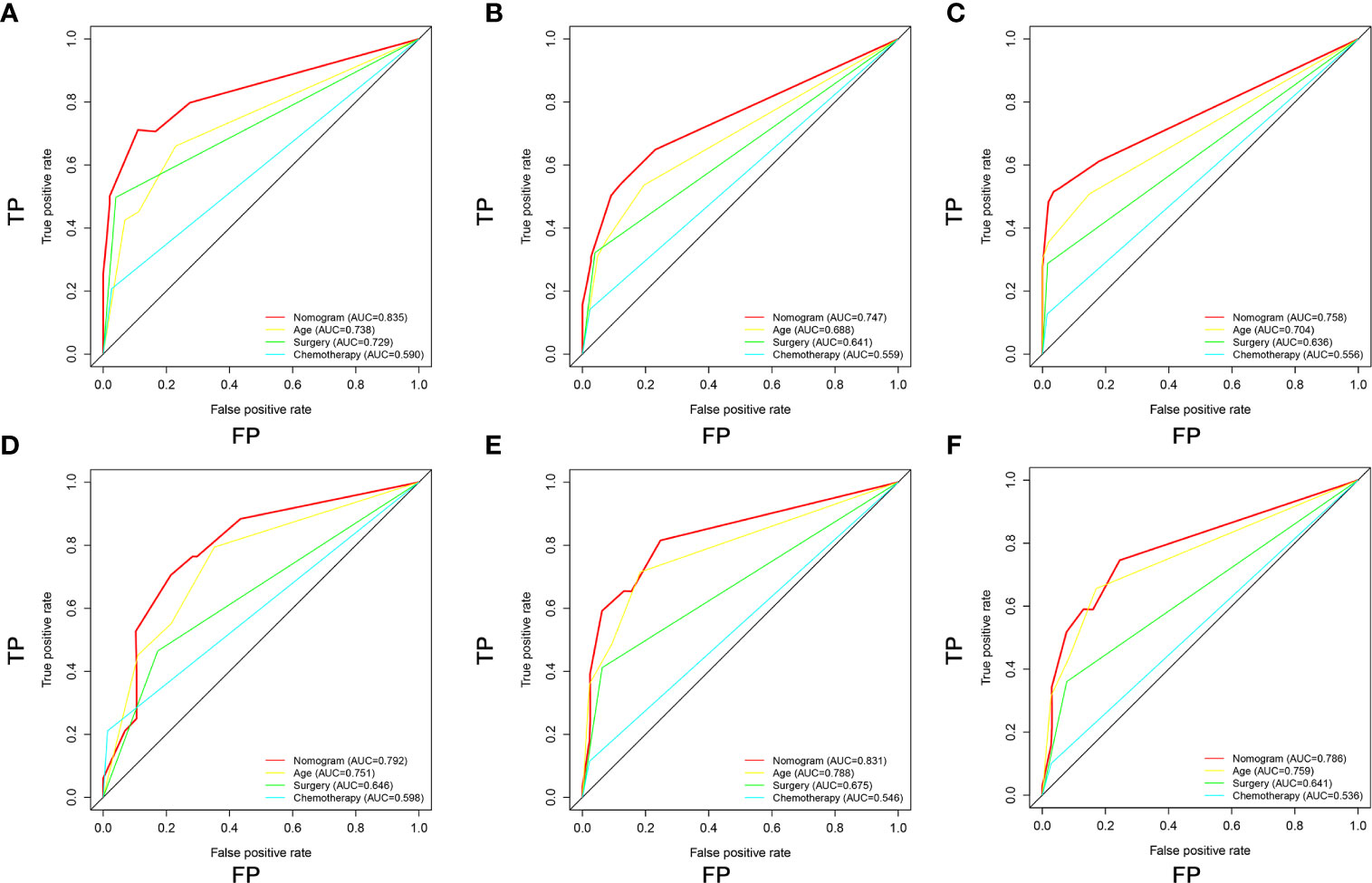
Figure 7 Comparison of area under the receiver operating characteristic curves between nomogram and all independent factors, including Age, Surgery, and Chemotherapy for the 12 (A, D), 24 (B, E), and 36 (C, F) months in the training set and validation set.
