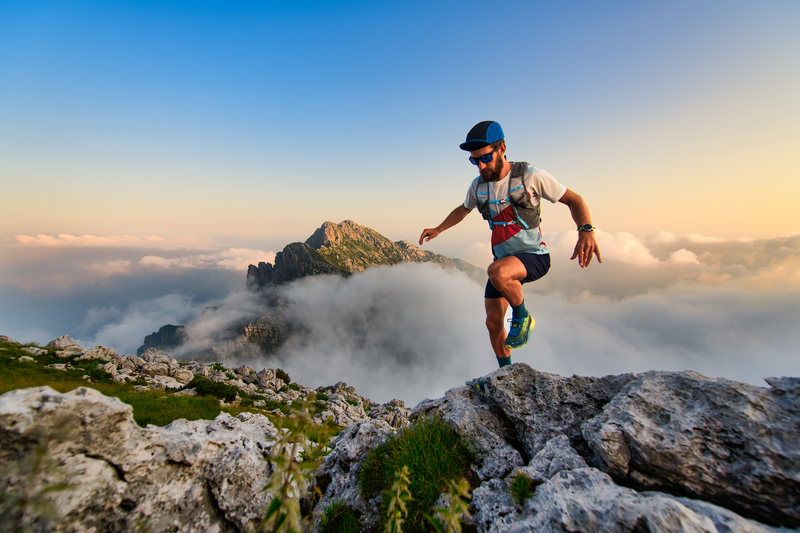
95% of researchers rate our articles as excellent or good
Learn more about the work of our research integrity team to safeguard the quality of each article we publish.
Find out more
ORIGINAL RESEARCH article
Front. Electron. , 29 April 2022
Sec. Wearable Electronics
Volume 3 - 2022 | https://doi.org/10.3389/felec.2022.895001
This article is part of the Research Topic Wearable and Implantable Electronics for the Next Generation of Human-Machine Interactive Devices View all 7 articles
Among all healthcare sectors and working processes, the janitorial section is a prominent source of work-related injuries due to its labor-intensive nature and rising need for a hygienic environment, thus requiring extra attention for prevention strategies. Advancement in robotic technology has allowed autonomous cleaning robots to be a viable solution to ease the burden of janitors. To evaluate the application of commercial-grade cleaning robots, a video-based survey was developed and distributed to participants. Results from 117 participants revealed that: 1) participants were less tolerant when their personal space was invaded by humans compared with the cleaning robot, 2) it is better to inform the surrounding humans that the cleaning robot has been sanitized to make them feel safe and comfortable during the pandemic, and 3) to make the interaction more socially acceptable, the cleaning robot should respect human personal space, especially when there is ample space to maneuver. The findings of the present study provide insight into the usage and Proxemic behaviors design of future cleaning robots.
Workers in the janitorial industry (e.g., janitors and cleaners), whose main role is to keep buildings and facilities clean and organized, are ubiquitous among all business sectors. In 2020, the Bureau of Labor Statistics (BLS) reported a total of 2.2 million people employed as janitors and building cleaners, with employment expected to expand by 6% between 2020 and 2030 (Bureau of Labor Statistics U.S. Department of Labor, 2021). Most janitors and cleaners work indoors (37% in buildings and dwellings) and perform tasks including trash emptying, supplies stocking, sweeping, mopping, vacuuming, and others (Bureau of Labor Statistics U.S. Department of Labor, 2021). In healthcare fields, due to the high requirements for a hygienic environment (e.g., to control the spread of pathogens), the expectation for janitors is even higher, with floor cleaning being one of the most demanding cleaning duties (Rutala and Weber 2019; Andersen et al., 2009).
There are considerable occupational hazards associated with janitorial work. The demand for awkward postures and repetitive labor (i.e., physical workload) from the aforementioned tasks is a normal occupational hazard that results in injuries, especially musculoskeletal disorders, sprains, bruises, and even fractures (Green et al., 2019; Schwartz et al., 2019). High exposure to cleaning agents and wet surfaces is another hazard that may lead to certain respiratory diseases and dermatologic diseases (Charles et al., 2009). In addition, they might have the possibility to suffer from stress, even mental disorders, if they perceive relatively high physical and mental workloads on their jobs (Charles et al., 2009; Schwartz et al., 2020). Although janitors and cleaners have the highest workplace incident rates (Bureau of Labor Statistics U.S. Department of Labor, 2016) and the hazards they face are quite severe, limited attention has been paid to injuring/illness prevention.
