- 1School of Environmental Science and Engineering, Liaoning Technical University, Fuxin, China
- 2School of Materials Science and Engineering, Liaoning Technical University, Fuxin, China
Neuromorphic computing is a promising paradigm for developing energy-efficient and high-performance artificial intelligence systems. The unique properties of lithium niobate-based (LiNbO3)-based memristors, such as low power consumption, non-volatility, and high-speed switching, make them ideal candidates for synaptic emulation in neuromorphic systems. This study investigates the potential of LiNbO3-based memristors to revolutionize neuromorphic computing by exploring their synaptic behavior and optimizing device parameters, as well as harnessing the potential of LiNbO3-based memristors to create efficient and high-performance neuromorphic computing systems. By realizing efficient and high-speed neural networks, this literature review aims to pave the way for innovative artificial intelligence systems capable of addressing complex real-world challenges. The results obtained from this investigation will be crucial for future researchers and engineers working on designing and implementing LiNbO3-based neuromorphic computing architectures.
Introduction
The quest for energy-efficient and high-performance computing systems has led to exploring novel materials for applications in emerging paradigms, such as neuromorphic computing. Neuromorphic computing (Marković et al., 2020), inspired by the remarkable efficiency of the human brain, has emerged as a promising alternative to traditional computing paradigms for building energy-efficient and high-performance artificial intelligence systems. This field focuses on developing brain-inspired architectures that mimic neural networks’ parallel and distributed processing capabilities. A crucial component of neuromorphic systems is the memristor (Volos et al., 2015), the fundamental building block that emulates synaptic behavior and enables efficient learning and adaptation in neural networks.
Among various materials investigated for memristor technology, LiNbO3-based memristors (Chua, 1971) have garnered significant attention due to their unique combination of properties. The ferroelectric nature, electro-optic properties, non-volatility, low power consumption, high-speed switching, and the capability for multi-level resistance states collectively position LiNbO3 as a multifaceted material for developing efficient and brain-inspired computing architectures. Accordingly, the exploration of LiNbO3 in neuromorphic computing stems from its potential to mimic synaptic behavior and facilitate energy-efficient computing processes (Saleh and Koldehofe, 2022; Xu et al., 2023).
The ferroelectric nature of LiNbO3
LiNbO3 is a ferroelectric material with multiple invaluable technological applications. Ferroelectricity in lithium niobate crystals can be attributed to face-sharing octahedra aligned along the crystal’s c-axis (Xue and Kitamura, 2003). In neuromorphic computing, a material’s ferroelectric nature becomes particularly intriguing due to its potential to mimic synaptic behavior—a key aspect of artificial neural networks inspired by the human brain (Suna et al., 2022; Wang et al., 2022; Xu et al., 2023). The study of LiNbO3 in neuromorphic computing is relevant in pursuing efficient and brain-like computing architectures. LiNbO3’s ferroelectricity property is crucial for emulating synaptic plasticity, allowing for the adjustment of synaptic weights in response to external stimuli (Wang et al., 2022; X; Pan et al., 2019; Wang et al., 2023a; Dongale et al., 2017). The reversible polarization of LiNbO3 aligns with the dynamic nature of synapses, providing a foundation for the material’s application in neuromorphic systems. The inherent ferroelectric nature of LiNbO3, characterized by spontaneous polarization (Birnie, 1990; Yoo et al., 2018), domain structures (McConville et al., 2020), and piezoelectricity (Vakulov et al., 2020; Chen et al., 2021a; Kislyuk et al., 2022), justifies its multifaceted utility. These articles serve as key references in comprehending the fundamental aspects of LiNbO3’s ferroelectric nature.
The spontaneous electric polarization that LiNbO3 exhibits can be reversed by applying an external electric field (Roshchupkin et al., 2009; Stone et al., 2011; Tayi et al., 2012). Besides, LiNbO3 crystallizes in the trigonal structure belonging to the R3c space group (Inbar and Cohen, 1997; Sánchez-Dena et al., 2020; Chen et al., 2021b; Palatnikov et al., 2023). The crystal structure comprises corner-sharing NbO6 octahedra and Li ions occupying interstitial sites (Nico et al., 2016). The non-centrosymmetric structure also contributes to the material’s ferroelectric properties (Sánchez-Dena et al., 2020). Additionally, LiNbO3 undergoes a ferroelectric phase transition at a high Curie temperature (TC) of between 1,141 and 1,210°C (McConville et al., 2020), where ferroelectric properties, including the spontaneous polarization, disappears (Voskresenskii et al., 2017; Lin et al., 2018; Chen et al., 2021a). Figure 1 illustrates the crystalline structure of LiNbO3 exhibiting ferroelectric properties with a stoichiometric composition that remains stable for temperatures below the curie temperature. The Curie temperature shifts depending on the chemical composition. The basic net consists of six evenly spaced layers of oxygen per unit length, arranged along the polar axis c.
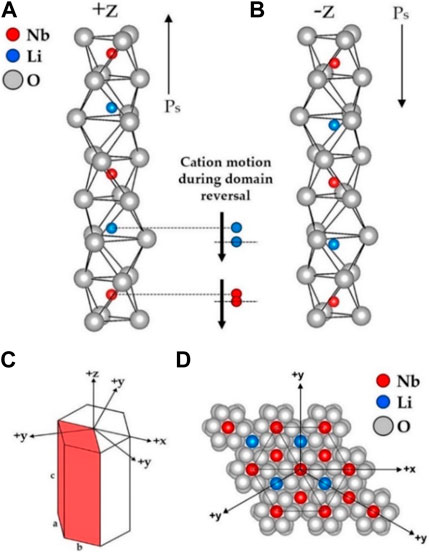
FIGURE 1. The crystal structure of ferroelectric LiNbO3 shows two potential cation displacement scenarios relative to each equidistant oxygen octahedron. In (A), the orientation shows Z+ cation displacement, while in (B), it illustrates Z-cation displacement. (C) exhibits the conventional choice of axes for the “ortho-hexagonal” setting (XYZ) about the hexagonal setting (a, c). Note that all axes possess equal lengths in the hexagonal setting, whereas in the Cartesian setting, they differ. (D) presents a projection of the atomic arrangement along the c-axis (Sánchez-Dena et al., 2020).
LiNbO3 has advantageous ferroelectric properties over other perovskite materials, making it a prominent candidate for various neuromorphic applications. Its high Tc ensures stability over a wider range of operating conditions than its counterparts, including lead zirconate titanate (PZT) (Hwang et al., 1998; Wolf and Trolier-McKinstry, 2004), barium strontium titanate (BST) (Ioachim et al., 2007; Gatea and Naji, 2020), strontium titanate (SrTiO3) (Li et al., 2004; Dec et al., 2005; Gatea and Naji, 2020), barium titanate (BaTiO3) (Sakayori et al., 1995; Chlup et al., 2023), and lead magnesium niobate-lead titanate (PMN-PT) (Ivan et al., 2012), which have much lower Curie temperatures. Below TC, LiNbO3 exhibits ferroelectric properties, and above it, the material becomes paraelectric. Multiple studies have also shown LiNO3’s sensitivity to the chemical composition (CC) on the whole range of the LiNbO3’s single phase, as shown in Figure 2.
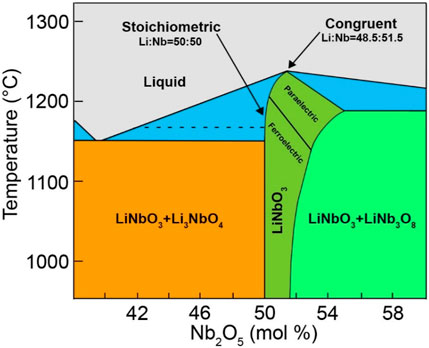
FIGURE 2. Schematic phase diagram of the Li2O—Nb2O5 pseudo-binary system near the congruent and stoichiometric composition of LiNbO3 (Narayanareddy, 2008; Sánchez-Dena et al., 2020; Chen et al., 2021b).
The binary phase diagram in Figure 2 shows the wide solid solution range exhibited by LiNbO3. This solution can be stable on lithium composition between 46.5 and 50 mol%. The diffuse peak at around 48.5% Li2O is evident in the liquid–solid curve, beyond which the formation of secondary phases such as LiNb3O8 and Li3NbO4 can occur (Chen et al., 2021a).
