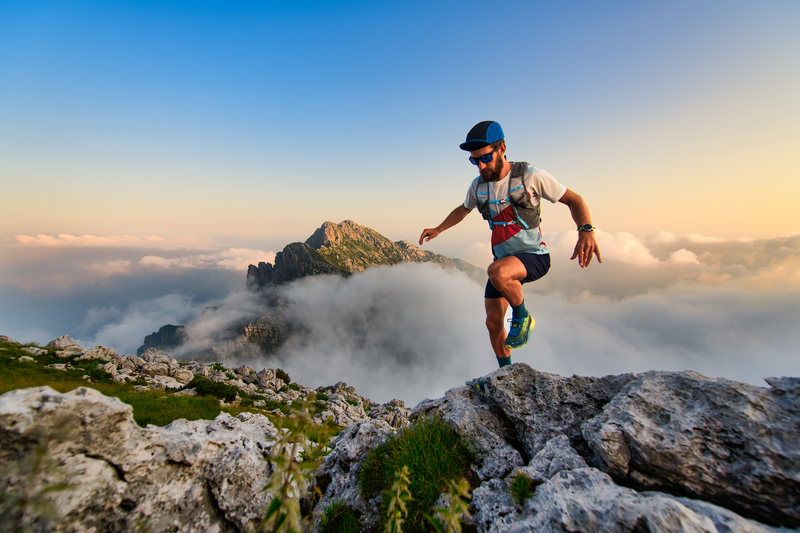
95% of researchers rate our articles as excellent or good
Learn more about the work of our research integrity team to safeguard the quality of each article we publish.
Find out more
ORIGINAL RESEARCH article
Front. Ecol. Evol. , 28 March 2025
Sec. Behavioral and Evolutionary Ecology
Volume 13 - 2025 | https://doi.org/10.3389/fevo.2025.1497949
Arrival timing in spring may be mediated by conditions experienced during migratory stopovers or staging areas, but our knowledge about their impact on migration timing and reproduction is limited. We explored the role of stopover duration on spring migration timing and successful incubation in cackling geese (Branta hutchinsii), which breed at high arctic latitudes where climate change effects are more pronounced. To track migration phenology and incubation duration, 236 light-level geolocators were deployed on cackling geese during the breeding period at Baffin Island, Nunavut, Canada between 2016 and 2018. Using data available for spring migration in the year following tag deployment (25 tags retrieved), we found that most geese had long, coastal stopovers (8–39 days) before crossing Hudson Bay on the last leg of their spring migration to their Baffin Island breeding area. We show that longer stopover durations at these Hudson Bay Lowland sites were associated with successful completion of incubation (a proxy for breeding success). Although spending more time at the stopover led to a later arrival date at the breeding ground, longer stopovers may increase the energy stores necessary for reproduction in these capital breeders. Stopover duration did not influence the incubation interval (number of days between arrival date at the breeding ground and start of incubation). Lastly, we found that the temperature at stopovers influenced migration timing, with higher temperatures resulting in earlier arrival at the breeding ground. Overall, our results demonstrate that conditions and behaviour at distant stopovers (1700–3000 km away) have important influence on timing and breeding success once birds arrive at their Arctic breeding sites. Therefore, our understanding of climate change impacts on these Arctic-breeding geese must also include the influence of en route conditions
Carry-over effects can be defined as activities or factors during one stage of the annual life cycle that have an impact on an animal’s performance in subsequent stages of the annual cycle (Harrison et al., 2011). These effects are particularly relevant during spring migration, when birds must balance the timing of their movements with environmental conditions to ensure successful arrival at their breeding grounds (Newton, 2010; Harrison et al., 2011; Schmaljohann and Both, 2017). Environmental conditions at key migratory stopover areas, such as precipitation patterns and temperature, could influence migrants (indirectly) through changes in food availability and quality, or (directly) through energy expenditure and could carry-over to affect subsequent migration or breeding events. For Arctic-breeding birds, the narrow temporal window for reproduction may be impacted by the variable conditions encountered along migratory routes (Madsen et al., 2007; Dickey et al., 2008; Harrison et al., 2011; McNamara and Westoby, 2011). For example, de Zwaan et al. (2022) showed the importance of carry-over effects of environmental conditions at stopovers close to the breeding site on reproductive success of an alpine breeding population of horned lark (Eremophila alpestris) in northern British Columbia, Canada. Understanding these interactions is essential for assessing how stopover use influences migration timing, breeding outcomes, and overall fitness in migratory species.
Bird migration can be categorized into two types: migratory flights and stationary periods (Schmaljohann et al., 2022). Stationary periods are commonly referred to as “stopovers”, with their overall duration typically being much longer than the total duration of all migratory flights (Green et al., 2002; Wikelski et al., 2003; Schmaljohann et al., 2012). Migratory birds may spend up to 90% of their migration time at stopover sites en route to and from their breeding grounds (Lindström, 2003; Paxton et al., 2008). These sites serve as critical resting and refuelling spots, allowing birds to avoid unfavourable weather conditions and use natural cues like sunset, stars, and the geomagnetic field to calibrate their compass system and orientation (Chernetsov, 2012). A comprehensive understanding of the factors influencing how stopovers impact subsequent migration timing and arrival date of birds is therefore important (Schmaljohann et al., 2022).
Some recent studies with migratory songbirds provide evidence for the impact of en route conditions on migration timing. In laboratory experiments, Klinner and Schmaljohann (2020) showed that increasing or decreasing temperature at simulated stopovers influenced subsequent migration timing of a medium and long-distance migratory songbird. In a wild setting, the probability of the northern wheatear (Oenanthe oenanthe), which includes Arctic-breeding populations, departing a stopover site was higher when the air temperature was lower at the beginning of autumn migration but increased as the temperature became higher towards the conclusion of the autumn migration period (Schmaljohann et al., 2017). Based on these results, we may predict similar patterns in other arctic-breeding species. However, the influence of stopover conditions on subsequent migration timing has been rarely investigated in capital breeding species such as Arctic-breeding migratory geese, where individuals accumulate the necessary energy for reproduction while migrating (Drent and Daan, 1980; Lepage et al., 1998). Thus our understanding of whether stopover conditions or behaviour carry-over to impact fitness has been limited.
The timing of migration and arrival at breeding sites can be flexible in response to local environmental conditions in migratory waterfowl. For example, some geese may track the advancing “green wave” of spring phenology (Van Wijk et al., 2012; Shariatinajafabadi et al., 2014). Many waterfowl species that migrate from their wintering grounds in the southern USA to their breeding sites at Delta Marsh in Manitoba arrive earlier with increasing temperature, and temperature or environmental factors en route may influence arrival timing at key stopover or breeding sites (Murphy-Klassen et al., 2005). For geese, the timing of arrival can be a crucial factor that affects the quality of forage they can access during their migration or upon reaching their breeding sites, as well as their subsequent reproductive output. In western Alaska, geese that arrived earlier at the breeding grounds in spring had access to higher quality foraging opportunities as compared to those arriving later (Beard et al., 2019). Kölzsch et al. (2015) demonstrated that barnacle geese (Branta leucopsis), a capital breeder, track the onset of spring phenology and access high quality food, resulting in earlier arrival at the breeding ground and the hatching of young during the peak of food availability. Migratory conditions that impact the timing of nesting could therefore greatly impact fitness. In greater snow geese (Anser caerulescens atlanticus L.), birds that nested later than the optimal time had lower reproductive success (Lepage et al., 2000). Additional work on the interactions between migration conditions and breeding success is needed in other arctic-breeding birds. Understanding how weather conditions during migration, particularly at key stopover areas, influence breeding success is critical for evaluating how some poorly studied species may respond to climate change. In this context, weather serves as a proxy for examining the potential impacts of climate variability on migration and breeding dynamics.