To counteract these occupational hazards and the relevant injuries and illnesses they cause, a new generation of cleaning tools may be introduced, leveraging advances in technology. Specifically, autonomous cleaning robots could be designed and implemented to allow businesses to redeploy their cleaning crews, with repetitive and mundane tasks shifted to robots whereas tasks that are more value-added and customer-facing (e.g., environmental perception, delicate manipulation, and social communication) retained by human workers. There is already an observable trend of cleaning robots being used in both in consumer and professional settings (Liu et al., 2016). Cleaning robots have been developed and merchandised in varied scales spanning from the home environment (e.g., robot vacuum cleaner) to the retail environment (e.g., autonomous floor scrubber). However, the deployment of autonomous cleaning tools may raise new safety concerns, especially for commercial-grade cleaning robots (e.g., BrainOS-powered automated robots), which are bigger in size than household robot vacuum cleaners. The presence of such robots might unnerve surrounding humans who might not be exposed to robots in the first place Chen et al., 2020. To better design an autonomous cleaning robot that can harmoniously coexist or even interact with surrounding humans, several key aspects need to be properly considered and addressed.
First, it is necessary to understand humans’ acceptance level of cleaning robots when they are sharing the same environment. Recent studies have shown that robot applications are widespread in all kinds of domains, including but not limited to space exploration (Bogue, 2012), manufacturing (Pedersen et al., 2016), agriculture (Vasconez et al., 2019), and healthcare (Shibata and Wada, 2011). In general, people have relatively positive attitudes towards social robots, with considerable variation in the acceptance level of social robots between studies (Louie et al., 2014; Naneva et al., 2020). Whereas some people still hold prejudices towards new technologies, including robots, especially when the robot fails to follow basic social norms (Kuhnert et al., 2017; de Graaf et al., 2019). However, among most of these applications, robots only have remote physical interaction (i.e., Humans and robots are separated spatially), or social interaction (e.g., entertainment, teaching, and emotional support) with humans (Goodrich and Schultz, 2008; Sheridan, 2016). The lack of proximate physical interaction, i.e., humans and robots share the same space, making the acceptance level of humans not well understood. This is important as a positive acceptance level of robots can result in tolerance of and even interest in the robot, however humans with a negative acceptance level may feel uncomfortable with the presence of robots in their close proximity (Nomura et al., 2006). Given the fact that cleaning robots have to operate in close proximity of surrounding humans, it is essential to evaluate the acceptance level of humans under these circumstances.
Second, humans’ attitudes towards the hygiene condition of major public areas, such as healthcare sectors, have evolved over time, particularly during the coronavirus pandemic. These public areas have more hygiene concerns associated with them as they are often acknowledged as potential risk sources of uncontrollable spread of infections (Munster et al., 2018; Garzaro et al., 2020). In this regard, when designing autonomous cleaning robots working in healthcare sectors, approaches to relieve human hygiene concerns are worth investigating. Moreover, even if the health fears about the coronavirus pandemic abate, the importance of hygiene is unlikely to diminish.
Last, in addition to the acceptance level and the effect of COVID-19, robot proxemic behaviors design is also of great importance, as cleaning robots usually operate near humans. Unlike physical safety, which simply requires the robot not to physically injure humans (Asimov, 2004), human mental safety and comfort demands more from the robot, as it requires not just collision-free robot motions, but also consideration and respect for social norms observed in human-human interaction (Salvini et al., 2021). Proxemics, first defined by Edward T. Hall as the physical and psychological distancing from others (Hall, 1966), is one of the most well investigated social norms in robot proxemic behaviors design (Sisbot et al., 2007; Scandolo & Fraichard, 2011). Proxemics is the theory of human behavior in which people maintain appropriate distance from each other. In a large, open room, for example, individuals tend to stand farther apart than in a crowded elevator. The perceived crowding is the factor that relates personal space to one’s perceived capability to regulate social interactions (Evans and Wener, 2007). As a relation between the number of individuals and units of space, the perceived crowding of the workspace will be dependent on its layout and arrangement. With that being said, the relative physical distancing and the environment layout are both important to consider in the robot proximal behavior design to assure human mental safety and comfort in the workspace.
Therefore, the main objectives of the study were to answer the following research questions:
RQ1: Do people tolerate cleaning robots differently than they tolerate humans in terms of the physical social distancing aspect?
RQ2: Do different hygiene conditions affect people’s comfort in terms of their physical social distancing from the cleaning robot?
RQ3: Do people have different preferences for their physical social distancing from cleaning robots and humans, and how does the preference change between different environment layouts?
Results from the study not only provide a better understanding of people’s acceptance of the cleaning robots, but can also be used to guide both the usage and the design of the proxemic behavior of it.
The data used in this study was collected via an online survey posted on Amazon Mechanical Turk, a platform that is commonly used for survey data gathering due to its large participant pool and simple participant recruitment process (Kim et al., 2020; Madathil & Greenstein, 2021). After data collection, incomplete or erratic responses were rejected and withdrawn. Results from a total of 117 participants (58 males and 59 females) were collected. The ages of participants ranged from 21 to 74, with the mean (SD) age of 42.79 (11.72). Among the 117 participants, 16 of them were Asians, 12 were Black or African Americans, and 89 were Whites. The study was approved by the University of Florida Institutional Review Board (IRB202100165). The participants were given $3 as compensation for participating in the study.