The ferroelectric property makes lithium niobate have significant applications in various fields, including optics, acoustics, and information processing (Edon et al., 2009; Wu et al., 2020; Chen et al., 2021b; Lin et al., 2022). For instance, LiNbO3 is applied in polarization management due to high fidelity polarization generation with an extinction ratio that can exceed 41.9 dB and a high polarization scrambling rate of over 64 Mrad s−1 (Lin et al., 2022; Hou et al., 2023).
Piezoelectric phenomena in LiNbO3
While investigating the piezoelectric nature of LiNbO3, studies have offered a detailed exploration of the material’s response to mechanical stress (Du et al., 2007; Zhang et al., 2019). LiNbO3’s non-centrosymmetric crystal structure imparts unique electrical properties upon applying mechanical stress. Research has elucidated the crystal’s anisotropic nature, emphasizing its sensitivity to different stress directions (Weis and Gaylord, 1985; Rivera et al., 2011; Gruber et al., 2018). Accordingly, studies underscore the intricate relationship between ferroelectricity and piezoelectricity in LiNbO3, showcasing its potential for applications in sensors, actuators, and other electromechanical devices (Chen et al., 2021a) LiNbO3 also exhibits strong electro-optic effects (Meng et al., 2007) Accordingly, it is extensively used in the field of telecommunications for the development of modulators since the electro-optic effect in LiNbO3 enables the modulation of light signals, which is crucial in devices like electro-optic modulators used in fiber-optic communication systems. Besides, LiNbO3 offers superior piezoelectric coefficients and mechanical stability compared to other perovskite materials, such as PZT, BST, SrTiO3, BaTiO3, and PMN-PT (Sumets, 2018). These properties make LiNbO3 ideal for use in ultrasonic transducers, acoustic wave devices, and actuators, where high sensitivity and durability are paramount. As a material with a rich tapestry of properties, LiNbO3 continues to captivate researchers, laying the groundwork for innovations across diverse scientific disciplines, including neuromorphic computing.
Non-volatility and low power consumption
Although lithium niobate is not typically associated with memory retention in the same way as traditional electronic materials used in computer memory, such as silicon-based technologies, it has been explored for multiple applications, including non-volatile memory and optical memory devices. The non-volatile characteristics of LiNbO3 play a pivotal role in reducing the power requirements for maintaining synaptic weights. One of the defining features that positions LiNbO3 as a frontrunner in neuromorphic computing is its non-volatile nature, which allows for the retention of synaptic weights even when power is removed. Xu et al. (2023) and Wang et al. (2015) discuss how this characteristic allows for the retention of synaptic weights even in the absence of power, mimicking the persistent connectivity of biological synapses. Besides, Wang et al. (2017) show that the ferroelectric property of LiNbO3 enables the retention of synaptic weights even when power is removed. This persistence aligns with the essence of non-volatile memory, mirroring the sustained connectivity observed in biological synapses. The non-volatile aspect is fundamental for the stability and integrity of neural networks.
In Figure 3A left, a LiNbO3 device retention test was conducted as the device was set to RMIN and was measured at regular intervals within 24 h. Data reveals negligible variation in the programed device state when measured at room temperature over that period. Figure 3A right shows the results of a retention test on a LiNbO3 device at different temperatures, considering a programmed intermediate state at approximately 1 mA. The tests were also performed on the LiNbO3 device at temperatures between 20 and 100°C (Wang et al., 2017). Results showed negligible current change as device temperatures are increased. Likewise, Figure 3E illustrates how LiNbO3 device demonstrates outstanding data retention performance over 27.7 h at room temperature. Additionally, Figure 3F shows conductance undergoes only a 0.74% decay process after approximately 16.7 h from programming the device to a high conductance state, as it remains stable without degradation. As a result, Wang et al. (2023c) speculates that the device retention lifetime at room temperature can be up to 10 years.
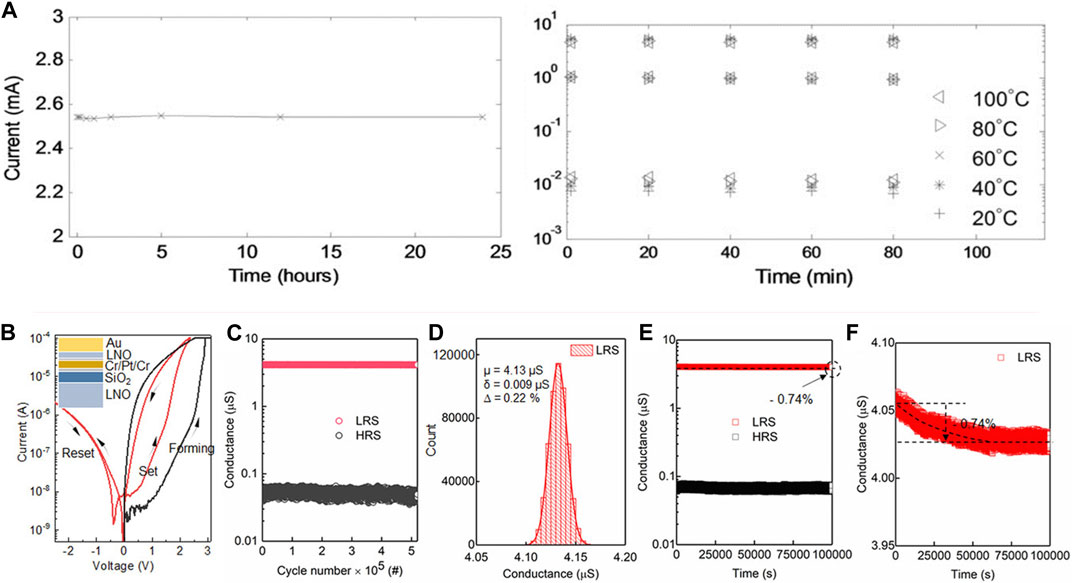
FIGURE 3. (A) Shows a retention test to prove the non-volatility characteristics of LiNbO3 memristor (Wang et al., 2017). (B) Shows the electroforming process and resulting I–V curve are typical, with a cross-sectional diagram of the memristor showing its structure as Au/LNO/Cr/Pt/Cr. In (C), device endurance is demonstrated over 5 × 105 cycles with a switching window of 80, indicating robust performance. (D) Reveals detailed statistical analysis that the device effectively switches with minimal fluctuations, as evidenced by the Normal distribution function fitting of the low-resistance state (LRS). Just as in (A), (E) reveals that retention testing over a prolonged period of 105 s shows stable behavior. (F) Detailed analysis of LRS retention over the same period indicates minimal conductance decay, with stability maintained for approximately 16.7 h after programming the device to a high-conductance state (LRS) (Wang et al., 2023b).
LiNbO3-based memristors also exhibit low power consumption, addressing the demand for energy-efficient computing systems, as revealed by Zaman et al. (2017), Xu et al. (2023), Wang et al. (2015), and Nakajima et al. (2022). Examining the energy efficiency of LiNbO3, Zaman (2020) discusses how the material’s non-volatility contributes to low power consumption during synaptic emulation. Besides, research emphasizes that the inherent properties of LiNbO3 allow for efficient information storage and retrieval, facilitating energy-conscious neuromorphic operations (Chaudhary et al., 2020). This breakthrough aligns with the broader goal of creating sustainable and environmentally friendly computing architectures. Through advanced fabrication techniques, studies demonstrate that LiNbO3 memristors can achieve efficient and stable operation with minimal power requirements (Zaman et al., 2019; Huang et al., 2021; Liang et al., 2021). For instance, lithium niobate’s non-volatility, coupled with its minimal power requirements during read and write operations and low operating voltages as shown to be ∼1.20 V at room temperature and ∼0.95 V at 95°C for a bilayer LiNbO3-based memristor device, makes LiNbO3-based memristors competitive against alternatives such as SrTiO3 and BaTiO3 (Zaman et al., 2020). Such experimental validations reinforce the theoretical understanding of LiNbO3 as a material capable of supporting low-power neuromorphic computations.