Our understanding of the potential impacts of climate variability on populations of cackling geese (Branta hutchinsii), as a capital breeder, is limited by a lack of critical data on their migration ecology and spring staging areas. Cackling geese, reclassified as a distinct species from Canada geese (Branta canadensis) through genetic research (Banks et al., 2004), nest in the Canadian Territories, with their range extending south to ~60° N (Mowbray et al., 2002). Band recovery data show mid-continent populations migrate through Manitoba. These medium- to long-distance migratory birds winter in the central and south-central U.S. (Mowbray et al., 2002) and follow the “green wave” northward in spring, timing their migration with snowmelt and vegetation growth to reach their nesting sites (van der Graaf et al., 2006).
Cackling geese returning to nest on Baffin Island from overwintering sites in mid-continent states, typically migrate through southern Manitoba, then stop along the Hudson Bay Lowlands to refuel, and then fly onward to arctic breeding sites, as indicated by data from recovered bird bands (https://www.agjv.ca/). Cackling geese are known to breed in the northernmost regions of Canada in Nunavut and the Northwest Territories, at approximately 60° N (Mowbray et al., 2002). Birds from mid-continental overwintering sites typically leave their wintering grounds around early April and reach their breeding grounds in early June (Neufeld, 2021).
We used light-level geolocators to directly track individual cackling geese, providing an opportunity to identify factors along spring migratory routes that may affect migration behaviour and arrival timing. We aimed to investigate the potential carry-over effects of the spring phenology at key stopover areas on arrival timing at arctic breeding sites and subsequent incubation success, which is a proxy for breeding success. Our specific objectives were to: 1) determine the core spring stopover locations and their timing. We expected that prolonged stopovers would occur at sites proximal to the breeding grounds, such as the Hudson Bay Lowlands, which are known to provide critical resources during migration (Neufeld, 2021). 2) investigate how spring phenology at stopover (temperature and snow depth) influences subsequent timing. We predicted that warmer temperatures and lower snow depth at stopover sites would enable earlier departure, aligning with findings in other migratory species (Schmaljohann et al., 2017; Klinner and Schmaljohann, 2020). Conversely, colder temperatures and higher snow cover were expected to delay departure, as birds would require extended time for refuelling to offset increased energetic demands. 3) determine the impact of stopover conditions on incubation success after arrival at the arctic breeding ground. Incubation success was measured by proxy as the completion of incubation, which we could detect through the use of geolocators mounted on the legs of geese because the light sensor was covered during active incubation. We expected that longer stopovers at sites with favourable conditions (e.g., higher temperatures and lower snow cover) would positively influence incubation success by allowing geese to accumulate sufficient energy reserves before arriving at breeding grounds. This prediction is consistent with studies showing that pre-breeding conditions and energy stores significantly impact reproductive success in Arctic-nesting geese (de Zwaan et al., 2022). We also anticipated that warmer temperatures at the breeding grounds would create better conditions for breeding, leading to quicker migration rates (Lawrence et al., 2022). In addition to yielding new insight into spring migration of an arctic breeding species, we also anticipated that our results would provide valuable information on the ecological dynamics influencing migration and reproduction in cackling geese. These findings may also inform conservation strategies by highlighting the role of stopover habitats in sustaining migratory populations under changing environmental conditions.
We deployed 236 light-level geolocators on adult female arctic-breeding, mid-continent cackling geese from Baffin Island (65.421°N, −70.966° E), Nunavut, Canada between 2016 and 2018. The deployment of tags was conducted by the Canadian Wildlife Service (CWS) as part of annual pre-hunting season banding activities. Of the 236 geolocators deployed on breeding females between 2016 and 2018, 25 units were successfully retrieved between 2016 and 2020. Of these, 8 geolocators were recovered at the original deployment locations, while 17 were retrieved from hunters. A total of 20 geolocators provided usable data, resulting in 41 complete migration tracks. Thirteen of these geolocators recorded data across multiple years, capturing more than one migration year for some individuals. The remaining five geolocators were excluded from the analysis due to incomplete data or missing spring migration records.
Geese were captured in a relatively short period each year, between 1 and 10 August. Flocks of adult geese along with their goslings were directed into portable net enclosures using a helicopter and strategically placed personnel. Capture was timed to occur during the annual wing molt of adults and when goslings were still flightless. The corralling was accomplished using either helicopters for aerial transport or kayaks and ground personnel for movement across water and land. Geolocators were installed as part of the yearly pre-hunting season banding activities. The weight of geolocators (Intigeo-C330, Migrate Technology Ltd.) was about 0.3% of the average cackling geese body mass (1,840 g ± 170 SD) (Mowbray et al., 2020). The geolocators were attached to a plastic leg band using a zip-tie and positioned on the tarsometatarsus bone. Birds were also banded with a federally-issued aluminium leg band with a unique number for identification. The sex of individuals was determined by cloacal examination and the presence of a brood patch. Only adult females with a brood patch (Jonsson et al., 2006), indicating that they were part of the local breeding population, were selected for geolocator tags. Geolocators were retrieved by recapturing the birds in subsequent years at the breeding location, or when they were harvested by hunters who reported them to the USGS Bird Banding Laboratory.
A light-level geolocator provides one pair of geographic coordinates (latitude and longitude) for each day (Lisovski et al., 2012). Geolocator data were downloaded from each tag using an Intigeo IF (interface unit). The R packages BAStag (version 0.1.3, Wotherspoon et al., 2016) and GeoLight (version 2.0.0, Lisovski and Hahn, 2012) were used to determine sunrise and sunset times, and for editing or omitting false twilights according to the light-level threshold method (Lisovski and Hahn, 2012). The threshold was set at 2 for most tracks/days but was increased to 12 when there was light pollution during dark periods to remove false twilights. The FLightR package (version 0.5.0, Rakhimberdiev et al., 2017) was used to process and refine the data and establish a track of each individual. Position error using geolocators can vary (~50–400 km) by species, position, and geolocator type (Lisovski et al., 2012). The spring departure date was defined as the date when birds passed 44° N, as 2 degrees below 44° N is their wintering ground (Mowbray et al., 2002). Geolocators no longer provide sunrise/sunset or daylength data (which is used to infer latitude and longitude) once birds reach continuous daylight at high arctic latitudes. Therefore, we defined the spring arrival date as the date when there was no longer any shading in the light data, indicating that birds had reached the high arctic and their breeding areas (Hupp et al., 2018) at 66.5° N, following rapid, over-water (Hudson Bay) flights from stopover areas to the southwest where geolocation by light was still possible (Neufeld, 2021). Given that no suitable habitat is available for landing or stopover during this last leg of travel, we expect that birds made direct, rapid flights toward their breeding destinations. Based on average flight speed (km/day) for geese (69.34 ± 18.55), we estimate our breeding arrival dates were accurate as most geolocators (about 90%) were retrieved at the same breeding locations where they were deployed, so migration distance from last stopover was measured as the distance between breeding locations and the last stopover. For geolocators retrieved by hunters during migration (about 8%), we used the breeding deployment location as the presumed breeding area for the same birds in the following spring. For incubating birds, long periods of complete darkness in the geolocator data occur due to the complete coverage of the geolocator’s light sensor by the bird’s body when they sat on the eggs to start incubation. We expected that the constancy of incubation resulting in continuous shading of the light sensor enabled us to discern between incubation and periods of rest where geese may intermittently shade the light sensor when sitting on their legs.
The Leaflet package (version 2.0.2, Cheng et al., 2018) was used to plot locations. The distance of spring migration, measured as Euclidean or Great Circle distance, was determined using the “sp” package (version 1.4–4, Bivand et al., 2013). The stationary.migration.summary function with a minimum duration of three days at one point (min.stay= 3) (Klaassen et al., 2011), from the FLightR package, was used to estimate stopover timing and duration as well as the location.