The mobile robot used in the study was the Fetch Freight Base (Fetch Robotics, Inc, San Jose, CA). The Freight Base is 359 mm tall, 508 mm wide, and 559 mm diameter, with a payload of up to 100 kg. For real-time safety precaution, the robot was equipped with an emergency stop button. To better simulate the dimension of the cleaning robot, modifications were made on the Freight Base to make the whole system approximately 1,690 mm tall (a comparable size of normal commercial-grade cleaning robots). During the video recording, the robot was tele-operated by the researcher at around 0.4 m/s. The travel speed of the robot was determined based on our pilot test, in which we measured the worker, acted by a researcher, moving at about 0.4 m/s while pushing the cart.
As a compromise during the pandemic, an online survey containing video-based materials was provided to the participants for data collection. There were 16 video clips in the survey, with durations ranging from 10 to 30 seconds. Each video clip consists of two components: a real-world recording and a 2D simulation animation (Figure 1A,B). The real-world recordings were collected in a lab space that mimicked the environment of the medical supply storage room. The worker in the green shirt was acted by a researcher, who was searching and picking an item off the shelf without moving from his place, while the other agent, either a cleaning robot (Figure 1A) or worker in blue pushing the cart (Figure 1A), passed the customer in green in order to travel to the target position and accomplish a certain task. Before watching each video, participants were informed that the task for the cleaning robot was to clean up spills and/or contaminants on the floor, and the customer in blue was to pick the item at the target location. The animations were generated using MATLAB R2019B (MathWorks, Inc, Natick, MA). As shown in Figure 1A,B, black blocks represented the shelves, the green block represented the worker in green in the real-world recording, the blue block represented the cleaning robot or the worker in blue, and their trajectory along with the start and target positions were also provided. The real-world recording and the 2D simulation animation were placed side by side and synced such that the moving speed of the blue agent was roughly the same. Each of the videos could be played as many times as possible.
FIGURE 1. (A) A screenshot of the video clip in which the cleaning robot passed the worker in the green shirt (B) A screenshot of the video clip in which the worker in the green shirt was passed by another worker pushing a cart.
In order to answer the three research questions given above, the following independent variables were included:
Agent type (Agent) - Agent type refers to the type of agent with whom the worker in green was sharing space with. Two types of agents were tested in the study. As shown in Figure 1A,B, the worker in green was passed by either a cleaning robot or another worker pusher a cart. The task for the cleaning robot was to clean up spills and/or contaminants on the floor, and the worker in blue was to pick the item at the target location.
Hygiene condition (Hygiene) - For every type of agent, two levels of hygiene conditions were tested. For the cleaning robot, the two levels were: with and without a “sanitized” sign (Figure 2A). For the customer in blue, the two levels were: with and without a mask on (Figure 2B). The hygiene condition was included to test the potential effect of COVID-19.
FIGURE 2. (A) The sign that the cleaning robot had or not (B) The mask that the customer in blue had or not. The same pictures were shown to the participants to reinforce their awareness of the hygiene condition before each video.
Environment layout (Layout) - Two levels of the healthcare sector layouts were tested: narrow aisle and wide aisle (Figure 3). The narrow aisle represented the environment where only two people may pass at a time, while the wide aisle represented an environment with plenty of room.
Physical distancing (Distancing) - At the moment the worker in green was passed by the other agent, the distance between them was the physical distancing. This was tested at two levels for each environment layout: close and far. As shown in Figure 3, while close physical distancing refers to the cleaning robot or the worker in blue passed right behind the worker in green in both layouts, far physical distancing refers to the cleaning robot or the worker in blue passed the worker in green by taking a detour in the narrow aisle layout or maintaining a distance around 2 m in the wide aisle layout.
The four independent variables, with two different levels of each, produced a total of 16 (2x2x2x2) video clips for every participant.
The study only looked at one dependent variable: the participants’ level of comfort in terms of their physical distancing from the other agent, which will be referred to as the spatial comfort, or rating, interchangeably. After each of the videos, participants were asked to rate their spatial comfort on a Likert scale ranging from very uncomfortable (i.e., 0) to very comfortable (i.e., 10). According to anthropologists’ observations, most people value their personal space and feel discomfort, anger, or anxiety when their personal space is invaded (Hall, 1966). Allowing someone to enter personal space or not could be seen as an indicator of perception of their relationship.