In the pursuit of prolonged neural network operations (Wang et al., 2023b), shed light on LiNbO3’s role in enabling persistent synaptic connections. The non-volatile property of LiNbO3 ensures that the information encoded in synaptic weights remains intact over extended periods. This feature is particularly crucial for applications requiring continuous learning and adaptation, positioning LiNbO3 as a material with profound implications for the longevity of neuromorphic systems. As this field advances, integrating LiNbO3 into neuromorphic architectures holds promise for creating sustainable, high-performance artificial intelligence systems. Likewise, non-volatile memory is crucial for retaining information even when power is disconnected, offering energy efficiency and data persistence advantages. Sun et al. (2023) explore the implications of LiNbO3’s ferroelectricity in non-volatile memory applications. The study investigates ferroelectric field-effect transistors based on LiNbO3, highlighting its potential for high-density memory storage. This work underlines the relevance of LiNbO3’s ferroelectric nature in advancing data storage technologies. Indeed, LiNbO3-based memristors exhibit low power consumption and efficient memory retention, crucial for extending the operational life of neural network systems, especially in scenarios where energy conservation is imperative.
High-speed switching and synaptic plasticity
High-speed switching and synaptic plasticity support neuromorphic computing systems’ dynamic learning and adaptation processes. Multiple studies collectively reveal the important role of LiNbO3 in enabling high-speed switching and synaptic plasticity within neuromorphic computing. LiNbO3’s high-speed switching capability is critical for emulating synaptic plasticity in neuromorphic systems. For instance, Figure 3B illustrates the typical electroforming process and successive I–V sweeps for the LNO-based devices. Following electroforming, the device exhibits analog switching behavior. According to J. Wang et al. (2023c), Ar+ beam irradiation allows the device to acquire switching characteristics and significantly reduces the operating voltage. Figure 3C shows the endurance property of the LiNbO3 memristor, demonstrating reliable endurance characteristics. More detailed cyclic switching analyses are depicted in Figure 3D in which the cyclic conductance states show that the device effectively switches in every cycle without becoming stuck at any state. Indeed, memristors based on LiNbO3 have demonstrated rapid switching speeds, enabling efficient adaptation to changing neural network conditions (Yakopcic et al., 2017; Eid et al., 2020; Wang et al., 2023a). Emulating synaptic plasticity is crucial for learning and memory functions in artificial neural networks. Yakopcic et al. (2017) elaborate on the significance of rapid switching in neuromorphic computing, highlighting how it facilitates the swift adaptation of synaptic weights—a fundamental requirement for learning and memory functions in artificial neural networks.
Research has also focused on realizing synaptic plasticity in LiNbO3-based memristors. For instance, Wang et al. (2022) and Liang et al. (2021) demonstrate the material’s efficacy in emulating the plastic nature of biological synapses, demonstrating the potential for long-term potentiation and depression. This plasticity is vital for encoding and retaining information adaptively, mirroring the cognitive capabilities of the human brain. Additionally, Tong et al. (2021) explore the intersection of optoelectronics and synaptic behavior in LiNbO3, implying that optoelectronic modulation can enhance the synaptic plasticity of LiNbO3-based devices. The ability to manipulate synaptic weights through light-induced processes opens avenues for innovative neuromorphic computing architectures, allowing for dynamic and flexible adaptation under varying conditions. Some studies are based on non-volatile synaptic characteristics and high-precision memory recognition based on lithium niobate. For instance (Wang et al., 2023b), emphasize the role of LiNbO3 in achieving stable and precise synaptic weight updates. The high-speed switching dynamics of LiNbO3 contribute to the efficiency of weight update operations, a crucial element for the overall performance of neuromorphic systems.
Multi-level resistance states and weighted synapses
LiNbO3 memristors play a significant role in achieving multi-level resistance states and weighted synapses within neuromorphic computing. LiNbO3-based memristors exhibit multi-level resistance states, enabling the implementation of weighted synapses. This aspect, discussed by (Zaman et al., 2017), is pivotal for the precision and flexibility of synaptic emulation. The ability to represent the strength of connections between artificial neurons with high granularity enhances neuromorphic systems’ computational power and accuracy. LiNbO3-based memristors exhibit multi-level resistance states, providing the opportunity for implementing weighted synapses. This feature is essential for accurately representing the strength of connections between artificial neurons in a neuromorphic system (Zaman et al., 2017; Zaman, 2020). Multi-level resistance states contribute to the precision and versatility of synaptic emulation.
Figure 4A shows experimental findings concerning the stability challenges influencing the non-volatile LiNbO3 circuit element at room temperature. The plot in Figure 4A illustrates a steady rise in resistance levels and the stability of the state for voltage pulses at 0.1, 0.4, and 0.7 V. Stable resistance states between the high and low resistance states of the Ti/LiNbO3/Pt MIM structure were successfully attained for the three distinct pulse voltages. Besides, Figure 4B confirms the LiNbO3 memristor’s high reliability across multiple conductance levels (16 distinguishable states) for weight updating in artificial neural networks (Wang et al., 2023b).
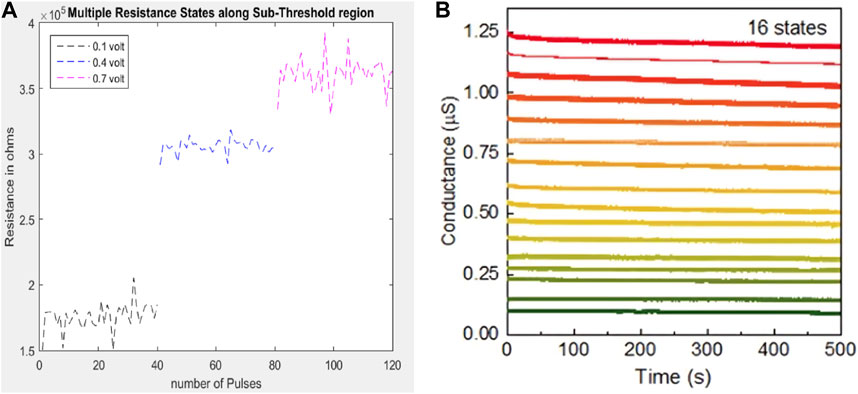
FIGURE 4. (A) Multiple resistance levels along the sub-threshold region within a 7.5 um2 memristor device at room temperature (Zaman et al., 2017). (B) Retention performance of 4-bit distinguishable intermediate states of a LiNbO3 memristor (Wang et al., 2023a).
Zaman (2020) and Morozov et al. (2022) delve into the precision of synaptic weight representation achievable with LiNbO3-based memristors. These studies imply how the multi-level resistance states of LiNbO3 allow for a fine-grained and continuous adjustment of synaptic weights. This precision is essential for accurately mimicking the diverse strengths of synaptic connections observed in biological neural networks. Zaman (2020) also contributes to the discourse by showcasing the versatility of LiNbO3-based memristors in creating weighted synapses for various neuromorphic tasks, emphasizing the precision of LiNbO3 in representing synaptic weights and demonstrating its applicability across a spectrum of neuromorphic computing applications. This versatility positions LiNbO3 as a material capable of meeting the diverse requirements of artificial neural networks.
Zaman et al. (2020) provide experimental evidence supporting the feasibility of achieving weighted synapses with LiNbO3-based memristors. Experimental studies also reveal how LiNbO3’s inherent properties, when harnessed through advanced fabrication techniques, enable the reliable and reproducible creation of memristors with multiple resistance states (Wang et al., 2017; Zaman et al., 2019). These experimental validations reinforce the theoretical foundation for LiNbO3 as a material for implementing weighted synapses. Besides, Wang et al. (2017) explore the role of weighted synapses in enabling dynamic adaptation within neuromorphic systems. Their research demonstrates how the precise adjustment of synaptic weights, facilitated by LiNbO3’s multi-level resistance states, contributes to the efficient emulation of cognitive functions such as pattern recognition and deep learning capabilities. This dynamic adaptation is a hallmark of intelligent systems inspired by biological neural networks.
Expanding on the scalability (Saleh and Koldehofe, 2022) of LiNbO3-based memristors, Pan et al. (2019) and Wang et al. (2017) investigate how the material can be integrated into large-scale neuromorphic architectures. These studies emphasize that LiNbO3’s multi-level resistance states and compatibility with advanced fabrication techniques make it a promising candidate for building complex neural networks. This scalability is crucial for realizing sophisticated neuromorphic systems that can tackle increasingly complex cognitive tasks. As this field progresses, the integration of LiNbO3 into neuromorphic architectures holds the promise of creating highly adaptive, precise, and efficient artificial intelligence systems.
