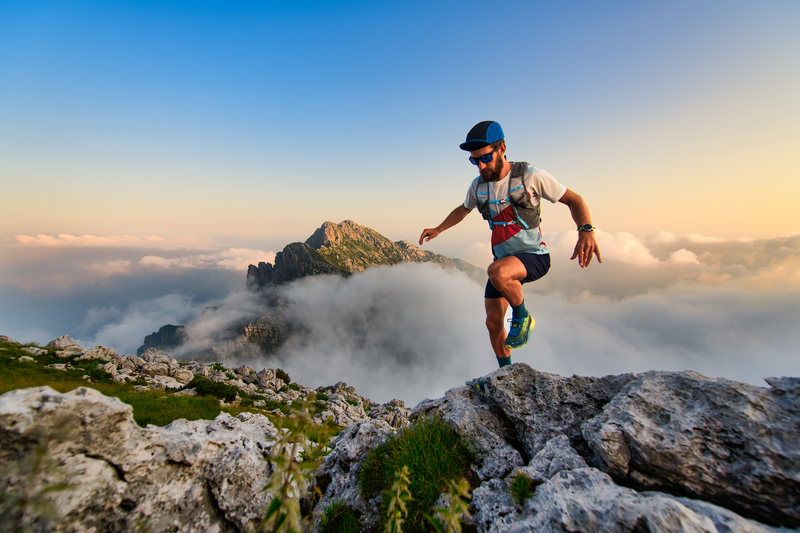
94% of researchers rate our articles as excellent or good
Learn more about the work of our research integrity team to safeguard the quality of each article we publish.
Find out more
ORIGINAL RESEARCH article
Front. Ecol. Evol.
Sec. Urban Ecology
Volume 13 - 2025 | doi: 10.3389/fevo.2025.1539909
This article is part of the Research Topic Extended Mind for the Design of Human Environment View all 11 articles
The final, formatted version of the article will be published soon.
You have multiple emails registered with Frontiers:
Please enter your email address:
If you already have an account, please login
You don't have a Frontiers account ? You can register here
In the context of the ‘dual-carbon’ goal, studying the complex relationship between carbon emissions and ecosystem service values brought about by land-use change is of great significance in promoting regional low-carbon optimization, territorial spatial governance and the achievement of the goal of carbon peaking and carbon neutrality. This study takes 286 cities in China as the research object, and based on the data of China’s land use and ecosystem service value (ESV) from 2012 to 2022, and adopts geo-spatial analysis techniques such as spatial autocorrelation and geographically-weighted regression models to study the spatial and temporal characteristics, the degree of coupling coordination, and the influencing factors of China’s municipal total land use carbon emissions and ESV. The results show that: (1) China’s total land-use carbon emissions have exhibited an overall increasing trend from 2012-2022. The total ESV has shown a downward trend characterized by an inverted “N” shape. (2) The coupling coordination effect between land-use carbon emissions and ESV in China generally shows a mirrored “L” growth shape. (3) There is a significant spatial negative correlation between land-use carbon emissions and ESV, primarily manifested as “high-high,” “high-low,” and “low-low” clustering characteristics. (4) Three socio-economic factors—local fiscal general budget expenditure, energy utilization efficiency, and total population—positively influence the coupling coordination of land-use carbon emissions and ESV.
Keywords: Land-use carbon emissions, ecosystem service value, Coupling coordination degree, Influencing mechanisms, Geographically weighted regression models, spatial autocorrelation
Received: 05 Dec 2024; Accepted: 26 Feb 2025.
Copyright: © 2025 Zhao, Yu and Liu. This is an open-access article distributed under the terms of the Creative Commons Attribution License (CC BY). The use, distribution or reproduction in other forums is permitted, provided the original author(s) or licensor are credited and that the original publication in this journal is cited, in accordance with accepted academic practice. No use, distribution or reproduction is permitted which does not comply with these terms.
* Correspondence:
Zhengyong Yu, Yunnan University, Kunming, 650500, Yunnan Province, China
Wei Liu, Tsinghua University, Beijing, China
Disclaimer: All claims expressed in this article are solely those of the authors and do not necessarily represent those of their affiliated organizations, or those of the publisher, the editors and the reviewers. Any product that may be evaluated in this article or claim that may be made by its manufacturer is not guaranteed or endorsed by the publisher.
Research integrity at Frontiers
Learn more about the work of our research integrity team to safeguard the quality of each article we publish.