To address immediate environmental cues influencing migration and breeding, we used temperature and snow cover data instead of NDVI. This choice was made because temperature provides direct and immediate cues for migration decisions, such as snowmelt and food accessibility at stopover and breeding sites. Also, snow cover directly constrains habitat availability and foraging during critical stages of migration. NDVI, while valuable for assessing cumulative vegetation growth, is less reliable in Arctic regions due to sparse vegetation and lagged responses relative to immediate phenological changes (Walker et al., 2003; Raynolds et al., 2006). The temperature and snow depth data were downloaded using the Movebank Environmental Data Automated Track Annotation System (Dodge et al., 2013). This provided daytime air temperature (2m above the round) temperature (Kelvin) of the dates of departure and arrival at stopover as well as arrival at the breeding ground (extracted from NCEP North American Regional Reanalysis products [NCEP NARR, Land Surface Day Temperature 0.03° (∼3–3 km)], using Bilinear interpolation as recommended by Movebank (Dodge et al., 2013). Snow depth (m) at the date of departure and arrival at stopover as well as arrival at the breeding ground, was used [extracted from NCEP NARR, the snow depth at surface 0.03° (∼3–3 km)], at 3-hourly temporal resolution, using nearest neighbour interpolation (Dodge et al., 2013).
All the data processes and analyses were performed in R (version 3.6.3) using Rstudio (version 1.2.5019, R Core Team, 2023). After determining spring stopover locations of all individuals during different years of migration, kernel density analysis was used to determine the main spring stopovers during spring migration based on the stopover points of each individual bird, using fixed kernel densities in the program ArcGIS 10.7 (Esri, 2018). We determined kernel densities at 50, 80, and 100 percent of the total densities with cell size (0.078 km2). We did not define the radius because there was no information about the foraging distance of cackling geese.
To investigate which variables explain variation in the arrival date of cackling geese at their breeding grounds, we used linear mixed-effects models (LMMs) fit by REML using the ‘lme4’ package (version 1.1–19, Bates et al., 2015). In the global model, we used fixed effects of latitude and longitude of the breeding ground, the spring departure date of the wintering ground, the temperature at the arrival date and departure date at the core stopovers, the temperature at the arrival date at the breeding ground, the snow depth at the arrival date at the breeding ground, number of stopovers, and stopover duration at the core stopovers. The factor of total stopover duration during migration was omitted due to high correlation. We used geolocator ID (to account for individual-level variation) and the year in which the track began (to account for annual variation) as the random effects. The normality distribution of residuals and equal variance in the residuals of models were appropriate (Zuur et al., 2010). Collinearity among the predictor variables using variance inflation factor (VIF) (Marchowski et al., 2017) was less than 3. We used Cook’s distance to check the influence of outliers. The random effects that did not significantly specify any residual variance (using likelihood ratio tests) were removed from further analysis.
We defined the migration rate (km per day) as the distance between the core stopover site and the breeding ground, divided by the number of days used. To examine whether factors at the core stopover location would influence subsequent migration rate, we fitted LMMs. As fixed effects, we included temperature and snow depth at the departure date at the core stopover, temperature and snow depth at the arrival date at the breeding ground, distance between stopover and the breeding ground, and arrival and departure date at the core stopover. Random effects in this model were individual ID and year. To meet the assumption of residual normal distribution and equal variance we ran the model with the log 10 of migration rate. The VIF among the variables was less than 5.
To investigate influential factors on core stopover duration, we first conducted LMMs with fixed effects spring departure date, spring migration distance (km), temperature at the stopover at the arrival and departure date, snow depth at the stopover at the time of arrival and departure dates, as well as temperature and snow depth at the breeding ground at the time of arrival date. Year and individual ID (geolocator ID) were classified as random factors. None of the random factors impacted residual variance (using likelihood ratio tests) and were therefore removed from the model, and we fit LMs for the remaining variables. Residuals were normally distributed of equal variance (Zuur et al., 2010) and the VIF among the variables was less than 5.
We also investigated factors that would impact the probability of nest initiation using binomial generalized linear mixed effects models. We defined incubation success as a full incubation period lasting between 26 and 29 days (Jarvis and Bromley, 2000). Any incubation period shorter than 26 days, such as instances where only one day of incubation occurred, was classified as incubation failure. Therefore, we used 34 individuals data in the incubation analysis, resulting in data for 34 individuals for the incubation analysis. We fitted LMMs to examine influential factors on incubation success of the cackling geese. The fixed effects included spring arrival date, core stopover duration, temperature and snow depth at the breeding ground at the date of arrival (as an indicator of spring phenology at the breeding ground), temperature and snow depth at the core stopover at the date of departure, and the arrival to breeding interval (the number of days between arrival date and laying egg date). Year and individual ID (geolocator ID) were classified as random factors. We λ transformed the response variable (using boxcox function) to allow the model to converge. The random factor of year did not significantly specify residual variance (using likelihood ratio tests) and was therefore removed from the model. We fit LMMs with individual ID for the remaining variables.
All variables had a collinearity value of less than 3. To identify the best-fitting model, we used the Akaike Information Criteria corrected for small sample size (AICc) (Burnham and Anderson, 2002). AICc (‘MuMIn’ package (Barton, 2019)) helps to balance model complexity and goodness-of-fit, selecting models that explain the data well without overfitting. We built candidate models based on ecologically justified hypotheses and tested their performance using AICc. Models with ΔAICc < 2 were considered competitive, as they had similar levels of support in explaining the data. Then we considered the highest value of the marginal R2 (the fixed effects variance) and the conditional R2 (the whole model variance) to select the final model. This approach ensured that the selected model was not only parsimonious (low AICc) but also robust in explaining the observed variation in the data (Nakagawa and Schielzeth, 2013).
The kernel density analysis defined key geographic areas where most individuals (50–80% of the stopover points of different individuals) stopped over during spring migration. The first area spanned the early migratory period, straddling the U.S.-Canada border (Figure 1). This area represents the northern Great Plains, characterized by flat to rolling prairie landscapes transitioning to boreal forests in the north (Natural Resources Canada, 2023). Stopover durations in this area were between 3 and 39 days (16.43± 1.57) at the individual level. The second core stopover area was located along the southeast coast of Hudson Bay, where stopover duration was between 8 and 39 days (19.9± 1.0) at the individual level. We focused our subsequent analyses on this second stopover region (“last” hereafter). We considered this as the last stop before crossing Hudson Bay and arrival at the breeding grounds and that conditions here would be the most influential on subsequent migration timing and breeding. Spring arrival dates at the last stopover ranged from April 22 to May 21, and departure dates from the last stopover ranged from May 22 to June 11. The mean spring arrival date at the last stopover was May 12 (Julian day133 ± 0.70). The mean spring departure date from the last stopover was May 31 (Julian day133 153 ± 0.79).
Figure 1. The last spring stopovers of cackling geese along Hudson Bay just before crossing the bay and arriving at the breeding ground (Baffin Island). The map shows kernels of 50 (orange areas) and 80% (orange and grey areas) of the total density of the stopover site. The red points are all spring stopovers of cackling geese.
Spring departure dates from the wintering ground were between March 7 and April 21. The mean spring departure date was April 2 (Julian day133 93 ± 1.78). Spring arrival dates at the breeding ground ranged from May 31 to June 16. The average spring arrival date (2016–2020) was June 8 (Julian day 133 160 ± 0.59).
Birds with more northern breeding latitude and later departure timing, arrived about 2 (± 0.71, 95% CI 0.48–3.28) and 0.21 (± 0.04, 95% CI 0.13– 0.29) days later, respectively, than birds breeding at lower latitudes with earlier departure dates (Table 1). The arrival date at the breeding grounds was 0.17 (± 0.06, 95% CI 0.04–2.92) days later for each day that birds spent at the last stopover. Moreover, for each 1° C lower temperature at the time of departure from the last stopover site, arrival dates on the breeding grounds were about 0.28 (± 0.09, 95% CI −0.46–−1.06) days earlier. By increasing the number of stopovers, birds arrived later at the breeding grounds (1 ± 0.41days, 95% CI 0.17– 0.81) (Table 1).
Table 1. Summary of linear models (LMs) for spring migration timing and breeding arrival date in cackling geese.
The log of migration rate between the last stopover and the breeding ground was higher for birds with greater distance to travel during this last leg of migration to the breeding ground (estimate ± SD) (0.0002 ± 0.001, 95% CI 0.000046–00047) (Table 2). The birds that departed the last stopover location later had the highest log migration rate (estimate ± SD) (0.04 ± 0.007, 95% CI 0.03–0.06). Higher snow depth and temperature at the breeding ground were associated with the lower log of migration rate (estimate ± SD) (−2.65 ± 0.90, 95% CI −4.42–−0.88) and (−0.02 ± 0.009, 95% CI −0.03–−0.002), respectively (Table 2).