Electro-optic and nonlinear optical properties
LiNbO3’s electro-optic and nonlinear optical properties add a layer of versatility to its utility in neuromorphic computing. Studies have extensively explored these properties, highlighting how LiNbO3 facilitates various nonlinear optical processes, including frequency conversion and generation of new wavelengths through processes like second harmonic generation. Abdul-Hussein and Almusawe (2023) emphasize how electro-optic and nonlinear optical properties can be harnessed to create efficient optoelectronic synapses, paving the way for innovative neuromorphic devices. They further show that the effect of doping by Mo improves optical properties, such as the material’s absorption spectrum. It also increases the effect of second harmonic generation, a fundamental prerequisite for designing optical devices in many photonic applications (Cabuk, 2012; Abdul-Hussein and Almusawe, 2023). Doping with MgO can also enhance the electro-optic coefficient (Kang et al., 2006). Combining electro-optic effects and nonlinear responses in LiNbO3 expands the possibilities for developing advanced neuromorphic architectures.
Foundational works by Weigand et al. (2021), Chen et al. (2021b), and Xu et al. (2022) delve into the electro-optic effect in LiNbO3, emphasizing its role in light modulation. This property allows precise refractive index control response to an applied electric field, making LiNbO3 a cornerstone in developing electro-optic modulators for optical communication systems (Weiss et al., 2022). Additionally, experimental studies have expanded the discourse by exploring electro-optic tuning in resonant photonic devices. Research demonstrates how LiNbO3’s electro-optic properties can be harnessed to dynamically tune the resonance in micro-resonators and photonic circuits, offering a pathway toward reconfigurable and adaptive optical systems (Witmer et al., 2017; Boes et al., 2018; Lin et al., 2020). Periodically poled lithium niobate (PPLN) photonic wires have also shown potential for developing a wide range of nonlinear wavelength converters and devices for all-optical signal processing (Hu et al., 2009; Poberaj et al., 2012).
Eid et al. (2020), Pan et al. (2021), and Minakata (2001) discuss the applications of LiNbO3 high-speed modulators in optical communication systems, highlighting the intrinsic advantages of LiNbO3 in achieving gigahertz-speed modulation, a crucial factor in the development of high-performance optical communication networks. Zheng and Chen (2021) shift focus to parametric processes enabled by LiNbO3, particularly four-wave mixing. The nonlinear response of LiNbO3 is exploited for generating new frequencies through the interaction of intense optical waves, a phenomenon crucial for applications in wavelength conversion and signal processing (Liu et al., 2019). Likewise, Zeng et al. (2008), Lefort et al. (1999), Bache and Wise (2010), Hu et al. (2012), and Yu et al. (2022) investigate the ultrafast nonlinear optical properties of LiNbO3. These studies highlight the potential of LiNbO3 in ultrafast pulse compression, an essential capability for applications in telecommunications, and the generation of ultrashort laser pulses. Sang et al. (2006), Zhao et al. (2020), Jin et al. (2014), and Suhara (2009) delve into the potential of LiNbO3 in generating entangled photon pairs through nonlinear processes, showcasing its relevance in the burgeoning field of quantum optics. Finally, Wei et al. (2018) and Sun et al. (2020) demonstrate how integrating LiNbO3 into photonic crystal structures enhances nonlinearities, paving the way for compact and efficient nonlinear photonic devices. Collectively synthesizing multiple findings reveals LiNbO3’s richness in electro-optic and nonlinear optical properties. From high-speed modulators to quantum information processing, LiNbO3’s diverse capabilities in photonics make it an important material in advancing optical communication and signal processing technologies.
Volatile properties of LiNbO3 memristors
Dynamic memristors based on LiNbO3 exhibit unique volatile properties, primarily attributed to its nonlinear I-V (current-voltage) characteristics. As shown in Figure 5, LiNbO3 device exhibits bi-directional switching between a HRS and a LRS when the voltage surpasses specific threshold values of either polarity. Once the voltage intensity decreases below a certain hold value, spontaneous transition from LRS to HRS occurs, indicating the device’s volatile memristive behavior. Besides, its I-V characteristics display nonlinearity, regardless of varying sizes, signifying volatile switching across all lithium niobate devices. Despite size differences, all devices switch to LRS at approximately 2 V. Additionally, Figure 5F illustrates the dependency of LRS and HRS currents on device size, where HRS current escalates with device size while LRS current remains relatively constant. This suggests a filamentary nature in volatile switching. The I-V curves in Figure 5F for a specific device size under positive voltage sweepings with different stop voltages reveal an increase in LRS current with higher stop voltages, indicating thicker conducting filament formation under stronger voltages. The nonlinearity in the I-V curve enables the memristor to have short-term memory, a characteristic that sets it apart from traditional resistive switching devices (Zhao et al., 2023).
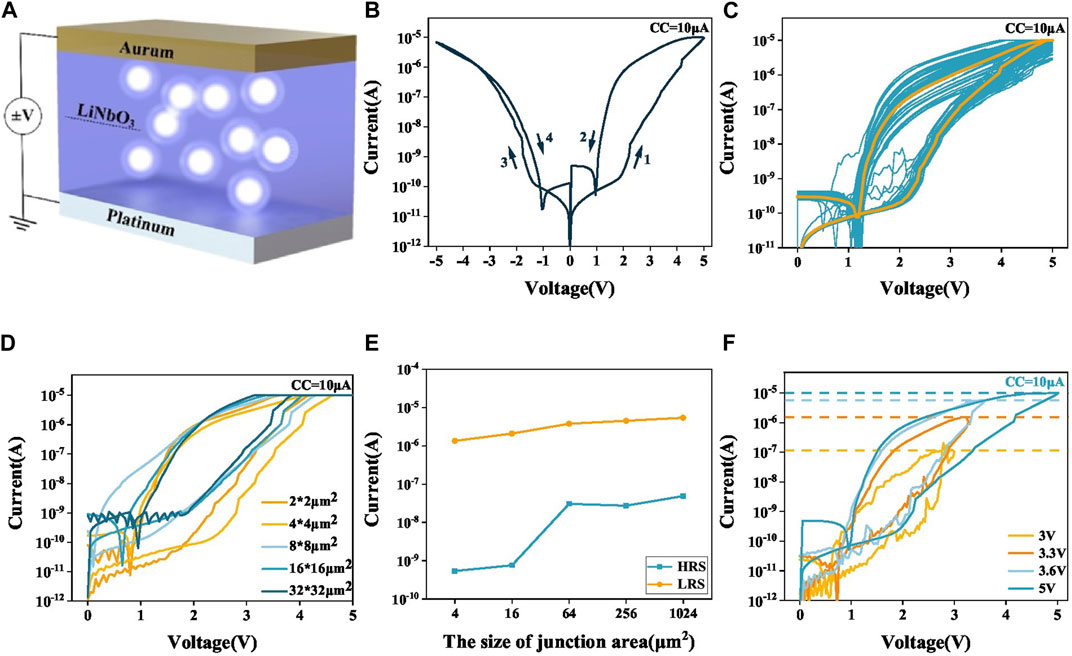
FIGURE 5. (A) shows the schematic structure of the Pt/LiNbO3/Au memristor, while (B) illustrates the typical I-V characteristics of the lithium niobate memristor. (C) displays the results of a fifty-cycle endurance test conducted on the lithium niobate memristor. (D) presents the I-V characteristics of five lithium niobate memristors of varying sizes. (E) shows the currents flowing through five lithium niobate memristors of different sizes in both their low resistance states and high resistance states. (F) exhibits the I-V characteristics obtained by altering the stop voltage during the sweeping process (Zhao et al., 2023).
The volatile property stems from the material’s ability to undergo reversible changes in resistance, enabling swift information storage and retrieval within short time frames (Hu et al., 2021). This short-term memory effect indicates that the device’s resistance state can be altered temporarily, making it suitable for applications where dynamic or volatile memory is desirable, including reservoir computing and domain wall random access memory (Hu et al., 2021; Wang et al., 2023a; Zhao et al., 2023). Hence, the short-term memory capabilities of lithium niobate memristors make these devices suitable for neuromorphic computing.