The survey consisted of four parts: 1) a description of the study’s purpose, 2) an informed consent, 3) a demographic questionnaire, and 4) a series of videos followed by video-related questions. Participants were first given an introduction regarding the purpose of the study and the experimental task (i.e., watching videos and answering questions related to them). Next, every participant was asked to read and sign the informed consent to indicate their voluntary participation in the study. The participants then completed a demographic questionnaire, including questions about their age, gender, race, and ethnicity, followed by experimental tasks. The main task for participants was to watch videos, imagine themselves as the worker in green searching and picking an item from the shelf, and rate their spatial comfort at the time the other agent (could be either the cleaning robot or another worker in blue pushing a cart) passed them on a Likert scale ranging from very uncomfortable (i.e., 0) to very comfortable (i.e., 10). A CAPTCHA was utilized at the end of the survey to ensure bots were not used to complete the tasks.
According to the Central Limit Theorem, and the fact that the sample size was substantially larger than 30, the assumption of normality could be relaxed (Pek et al., 2018; Islam, 2018). Therefore, four-way repeated measures analyses of variance (ANOVA) were performed with “participant” being the blocking variable. Interaction effects were included in our model. In the case of significant interactions, paired t-tests were conducted to evaluate follow up paired comparisons (Dixon et al., 2018). The test statistics from ANOVA, F-statistic, was used to reflect the ratio of variances per between-group and within-group variance (Kim, 2017). The corresponding significance probability value, p-value, was then used to measure the evidence against the null hypothesis (Hoijtink et al., 2019). All analyses were conducted using SPSS v26 (IBM Corporation, Armonk, NY) with statistical significance achieved when p < 0.05. Partial Eta squared ηp2 was used to determine the effect size.
Table 1 summarizes the statistical analysis results of the main effects of agent, hygiene, layout, and distancing amount. A significant effect of agent type on participants’ spatial comfort was observed (F (1,1740) = 72.810, p < 0.001, ηp2 = 0.040), more specifically, participants’ subjective rating when passed by the cleaning robot (7.1) was significantly higher than passed by the human customer (6.4). Hygiene condition was also found to have a significant effect on participants’ spatial comfort (F (1,1740) = 145.435, p < 0.001, ηp2 = 0.077), i.e., a “sanitized” sign or a mask significantly increased participants’ spatial comfort when they were passed by the other agent (from 6.2 to 7.3). In addition, the spatial comfort of participants in different environment layouts was revealed to be significantly varied (F (1,1740) = 217.100, p < 0.001, ηp2 = 0.111): when participants were passed by the other agent, their spatial comfort in the narrow aisle (7.4) was significantly greater than in the wide aisle (6.1). Furthermore, participants’ spatial comfort was observed to be significantly affected by physical distancing (F (1,1740) = 1,552.204, p < 0.001, ηp2 = 0.471). Specifically, participants’ spatial comfort was significantly higher when the distance between them and the other agent was greater (compared 8.5 to 5.0).
All interaction effects are summarized in Table 2. Results revealed a significant interaction between agent type and hygiene condition (F (1,1740) = 43.143, p < 0.001, ηp2 = 0.024). Follow-up paired t-tests (Figure 4A) showed that participants’ spatial comfort of the human customer was significantly lower than the cleaning robot in the “w/o” hygiene condition (change of 1.4, p < 0.001), while a non-significant difference was found in the “w/” condition (change of 0.2, p = 0.092). A significant agent * layout interaction was also observed (F (1,1740) = 4.096, p = 0.043, ηp2 = 0.002). Follow-up paired t-tests (Figure 4B) revealed that participants’ spatial comfort of the human customer was significantly lower than the cleaning robot in either the narrow aisle (p < 0.001) or the wide aisle (p < 0.001), moreover, the difference was greater in the narrow aisle (change of 0.9) compared to the wide aisle (change of 0.6). Additionally, the interaction between agent type and physical distancing was also found to be significant (F (1,1740) = 4.000, p = 0.046, ηp2 = 0.002). Follow-up paired t-tests (Figure 4C) showed that participants’ spatial comfort of the human customer was significantly lower than the cleaning robot in both the “close” (p < 0.001) and “far” (p < 0.001) conditions and the difference was greater in the “close” condition (change of 1.0) than the “far” condition (change of 0.6). Furthermore, layout * distancing was also shown to be a significant interaction (F (1,1740) = 131.513, p < 0.001, ηp2 = 0.070). As shown in Figure 4D, paired t-tests revealed that participants’ spatial comfort was significantly higher when the distance between them and the other agent was greater, regardless of the environment layout (p < 0.001 for both pairs), and the difference was greater in the “wide” condition (change of 4.6) than the “narrow” condition (change of 2.5). Interactions other than the aforementioned four were all non-significant according to our statistical analysis.
FIGURE 4. Summary of interaction effects between agent * hygiene (A), agent * layout (B), agent * distancing (C), and layout * distancing (D). The star symbol indicates a significant difference between pairs. Mean values of the subjective rating are represented by the bars and the numbers on it. The error bars show the confidence intervals.