Table 2. Summary of linear models (LMs) for log 10 of migration rate between the last stopover and the breeding ground.
At the individual level, the shortest stopover duration was 8 days, while the longest stopover duration was 39 days. The mean stopover duration was (estimate ± SD) 19.9 ± 1.05 days. When the temperature at the arrival date of geese at the stopover was lower, the birds stayed longer at the stopover (estimate ± SD) by 1.06 ± 0.25 days (95% CI −1.55–−0.56) (Table 3).
The best model for the probability of breeding (incubation) success showed that longer stops were associated with longer (successful) incubation periods (estimate ± SD) 8.38± 2.84 (95% CI 2.05–14.19) (Figure 2). Birds with earlier arrival dates at the breeding ground had longer incubation periods (estimate ± SD) −16.83 ± 5.16 (95% CI −27.38–−5.21), thus incubation success. Moreover, higher temperature at arrival date at the breeding ground was also associated with longer incubation periods (estimate ± SD) 11.05± 4.10 (95% CI 2.28–19.42) (Table 4; Figure 3).
Figure 2. The last spring stopovers of cackling geese along Hudson Bay just before crossing the bay and arriving at the breeding ground (Baffin Island). The map shows kernels of 50 (orange areas) and 80% (orange and grey areas) of the total density of the stopover site. The red points are all spring stopovers of cackling geese.
Figure 3. Relationship between spring stopover duration and the duration of the incubation period (proxy for breeding success) for cackling geese (estimate ± SD) 8.38± 2.84. The Pearson correlation analysis resulted in R=0.14 (95% CI: −0.27–0.5). The blue line represents the predicted trend or regression line, and the grey ribbon illustrates the confidence intervals.
The analysis did not show a significant relationship between stopover duration at Hudson Bay and the likelihood of nest initiation (0.047 ± 0.059, 95% CI −0.06–0.16). This suggests that stopover duration at Hudson Bay may not strongly influence nest initiation probability in this dataset (Table 5).
For the first time, we demonstrate the core spring stopover areas for a Baffin Island breeding population of cackling geese. This stopover area is located in the Hudson Bay Lowlands and is the last one used before a long migratory flight crossing the bay before arrival at the breeding area. The timing and environmental conditions upon arrival at the breeding grounds in spring are closely linked to the breeding success of migrants, as the window for reproduction is limited (Alerstam and Lindström, 1990; Clark and Butler, 1999; Prop et al., 2003), particularly at higher latitudes. Consequently, changes along the migration route can have a profound impact on a bird’s reproductive success and ultimately affect population dynamics (Both and Visser, 2001; Forchhammer et al., 2002; Gordo et al., 2005). Our results demonstrate the importance of conditions and duration at the last spring stopover on subsequent spring migration phenology and reproductive success.
Cackling geese have a short pre-breeding interval and undergo rapid follicle development well before arrival on the breeding grounds, thus they are thought to employ a capital breeding strategy (Si et al., 2015; Neufeld, 2021). Our results suggest that they are strongly dependent on stopovers at Hudson Bay Lowlands during migration to refuel and gain energy stores required for reproduction, similar to lesser snow geese (Thomas and Prevett, 1982). Increased time at the last stopover led to later arrival on the breeding grounds, suggesting a potential trade-off between nutrient acquisition and timing of nesting. Spring phenology may play a role in nutrient dynamics. In warmer years, individuals departed for the breeding grounds earlier, perhaps because warmer conditions allowed them to reduce energetic expenditures and reach optimal breeding conditions earlier. Often, Arctic-breeding birds (e.g., Arctic-breeding goose species) arrive at the breeding ground before the snowmelt (Najafabadi et al., 2015; Si et al., 2015). Stopovers play a crucial role in allowing geese to adjust their migration timing based on environmental conditions. The weather conditions at the last stopover often correspond to those at the breeding grounds, providing birds with cues about conditions at their destination. During colder years, birds may extend their stay at stopovers to avoid harsh weather at higher latitudes. In contrast, during warmer years, geese may depart their last stopover earlier, as favourable conditions at the stopover and breeding grounds such as earlier snowmelt and advanced spring phenology, indicate that the breeding season has started earlier. Our results showed the birds that had fewer stopovers during spring migration, arrived earlier. This could be due to a trade-off between travel speed and energy reserves. Stopover use depends on factors like environmental conditions, food availability, and the bird’s energetic state (Hedenström and Alerstam, 1997). Birds in poorer condition may require more stops to refuel, delaying migration, while birds with sufficient reserves can skip stops, gaining reproductive advantages through early arrival (Møller, 1994; Kokko, 1999). Further research is needed to investigate why some birds make more stops. Factors such as age, health, and environmental conditions may be influential on the number of stopovers birds take during migration (Newton, 2010), and climate change may further impact stopover habitat conditions and available food (Gordo et al., 2005).
Cackling geese with later spring departure dates tended to compensate for their delayed migration by increasing their migration rate during the last leg, likely to arrive at breeding areas competitively. This flexible response reflects an ability to adjust migration speed in alignment with timing constraints, which is consistent with findings in other Arctic-breeding geese, where earlier arrivals at breeding grounds are generally associated with greater reproductive success (Kokko, 1999; Moore et al., 2005; Drent et al., 2007; Si et al., 2015; Lawrence et al., 2022). While we expected higher temperatures at the breeding ground to provide more favourable conditions for breeding resulting in faster migration rates, we found that breeding ground temperature and log of migration rate were not positively associated, possibly due to the independent influence of snow depth at nesting areas. Higher snow depth at the breeding grounds creates challenging conditions for cackling geese by covering food sources and making it harder to find adequate nourishment. To mitigate these challenges according to our results, geese appear to adjust their migration rate, slowing down during the last leg of migration. Specifically, migration rate decreased by approximately 2.65 log-units for each unit increase in snow depth at the breeding grounds, equating to a reduction of about 6.41 km/day for every additional 2.5 cm of snow. This highlights the birds’ flexibility in responding to environmental cues both at stopovers and their breeding sites. While photoperiod is a primary cue for migration timing in Arctic-breeding geese, ensuring consistent spring arrival (Mickelson, 1975), local conditions such as snow depth directly influence the onset of nesting and may have impacted migration rate and arrival timing in our study system.
We also examined the effect of the distance between the last stopover and the breeding site on migration rate, finding that birds travelled faster over longer distances. However, we acknowledge that the estimated effect size for this relationship was extremely small (0.0002 ± 0.001, 95% CI: 0.000046–0.00047), and while statistically significant, it may not show a meaningful biological effect, thus such small effect sizes should be interpreted cautiously. Generally, birds traveling longer distances may have higher rates (Newton, 2010) and higher rates of travel in our study system may also reflect selection for an earlier arrival. Another factor that we could not measure that may influence the migration rate is the amount of fuel that geese carry, which could impact the time they need to refuel at stopover sites. Higher fuel loads enable more efficient refuelling and shorter stopovers, resulting in a faster migration rate, while lower fuel loads might lead to longer stopover periods and slower migration speeds (Newton, 2010; Shamoun-Baranes et al., 2012).
We found that arrival dates were later for birds that spent longer at the last stopover, yet these birds tended to have a higher rate of incubation success. This contrasts with general patterns in migratory species, where individuals arriving earlier at breeding grounds tend to breed earlier and have higher reproductive success (Møller, 1994; Tombre and Erikstad, 1996; Potti, 1998; Bêty et al., 2003). Late-nesting Arctic-breeding birds generally have lower reproductive success across various measures (Perrins, 1970; Cooke et al., 1995), partly due to increased predation risk later in the season as predators, such as foxes or jaegers, become more active while feeding their young (Smith and Wilson, 2010; McKinnon et al., 2010; Brown et al., 2022). However, relatively few studies have explored the potential cost of earlier arrival, particularly in capital breeders that rely on internal reserves for successful reproduction. The potential disadvantages of earlier arrival could offset its benefits. Some costs include encountering severe weather conditions (Møller, 1994; Brown and Brown, 2000), limited food resources early in the season (Prop and de Vries, 1993; Bêty et al., 2004), and increased predation risk associated with poorly concealed nests or resource-limited territories (Palomino et al., 1998). While earlier arrivals are typically associated with higher reproductive success (Bêty et al., 2003), our findings suggest that later arrivals, supported by longer stopovers and better body condition, may offset some costs of early arrival, such as harsh weather and limited resources.