Compatibility with advanced fabrication techniques
The seamless integration of novel materials into neuromorphic devices necessitates compatibility with advanced fabrication techniques. LiNbO3 aligns with this requirement, enabling precise manufacturing processes and contributing to developing high-performance neuromorphic devices. Advancements in fabrication techniques have facilitated the integration of LiNbO3 into memristor arrays. The compatibility of LiNbO3 with various fabrication methods, such as chemical vapor deposition and sputtering (Marković et al., 2020; Liang et al., 2021; Wang et al., 2023a) ensures scalable and reproducible production of memristor devices for potential large-scale neuromorphic computing applications (Bornand et al., 2003; Uchino, 2017). Additionally, the advancements in lithography and etching techniques directly influence the properties of the underlying LNOI material. Lin et al. (2020) and Qi and Li (2020) provide insights into the compatibility of LiNbO3 with photonic lithography techniques. Likewise, the focused ion beam (FIB) lithography technique has great potential to precisely, etch LiNbO3 thin films (Chen et al., 2020; Jia et al., 2021; Leng et al., 2024). Such studies demonstrate that LiNbO3-based structures can be precisely patterned at the submicron scale, which can allow for the creation of intricate neuromorphic circuits. This compatibility with advanced fabrication techniques opens avenues for the development of densely packed and intricately designed neural networks, crucial for enhancing computational efficiency.
Besides, integrating electro-optic devices on lithium-niobate-on-insulator (LNOI) holds promise for enhancing the functionality and performance of memristors in neuromorphic systems. LNOI has an edge over indium phosphorus, silicon, silicon nitride, and silicon oxide materials due to multiple features such as intrinsic electro-optical effects, which makes LNOI’s optical switching speed faster with lower power consumption than its counterparts that depend on thermal-optical effects to attain the modulation of optical phase (Hu et al., 2012; Yuan et al., 2021). Likewise, LNOI combines the exceptional electro-optic properties of lithium niobate with the advantages of an insulating layer, enhancing the performance of photonic devices. Boes et al. (2018), Lin et al. (2020), and Saravi et al. (2021) explore the fabrication technology and applications of photonic structures on LNOI. Incorporating photonic structures on LNOI platforms thus opens avenues for developing compact, energy-efficient, and high-performance photonic devices with implications for telecommunications, sensing, and quantum technologies. Likewise, Lewis et al. (2012) shed light on using nanoimprint lithography for LiNbO3-based devices. Nanoimprint lithography, known for its high-throughput capabilities, is compatible with LiNbO3, allowing for the rapid and cost-effective fabrication of neuromorphic components. This compatibility is pivotal for the large-scale manufacturing of neuromorphic devices, a crucial step towards the practical implementation of LiNbO3 in neuromorphic computing and artificial intelligence systems.
Studies have demonstrated the compatibility of LiNbO3 with advanced fabrication techniques. This adaptability positions LiNbO3 as a versatile material that can be seamlessly integrated into the rapidly evolving landscape of neuromorphic device manufacturing, presenting a potential for developing precise, scalable, and multifunctional artificial intelligence systems. The intricate interplay between advanced fabrication methods and the unique characteristics of LiNbO3 memristor devices opens avenues for tailoring neuromorphic devices with unprecedented precision, paving the way for their seamless integration into the rapidly evolving landscape of artificial intelligence and cognitive computing.
Conclusion
The significance of lithium niobate memristors in neuromorphic computing and its potential impact on artificial intelligence applications cannot be ignored. The literature reviewed reveals the unique properties of LiNbO3 that make it an excellent candidate for neuromorphic computing. Its unique set of electro-optic and nonlinear optical properties, non-volatile nature, low power consumption, high-speed switching, multi-level resistance states, I-V volatile properties, and compatibility with advanced fabrication techniques position LiNbO3 as a versatile material for efficient and high-performance memristor-based neuromorphic systems. As the field advances, integrating LiNbO3 into neuromorphic architectures holds immense promise for realizing dynamic, responsive, and efficient artificial intelligence systems.
Author contributions
CK: Resources, Writing–original draft, Writing–review and editing. YL: Resources, Supervision, Writing–review and editing, Conceptualization.
Funding
The author(s) declare that no financial support was received for the research, authorship, and/or publication of this article.
Acknowledgments
The authors would like to thank postgraduate students and academics at Liaoning Technical University, especially Polycarp Shizawaliyi Yakoi, who assisted during the article’s editing process. The authors wish to thank the editor and reviewers for their valuable time in reviewing the manuscript.
Conflict of interest
The authors declare that the research was conducted in the absence of any commercial or financial relationships that could be construed as a potential conflict of interest.
Publisher’s note
All claims expressed in this article are solely those of the authors and do not necessarily represent those of their affiliated organizations, or those of the publisher, the editors and the reviewers. Any product that may be evaluated in this article, or claim that may be made by its manufacturer, is not guaranteed or endorsed by the publisher.
References
Abdul-Hussein, W. A., and Almusawe, A. J. (2023). Structural, electronic, linear, and nonlinear optical properties of undoped and Mo (I, II)-doped LiNbO3 crystal. Tech. Romanian J. Appl. Sci. Technol. 13, 88–101. doi:10.47577/technium.v13i.9623
Bache, M., and Wise, F. W. (2010). Type-I cascaded quadratic soliton compression in lithium niobate: compressing femtosecond pulses from high-power fiber lasers. Phys. Rev. A - Atomic, Mol. Opt. Phys. 81 (5), 053815. doi:10.1103/PhysRevA.81.053815
Birnie, D. P. (1990). The spontaneous polarization as evidence for lithium disordering in LiNbO 3. J. Mater. Res. 5 (9), 1933–1939. doi:10.1557/JMR.1990.1933
Boes, A., Corcoran, B., Chang, L., Bowers, J., and Mitchell, A. (2018). Status and potential of lithium niobate on insulator (LNOI) for photonic integrated circuits. Laser Photonics Rev. 12 (4). doi:10.1002/lpor.201700256
Bornand, V., Huet, I., and Papet, P. (2003). LiNbO3 thin films deposited on Si substrates: a morphological development study. Mater. Chem. Phys. 77 (2), 571–577. doi:10.1016/S0254-0584(02)00107-4
Cabuk, S. (2012). The nonlinear optical susceptibility and electro-optic tensor of ferroelectrics: first-principle study. Central Eur. J. Phys. 10 (1), 239–252. doi:10.2478/s11534-011-0079-3
Chaudhary, P., Lu, H., Lipatov, A., Ahmadi, Z., McConville, J. P. V., Sokolov, A., et al. (2020). Low-voltage domain-wall LiNbO3Memristors. Nano Lett. 20 (8), 5873–5878. doi:10.1021/acs.nanolett.0c01836
Chen, F., Amekura, H., and Jia, Y. (2020). Photonic structures based on thin films produced by ion beams. Berlin, Germany: Springer, 45–60. doi:10.1007/978-981-15-4607-5_3
Chen, G., Lin, H.-L., Ng, J. D., and Danner, A. J. (2021a). Integrated electro-optic modulator in Z-cut lithium niobate thin film with vertical structure. IEEE Photonics Technol. Lett. 33 (23), 1285–1288. doi:10.1109/LPT.2021.3114993
