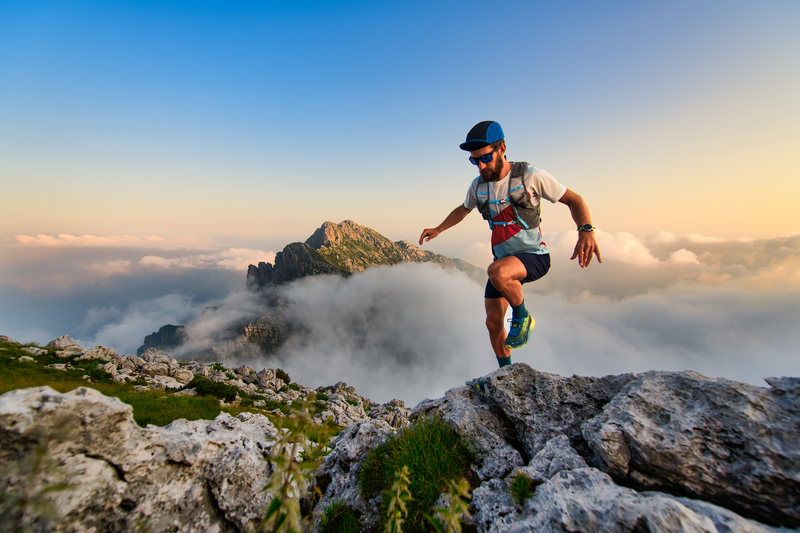
94% of researchers rate our articles as excellent or good
Learn more about the work of our research integrity team to safeguard the quality of each article we publish.
Find out more
ORIGINAL RESEARCH article
Front. Ecol. Evol.
Sec. Models in Ecology and Evolution
Volume 13 - 2025 | doi: 10.3389/fevo.2025.1549532
The final, formatted version of the article will be published soon.
You have multiple emails registered with Frontiers:
Please enter your email address:
If you already have an account, please login
You don't have a Frontiers account ? You can register here
Determining the suitable areas for pests and the dynamic shift of these areas under climate change is crucial for their management and control. The black planthopper (Ricania speculum) is an economically important pest in China, but knowledge about the suitable areas for its habitation is limited. Therefore, in this study, a Maxent model was constructed using 111 occurrence records and related environmental variables to predict the suitable areas for it to inhabit now and in the future (2041–2060, 2061–2080, and 2081–2100) in China, following by exploration of their dynamic shift. The model results showed that the currently suitable areas are predominantly in central and southern provinces such as Henan, Anhui, Hubei, and Guizhou, while the areas that are highly suitable are the most abundant among the three levels of suitability. Furthermore, the number of suitable areas for R. speculum in the three future periods was predicted to increase compared with that in the current period; the results also revealed that the suitable areas for R. speculum will consistently expand northward. The results of this study deepen our understanding of the impacts of climate change on the distribution of R. speculum and should also be beneficial for farmers and government departments to develop appropriate and cost-effective pest management strategies to reduce economic damage to China’s agricultural systems.
Keywords: Climate Change, MAXENT model, Suitable area, pest management, Ricania speculum
Received: 21 Dec 2024; Accepted: 06 Mar 2025.
Copyright: © 2025 Feng, Zhao, Wang and Zhang. This is an open-access article distributed under the terms of the Creative Commons Attribution License (CC BY). The use, distribution or reproduction in other forums is permitted, provided the original author(s) or licensor are credited and that the original publication in this journal is cited, in accordance with accepted academic practice. No use, distribution or reproduction is permitted which does not comply with these terms.
* Correspondence:
Yubo Zhang, Anshun University, Anshun, China
Disclaimer: All claims expressed in this article are solely those of the authors and do not necessarily represent those of their affiliated organizations, or those of the publisher, the editors and the reviewers. Any product that may be evaluated in this article or claim that may be made by its manufacturer is not guaranteed or endorsed by the publisher.
Research integrity at Frontiers
Learn more about the work of our research integrity team to safeguard the quality of each article we publish.