We found that duration at the last stopover had a negative relationship with the stopover temperature at arrival (i.e., cooler temperatures at arrival to the stopover induced longer stopover durations) and a positive relationship with temperature upon arrival at the breeding ground. This is consistent with our results for the breeding arrival date, where warmer breeding ground temperatures (indicating earlier spring phenology) promoted earlier departure from the last stopover and earlier breeding arrival. The onset of spring green-up is closely tied to temperature (Fitzjarrald et al., 2001; Suzuki et al., 2003) and we predicted that the higher the temperature at the breeding ground, the earlier the spring phenology. We expected that birds at their last stopover may receive cues as to conditions at their breeding sites and could depart accordingly. Our results support this prediction in that stopover duration was shorter when conditions at breeding sites were more advanced. However, we also show that conditions at stopover could be influential on timing and carry-over to affect incubation, thus conditions across late spring migration may interact to impact phenology and breeding success. While here we have used correlations to examine carry-over effects, an experimental approach would be stronger, but such approaches may be limited in long-distance migratory systems. Further work with direct tracking devices that provide greater temporal and spatial precision, could build upon our inferences here regarding potential carry-over effects of last stopover conditions on subsequent timing and breeding.
A common phenomenon in various species is that photoperiod is the primary stimulus that initiates gonadal development and courtship, while ambient temperature and food availability are employed for more precise determinations of the optimal timing for breeding (Hahn et al., 1997; Pulido and Berthold, 2004). Consistent with this, our study shows that temperature at the time of arrival at the breeding ground had a positive correlation with incubation duration in cackling geese (Table 4). However, our results also suggest the influence of pre-arrival conditions on timing and incubation success, as we found that duration at the last stopover had a positive relationship with the probability of successful incubation. Consistent with our results, previous studies have shown that time spent at pre-Arctic stopovers may serve two purposes: First, it enables birds to get within close proximity to their Arctic breeding areas, so they can reach their destination in less than a day (Burger et al., 2022). Additionally, it provides them with the opportunity to assess local cues to flexibly determine the best time to make the final flight. Second, this stopover may be of sufficient duration to allow birds to accumulate enough nutrient reserves, which are important for successful reproduction (Baldwin et al., 2022; Burger et al., 2022). Moreover, birds that arrived earlier at the breeding grounds had a longer incubation period, a proxy for breeding success, and higher chance for renesting in the case of the first clutch being lost (Gunnarsson et al., 2005). Birds arriving earlier than their competitors may have access to higher-quality breeding resources and territories, supporting higher success.
While this study provides valuable insight into the migration ecology of cackling geese and the impact of environmental conditions on incubation success, there are several limitations and biases that need to be recognized. This study does not account for post-hatching survival or other reproductive outcomes. Our metric of breeding success (i.e. incubation success) is only an estimate and should be supported by other measures in future studies. Further, our findings are based on a single breeding population and migratory system and replication in different populations is essential to validate and generalize our conclusions, particularly considering environmental variability across the broad geographic range of cackling geese. Also, the timing and environmental conditions during migration and at stopovers may introduce biases. Factors such as year-to-year variability in temperature and snow depth at greater timescales, as well as unmeasured variables like wind conditions or food availability, could influence migration phenology and breeding outcomes. Advancements in tracking technologies, such as archival GPS units and satellite-transmitting devices, offer higher spatial and temporal precision than geolocators and can address limitations like spatial accuracy near the equinox or at stopovers inherent in the use of geolocators (Bridge et al., 2013; Kays et al., 2015). Integrating these tools with remote sensing data, such as vegetation indices, can enhance understanding of how climate change influences migration and breeding (McKinnon and Love, 2018). Further technologies, such as machine learning, could be employed to assist in analysing large datasets and expanding studies across populations. These methods could help to make important additional advancements in our understanding migration ecology in these systems and thus inform conservation efforts under changing climate conditions (Hochachka et al., 2007). Future research that integrates additional environmental and physiological data will be critical to building on the findings presented here.
The Hudson Bay Lowlands serve as a critical stopover site for cackling geese, providing essential resources for refuelling for successful breeding. Longer stopovers in this region were linked to higher incubation success, emphasizing its importance in reproductive outcomes. Similar findings in other Arctic-nesting geese, such as lesser snow geese, highlight the role of pre-Arctic stopovers in supporting nutrient acquisition and migration timing (Thomas and Prevett, 1982; Bêty et al., 2003). Changes to the Hudson Bay Lowlands staging areas, due to climate change or resource competition, could profoundly affect cackling geese populations, underscoring the need for continued monitoring and conservation efforts.
Our results suggest that weather conditions at migratory stopover influence the timing of arrival and breeding in Arctic geese, where warmer conditions at stopover were associated with earlier arrival dates at high-Arctic breeding sites. Furthermore, longer stopover durations in the Hudson Bay Lowlands were associated with a higher likelihood of successful incubation once birds arrived at their breeding sites on Baffin Island, suggesting that stopover use plays a key role in supporting reproductive success. Overall, our results demonstrate that conditions experienced en route can influence arrival date and breeding outcomes, emphasizing the importance of the migratory period when assessing the potential impacts of environmental change on Arctic breeding birds. Finally, this research provides new evidence for the reliance of cackling geese on the Hudson Bay Lowlands during migration, which will be important for the ongoing assessment of potential impacts of environmental change and resource development on this species, as well as foraging competition with other goose populations that utilize the same staging areas.
The original contributions presented in the study are publicly available. This data can be found here: Dryad, doi: 10.5061/dryad.5dv41nsfw.
The animal study was reviewed and approved by the University of Manitoba’s Animal Care Committee [Animal Care Protocol Number F18 031/1(AC11388)]. The study was conducted in accordance with the local legislation and institutional requirements. Capture, handling, and marking of cackling geese were conducted in accordance with the Canadian Wildlife Service Animal Care and Use Guidelines, under Animal Care and Use Protocol 20JL04, and Scientific Permit to Capture and Band Migratory Birds (permits 10271) issued by the Canadian Wildlife Service, Environment and Climate Change Canada, Government of Canada.
SB: Data curation, Formal Analysis, Software, Writing – original draft, Writing – review & editing. FB: Methodology, Resources, Writing – review & editing. LN: Software, Writing – review & editing. KF: Conceptualization, Methodology, Resources, Supervision, Writing – review & editing.
The author(s) declare that financial support was received for the research and/or publication of this article. Arctic Goose Joint Venture administered by the U.S. Fish and Wildlife Service, the University of Manitoba, NSERC Discovery Grant program, the Province of Manitoba, the Manitoba Fish and Wildlife Enhancement Fund, Delta Waterfowl.
We thank all the collaborators, assistants, and volunteers who have helped to deploy and retrieve geolocators used in this study, especially J. Leafloor (Canadian Wildlife Service). We also thank the funding agencies that have made this study possible, including support through the Arctic Goose Joint Venture administered by the U.S. Fish and Wildlife Service, the University of Manitoba, NSERC Discovery Grant program, the Province of Manitoba, the Manitoba Fish and Wildlife Enhancement Fund, and Delta Waterfowl. We also thank the Mississippi Flyway Council and the Arctic Goose Joint Venture for their in-kind support of long-term arctic banding operations.
The authors declare that the research was conducted in the absence of any commercial or financial relationships that could be construed as a potential conflict of interest.
All claims expressed in this article are solely those of the authors and do not necessarily represent those of their affiliated organizations, or those of the publisher, the editors and the reviewers. Any product that may be evaluated in this article, or claim that may be made by its manufacturer, is not guaranteed or endorsed by the publisher.