Validating the Prognostic Nomogram in an Expanded Testing Set
A total of 363 patients with DM with complete age, chemotherapy, and surgery information from the SEER database were enrolled to form an expanded testing set. In the expanded testing set, the favorable calibration plots of the prognostic nomogram implied that OS of patients with DM predicted by the nomogram were highly consistent with the actual observation (Figures 8A–C). Additionally, DCA was performed and the results proved that the prognostic nomogram can serve as an effective clinical tool (Figures 8D–F). Also, the discrimination of nomogram was better than three independent predictors in 12, 24, and 36 months (Figures 8G–I). Moreover, the AUCs of patients for 12, 24, and 36 months OS prediction were 0.804, 0.793, and 0.782 (Figure 8J), and the results of the K–M survival analysis suggested that there existed different survival patterns among patients in the high- and low-risk groups (Figure 8K).
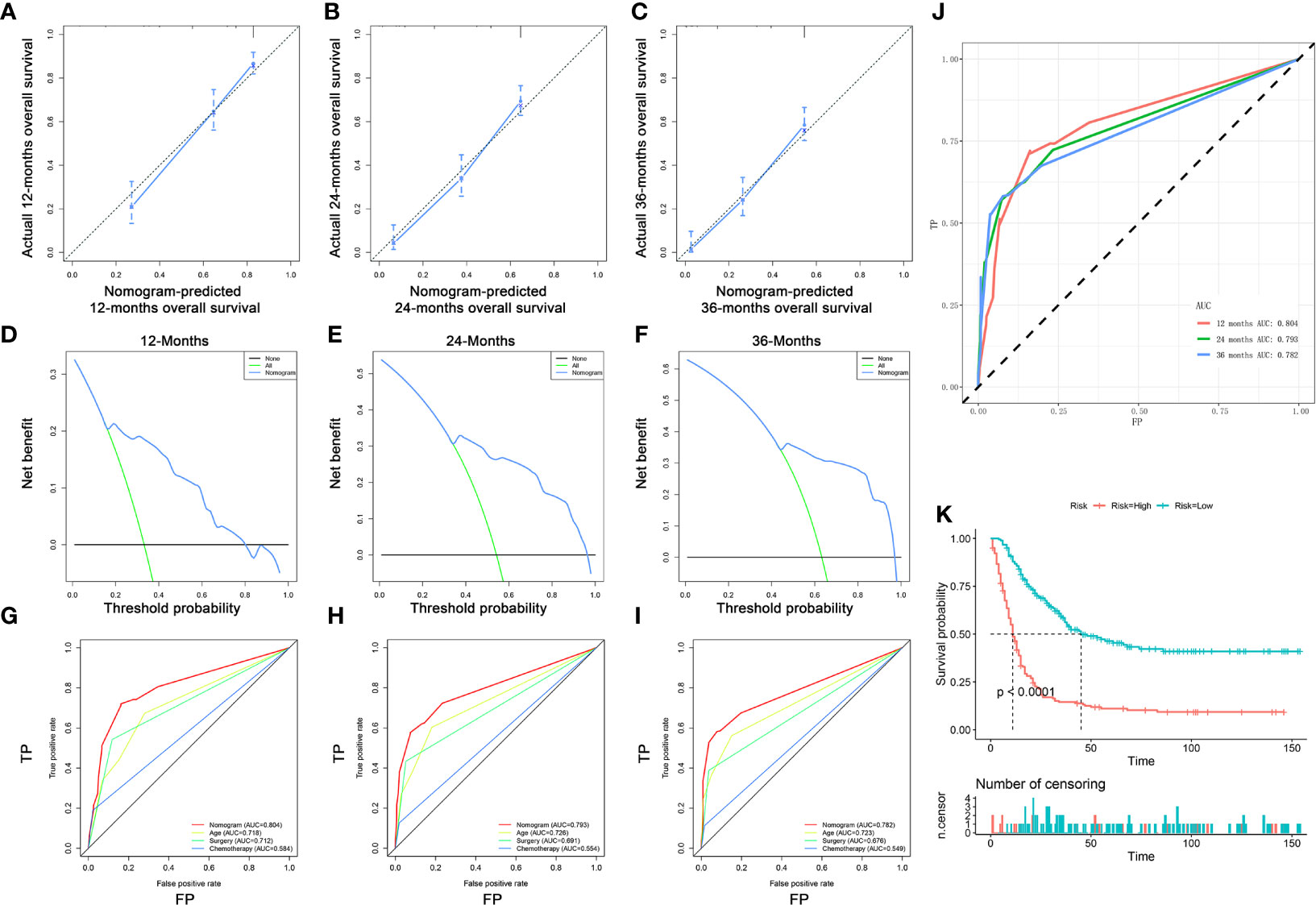
Figure 8 Validating the prognostic nomogram in the expanded testing set. The calibration curves of the nomogram for the 12 (A), 24 (B), and 36 months (C) in the expanded testing set. The decision curve analysis of the nomogram for the 12 (D), 24 (E), and 36 months (F) in the expanded testing set. Comparison of area under the receiver operating characteristic curves between nomogram and all independent factors for the 12 (G), 24 (H), and 36 (I) months in the expanded testing set. (J) Time-dependent ROC curve analysis of the nomogram for the 12, 24, and 36 months in the expanded testing set. (K) The Kaplan–Meier survival curve of the patients in the expanded testing set.