To begin with, our statistical analysis revealed that, in general, participants had significantly higher tolerances for the cleaning robot than the human worker in terms of their spatial comfort (p < 0.001). More specifically, a significant interaction between agent and hygiene was observed (p < 0.001). Follow-up paired t-tests showed that when passed by another agent in the same space, participants’ tolerances for a cleaning robot without a “sanitized” sign was significantly higher than a customer without a mask (Figure 4A, p < 0.001). Consistent with the finding in Cartaud et al., 2020, we argue that due to the COVID-19 pandemic, which is currently ongoing at the time of this study, the public has come to expect most people they interact with to wear masks. Violating this norm may result in a reduction in their spatial comfort. On the contrary, robots may not be treated as a common medium of COVID transmission, this might explain why participants’ spatial comfort dropped more significantly when they passed by the unmasked human customer over a cleaning robot without a “sanitized” sign on it. A similar trend was found in the paired t-test shown in Figure 4B, showing that participants were significantly less tolerant of the human customer than the cleaning robot in both environment layouts. These findings could serve as evidence that introducing cleaning robots into healthcare sectors will not increase public concern about COVID. However, we still observed a decrease in participants’ spatial comfort when they passed by a robot without a “sanitized'' sign compared to a robot with the sign (Figure 4A), hence, it is necessary to not only sanitize the cleaning robot on a regular basis, but also to inform the customers that the robot has been sanitized. According to the study results, one effective approach to delivering such information could be putting a “sanitized'' sign on the cleaning robot.
In addition to the agent type and hygiene condition, physical distancing showed a significant effect on participants’ spatial comfort as well (p < 0.001). Our results indicated that participants strongly prefer the other agent to pass them while maintaining a greater distance. According to Proxemics theory (Hall, 1966), personal space surrounding each person could be divided into four different zones: 1) intimate zone (0–0.45 m), 2) personal zone (0.45–1.2 m), 3) social zone (1.2–3.6 m), and 4) public zone (>3.6 m). Given that the distances between the two agents in videos were controlled to less than 1 m (intimate zone or personal zone) in the “close” condition and around 2 m (social zone) in the “far” condition, our finding supported that individuals are discomforted when their personal space is invaded even in virtual settings (Chandra et al., 2020). Furthermore, the significant agent * distancing interaction (p = 0.046) indicated that participants’ tolerance for the cleaning robot were even higher than the human customer in the “close” condition (Figure 4C). This was contradictory to the finding in Joosse et al., 2013, in which the authors found that participants’ reactions were stronger when their personal space was invaded by a robot compared with a person. We argue that the inconsistent results may be due to the difference in context, but more importantly, in our study, the role of the robot (i.e., cleaning robot) and its intention (i.e., clean up spills and/or contaminants on the floor) were well delivered to the participants, whereas in Joosse et al., 2013, such information was not specified. In line with our prior works (Chen et al., 2021; Smith et al., 2021), the findings suggest that for the design of the proxemic behavior of cleaning robots, it is necessary to take human personal space into account to make the robot motions more socially acceptable.
Participants’ spatial comfort was also found to be significantly affected by the environment layout (p < 0.001). Our results indicated that participants’ tolerances for spatial comfort are significantly higher in the narrow aisle, where only two individuals may pass at a time, than in the wide aisle. Although the physical collision chance could be lower in a large and open space, participants’ expectation of respect for their personal space was higher. This finding was aligned with our expectation, i.e., people have stronger expectations of others to respect their personal space when there is plenty of space. Therefore, participants would be less tolerant of personal space invasions in the wide aisle setting. This point of view could be supported by the significant interaction between environment layout and physical distancing (p < 0.001). As shown in Figures 4A,D significantly lower rating was observed when the distance between the two agents changed from “far” to “close” in both narrow and wide settings (p < 0.001 for layout conditions). Moreover, the decrease in participants’ spatial comfort was more substantial for the “wide” condition, further proving our claim. In summary, for robot proxemic behaviors design, the robots must respect human personal space when interacting with people to increase their spatial comfort, especially when there is plenty of room to maneuver.
Several limitations have to be mentioned for this study. First, although the video-based survey approaches further improved the engagement compared to traditional text and image based surveys, participants’ perceptions could still be different from physically conducting the experiment by themselves. Second, the commercial-grade cleaning robot in the study was simulated with a customized general purpose mobile robot platform. Although they are similar in dimensions, differences in appearance, sounds made, and other factors may influence participants’ perception of safety and comfort.