Chen, K., Zhu, Y., Liu, Z., and Xue, D. (2021b). State of the art in crystallization of linbo3 and their applications. Molecules 26 (22), 7044. doi:10.3390/molecules26227044
Chlup, Z., Drdlík, D., Hadraba, H., Ševeček, O., Šiška, F., Erhart, J., et al. (2023). Temperature effect on elastic and fracture behaviour of lead-free piezoceramic BaTiO3. J. Eur. Ceram. Soc. 43 (4), 1509–1522. doi:10.1016/j.jeurceramsoc.2022.11.030
Chua, L. (1971). Memristor-The missing circuit element. IEEE Trans. Circuit Theory 18 (5), 507–519. doi:10.1109/TCT.1971.1083337
Dec, J., Kleemann, W., Boldyreva, K., and Itoh, M. (2005). Unique features of strontium titanate. Ferroelectrics 314 (1), 7–18. doi:10.1080/00150190590926003
Dongale, T. D., Mohite, S. V., Bagade, A. A., Kamat, R. K., and Rajpure, K. Y. (2017). Bio-mimicking the synaptic weights, analog memory, and forgetting effect using spray deposited WO 3 memristor device. Microelectron. Eng. 183–184, 12–18. doi:10.1016/j.mee.2017.10.003
Du, H., Tang, F., Liu, D., Zhu, D., Zhou, W., and Qu, S. (2007). The microstructure and ferroelectric properties of (K0.5Na0.5)NbO3–LiNbO3 lead-free piezoelectric ceramics. Mater. Sci. Eng. B 136 (2–3), 165–169. doi:10.1016/j.mseb.2006.09.031
Edon, V., Rèmiens, D., and Saada, S. (2009). Structural, electrical and piezoelectric properties of LiNbO3 thin films for surface acoustic wave resonators applications. Appl. Surf. Sci. 256 (5), 1455–1460. doi:10.1016/j.apsusc.2009.09.002
Eid, M. M. A., Rashed, A. N. Z., and Amiri, I. S. (2020). Fast speed switching response and high modulation signal processing bandwidth through LiNbO3electro-optic modulators. J. Opt. Commun. 0. doi:10.1515/joc-2020-0012
Gatea, H. A., and Naji, I. S. (2020). The effect of Ba/Sr ratio on the Curie temperature for ferroelectric barium strontium titanate ceramics. J. Adv. Dielectr. 10 (05), 2050021. doi:10.1142/S2010135X20500216
Gruber, M., Konetschnik, R., Popov, M., Spitaler, J., Supancic, P., Kiener, D., et al. (2018). Atomistic origins of the differences in anisotropic fracture behaviour of LiTaO3 and LiNbO3 single crystals. Acta Mater. 150, 373–380. doi:10.1016/j.actamat.2018.03.020
Hou, S., Chen, P., Shah, M., Briggs, I., Xing, W., Liu, Z., et al. (2023). Programmable optical filter in thin-film lithium niobate with simultaneous tunability of extinction ratio and wavelength. ACS Photonics 10 (11), 3896–3900. doi:10.1021/acsphotonics.3c00574
Hu, H., Ricken, R., and Sohler, W. (2009). Lithium niobate photonic wires. Opt. Express 17 (26), 24261. doi:10.1364/OE.17.024261
Hu, H., Yang, J., Gui, L., and Sohler, W. (2012). Lithium niobate-on-insulator (LNOI): status and perspectives. Proc. SPIE - Int. Soc. Opt. Eng. 84311. doi:10.1117/12.922401
Hu, X., Hou, X., Zhang, Y., Chai, X., Lian, J., Wang, C., et al. (2021). Size-controlled polarization retention and wall current in lithium niobate single-crystal memories. ACS Appl. Mater. Interfaces 13 (14), 16641–16649. doi:10.1021/acsami.0c22969
Huang, S., Luo, W., Pan, X., Zhao, J., Qiao, S., Shuai, Y., et al. (2021). Resistive switching effects of crystal-ion-slicing fabricated LiNbO 3 single crystalline thin film on flexible polyimide substrate. Adv. Electron. Mater. 7 (9). doi:10.1002/aelm.202100301
Hwang, H. J., Nagai, T., Ohji, T., Sando, M., Toriyama, M., and Niihara, K. (1998). Curie temperature anomaly in lead zirconate titanate/silver composites. J. Am. Ceram. Soc. 81 (3), 709–712. doi:10.1111/j.1151-2916.1998.tb02394.x
Inbar, I., and Cohen, R. E. (1997). Origin of ferroelectricity in LiNbO3 and LiTaO3. Ferroelectrics 194 (1–4), 83–95. doi:10.1080/00150199708016084
Ioachim, A., Toacsan, M. I., Banciu, M. G., Nedelcu, L., Dutu, A., Antohe, S., et al. (2007). Transitions of barium strontium titanate ferroelectric ceramics for different strontium content. Thin Solid Films 515 (16), 6289–6293. doi:10.1016/j.tsf.2006.11.097
Ivan, I. A., Agnus, J., and Lambert, P. (2012). PMN–PT (lead magnesium niobate–lead titanate) piezoelectric material micromachining by excimer laser ablation and dry etching (DRIE). Sensors Actuators A Phys. 177, 37–47. doi:10.1016/j.sna.2011.09.015
Jia, Y., Wang, L., and Chen, F. (2021). Ion-cut lithium niobate on insulator technology: recent advances and perspectives. Appl. Phys. Rev. 8 (1). doi:10.1063/5.0037771
Jin, H., Liu, F. M., Xu, P., Xia, J. L., Zhong, M. L., Yuan, Y., et al. (2014). On-Chip generation and manipulation of entangled photons based on reconfigurable lithium-niobate waveguide circuits. Phys. Rev. Lett. 113 (10), 103601. doi:10.1103/PhysRevLett.113.103601
Kang, B., Rhee, B. K., and Joo, G. T. (2006). Variation of electro-optic coefficients in MgO-doped LiNbO3 single crystals. Mater. Lett. 60 (17–18), 2306–2308. doi:10.1016/j.matlet.2005.12.144
Kislyuk, A. M., Ilina, T. S., Kubasov, I. V., Kiselev, D. A., Temirov, A. A., Turutin, A. V., et al. (2022). Degradation of the electrical conductivity of charged domain walls in reduced lithium niobate crystals. Mod. Electron. Mater. 8 (1), 15–22. doi:10.3897/j.moem.8.1.85251
Lefort, L., Puech, K., Butterworth, S. D., Svirko, Y. P., and Hanna, D. C. (1999). Generation of femtosecond pulses from order-of-magnitude pulse compression in a synchronously pumped optical parametric oscillator based on periodically poled lithium niobate. Opt. Lett. 24 (1), 28. doi:10.1364/ol.24.000028
Leng, B., Zhang, Y., Tsai, D. P., and Xiao, S. (2024). Meta-device: advanced manufacturing. Light Adv. Manuf. 5 (1), 1. doi:10.37188/lam.2024.005
Lewis, R. W. C., Allsopp, D. W. E., Shields, P., Šatka, A., Yu, S., Topolov, V. Y., et al. (2012). Nano-imprinting of highly ordered nano-pillars of lithium niobate (LiNbO 3). Ferroelectrics 429 (1), 62–68. doi:10.1080/00150193.2012.676955
Li, L., Zhao, J., and Gui, Z. (2004). The thermal sensitivity and dielectric properties of SrTiO3-based ceramics. Ceram. Int. 30 (7), 1073–1078. doi:10.1016/j.ceramint.2003.12.027
Liang, X., Chen, X., Yang, X., and Ni, J. (2021). The fabrication of LiNbO 3 memristors for electronic synapses using oxygen annealing. Nanotechnology 32 (2), 025706. doi:10.1088/1361-6528/abb1eb
Lin, J., Bo, F., Cheng, Y., and Xu, J. (2020). Advances in on-chip photonic devices based on lithium niobate on insulator. Photonics Res. 8 (12), 1910. doi:10.1364/PRJ.395305
Lin, S., Xiong, C., Ma, D., Li, H., Long, S., and Wang, B. (2018). Persistent luminescence found in Mg 2+ and Pr 3+ co-doped LiNbO 3 single crystal. J. Mater. Chem. C 6 (37), 10067–10072. doi:10.1039/C8TC03783C
Lin, Z., Lin, Y., Li, H., Xu, M., He, M., Ke, W., et al. (2022). High-performance polarization management devices based on thin-film lithium niobate. Light Sci. Appl. 11 (1), 93. doi:10.1038/s41377-022-00779-8
Liu, S., Zheng, Y., Fang, Z., Ye, X., Cheng, Y., and Chen, X. (2019). Effective four-wave mixing in the lithium niobate on insulator microdisk by cascading quadratic processes. Opt. Lett. 44 (6), 1456. doi:10.1364/OL.44.001456
Marković, D., Mizrahi, A., Querlioz, D., and Grollier, J. (2020). Physics for neuromorphic computing. Nat. Rev. Phys. 2 (9), 499–510. doi:10.1038/s42254-020-0208-2
McConville, J. P. V., Lu, H., Wang, B., Tan, Y., Cochard, C., Conroy, M., et al. (2020). Ferroelectric domain wall memristor. Adv. Funct. Mater. 30 (28), 2000109. doi:10.1002/adfm.202000109
Meng, X. Y., Wang, Z. Z., Zhu, Y., and Chen, C. T. (2007). Mechanism of the electro-optic effect in the perovskite-type ferroelectric KNbO3 and LiNbO3. J. Appl. Phys. 101 (10). doi:10.1063/1.2734534
Minakata, M. (2001). Recent Progress of 40 GHz high-speed LiNbO3 optical modulator. http://spiedl.org/terms.