Discussion
Osteosarcoma is an aggressive tumor of bone and prone to DM, occurring in 15–40% of patients (22, 23). Almost all deaths in patients with osteosarcoma are caused by DM (24, 25). Once osteosarcoma patients develop DM, the OS decreases dramatically and the 5-year survival rate decreases to 20% (15, 26). The reason of poor prognosis of advanced osteosarcoma patients is that patients with DM could not benefit much from surgery, chemotherapy, and novel immunotherapy (6, 27). Therefore, we must identify the effective risk and prognostic factors for osteosarcoma patients with DM to diagnose at early stage, facilitate the early prevention, and evaluate the prognosis of osteosarcoma patients with DM. In the present study, we constructed a diagnostic nomogram for predicting the DM in newly diagnosed osteosarcoma patients, and a prognostic nomogram for patients with DM. By obtaining the data of several key accessible variables on the nomograms, diagnosis-related and prognosis-related scores can be calculated, which can provide guidance for further clinical evaluation and intervention.
Recently, there are many studies focused on DM in osteosarcoma, but most of them are performed at the molecular level rather than clinicopathologic features. The expression of chemokine receptor CXCR3 (28), lncRNA HNF1A-AS1 (29), and miR-206 (30) were identified to be associated with DM in osteosarcoma patients, and m6A-related signature (31), and tumor microenvironment (TME)-related signature (32) were constructed to have an early detection of DM. However, we should point out that the sample size of these studies was usually small and they were single-center studies that lacked sufficient validation, which caused these biomarkers unpractical and difficult to apply immediately to clinical management. Moreover, as for clinical characteristics research, Miller et al. determined that advanced age, tumor in the axial skeleton, larger tumor size, and residence in a less affluent county were independent predictors of metastatic disease in osteosarcoma patients (33). Another similar study that focusing on osseous neoplasms (including osteosarcoma) found that higher tumor grade, Ewing sarcoma and osteosarcoma, and larger tumor size were associated with an increased risk of lung metastasis (34). In this study, we incorporated the latest large samples with comprehensive clinical information from the SEER database and find that the incidence of DM was 16.11%, which was lower than the previous study. Four significant predictors for DM in osteosarcoma patients were determined, namely, age, N stage, T stage, and Grade. The association between TNM stage and DM in osteosarcoma patients has been confirmed in the previous studies (35, 36). However, it was unexpected that patients with age younger than 18 or older than 50 are more likely to have metastasis disease. We speculated that it may be caused by physical development status, while children’s bodies are not yet fully developed and old people are decaying. The children’s immune systems are not fully mature, and aging is accompanied by cell aging, including changes of protein, metabolism, and nuclear genome instability (37, 38), which may be involved in the occurrence and progression of tumors.