Cleaning robots, being a new generation of cleaning tools, are now capable of being deployed in close proximity to humans. In this study, a video-based survey was developed to test participants’ acceptance of the commercial-grade cleaning robot and their spatial comfort when interacting with cleaning robots in multiple conditions. Results showed that when passed by another agent in the shared retail environment, participants had a significantly higher tolerance for the cleaning robot than for another customer in terms of their spatial comfort. The presence of other people had a stronger effect on participant proxemics and participants were less tolerant when their personal space was violated by the customer compared with the cleaning robot. In addition, when sharing the space with another customer, participants’ spatial comfort was higher if the customer was masked. Similarly, it is also necessary to inform people that the cleaning robot has been sanitized during the pandemic. Furthermore, results from the study revealed that participants preferred to be passed with a distance of roughly 2 m between them and the other agent over a shorter distance (<1 m). The preference is stronger in a large and open environment. Indicating the importance of considering and respecting human personal space in robot proxemic behaviors design, especially when there is ample space to maneuver.
The raw data supporting the conclusions of this article will be made available by the authors, without undue reservation.
The studies involving human participants were reviewed and approved by The study was approved by the University of Florida Institutional Review Board (IRB202100165). The patients/participants provided their written informed consent to participate in this study.
BH and YC designed research. YC and YL performed research and analyzed the data. YC, YL and BH wrote and revised the article. All authors contributed to the article and approved the submitted version.
This investigation was made possible by Grant No. T42OH00 8673 from the National Institute for Occupational Safety and Health (NIOSH) and Award No. 2026276 from the National Science Foundation (NSF).
The authors declare that the research was conducted in the absence of any commercial or financial relationships that could be construed as a potential conflict of interest.
All claims expressed in this article are solely those of the authors and do not necessarily represent those of their affiliated organizations, or those of the publisher, the editors and the reviewers. Any product that may be evaluated in this article, or claim that may be made by its manufacturer, is not guaranteed or endorsed by the publisher.
The authors would like to thank Yu Gu for providing professional advice on robotics; Shuai Hao, Jared Yu, and Jack Gonzales for their assistance in video editing and survey development.
Andersen, B. M., Rasch, M., Kvist, J., Tollefsen, T., Lukkassen, R., Sandvik, L., et al. (2009). Floor Cleaning: Effect on Bacteria and Organic Materials in Hospital Rooms. J. Hosp. Infect. 71 (1), 57–65. doi:10.1016/j.jhin.2008.09.014
Bogue, R. (2012). Robots for Space Exploration. Ind. Robot: Int. J. 39 (4), 323–328. doi:10.1108/01439911211227872
Bureau of Labor Statistics U.S. Department of Labor (2016). Nonfatal Occupational Injuries and Illnesses Requiring Days Away from Work, 2015. Available at: https://www.bls.gov/news.release/archives/osh2_11102016.pdf (Accessed Nov 11, 2021).
Bureau of Labor Statistics U.S. Department of Labor (2021). Occupational Outlook Handbook. Janitors and Building Cleaners. Available at: https://www.bls.gov/ooh/building-and-grounds-cleaning/janitors-and-building-cleaners.htm?_9999%3Fqbd#tab-1 (Accessed Nov 11, 2021).
Cartaud, A., Quesque, F., and Coello, Y. (2020). Wearing a Face Mask against Covid-19 Results in a Reduction of Social Distancing. Plos one 15 (12), e0243023. doi:10.1371/journal.pone.0243023
Chalil Madathil, K., and Greenstein, J. S. (2021). Designing Comprehensible Healthcare Public Reports: An Investigation of the Use of Narratives and Tests of Quality Metrics to Support Healthcare Public Report Sensemaking. Appl. Ergon. 95, 103452. doi:10.1016/j.apergo.2021.103452
Chandra, S., Shirish, A., and Srivastava, S. C. (2020). Theorizing Technological Spatial Intrusion for ICT Enabled Employee Innovation: The Mediating Role of Perceived Usefulness. Technol. Forecast. Soc. Change 161, 120320. doi:10.1016/j.techfore.2020.120320
