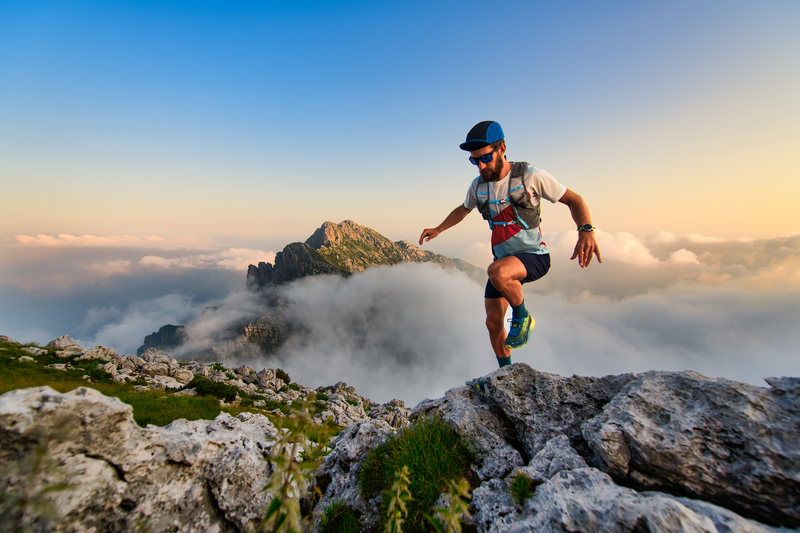
94% of researchers rate our articles as excellent or good
Learn more about the work of our research integrity team to safeguard the quality of each article we publish.
Find out more
ORIGINAL RESEARCH article
Front. Ecol. Evol.
Sec. Conservation and Restoration Ecology
Volume 13 - 2025 | doi: 10.3389/fevo.2025.1565632
This article is part of the Research Topic Ecosystem Condition Assessments: Progress towards a Global Standard View all 6 articles
The final, formatted version of the article will be published soon.
You have multiple emails registered with Frontiers:
Please enter your email address:
If you already have an account, please login
You don't have a Frontiers account ? You can register here
Ecosystem services (ESs) assessment plays a significant role in managing ecological resources. From the perspective of land use, this research aims to uncover the complex interdependence between ESs and their key drivers to clarify and optimize the ecological function zoning in the region. Thus, this research focuses on Henan Province in China, quantifying five key ESs, namely, carbon storage (CS), habitat quality (HQ), soil conservation (SC), water conservation (WC), and water yield (WY), and assessing their interactions from 2000 to 2020 and in 2035. Moreover, this study explores the social -ecological driving factors influencing these ESs. Finally, it classifies five types of ecosystem service bundles (ESBs). Results revealed the following: (1) From 2000 to 2020, the land use evolution was characterized by a large expansion of construction land, a continuous decrease in cultivated land area, and relatively stable changes in other land types. In the ecological protection (EP) scenario of 2035, the cultivated land area decreased the most, and the forest land area increased slightly. CS and HQ showed a trend of degradation, while SC, WY, and WC first fluctuated and then increased. (2) The synergistic relationship between each function was the main one, among which WC-WY, CS-HQ and HQ-SC showed significant synergistic relationships, CS-WC and HQ-WC showed a change from trade-off to synergistic relationship, while CS-WC and HQ-WC were mainly trade-off relationships. Meanwhile, most of the B4 bundles in the central part of the region were dominated, and the rest of the service bundles showed a decrease in volatility. (3) Elevation and slope are the dominant factors restricting the spatiotemporal distribution of CS, HQ, and SC. Temperature and precipitation are the primary conditions affecting the spatiotemporal differentiation of WY and WC. Moreover, the interaction between topographic factors and climatic conditions has a greater impact on each function than a single factor. The results of the study can provide a reference for the spatial management of land and ecological environmental protection in Henan Province.
Keywords: Land use type, ecosystem services, Multi-scenario prediction, spatiotemporal correlations, Geographic detector, Henan Province
Received: 23 Jan 2025; Accepted: 11 Mar 2025.
Copyright: © 2025 SUN, Qi, Wang, Cai and Tian. This is an open-access article distributed under the terms of the Creative Commons Attribution License (CC BY). The use, distribution or reproduction in other forums is permitted, provided the original author(s) or licensor are credited and that the original publication in this journal is cited, in accordance with accepted academic practice. No use, distribution or reproduction is permitted which does not comply with these terms.
* Correspondence:
Jun Cai, Anhui Agricultural University, Hefei, China
Disclaimer: All claims expressed in this article are solely those of the authors and do not necessarily represent those of their affiliated organizations, or those of the publisher, the editors and the reviewers. Any product that may be evaluated in this article or claim that may be made by its manufacturer is not guaranteed or endorsed by the publisher.
Research integrity at Frontiers
Learn more about the work of our research integrity team to safeguard the quality of each article we publish.