As the prognosis is extremely poor in osteosarcoma patients with DM, the early discovery of DM is crucial for patients to receive appropriate surgical resection and chemotherapy (39). To date, most studies stopped at independent risk factors and only one realistic model has been established to predict the risk of DM in osteosarcoma patients. In the similar predictive tool established by Li et al. (40), surgery, a post-diagnosis treatment was included in the nomogram for the diagnosis of DM. This sequential relationship was reverse and irrational, resulting in the model’s uselessness. To address this inadequacy, we developed a novel diagnostic nomogram based on four independent predictors, and the excellent performance was demonstrated by calibration curves, ROC curves, and DCA, which may improve the current situation of risk assessment and make the individualized clinical decision more accurate.
Although osteosarcoma patients with DM dramatically develop more lesions and become resistant to chemotherapy (14), underscoring a critical need for new treatments strategies, continuous chemotherapy still plays an important role in prolonging patients’ life and several clinical trials are still ongoing (41, 42). Surgery alone, the only effective way to treat osteosarcoma decades ago, which consisted of removing the tumor of amputating, didn’t reduce mortality below 80%, but there is still a place for osteosarcoma patients with DM (1). Interestingly, our results showed that the absence of surgery and chemotherapy had a significant negative impact on the OS, which is consistent with the above results. Radiotherapy had no significant effect on prognosis, which was consistent with the previous study (43). Moreover, it is generally believed that osteosarcoma patients with DM with higher age had a poorer OS prognosis than younger patients (44). Our study showed that patients with higher age are more likely to have poorer OS. Notably, we constructed a novel prognostic nomogram to predict the prognosis of osteosarcoma patients with DM, and the discrimination of nomogram was confirmed higher than any independent predictors, which indicated that the nomogram may open up a new prospect for personalized assessment and clinical decision-making. Although some predictive nomograms have been established in previous studies, we think our study improves upon the previous work. In the comprehensive nomogram based on gene signature and clinical predictors established by Fu et al. and Zhang et al. (45, 46), using gene signature to predict prognosis is more costly and less convenient than our nomogram with only three simple clinical variables. Additionally, the advantages of our study compared with the existing prognostic nomograms (18, 47–50) are as follow. First, we do not study the same subjects as they do. Jiang et al. used juvenile osteosarcoma as a research object (47), Gao et al. used chondroblastic osteosarcoma as a study object (48), He et al. studied patients with osteosarcoma as secondary malignancy (49), and Zhang et al. and Yang et al. made all osteosarcoma patients as study objects (18, 50), while we selected the patient with DM who lacked effective treatment and had a poor prognosis as a research object, which is more specific clinically and has not been studied. Second, our research included fewer clinical variables and had comparable or better AUC values. Third, all in the absence of external data, our study implemented more adequate verification tools, and went back to the SEER database to verify the performance of the nomogram again.
Nevertheless, we should acknowledge that this study has some shortcomings. First, the limited number of osteosarcoma patients with DM (N = 267) may have contributed to the possible error. Second, although our nomograms were constructed in the training set and validated in the validation and expanded testing sets, no available publicly osteosarcoma data in other database was enrolled, which has an inherent bias. Third, the information collected in the SEER database was about the disease at the time of initial diagnosis, which meant that the DM which occurred in the latter stage cannot be included. Additionally, although race has no effect on osteosarcoma DM and prognosis of patients with DM, most of our subjects were white, which makes the applicability of our models to other ethnic groups unknown and requires further study. Finally, we did not have specific information about systemic treatments, and this was a retrospective study and only patients with complete information were recorded.
Conclusions
Our study determined that age, N stage, T stage, and grade stage were the independent risk factors of DM for osteosarcoma, and age, surgery, and chemotherapy were the independent prognostic factors for the patients with DM. Two nomograms could be used as an intuitive graphic tool in osteosarcoma to quantitatively evaluate the risk and prognosis of osteosarcoma with DM, and guide the clinical decision-making.
Data Availability Statement
The dataset from SEER database generated and/or analyzed during the current study are available in the SEER dataset repository (https://seer.cancer.gov/).
Author Contributions
BC, YZ, and JFM conceived of and designed the study. GXL, ZXX, BL and JYC performed literature search. BC, BL and YY generated the figures and tables. BC, ZYZ and YJL analyzed the data. YZ, and BL wrote the manuscript and BC critically reviewed the manuscript. JFM supervised the research. All authors have read and approved the manuscript. BC, YZ and BL contributed equally to this work.