Charles, L. E., Loomis, D., and Demissie, Z. (2009). Occupational Hazards Experienced by Cleaning Workers and Janitors: a Review of the Epidemiologic Literature. Work 34 (1), 105–116. doi:10.3233/wor-2009-0907
Chen, Y., Smith, T., Hewitt, N., Gu, Y., and Hu, B. (2021). “Effects of Human Personal Space on the Robot Obstacle Avoidance Behavior: A Human-In-The-Loop Assessment,” in Proceedings of the Human Factors and Ergonomics Society Annual Meeting, Baltimore, Maryland, October 4–7, 2021 (Sage CALos Angeles, CA: SAGE Publications) 65 (1), 1195–1199. doi:10.1177/1071181321651098
Chen, Y., Yang, C., Song, B., Gonzalez, N., Gu, Y., and Hu, B. (2020). “Effects of Autonomous Mobile Robots on Human Mental Workload and System Productivity in Smart Warehouses: A Preliminary Study,” in Proceedings of the Human Factors and Ergonomics Society Annual Meeting, Virtual Meeting, October 5–9, 2020 (Los Angeles, CA: Sage CASAGE Publications) 64 (1), 1691–1695. doi:10.1177/1071181320641410
de Graaf, M. M. A., Ben Allouch, S., and Van Dijk, J. A. G. M. (2019). Why Would I Use This in My home? A Model of Domestic Social Robot Acceptance. Human-Computer Interaction 34 (2), 115–173. doi:10.1080/07370024.2017.1312406
Dixon, P. C., Schütte, K. H., Vanwanseele, B., Jacobs, J. V., Dennerlein, J. T., and Schiffman, J. M. (2018). Gait Adaptations of Older Adults on an Uneven brick Surface Can Be Predicted by Age-Related Physiological Changes in Strength. Gait & posture 61, 257–262. doi:10.1016/j.gaitpost.2018.01.027
Evans, G. W., and Wener, R. E. (2007). Crowding and Personal Space Invasion on the Train: Please Don't Make Me Sit in the Middle. J. Environ. Psychol. 27 (1), 90–94. doi:10.1016/j.jenvp.2006.10.002
Garzaro, G., Clari, M., Ciocan, C., Grillo, E., Mansour, I., Godono, A., Borgna, L. G., Sciannameo, V., Costa, G., Raciti, I. M., Bert, F., Berchialla, P., Coggiola, M., and Pira, E. (2020). COVID-19 Infection and Diffusion Among the Healthcare Workforce in a Large university-hospital in Northwest Italy. Med. Lav 111 (3), 184–194. doi:10.23749/mdl.v111i3.9767
Goodrich, M. A., and Schultz, A. C. (2008). Human-robot Interaction: A Survey. Boston, MA: Now Publishers Inc.
Green, D. R., Gerberich, S. G., Kim, H., Ryan, A. D., McGovern, P. M., Church, T. R., Schwartz, A., and Arauz, R. F. (2019). Occupational Injury Among Janitors. J. Occup. Environ. Med. 61 (2), 153–161. doi:10.1097/jom.0000000000001505
Hoijtink, H., Mulder, J., van Lissa, C., and Gu, X. (2019). A Tutorial on Testing Hypotheses Using the Bayes Factor. Psychol. Methods 24 (5), 539–556. doi:10.1037/met0000201
Islam, M. R. (2018). Sample Size and its Role in Central Limit Theorem (CLT). Comput. Appl. Math. J. 4 (1), 1–7. doi:10.31295/pm.v1n1.42
Joosse, M., Sardar, A., Lohse, M., and Evers, V. (2013). BEHAVE-II: The Revised Set of Measures to Assess Users' Attitudinal and Behavioral Responses to a Social Robot. Int. J. Soc. Robotics 5 (3), 379–388. doi:10.1007/s12369-013-0191-1
Kim, T. K. (2017). Understanding One-Way ANOVA Using Conceptual Figures. Korean J. Anesthesiol 70 (1), 22. doi:10.4097/kjae.2017.70.1.22
Kim, W., Kim, N., Lyons, J. B., and Nam, C. S. (2020). Factors Affecting Trust in High-Vulnerability Human-Robot Interaction Contexts: A Structural Equation Modelling Approach. Appl. Ergon. 85, 103056. doi:10.1016/j.apergo.2020.103056
Kuhnert, B., Ragni, M., and Lindner, F. (2017). “The gap between Human's Attitude towards Robots in General and Human's Expectation of an Ideal Everyday Life Robot,” in 2017 26th IEEE international symposium on robot and human interactive communication (RO-MAN), Lisbon, Portugal, August 28–September 1, 2017 (IEEE), 1102–1107. doi:10.1109/roman.2017.8172441
Liu, S., Zheng, L., Wang, S., Li, R., and Zhao, Y. (2016). “June). Cognitive Abilities of Indoor Cleaning Robots,” in 2016 12th World Congress on Intelligent Control and Automation (WCICA) (IEEE), 1508
Louie, W.-Y. G., McColl, D., and Nejat, G. (2014). Acceptance and Attitudes toward a Human-like Socially Assistive Robot by Older Adults. Assistive Techn. 26 (3), 140–150. doi:10.1080/10400435.2013.869703
Munster, V. J., Bausch, D. G., de Wit, E., Fischer, R., Kobinger, G., Muñoz-Fontela, C., Olson, S. H., Seifert, S. N., Sprecher, A., Ntoumi, F., Massaquoi, M., and Mombouli, J.-V. (2018). Outbreaks in a Rapidly Changing Central Africa - Lessons from Ebola. N. Engl. J. Med. 379 (13), 1198–1201. doi:10.1056/nejmp1807691