Morozov, A. Y., Abgaryan, K. K., and Reviznikov, D. L. (2022). Interval model of a memristor crossbar network. Phys. Status Solidi (b) 259 (11). doi:10.1002/pssb.202200150
Nakajima, M., Inoue, K., Tanaka, K., Kuniyoshi, Y., Hashimoto, T., and Nakajima, K. (2022). Physical deep learning with biologically plausible training method. https://arxiv.org/abs/2204.13991.
Narayanareddy, B. (2008). “Growth and physical properties of LiNbO3, CsLiB6O10 and CsH(C4H4O5)H2O,” in Nonlinear optical crystals. (Bengaluru, India: Indian Institute of Science).
Nico, C., Monteiro, T., and Graça, M. P. F. (2016). Niobium oxides and niobates physical properties: review and prospects. Prog. Mater. Sci. 80, 1–37. doi:10.1016/j.pmatsci.2016.02.001
Ong, L.-H., Osman, J., and Tilley, D. R. (2001). Landau theory of second-order phase transitions in ferroelectric films. Phys. Rev. B 63 (14), 144109. doi:10.1103/PhysRevB.63.144109
Palatnikov, M., Makarova, O., Kadetova, A., Sidorov, N., Teplyakova, N., Biryukova, I., et al. (2023). Structure, optical properties and physicochemical features of LiNbO3:Mg,B crystals grown in a single technological cycle: an optical material for converting laser radiation. Materials 16 (13), 4541. doi:10.3390/ma16134541
Pan, B., Hu, J., Huang, Y., Song, L., Wang, J., Chen, P., et al. (2021). Demonstration of high-speed thin-film lithium-niobate-on-insulator optical modulators at the 2-µm wavelength. Opt. Express 29 (12), 17710. doi:10.1364/OE.416908
Pan, X., Shuai, Y., Wu, C., Zhang, L., Guo, H., Cheng, H., et al. (2019). Ar+ ions irradiation induced memristive behavior and neuromorphic computing in monolithic LiNbO3 thin films. Appl. Surf. Sci. 484, 751–758. doi:10.1016/j.apsusc.2019.04.114
Poberaj, G., Hu, H., Sohler, W., and Günter, P. (2012). Lithium niobate on insulator (LNOI) for micro-photonic devices. Laser Photonics Rev. 6 (4), 488–503. doi:10.1002/lpor.201100035
Qi, Y., and Li, Y. (2020). Integrated lithium niobate photonics. Nanophotonics 9 (6), 1287–1320. doi:10.1515/nanoph-2020-0013
Rabe, K. M., Ahn, C., and Triscone, J.-M. (2007). Physics of ferroelectrics: a modern perspective. Berlin, Germany: Springer.
Rivera, A., Garcia, G., Olivares, J., Crespillo, M. L., and Agulló-López, F. (2011). Elastic (stress–strain) halo associated with ion-induced nano-tracks in lithium niobate: role of crystal anisotropy. J. Phys. D Appl. Phys. 44 (47), 475301. doi:10.1088/0022-3727/44/47/475301
Roshchupkin, D. V., Irzhak, D. V., and Antipov, V. V. (2009). Study of LiNbO3 and LiTaO3 ferroelectric domain structures using high-resolution x-ray diffraction under application of external electric field. J. Appl. Phys. 105 (2). doi:10.1063/1.3066481
Sakayori, K., Matsui, Y., Abe, H., Nakamura, E., Kenmoku, M., Hara, T., et al. (1995). Curie temperature of BaTiO 3. Jpn. J. Appl. Phys. 34 (9S), 5443. doi:10.1143/JJAP.34.5443
Saleh, S., and Koldehofe, B. (2022). On memristors for enabling energy efficient and enhanced cognitive network functions. IEEE Access 10, 129279–129312. doi:10.1109/ACCESS.2022.3226447
Sánchez-Dena, O., Fierro-Ruiz, C. D., Villalobos-Mendoza, S. D., Flores, D. M. C., Elizalde-Galindo, J. T., and Farías, R. (2020). Lithium niobate single crystals and powders reviewed—Part I. Crystals 10 (11), 973. doi:10.3390/cryst10110973
Sang, X., Yu, C., Islam, M. K., and Lu, N. (2006). Generation of photon pairs in highly nonlinear photonic crystal fibres for quantum information processing. J. Optoelectron. Adv. Mater. 8 (5).
Saravi, S., Pertsch, T., and Setzpfandt, F. (2021). Lithium niobate on insulator: an emerging platform for integrated quantum photonics. Adv. Opt. Mater. 9 (22). doi:10.1002/adom.202100789
Stone, G., Knorr, B., Gopalan, V., and Dierolf, V. (2011). Frequency shift of Raman modes due to an applied electric field and domain inversion in LiNbO. Phys. Rev. B 84 (13), 134303. doi:10.1103/PhysRevB.84.134303
Suhara, T. (2009). Generation of quantum-entangled twin photons by waveguide nonlinear-optic devices. Laser and Photonics Rev. 3 (4), 370–393. doi:10.1002/lpor.200810054
Sumets, M. (2018). “Thin films of lithium niobate: potential applications, synthesis methods, structure and properties,” in Lithium niobate-based heterostructures (Bristol, United Kingdom: IOP Publishing). doi:10.1088/978-0-7503-1729-0ch1
Sun, D., Zhang, Y., Wang, D., Song, W., Liu, X., Pang, J., et al. (2020). Microstructure and domain engineering of lithium niobate crystal films for integrated photonic applications. Light Sci. Appl. 9 (1), 197. doi:10.1038/s41377-020-00434-0
Sun, J., Jiang, A.-Q., and Sharma, P. (2023). Ferroelectric domain wall memory and logic. ACS Appl. Electron. Mater. 5 (9), 4692–4703. doi:10.1021/acsaelm.3c00928
Suna, A., Baxter, O. E., McConville, J. P. V., Kumar, A., McQuaid, R. G. P., and Gregg, J. M. (2022). Conducting ferroelectric domain walls emulating aspects of neurological behavior. Appl. Phys. Lett. 121 (22). doi:10.1063/5.0124390
Tayi, A. S., Shveyd, A. K., Sue, A. C. H., Szarko, J. M., Rolczynski, B. S., Cao, D., et al. (2012). Room-temperature ferroelectricity in supramolecular networks of charge-transfer complexes. Nature 488 (7412), 485–489. doi:10.1038/nature11395
Tong, L., Peng, Z., Lin, R., Li, Z., Wang, Y., Huang, X., et al. (2021). 2D materials–based homogeneous transistor-memory architecture for neuromorphic hardware. Science 373 (6561), 1353–1358. doi:10.1126/science.abg3161
Uchino, K. (2017). Advanced piezoelectric materials: science and technology. Sawston, United Kingdom: Woodhead Publishing. doi:10.1016/C2015-0-01989-X
Vakulov, Z., Geldash, A., Khakhulin, D., Il’ina, M. V., Il’in, O. I., Klimin, V. S., et al. (2020). Piezoelectric energy harvester based on LiNbO3 thin films. Materials 13 (18), 3984. doi:10.3390/ma13183984
Volos, C. K., Kyprianidis, I. M., Stouboulos, I. N., Tlelo-Cuautle, E., and Vaidyanathan, S. (2015). Memristor: a new concept in synchronization of coupled neuromorphic circuits. J. Eng. Sci. Technol. Rev. 8 (2), 157–173. doi:10.25103/jestr.082.21
Voskresenskii, V. M., Starodub, O. R., Sidorov, N. V., and Palatnikov, M. N. (2017). Investigation of the cluster formation in lithium niobate crystals by computer modeling method. Crystallogr. Rep. 62 (2), 205–209. doi:10.1134/S1063774517020316
Wang, J., Pan, X., Luo, W., Shuai, Y., Xie, Q., Xu, J., et al. (2023a). Memristive synapse based on single-crystalline LiNbO 3 thin film with bioinspired microstructure for experience-based dynamic image mask generation. Adv. Electron. Mater. 9 (3). doi:10.1002/aelm.202201064
Wang, J., Pan, X., Wang, Q., Luo, W., Shuai, Y., Xie, Q., et al. (2022). Reliable resistive switching and synaptic plasticity in Ar+-irradiated single-crystalline LiNbO3 memristor. Appl. Surf. Sci. 596, 153653. doi:10.1016/j.apsusc.2022.153653