Conflict of Interest
The authors declare that the research was conducted in the absence of any commercial or financial relationships that could be construed as a potential conflict of interest.
Publisher’s Note
All claims expressed in this article are solely those of the authors and do not necessarily represent those of their affiliated organizations, or those of the publisher, the editors and the reviewers. Any product that may be evaluated in this article, or claim that may be made by its manufacturer, is not guaranteed or endorsed by the publisher.
Supplementary Material
The Supplementary Material for this article can be found online at: https://www.frontiersin.org/articles/10.3389/fendo.2021.672024/full#supplementary-material
References
2. Bielack S, Kempf-Bielack B, Delling G, Exner G, Flege S, Helmke K, et al. Prognostic Factors in High-Grade Osteosarcoma of the Extremities or Trunk: An Analysis of 1,702 Patients Treated on Neoadjuvant Cooperative Osteosarcoma Study Group Protocols. J Clin Oncol (2002) 20(3):776–90. doi: 10.1200/jco.2002.20.3.776
3. Wang Y, Huang Y, Xiang P, Tian W. LncRNA Expression and Implication in Osteosarcoma: A Systematic Review and Meta-Analysis. Oncol Targets Ther (2017) 10:5355–61. doi: 10.2147/ott.s149889
4. Wu G, Zhang M. A Novel Risk Score Model Based on Eight Genes and a Nomogram for Predicting Overall Survival of Patients With Osteosarcoma. BMC Cancer (2020) 20(1):456. doi: 10.1186/s12885-020-06741-4
5. Luetke A, Meyers P, Lewis I, Juergens H. Osteosarcoma Treatment - Where Do We Stand? A State of the Art Review. Cancer Treat Rev (2014) 40(4):523–32. doi: 10.1016/j.ctrv.2013.11.006
6. Jafari F, Javdansirat S, Sanaie S, Naseri A, Shamekh A, Rostamzadeh D, et al. Osteosarcoma: A Comprehensive Review of Management and Treatment Strategies. Ann Diagn Pathol (2020) 49:151654. doi: 10.1016/j.anndiagpath.2020.151654
7. Tian Z, Niu X, Yao W. Receptor Tyrosine Kinases in Osteosarcoma Treatment: Which Is the Key Target? Front Oncol (2020) 10:1642. doi: 10.3389/fonc.2020.01642
8. Shankar G, Clarke M, Ailon T, Rhines L, Patel S, Sahgal A, et al. The Role of Revision Surgery and Adjuvant Therapy Following Subtotal Resection of Osteosarcoma of the Spine: A Systematic Review With Meta-Analysis. J Neurosurg Spine (2017) 27(1):97–104. doi: 10.3171/2016.12.spine16995
9. Smeland S, Bielack S, Whelan J, Bernstein M, Hogendoorn P, Krailo M, et al. Survival and Prognosis With Osteosarcoma: Outcomes in More Than 2000 Patients in the EURAMOS-1 (European and American Osteosarcoma Study) Cohort. Eur J Cancer (2019) 109:36–50. doi: 10.1016/j.ejca.2018.11.027
10. Kager L, Zoubek A, Pötschger U, Kastner U, Flege S, Kempf-Bielack B, et al. Primary Metastatic Osteosarcoma: Presentation and Outcome of Patients Treated on Neoadjuvant Cooperative Osteosarcoma Study Group Protocols. J Clin Oncol (2003) 21(10):2011–8. doi: 10.1200/jco.2003.08.132
11. Tsuchiya H, Kanazawa Y, Abdel-Wanis M, Asada N, Abe S, Isu K, et al. Effect of Timing of Pulmonary Metastases Identification on Prognosis of Patients With Osteosarcoma: The Japanese Musculoskeletal Oncology Group Study. J Clin Oncol (2002) 20(16):3470–7. doi: 10.1200/jco.2002.11.028