Naneva, S., Sarda Gou, M., Webb, T. L., and Prescott, T. J. (2020). A Systematic Review of Attitudes, Anxiety, Acceptance, and Trust towards Social Robots. Int. J. Soc. Robotics 12 (6), 1179–1201. doi:10.1007/s12369-020-00659-4
Nomura, T., Suzuki, T., Kanda, T., and Kato, K. (2006). Measurement of Negative Attitudes toward Robots. Is 7 (3), 437–454. doi:10.1075/is.7.3.14nom
Pedersen, M. R., Nalpantidis, L., Andersen, R. S., Schou, C., Bøgh, S., Krüger, V., et al. (2016). Robot Skills for Manufacturing: From Concept to Industrial Deployment. Robotics and Computer-Integrated Manufacturing 37, 282–291. doi:10.1016/j.rcim.2015.04.002
Pek, J., Wong, O., and Wong, A. C. M. (2018). How to Address Non-normality: A Taxonomy of Approaches, Reviewed, and Illustrated. Front. Psychol. 9, 2104. doi:10.3389/fpsyg.2018.02104
Rutala, W. A., and Weber, D. J. (2019). Best Practices for Disinfection of Noncritical Environmental Surfaces and Equipment in Health Care Facilities: A Bundle Approach. Am. J. Infect. Control. 47, A96–A105. doi:10.1016/j.ajic.2019.01.014
Salvini, P., Paez-Granados, D., and Billard, A. (2021). On the Safety of Mobile Robots Serving in Public Spaces. J. Hum.-Robot Interact. 10, 1–27. doi:10.1145/3442678
Scandolo, L., and Fraichard, T. (2011). An Anthropomorphic Navigation Scheme for Dynamic Scenarios.” in IEEE International Confer-Ence on Robotics and Automation, Shanghai, China, May 9–13, 2011. IEEE, 809–814.
Schwartz, A., Gerberich, S. G., Albin, T., Kim, H., Ryan, A. D., Church, T. R., Green, D. R., McGovern, P. M., Erdman, A. G., and Arauz, R. F. (2020). The Association between Janitor Physical Workload, Mental Workload, and Stress: The SWEEP Study. Work 65 (4), 837–846. doi:10.3233/wor-203135
Schwartz, A., Gerberich, S. G., Kim, H., Ryan, A. D., Church, T. R., Albin, T. J., McGovern, P. M., Erdman, A. E., Green, D. R., and Arauz, R. F. (2019). Janitor Ergonomics and Injuries in the Safe Workload Ergonomic Exposure Project (SWEEP) Study. Appl. Ergon. 81, 102874. doi:10.1016/j.apergo.2019.102874
Sheridan, T. B. (2016). Human-Robot Interaction: Status and Challenges. Hum. Factors 58 (4), 525–532. doi:10.1177/0018720816644364
Shibata, T., and Wada, K. (2011). Robot Therapy: A New Approach for Mental Healthcare of the Elderly – A Mini-Review. Gerontology 57 (4), 378–386. doi:10.1159/000319015
Sisbot, E. A., Marin-Urias, L. F., Alami, R., and Simeon, T. (2007). A Human Aware mobile Robot Motion Planner. IEEE Trans. Robot. 23 (5), 874–883. doi:10.1109/tro.2007.904911
Smith, T., Chen, Y., Hewitt, N., Hu, B., and Gu, Y. (2021). Socially Aware Robot Obstacle Avoidance Considering Human Intention and Preferences. Int. J. Soc. Robot 2021, 1–18. doi:10.1007/s12369-021-00795-5
Keywords: healthcare janitorial services, autonomous cleaning robots, human-robot interaction, surveys and questionnaires, proxemic behaviors design
Citation: Chen Y, Luo Y and Hu B (2022) Towards Next Generation Cleaning Tools: Factors Affecting Cleaning Robot Usage and Proxemic Behaviors Design. Front. Electron. 3:895001. doi: 10.3389/felec.2022.895001
Received: 12 March 2022; Accepted: 04 April 2022;
Published: 29 April 2022.
Edited by:
Shuo Gao, Beihang University, ChinaCopyright © 2022 Chen, Luo and Hu. This is an open-access article distributed under the terms of the Creative Commons Attribution License (CC BY). The use, distribution or reproduction in other forums is permitted, provided the original author(s) and the copyright owner(s) are credited and that the original publication in this journal is cited, in accordance with accepted academic practice. No use, distribution or reproduction is permitted which does not comply with these terms.
*Correspondence: Boyi Hu, Ym95aWh1QGlzZS51ZmwuZWR1
Disclaimer: All claims expressed in this article are solely those of the authors and do not necessarily represent those of their affiliated organizations, or those of the publisher, the editors and the reviewers. Any product that may be evaluated in this article or claim that may be made by its manufacturer is not guaranteed or endorsed by the publisher.
Research integrity at Frontiers
Learn more about the work of our research integrity team to safeguard the quality of each article we publish.