Wang, J., Pan, X., Zhao, Z., Xie, Y., Luo, W., Xie, Q., et al. (2023b). An infrared near-sensor reservoir computing system based on large-dynamic-space memristor with tens of thousands of states for dynamic gesture perception. Adv. Sci. 11, e2307359. doi:10.1002/advs.202307359
Wang, J., Zeng, H., Xie, Y., Zhao, Z., Pan, X., Luo, W., et al. (2023c). Analog ion-slicing LiNbO 3 memristor based on hopping transport for neuromorphic computing. Adv. Intell. Syst. 5 (10). doi:10.1002/aisy.202300155
Wang, S., Wang, W., Yakopcic, C., Shin, E., Kim, R. S., Subramanyam, G., et al. (2015). “Lithium based memristive device,” in 2015 National Aerospace and Electronics Conference (NAECON), Dayton, OH, USA, June, 2015, 333–335. doi:10.1109/NAECON.2015.7443092
Wang, S., Wang, W., Yakopcic, C., Shin, E., Subramanyam, G., and Taha, T. M. (2017). Experimental study of LiNbO3 memristors for use in neuromorphic computing. Microelectron. Eng. 168, 37–40. doi:10.1016/j.mee.2016.10.007
Wei, D., Wang, C., Wang, H., Hu, X., Wei, D., Fang, X., et al. (2018). Experimental demonstration of a three-dimensional lithium niobate nonlinear photonic crystal. Nat. Photonics 12 (10), 596–600. doi:10.1038/s41566-018-0240-2
Weigand, H., Vogler-Neuling, V. V., Escalé, M. R., Pohl, D., Richter, F. U., Karvounis, A., et al. (2021). Enhanced electro-optic modulation in resonant metasurfaces of lithium niobate. ACS Photonics 8 (10), 3004–3009. doi:10.1021/acsphotonics.1c00935
Weis, R. S., and Gaylord, T. K. (1985). Lithium niobate: summary of physical properties and crystal structure. Appl. Phys. A Solids Surfaces 37 (4), 191–203. doi:10.1007/BF00614817
Weiss, A., Frydendahl, C., Bar-David, J., Zektzer, R., Edrei, E., Engelberg, J., et al. (2022). Tunable metasurface using thin-film lithium niobate in the telecom regime. ACS Photonics 9 (2), 605–612. doi:10.1021/acsphotonics.1c01582
Witmer, J. D., Valery, J. A., Arrangoiz-Arriola, P., Sarabalis, C. J., Hill, J. T., and Safavi-Naeini, A. H. (2017). High-Q photonic resonators and electro-optic coupling using silicon-on-lithium-niobate. Sci. Rep. 7, 46313. doi:10.1038/srep46313
Wolf, R. A., and Trolier-McKinstry, S. (2004). Temperature dependence of the piezoelectric response in lead zirconate titanate films. J. Appl. Phys. 95 (3), 1397–1406. doi:10.1063/1.1636530
Wu, J., Huang, Y., Lu, C., Ding, T., Zheng, Y., and Chen, X. (2020). Tunable linear polarization-state generator of single photons on a lithium niobate chip. Phys. Rev. Appl. 13 (6), 064068. doi:10.1103/PhysRevApplied.13.064068
Xu, M., Chen, X., Guo, Y., Wang, Y., Qiu, D., Du, X., et al. (2023). Reconfigurable neuromorphic computing: materials, devices, and integration. Adv. Mater. 35 (51), e2301063. doi:10.1002/adma.202301063
Xu, S., Ren, Z., Dong, B., Zhou, J., Liu, W., and Lee, C. (2022). Mid-infrared silicon-on-lithium-niobate electro-optic modulators toward integrated spectroscopic sensing systems. Adv. Opt. Mater. 11 (4). doi:10.1002/adom.202202228
Xue, D., and Kitamura, K. (2003). Crystal structure and ferroelectricity of lithium niobate crystals. Ferroelectrics 297, 19–27. doi:10.1080/713642470
Yakopcic, C., Wang, S., Wang, W., Shin, E., Subramanyam, G., and Taha, T. M. (2017). “Methods for high resolution programming in lithuim niobate memristors for neuromorphic hardware,” in 2017 International Joint Conference on Neural Networks (IJCNN), Anchorage, AK, USA, May, 2017, 1704–1708. doi:10.1109/IJCNN.2017.7966056
Yoo, T. S., Lee, S. A., Roh, C., Kang, S., Seol, D., Guan, X., et al. (2018). Ferroelectric polarization rotation in order-disorder-type LiNbO 3 thin films. ACS Appl. Mater. Interfaces 10 (48), 41471–41478. doi:10.1021/acsami.8b12900
Yu, M., Barton, D., Cheng, R., Reimer, C., Kharel, P., He, L., et al. (2022). Integrated femtosecond pulse generator on thin-film lithium niobate. Nature 612 (7939), 252–258. doi:10.1038/s41586-022-05345-1
Yuan, S., Hu, C., Pan, A., Ding, Y., Wang, X., Qu, Z., et al. (2021). Photonic devices based on thin-film lithium niobate on insulator. J. Semicond. 42 (4), 041304. doi:10.1088/1674-4926/42/4/041304
Zaman, A. (2020). Modeling and experimental characterization of memristor devices for neuromorphic computing. Dayton, OH, United States: University of Dayton.
Zaman, A., Subramanyam, G., Shin, E., Yakopcic, C., Taha, T. M., Islam, A. E., et al. (2020). Experimental verification of current conduction mechanism for a lithium niobate based memristor. ECS J. Solid State Sci. Technol. 9 (10), 103003. doi:10.1149/2162-8777/abc3ce
Zaman, A., Wang, W., and Subramanyam, G. (2017). “Modeling of memristor device and analysis of stability issues,” in 2017 IEEE National Aerospace and Electronics Conference (NAECON), Dayton, OH, USA, June, 2017, 263–266. doi:10.1109/NAECON.2017.8268782
Zaman, A., Yakopcic, C., Wang, S., Shin, E., Wang, W., Taha, T. M., et al. (2019). “Analysis of lithium niobate memristor devices for neuromorphic programability,” in 2019 IEEE National Aerospace and Electronics Conference (NAECON), Dayton, OH, USA, July, 2019, 41–45. doi:10.1109/NAECON46414.2019.9058020
Zeng, X., Ashihara, S., Chen, X., Shimura, T., and Kuroda, K. (2008). Two-color pulse compression in aperiodically-poled lithium niobate. Opt. Commun. 281 (17), 4499–4503. doi:10.1016/j.optcom.2008.04.080
Zhang, J., Xu, B., Wang, Y.-S., Qin, Z., and Ke, S.-H. (2019). First-principles investigation of the ferroelectric, piezoelectric and nonlinear optical properties of LiNbO3-type ZnTiO3. Sci. Rep. 9 (1), 17632. doi:10.1038/s41598-019-53986-6
Zhao, J., Ma, C., Rüsing, M., and Mookherjea, S. (2020). High quality entangled photon pair generation in periodically poled thin-film lithium niobate waveguides. Phys. Rev. Lett. 124 (16), 163603. doi:10.1103/PhysRevLett.124.163603
Zhao, Y., Duan, W., Wang, C., Xiao, S., Li, Y., Li, Y., et al. (2023). LiNbO3 dynamic memristors for reservoir computing. Front. Neurosci. 17, 1177118. doi:10.3389/fnins.2023.1177118
Keywords: neuromorphic computing, LiNbO3-based memristors, synaptic behavior, nonlinear optical properties, artificial intelligence, advanced fabrication techniques, synaptic plasticity
Citation: Kibebe CG and Liu Y (2024) LiNbO3-based memristors for neuromorphic computing applications: a review. Front. Electron. Mater. 4:1350447. doi: 10.3389/femat.2024.1350447
Received: 05 December 2023; Accepted: 29 February 2024;
Published: 11 March 2024.
Edited by:
Carlos M. Costa, University of Minho, PortugalCopyright © 2024 Kibebe and Liu. This is an open-access article distributed under the terms of the Creative Commons Attribution License (CC BY). The use, distribution or reproduction in other forums is permitted, provided the original author(s) and the copyright owner(s) are credited and that the original publication in this journal is cited, in accordance with accepted academic practice. No use, distribution or reproduction is permitted which does not comply with these terms.
*Correspondence: Yue Liu, liuyue@lntu.edu.cn