12. Whelan J, Davis L. Osteosarcoma, Chondrosarcoma, and Chordoma. J Clin Oncol (2018) 36(2):188–93. doi: 10.1200/jco.2017.75.1743
13. Bielack S, Smeland S, Whelan J, Marina N, Jovic G, Hook J, et al. Methotrexate, Doxorubicin, and Cisplatin (MAP) Plus Maintenance Pegylated Interferon Alfa-2b Versus MAP Alone in Patients With Resectable High-Grade Osteosarcoma and Good Histologic Response to Preoperative MAP: First Results of the EURAMOS-1 Good Response Randomized Controlled Trial. J Clin Oncol (2015) 33(20):2279–87. doi: 10.1200/jco.2014.60.0734
14. Zhou X, Wu J, Duan C, Liu Y. Retrospective Analysis of Adoptive TIL Therapy Plus Anti-PD1 Therapy in Patients With Chemotherapy-Resistant Metastatic Osteosarcoma. J Immunol Res (2020) 2020:7890985. doi: 10.1155/2020/7890985
15. Strauss S, Ng T, Mendoza-Naranjo A, Whelan J, Sorensen P. Understanding Micrometastatic Disease and Anoikis Resistance in Ewing Family of Tumors and Osteosarcoma. Oncol (2010) 15(6):627–35. doi: 10.1634/theoncologist.2010-0093
16. Fu P, Shi Y, Chen G, Fan Y, Gu Y, Gao Z. Prognostic Factors in Patients With Osteosarcoma With the Surveillance, Epidemiology, and End Results Database. Technol Cancer Res Treat (2020) 19:1533033820947701. doi: 10.1177/1533033820947701
17. Song K, Song J, Chen F, Lin K, Ma X, Jiang J. Prognostic Nomograms for Predicting Overall and Cancer-Specific Survival of High-Grade Osteosarcoma Patients. J Bone Oncol (2018) 13:106–13. doi: 10.1016/j.jbo.2018.09.012
18. Zhang J, Yang J, Wang H, Pan Z, Yan X, Hu C, et al. Development and Validation of a Nomogram for Osteosarcoma-Specific Survival: A Population-Based Study. Med (Baltimore) (2019) 98(23):e15988. doi: 10.1097/md.0000000000015988
19. Balachandran V, Gonen M, Smith J, DeMatteo R. Nomograms in Oncology: More Than Meets the Eye. Lancet Oncol (2015) 16(4):e173–80. doi: 10.1016/s1470-2045(14)71116-7
20. Song C, Yu D, Wang Y, Wang Q, Guo Z, Huang J, et al. Dual Primary Cancer Patients With Lung Cancer as a Second Primary Malignancy: A Population-Based Study. Front Oncol (2020) 10:515606. doi: 10.3389/fonc.2020.515606
21. Huang Z, Hu C, Liu K, Yuan L, Li Y, Zhao C, et al. Risk Factors, Prognostic Factors, and Nomograms for Bone Metastasis in Patients With Newly Diagnosed Infiltrating Duct Carcinoma of the Breast: A Population-Based Study. BMC Cancer (2020) 20(1):1145. doi: 10.1186/s12885-020-07635-1
22. Kansara M, Teng M, Smyth M, Thomas D. Translational Biology of Osteosarcoma. Nat Rev Cancer (2014) 14(11):722–35. doi: 10.1038/nrc3838
23. Joko R, Yamada D, Nakamura M, Yoshida A, Takihira S, Takao T, et al. PRRX1 Promotes Malignant Properties in Human Osteosarcoma. Trans Oncol (2021) 14(1):100960. doi: 10.1016/j.tranon.2020.100960
24. Zhang X, Guan Z. PET/CT in the Diagnosis and Prognosis of Osteosarcoma. Front Biosci (Landmark edition) (2018) 23:2157–65. doi: 10.2741/4593
25. Zhang M, Liu Y, Kong D. Identifying Biomolecules and Constructing a Prognostic Risk Prediction Model for Recurrence in Osteosarcoma. J Bone Oncol (2021) 26:100331. doi: 10.1016/j.jbo.2020.100331
26. Chou A, Kleinerman E, Krailo M, Chen Z, Betcher D, Healey J, et al. Addition of Muramyl Tripeptide to Chemotherapy for Patients With Newly Diagnosed Metastatic Osteosarcoma: A Report From the Children’s Oncology Group. Cancer (2009) 115(22):5339–48. doi: 10.1002/cncr.24566
