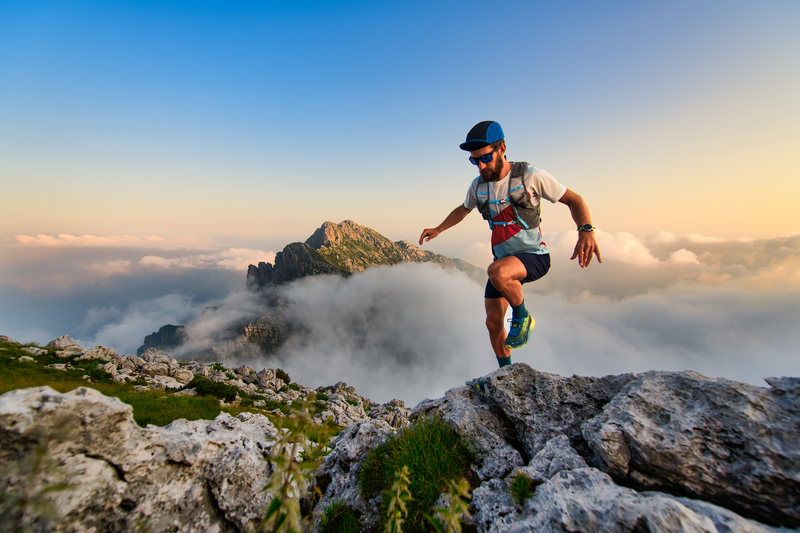
94% of researchers rate our articles as excellent or good
Learn more about the work of our research integrity team to safeguard the quality of each article we publish.
Find out more
METHODS article
Front. Ecol. Evol.
Sec. Chemical Ecology
Volume 13 - 2025 | doi: 10.3389/fevo.2025.1536032
The final, formatted version of the article will be published soon.
You have multiple emails registered with Frontiers:
Please enter your email address:
If you already have an account, please login
You don't have a Frontiers account ? You can register here
Proton Transfer Reaction Time-of-Flight Mass Spectrometry (PTR-TOF-MS) provides highresolution, real-time tracking of volatile organic compounds (VOCs), yet its analytical workflows for complex mixtures are often underexplored. This study presents a flexible data analysis workflow tailored for PTR-TOF-MS, designed to manage datasets with multiple replicates and experimental factors efficiently. Using lavender plants (Lavandula angustifolia Mill. var. diva) in a controlled greenhouse environment, we analyzed BVOC emissions over a 24-hour cycle. The workflow integrates data importation and optimization via the provoc R package, utilizing Multivariate Curve Resolution (MCR) to decompose spectra into biologically meaningful components and Redundancy Analysis (RDA) to assess component relevance. For this specific model experiment, we determined that normalization using the RUVr method and using and setting the MCR on a number of five components gave the most accurate results. However, this configuration is optimized for the current dataset; other experimental designs may require different normalization methods or numbers of components to achieve optimal results. The workflow enables effective differentiation of VOC emission patterns depending on experimental factors as well as an effective normalization method selection. It provides a systematic approach to PTR-TOF-MS data interpretation, adaptable to various experimental designs and scientific questions, making it valuable for studying complex VOC mixtures in chemical ecology and metabolomics. This method supports long-term, non-destructive sampling while delivering precise VOC profiling.
Keywords: PTR-ToF-MS (proton transfer reaction time-of-flight mass spectrometry), chemical ecology, Data analysis workflow, Lavandula angustifolia, VOC emission
Received: 28 Nov 2024; Accepted: 17 Mar 2025.
Copyright: © 2025 Fontez, Huguenin, JULLIEN, Moja, Proffit and Nicole. This is an open-access article distributed under the terms of the Creative Commons Attribution License (CC BY). The use, distribution or reproduction in other forums is permitted, provided the original author(s) or licensor are credited and that the original publication in this journal is cited, in accordance with accepted academic practice. No use, distribution or reproduction is permitted which does not comply with these terms.
* Correspondence:
Mathias Fontez, Université Jean Monnet, Saint-Étienne, 42023, Rhône-Alpes, France
Disclaimer: All claims expressed in this article are solely those of the authors and do not necessarily represent those of their affiliated organizations, or those of the publisher, the editors and the reviewers. Any product that may be evaluated in this article or claim that may be made by its manufacturer is not guaranteed or endorsed by the publisher.
Research integrity at Frontiers
Learn more about the work of our research integrity team to safeguard the quality of each article we publish.