The Supplementary Material for this article can be found online at: https://www.frontiersin.org/articles/10.3389/fevo.2025.1497949/full#supplementary-material
Supplementary Figure 1 | Individual-specific data at the main stopover sites. Each unique colour signifies an individual cackling goose.
Supplementary Table 1 | Summary of linear models (LMs) for spring migration timing in cackling geese.
Supplementary Table 2 | Summary of linear models (LMs) for stopover duration.
Supplementary Table 3 | Summary of linear mixed models (LMM) for incubation period.
Supplementary Table 4 | Summary of binary logistic regression models of probability of making a nest.
Alerstam T., Lindström Å. (1990). Optimal bird migration: the relative importance of time, energy, and safety. Bird migration: Physiol. Ecophysiol. pp, 331–351. doi: 10.1007/978-3-642-74542-3_22
Baldwin F., Alisauskas R., Leafloor J. (2022). Dynamics of pre-breeding nutrient reserves in subarctic staging Lesser Snow Geese (Anser caerulescens caerulescens) and Ross’s Geese (Anser rossii): implications for reproduction. Avian Conserv. Ecol. 17 (2), 38. doi: 10.5751/ACE-02326-170238
Banks R. C., Cicero C., Dunn J. L., Kratter A. W., Rasmussen P. C., Remsen J.V., et al. (2004). Forty-fifth supplement to the American Ornithologists’ Union check-list of North American birds. Auk. 121, 985–995. doi: 10.1093/auk/121.3.985
Barton K. (2019). MuMIn: Multi-Model Inference (R package version 1.43.6). Vienna, Austria: Comprehensive R Archive Network (CRAN). Available at: https://CRAN.R-project.org/package=MuMIn (Accessed February, 2020).
Bates D., Maechler M., Bolker B., Walker S. (2015). Fitting linear mixed-effects models using lme4. J. Stat. Software 67, 1–48. doi: 10.18637/jss.v067.i01
Beard K. H., Choi R. T., Leffler A. J., Carlson L. G., Kelsey K. C., Schmutz J. A., et al. (2019). Migratory goose arrival time plays a larger role in influencing forage quality than advancing springs in an Arctic coastal wetland. PloS One 14, e0213037. doi: 10.1371/journal.pone.0213037
Bêty J., Gauthier G., Giroux J. F. (2003). Body condition, migration, and timing of reproduction in snow geese: a test of the condition-dependent model of optimal clutch size. Am. Nat. 162, 110–121. doi: 10.1086/375680
Bêty J., Giroux J. F., Gauthier G. (2004). Individual variation in timing of migration: causes and reproductive consequences in greater snow geese (Anser caerulescens atlanticus). Behav. Ecol. Sociobiol. 57, 1–8. doi: 10.1007/s00265-004-0840-3
Bivand R. S., Pebesma E., Gomez-Rubio V. (2013). Applied spatial data analysis with R. 2nd ed. (NY, USA: Springer).
Both C., Visser M. E. (2001). Adjustment to climate change is constrained by arrival date in a long-distance migrant bird. Nature 411, 296–298. doi: 10.1038/35077063
Bridge E. S., Kelly J. F., Contina A., Gabrielson R. M., MacCurdy R. B., Winkler D. W. (2013). Advances in tracking small migratory birds: a technical review of light-level geolocation. J. Field Ornithol. 84, 121–137. doi: 10.1111/jofo.12011
Brown C. R., Brown M. B. (2000). Weather-mediated natural selection on arrival time in cliff swallows (Petrochelidon pyrrhonota). Behav. Ecol. Sociobiol. 47, 339–345. doi: 10.1007/s002650050674
Brown T. M., Olek V., Roth J., McKinnon L. (2022). Spatial variation in predator communities, predation risk, and shorebird daily nest survival near a sub-Arctic human settlement. Polar Biol. 45, 1175–1191. doi: 10.1007/s00300-022-03061-0
Burger J., Porter R. R., Niles L., Newstead D. J. (2022). Timing and duration of stopovers affects propensity to breed, incubation periods, and nest success of different wintering cohorts of red knots in the Canadian Arctic during the Years 2009 to 2016. Environ. Res. 215, 114227. doi: 10.1016/j.envres.2022.114227
Burnham K. P., Anderson D. R. (2002). “A Practical Information-Theoretic Approach,” in Model Selection And Multimodel Inference, vol. 10. (Springer, New York, NY).
Cheng J., Karambelkar B., Xie Y. (2018). Leaflet: Create interactive web maps with the JavaScript ‘Leaflet’ library (R package version 2.0.2). Vienna, Austria: Comprehensive R Archive Network (CRAN). Available at: https://CRAN.R-project.org/package=leaflet (Accessed July, 2018).
Chernetsov N. (2012). Passerine migration: stopovers and flight (Berlin & Heidelberg, Germany: Springer Science & Business Media).
Clark C. W., Butler R. W. (1999). Fitness components of avian migration: a dynamic model of Western Sandpiper migration. Evol. Ecol. Res. 1, 443–457.
Cooke F., Rockwell R. F., Lank D. B. (1995). The Snow Geese of La Pérouse Bay (Oxford University Press: Oxford).
de Zwaan D. R., Drake A., Camfield A. F., MacDonald E. C., Martin K. (2022). The relative influence of cross-seasonal and local weather effects on the breeding success of a migratory songbird. J. Anim. Ecol. 91, 1458–1470. doi: 10.1111/1365-2656.13705
Dickey M. H., Gauthier G., Cadieux M. C. (2008). Climatic effects on the breeding phenology and reproductive success of an arctic-nesting goose species. Glob. Change Biol. 14, 1973–1985. doi: 10.1111/j.1365-2486.2008.01622.x
Dodge S., Bohrer G., Weinzierl R., Davidson S. C., Kays R., Douglas D., et al. (2013). The environmental-data automated track annotation (Env-DATA) system: linking animal tracks with environmental data. Mov. Ecol. 1, 1–14. doi: 10.1186/2051-3933-1-3
Drent R. H., Daan S. (1980). The prudent parent: energetic adjustments in avian breeding 1. Ardea 55, 225–252. doi: 10.5253/arde.v68.p225
Drent R. H., Eichhorn G., Flagstad A., van der Graaf A. J., Litvin K. E., Stahl J. (2007). Migratory connectivity in Arctic geese: spring stopovers are the weak links in meeting targets for breeding. J. Ornithol. 148, 501–514. doi: 10.1007/s10336-007-0223-4
Esri R. (2018). ArcGIS Desktop: Release 10.7. (Redlands, CA: Environmental Systems Research Institute).
Fitzjarrald D. R., Acevedo O. C., Moore K. E. (2001). Climatic consequences of leaf presence in the eastern United States. J. Clim. 14, 598–614. doi: 10.1175/1520-0442(2001)014<0598:CCOLPI>2.0.CO;2
Forchhammer M. C., Post E., Stenseth N. C. (2002). North Atlantic Oscillation timing of long-and short-distance migration. J. Anim. Ecol. 71, 1002–1014. doi: 10.1046/j.1365-2656.2002.00664.x
Gordo O., Brotons L., Ferrer X., Comas P. (2005). Do changes in climate patterns in wintering areas affect the timing of the spring arrival of trans-Saharan migrant birds? Glob. Change Biol. 11, 12–21. doi: 10.1111/j.1365-2486.2004.00875.x
Green M., Alerstam T., Clausen P., Drent R., Ebbinge B. S. (2002). Dark-bellied Brent Geese Branta bernicla bernicla, as recorded by satellite telemetry, do not minimize flight distance during spring migration. Ibis 144, 106–121. doi: 10.1046/j.0019-1019.2001.00017.x
Gunnarsson T. G., Gill J. A., Newton J., Potts P. M., Sutherland W. J. (2005). Seasonal matching of habitat quality and fitness in a migratory bird. Proc. R. Soc B. 272, 2319–2323. doi: 10.1098/rspb.2005.3214
Hahn T. P., Boswell T., Wingfield J. C., Ball G. F. (1997). Temporal Flexibility in Avian Reproduction. In: Nolan V., Ketterson E. D., Thompson C. F. (eds). Curr. Ornithol. (Springer, Boston, MA) 14, 39–80. doi: 10.1007/978-1-4757-9915-6_2
Harrison X. A., Blount J. D., Inger R., Norris D. R., Bearhop S. (2011). Carry-over effects as drivers of fitness differences in animals. J. Anim. Ecol. 80, 4–18. doi: 10.1111/j.1365-2656.2010.01740.x
Hedenström A., Alerstam T. (1997). Optimum fuel loads in migratory birds: distinguishing between time and energy minimization. J. Theor. Biol. 189, 227–234. doi: 10.1006/jtbi.1997.0505
Hochachka W. M., Caruana R., Fink D., Munson A. R. T., Riedewald M., Sorokina D., et al. (2007). Data-mining discovery of pattern and process in ecological systems. J. Wildl. Manage. 71, 2427–2437. doi: 10.2193/2006-503
Hupp J. W., Ward D. H., Soto D. X., Hobson K. A. (2018). Spring temperature, migration chronology, and nutrient allocation to eggs in three species of arctic-nesting geese: Implications for resilience to climate warming. Glob. Change Biol. 24, 5056–5071. doi: 10.1111/gcb.14418
Jarvis R. L., Bromley R. G. (2000). Incubation behaviour of Richardson’s Canada geese on Victoria Island, Nunavut, Canada. In Dickson K. Ed. Towards conservation of the diversity of Canada Geese (Branta canadensis). Canadian Wildlife Service Occasional Paper No. 103. pp. 59–64.