27. Janeway K, Grier H. Sequelae of Osteosarcoma Medical Therapy: A Review of Rare Acute Toxicities and Late Effects. Lancet Oncol (2010) 11(7):670–8. doi: 10.1016/s1470-2045(10)70062-0
28. Tang Y, Gu Z, Fu Y, Wang J. CXCR3 From Chemokine Receptor Family Correlates With Immune Infiltration and Predicts Poor Survival in Osteosarcoma. Biosci Rep (2019) 39(11):BSR20192134. doi: 10.1042/bsr20192134
29. Cai L, Lv J, Zhang Y, Li J, Wang Y, Yang H. The lncRNA HNF1A-AS1 Is a Negative Prognostic Factor and Promotes Tumorigenesis in Osteosarcoma. J Cell Mol Med (2017) 21(11):2654–62. doi: 10.1111/jcmm.12944
30. Xu X, Qiu B, Yi P, Li H. Overexpression of miR-206 in Osteosarcoma and Its Associated Molecular Mechanisms as Assessed Through TCGA and GEO Databases. Oncol Lett (2020) 19(3):1751–8. doi: 10.3892/ol.2020.11270
31. Li J, Rao B, Yang J, Liu L, Huang M, Liu X, et al. Dysregulated M6a-Related Regulators Are Associated With Tumor Metastasis and Poor Prognosis in Osteosarcoma. Front Oncol (2020) 10:769. doi: 10.3389/fonc.2020.00769
32. Yang B, Su Z, Chen G, Zeng Z, Tan J, Wu G, et al. Identification of Prognostic Biomarkers Associated With Metastasis and Immune Infiltration in Osteosarcoma. Oncol Lett (2021) 21(3):180. doi: 10.3892/ol.2021.12441
33. Miller B, Cram P, Lynch C, Buckwalter J. Risk Factors for Metastatic Disease at Presentation With Osteosarcoma: An Analysis of the SEER Database. J Bone Joint Surg Am (2013) 95(13):e89. doi: 10.2106/jbjs.l.01189
34. Xie L, Huang W, Wang H, Zheng C, Jiang J. Risk Factors for Lung Metastasis at Presentation With Malignant Primary Osseous Neoplasms: A Population-Based Study. J Prthopaed Surg Res (2020) 15(1):32. doi: 10.1186/s13018-020-1571-5
35. Jin L, Luo Y, Zhao Y, Tao H. MiR-183-5p Promotes Tumor Progression of Osteosarcoma and Predicts Poor Prognosis in Patients. Cancer Manag Res (2021) 13:805–14. doi: 10.2147/cmar.s285909
36. Sun Z, Liu Q, Hong H, Zhang H, Zhang T. miR-19 Promotes Osteosarcoma Progression by Targeting SOCS6. Biochem Biophys Res Commun (2018) 495(1):1363–9. doi: 10.1016/j.bbrc.2017.10.002
37. Niedernhofer L, Gurkar A, Wang Y, Vijg J, Hoeijmakers J, Robbins P. Nuclear Genomic Instability and Aging. Annu Rev Biochem (2018) 87:295–322. doi: 10.1146/annurev-biochem-062917-012239
38. Soultoukis G, Partridge L. Dietary Protein, Metabolism, and Aging. Annu Rev Biochem (2016) 85:5–34. doi: 10.1146/annurev-biochem-060815-014422
39. Isakoff M, Bielack S, Meltzer P, Gorlick R. Osteosarcoma: Current Treatment and a Collaborative Pathway to Success. J Clin Oncol (2015) 33(27):3029–35. doi: 10.1200/jco.2014.59.4895
40. Lu S, Wang Y, Liu G, Wang L, Wu P, Li Y, et al. Construction and Validation of Nomogram to Predict Distant Metastasis in Osteosarcoma: A Retrospective Study. J Prthopaed Surg Res (2021) 16(1):231. doi: 10.1186/s13018-021-02376-8
41. Pramanik R, Agarwala S, Gupta Y, Thulkar S, Vishnubhatla S, Batra A, et al. Metronomic Chemotherapy vs Best Supportive Care in Progressive Pediatric Solid Malignant Tumors: A Randomized Clinical Trial. JAMA Oncol (2017) 3(9):1222–7. doi: 10.1001/jamaoncol.2017.0324
