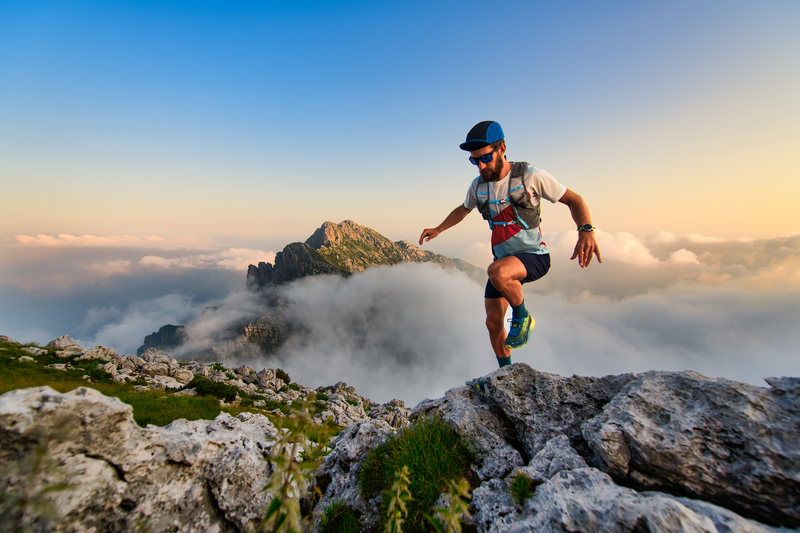
95% of researchers rate our articles as excellent or good
Learn more about the work of our research integrity team to safeguard the quality of each article we publish.
Find out more
ORIGINAL RESEARCH article
Front. Ecol. Evol.
Sec. Conservation and Restoration Ecology
Volume 13 - 2025 | doi: 10.3389/fevo.2025.1561640
This article is part of the Research Topic Impacts and Adaptations in Montane Ecosystems Facing Climate Change View all articles
The final, formatted version of the article will be published soon.
You have multiple emails registered with Frontiers:
Please enter your email address:
If you already have an account, please login
You don't have a Frontiers account ? You can register here
Prioritizing native and endemic species for conservation is fundamental to achieve larger broader objectives of safeguarding biodiversity, as these species are more pronevulnerable to extinction risks. Forecasting the climatic space niche of these species through species distribution models can be crucial for their habitat conservation and sustainable management in future. In the presentthis study, an ensemble modelling approach was used to predict the distribution of Bergenia stracheyi, a native alpine plant species of Himalayan region. The results revealed that the distribution of B. stracheyi is determined primarily influenced by Annual Mean Temperature (Bio1) and Annual Precipitation (Bio12). Ensemble model predictions revealed that under the current climatic conditions, the suitable habitats for B. stracheyi spread throughoutare distributed across higher elevations of Jammu and Kashmir and future ensemble model predictions indicated that, across all future climatic scenarios, the majority of the currently suitable habitats will remain suitable for the species in future. The model projects predicts a considerable increasesignificant expansion in suitable habitats for B. stracheyi, particularly under more severe climate change scenarios (RCP8.5). However, some areas currently identified as suitable, including parts of the Pir Panjal range and Mirpur (Pakistan), are predicted projected to become unsuitable for the species in the future. These shifts in plant distribution may have far-reaching consequences for ecosystem functioning and stability as well asand the services provided to human communities. Additionally, Tthese shifts may further lead to mismatches between the plant phenological events and pollinators leading to more devastating eventspotentially causing more ecological disruptions. Thus, the predicted range shifts in the distribution of B. stracheyi highlights the importance of local conservation measures to mitigate the impacts of climate change.
Keywords: Native and endemic, medicinal plants, Climate Change, ensemble modelling, Range change, Himalayan region
Received: 16 Jan 2025; Accepted: 31 Mar 2025.
Copyright: © 2025 Wani, Dar, Lone and Pant. This is an open-access article distributed under the terms of the Creative Commons Attribution License (CC BY). The use, distribution or reproduction in other forums is permitted, provided the original author(s) or licensor are credited and that the original publication in this journal is cited, in accordance with accepted academic practice. No use, distribution or reproduction is permitted which does not comply with these terms.
* Correspondence:
Javid Ahmad Dar, SRM University, Amaravathi, Andhra Pradesh, India
Disclaimer: All claims expressed in this article are solely those of the authors and do not necessarily represent those of their affiliated organizations, or those of the publisher, the editors and the reviewers. Any product that may be evaluated in this article or claim that may be made by its manufacturer is not guaranteed or endorsed by the publisher.
Research integrity at Frontiers
Learn more about the work of our research integrity team to safeguard the quality of each article we publish.