Jonsson J. E., Afton A. D., Homberger D. G., Henk W. G., Alisauskas R. T. (2006). Do geese fully develop brood patches? A histological analysis of lesser snow geese (Chen caerulescens caerulescens) and Ross’s geese (C. rossii). J. Comp. Physiol. B. 176, 453–462. doi: 10.1007/s00360-006-0066-y
Kays R., Crofoot M. C., Jetz W., Wikelski M. (2015). Terrestrial animal tracking as an eye on life and planet. Science 348, aaa2478. doi: 10.1126/science.aaa2478
Klaassen R. H. G., Alerstam T., Carlsson P., Fox J. W., Lindström Å. (2011). Great flights by great snipes: Long and fast non-stop migration over benign habitats. Biol. Lett. 7, 833–835. doi: 10.1098/rsbl.2011.0343
Klinner T., Schmaljohann H. (2020). Temperature change is an important departure cue in nocturnal migrants: controlled experiments with wild-caught birds in a proof-of-concept study. Proc. R. Soc B. 287, 20201650. doi: 10.1098/rspb.2020.1650
Kokko H. (1999). Competition for early arrival in migratory birds. J. Anim. Ecol. 68, 940–950. doi: 10.1046/j.1365-2656.1999.00343.x
Kölzsch A., Bauer S., De Boer R., Griffin L., Cabot D., Exo K. M., et al. (2015). Forecasting spring from afar? Timing of migration and predictability of phenology along different migration routes of an avian herbivore. J. Anim. Ecol. 84, 272–283. doi: 10.1111/1365-2656.12281
Lawrence K. B., Barlow C. R., Bensusan K., Perez C., Willis S. G. (2022). Phenological trends in the pre-and post-breeding migration of long-distance migratory birds. Glob. Change Biol. 28, 375–389. doi: 10.1111/gcb.15916
Lepage D., Gauthier G., Menu S. (2000). Reproductive consequences of egg-laying decisions in snow geese. J. Anim. Ecol. 69, 414–427. doi: 10.1046/j.1365-2656.2000.00404.x
Lepage D., Gauthier G., Reed A. (1998). Seasonal variation in growth of greater snow goose goslings: the role of food supply. Oecologia 114, 226–235. doi: 10.1007/s004420050440
Lindström Å. (2003). Fuel deposition rates in migrating birds: causes, constraints, and consequences. In Avian Migration (Springer), 307–320. doi: 10.1007/978-3-662-05957-9_2
Lisovski S., Hahn S. (2012). GeoLight - processing and analysing light-based geolocator data in R. Methods Ecol. Evol. 3, 1055–1059. doi: 10.1111/j.2041-210X.2012.00248.x
Lisovski S., Hewson C. M., Klaassen R. H., Korner-Nievergelt F., Kristensen M. W., Hahn S. (2012). Geolocation by light: accuracy and precision affected by environmental factors. Methods Ecol. Evol. 3, 603–612. doi: 10.1111/j.2041-210X.2012.00185.x
Madsen J., Tamstorf M., Klaassen M., Eide N., Glahder C., Rigét F. (2007). Effects of snow cover on the timing and success of reproduction in high-Arctic pink-footed geese Anser brachyrhynchus. Polar Biol. 30, 1363–1372. doi: 10.1007/s00300-007-0296-9
Marchowski D., Jankowiak Ł., Wysocki D., Ławicki Ł., Girjatowicz J. (2017). Ducks change wintering patterns due to changing climate in the important wintering waters of the Odra River Estuary. PeerJ 5, e3604. doi: 10.7717/peerj.3604
McKinnon E. A., Love O. P. (2018). Ten years tracking the migrations of small landbirds: Lessons learned in the golden age of bio-logging. Auk 135, 834–856. doi: 10.1642/AUK-17-202.1
McKinnon L., Smith P. A., Nol E., Martin J. L., Doyle F. I., Abraham K. F., et al. (2010). Lower predation risk for migratory birds at high latitudes. Science 327, 326–327. doi: 10.1126/science.118301
McNamara K. E., Westoby R. (2011). Local knowledge and climate change adaptation on Erub Island, Torres Strait. Local Environ. 16, 887–901. doi: 10.1080/13549839.2011.615304
Mickelson P. G. (1975). Breeding biology of cackling geese and associated species on the Yukon-Kuskokwim Delta, Alaska. Wildl. Monogr. 45, 3–35. Available at: https://www.jstor.org/stable/3830407 (Accessed July, 2023).
Møller A. P. (1994). Phenotype-dependent arrival time and its conse- quences in a migratory bird. Behav. Ecol. Sociobiol. 35, 115–122. doi: 10.1007/BF00171501
Moore F. R., Woodrey M. S., Buler J. J., Woltmann S., Simons T. R. (2005). “Understanding the stopover of migratory birds: a scale-dependent approach,” in Bird Conservation Implementation and Integration in the Americas: Proceedings of the Third International Partners in Flight Conference. 684–689 (US Department of Agriculture, Forest Service General Technical Report PSW-GTR-191).
Mowbray T. B., Ely C. R., Sedinger J. S., Trost R. E. (2002). Cackling Goose (Branta hutchinsii), version 2.0. The Birds of North America. Eds. Poole A. F., Gill F. B. (Ithaca, NY, USA: Cornell Lab of Ornithology). doi: 10.2173/bna.cacgoo1.02
Mowbray T. B., Ely C. R., Sedinger J. S., Trost R. E. (2020). “Cackling Goose (Branta hutchinsii), version 1.0,” in Birds of the World. Eds. Rodewald P. G., Keeney B. K. (Cornell Lab of Ornithology, Ithaca, NY, USA). doi: 10.2173/bow.cacgoo1.01
Murphy-Klassen H. M., Underwood T. J., Sealy S. G., Czyrnyj A. A. (2005). Long-term trends in spring arrival dates of migrant birds at Delta Marsh, Manitoba, in relation to climate change. Auk. 122, 1130–1148. doi: 10.1642/0004-8038(2005)122[1130:ltisad]2.0.co;2
Najafabadi M. S., Darvishzadeh R., Skidmore A. K., Kölzsch A., Vrieling A., Nolet B. A., et al. (2015). Satellite-versus temperature-derived green wave indices for predicting the timing of spring migration of avian herbivores. Ecol. Indic. 58, 322–331. doi: 10.1016/j.ecolind.2015.06.005
Nakagawa S., Schielzeth H. (2013). A general and simple method for obtaining R² from generalized linear mixed-effects models. Methods Ecol. Evol. 4, 133–142. doi: 10.1111/j.2041-210x.2012.00261.x
Natural Resources Canada. (2023). The atlas of Canada. Available online at: https://natural-resources.Canada.ca/maps-tools-and-publications/maps/atlas-Canada/10784 (Accessed July, 2023).