42. Piperno-Neumann S, Le Deley M, Rédini F, Pacquement H, Marec-Bérard P, Petit P, et al. Zoledronate in Combination With Chemotherapy and Surgery to Treat Osteosarcoma (OS2006): A Randomised, Multicentre, Open-Label, Phase 3 Trial. Lancet Oncol (2016) 17(8):1070–80. doi: 10.1016/s1470-2045(16)30096-1
43. Schwarz R, Bruland O, Cassoni A, Schomberg P, Bielack S. The Role of Radiotherapy in Oseosarcoma. Cancer Treat Res (2009) 152:147–64. doi: 10.1007/978-1-4419-0284-9_7
44. Evenhuis R, Acem I, Rueten-Budde A, Karis D, Fiocco M, Dorleijn D, et al. Survival Analysis of 3 Different Age Groups and Prognostic Factors Among 402 Patients With Skeletal High-Grade Osteosarcoma. Real World Data From a Single Tertiary Sarcoma Center. Cancers (Basel) (2021) 13(3):486. doi: 10.3390/cancers13030486
45. Yiqi Z, Ziyun L, Qin F, Xingli W, Liyu Y. Identification of 9-Gene Epithelial-Mesenchymal Transition Related Signature of Osteosarcoma by Integrating Multi Cohorts. Technol Cancer Res Treat (2020) 19:1533033820980769. doi: 10.1177/1533033820980769
46. Fu Y, Bao Q, Liu Z, He G, Wen J, Liu Q, et al. Development and Validation of a Hypoxia-Associated Prognostic Signature Related to Osteosarcoma Metastasis and Immune Infiltration. Front Cell Dev Biol (2021) 9:633607. doi: 10.3389/fcell.2021.633607
47. Jiang Y, Wang T, Wei Z. Construction and Validation of Nomograms for Predicting the Prognosis of Juvenile Osteosarcoma: A Real-World Analysis in the SEER Database. Technol Cancer Res Treat (2020) 19:1533033820947718. doi: 10.1177/1533033820947718
48. Gao Z, Zhou S, Song H, Wang Y, He X. Nomograms for Predicting Overall Survival and Cancer-Specific Survival of Chondroblastic Osteosarcoma Patients. J Surg Oncol (2020) 122(8):1676–84. doi: 10.1002/jso.26185
49. He Y, Liu H, Wang S, Zhang J. A Nomogram for Predicting Cancer-Specific Survival in Patients With Osteosarcoma as Secondary Malignancy. Sci Rep (2020) 10(1):12817. doi: 10.1038/s41598-020-69740-2
Keywords: osteosarcoma, distant metastasis, nomogram, predictor, prognosis
Citation: Chen B, Zeng Y, Liu B, Lu G, Xiang Z, Chen J, Yu Y, Zuo Z, Lin Y and Ma J (2021) Risk Factors, Prognostic Factors, and Nomograms for Distant Metastasis in Patients With Newly Diagnosed Osteosarcoma: A Population-Based Study. Front. Endocrinol. 12:672024. doi: 10.3389/fendo.2021.672024
Received: 25 February 2021; Accepted: 21 June 2021;
Published: 30 July 2021.
Edited by:
Chandi C. Mandal, Central University of Rajasthan, IndiaReviewed by:
Nadia Rucci, University of L’Aquila, ItalySubhashis Pal, Emory University, United States
Copyright © 2021 Chen, Zeng, Liu, Lu, Xiang, Chen, Yu, Zuo, Lin and Ma. This is an open-access article distributed under the terms of the Creative Commons Attribution License (CC BY). The use, distribution or reproduction in other forums is permitted, provided the original author(s) and the copyright owner(s) are credited and that the original publication in this journal is cited, in accordance with accepted academic practice. No use, distribution or reproduction is permitted which does not comply with these terms.
*Correspondence: Jinfeng Ma, bWFqaW5mZW5ncWRkeEB5ZWFoLm5ldA==
†These authors have contributed equally to this work