Neufeld L. (2021). Comparing migration ecology among geographically distinct populations of Canada Geese (Branta canadensis) and Cackling Geese (Branta hutchinsii). (Master’s thesis). University of Manitoba, Canada. Available at: http://hdl.handle.net/1993/35933 (Accessed July 2022).
Palomino J. J., Martin-Vivaldi M., Soler M., Soler J. J. (1998). Functional significance of nest size variation in the Rufous Bush Robin Cercotrichas galactotes. Ardea 86, 177–185.
Paxton K. L., Van Riper C. III, O’Brien C. (2008). Movement patterns and stopover ecology of Wilson’s Warblers during spring migration on the lower Colorado River in southwestern Arizona. Condor 110, 672–681. doi: 10.1525/cond.2008.8602
Perrins C. M. (1970). The timing of birds’ breeding seasons. Ibis 112, 242–255. doi: 10.1111/j.1474-919X.1970.tb00096.x
Potti J. (1998). Arrival time from spring migration in male pied flycatchers: individual consistency and familial resemblance. Condor. 100, 702–708. doi: 10.2307/1369752
Prop J., Black J. M., Shimmings P. (2003). Travel schedules to the high arctic: Barnacle geese trade-off the timing of migration with accumulation of fat deposits. Oikos 103, 403–414. doi: 10.1034/j.1600-0706.2003.12042.x
Prop J., de Vries J. (1993). Impact of snow and food conditions on the reproductive performance of barnacle geese Branta leucopsis. Ornis Scand. 24, 110–121. doi: 10.2307/3676360
Pulido F., Berthold P. (2004). Birds and Climate Change. In Advances in Ecological Research. Møller A. P., Fiedler W., Berthold P. Eds. (San Diego, CA: Academic Press) 35, 149–181. doi: 10.1016/S0065-2504(04)35006-3
Rakhimberdiev E., Saveliev A., Piersma T., Karagicheva J. (2017). FLightR: An R package for reconstructing animal paths from solar geolocation loggers. Methods Ecol. Evol. 8, 1482–1487. doi: 10.1111/2041-210X.12765
Raynolds M. K., Walker D. A., Maier H. A. (2006). NDVI patterns and phytomass distribution in the circumpolar Arctic. Remote Sens. Environ. 102, 271–281. doi: 10.1016/j.rse.2006.02.016
R Core Team. (2023). R: A language and environment for statistical computing (Vienna, Austria: R Foundation for Statistical Computing). Available at: https://www.R-project.org/ (Accessed February, 2023).
Schmaljohann H., Both C. (2017). The limits of modifying migration speed to adjust to climate change. Nat. Clim. Change 7, 573–576. doi: 10.1038/nclimate3336
Schmaljohann H., Eikenaar C., Sapir N. (2022). Understanding the ecological and evolutionary function of stopover in migrating birds. Biol. Rev. 97, 1231–1252. doi: 10.1111/brv.12839
Schmaljohann H., Fox J. W., Bairlein F. (2012). Phenotypic response to environmental cues, orientation and migration costs in songbirds flying halfway around the world. Anim. Behav. 84, 623–640. doi: 10.1016/j.anbehav.2012.06.018
Schmaljohann H., Lisovski S., Bairlein F. (2017). Flexible reaction norms to environmental variables along the migration route and the significance of stopover duration for total speed of migration in a songbird migrant. Front. Zool. 14, 1–16. doi: 10.1186/s12983-017-0203-3
Shamoun-Baranes J., Bom R., van Loon E. E., Ens B. J., Oosterbeek K., Bouten W. (2012). From sensor data to animal behaviour: an oystercatcher example. PloS One 7, e37997. doi: 10.1371/journal.pone.0037997
Shariatinajafabadi M., Wang T., Skidmore A. K., Toxopeus A. G., Kölzsch A., Nolet B. A., et al. (2014). Migratory herbivorous waterfowl track satellite-derived green wave index. PloS One 9 (9), e108331. doi: 10.1371/journal.pone.0108331
Si Y., Xin Q., De Boer W. F., Gong P., Ydenberg R. C., Prins H. H. T. (2015). Do Arctic breeding geese track or overtake a green wave during spring migration? Sci. Rep. 5, 8749. doi: 10.1038/srep08749
Smith P. A., Wilson S. (2010). Intraseasonal patterns in shorebird nest survival are related to nest age and defence behaviour. Oecologia 163, 613–624. doi: 10.1007/s00442-010-1644-y
Suzuki R., Nomaki T., Yasunari T. (2003). West–east contrast of phenology and climate in northern Asia revealed using a remotely sensed vegetation index. Int. J. Biometeorol. 47, 126–138. doi: 10.1007/s00484-003-0164-4
Thomas V. G., Prevett J. P. (1982). The role of horsetails (Equisetaceae) in the nutrition of northern-breeding geese. Oecologia 53, 359–363. doi: 10.1007/BF00389012
Tombre I. M., Erikstad K. E. (1996). An experimental study of incubation effort in high-Arctic barnacle geese. J. Anim. Ecol. 65, 325–331. doi: 10.2307/5878
Van der Graaf A. J., Stahl J., Klimkowska A., Bakker J. P., Drent R. H. (2006). Surfing on a green wave-how plant growth drives spring migration in the Barnacle Goose Branta leucopsis. Ardea-Wageningen- 94, 567.
Van Wijk R. E., Kölzsch A., Kruckenberg H., Ebbinge B. S., Müskens G. J., Nolet B. A. (2012). Individually tracked geese follow peaks of temperature acceleration during spring migration. Oikos 121, 655–664. doi: 10.1111/j.1600-0706.2011.20083.x
Walker D. A., Jia G. J., Epstein H. E., Raynolds M. K., Chapin Iii F. S., Copass C., et al. (2003). Vegetation-soil-thaw-depth relationships along a low-arctic bioclimate gradient, Alaska: synthesis of information from the ATLAS studies. Permafr. Periglac. Process 14, 103–123. doi: 10.1002/ppp.452
Wikelski M., Tarlow E. M., Raim A., Diehl R. H., Larkin R. P., Visser G. H. (2003). Costs of migration in free-flying songbirds. Nature 423, 704–704. doi: 10.1038/423704a
Wotherspoon S., Sumner M., Lisovski S. (2016). BAStag: Basic data processing for light-based geolocation archival tags (R package version 0.1.3). Available at: https://github.com/SWotherspoon/BAStag.
Keywords: carry-over effect, cackling goose (Branta hutchinsii), Hudson Bay Lowlands, migratory stopovers, incubation success, geolocator
Citation: Bani Assadi S, Baldwin F, Neufeld L and Fraser KC (2025) Temperature and stopover duration carry-over to affect Arctic arrival timing and breeding success in the cackling goose (Branta hutchinsii). Front. Ecol. Evol. 13:1497949. doi: 10.3389/fevo.2025.1497949
Received: 18 September 2024; Accepted: 03 March 2025;
Published: 28 March 2025.
Edited by:
Anindita Bhadra, Indian Institute of Science Education and Research Kolkata, IndiaReviewed by:
Francisco Castaño Vázquez, Universidad Complutense de Madrid, SpainCopyright © 2025 Bani Assadi, Baldwin, Neufeld and Fraser. This is an open-access article distributed under the terms of the Creative Commons Attribution License (CC BY). The use, distribution or reproduction in other forums is permitted, provided the original author(s) and the copyright owner(s) are credited and that the original publication in this journal is cited, in accordance with accepted academic practice. No use, distribution or reproduction is permitted which does not comply with these terms.
*Correspondence: Saeedeh Bani Assadi, YmFuaWFzc3NAbXl1bWFuaXRvYmEuY2E=
Disclaimer: All claims expressed in this article are solely those of the authors and do not necessarily represent those of their affiliated organizations, or those of the publisher, the editors and the reviewers. Any product that may be evaluated in this article or claim that may be made by its manufacturer is not guaranteed or endorsed by the publisher.
Research integrity at Frontiers
Learn more about the work of our research integrity team to safeguard the quality of each article we publish.