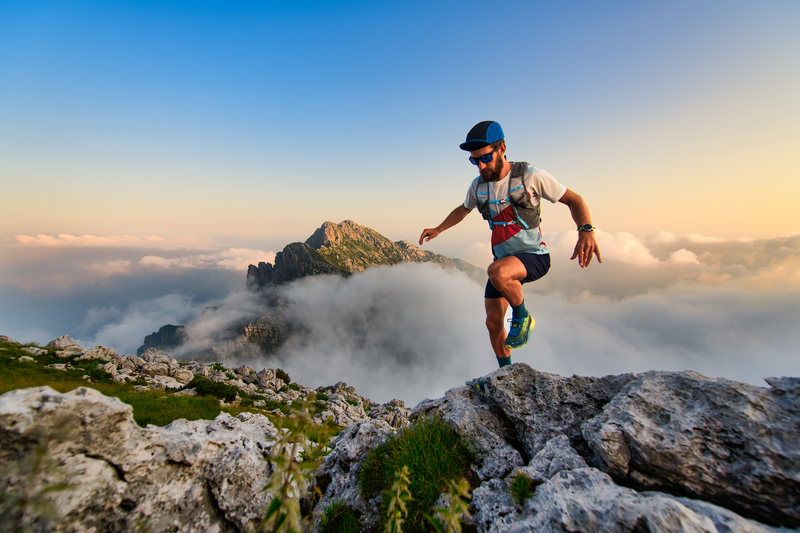
95% of researchers rate our articles as excellent or good
Learn more about the work of our research integrity team to safeguard the quality of each article we publish.
Find out more
REVIEW article
Front. Ecol. Evol. , 09 December 2024
Sec. Ecophysiology
Volume 12 - 2024 | https://doi.org/10.3389/fevo.2024.1505025
This article is part of the Research Topic Advances in Ecological Stoichiometry View all 4 articles
The ‘black boxes’ of ecological stoichiometry, planktonic microbes, have long been recognized to have considerable effects on global biogeochemical cycles. Significant progress has been made in studying these effects and expanding our understanding of microbial stoichiometry. However, the ‘black box’ has not been completely cracked open; there remain gaps in our knowledge of the fate of elements within the phytoplankton cell, and the effect of external processes on nutrient fluxes through their metabolism and into macromolecules and biomass - the eponymous ‘gray box’. In this review paper, we describe the development of an integrative modeling approach that involves a stoichiometrically explicit model of Macromolecular Allocation and Genome-scale Metabolic Analysis (MAGMA) to gain insights into the intra- and extracellular fluxes of nutrients using the cyanobacterium Parasynechococcus marenigrum WH8102 as a target model organism. We then describe an example of the genome-scale resources for P. marenigrum that can be used to build such an integrated modeling tool to see through the gray box of phytoplankton stoichiometry and improve our understanding of the effects of resource supplies and other environmental drivers, especially temperature, on C:N:P demand, acquisition, and allocation at the cellular level.
In 1934, Alfred Redfield observed that the molar ratio of carbon (C), nitrogen (N), and phosphorus (P) in phytoplankton biomass was approximately 106:16:1. This pattern was found to be consistent throughout the world’s oceans, from surface waters to deep dissolved nutrient pools (Redfield, 1934). Redfield proposed that this ratio was shaped by intracellular processes in phytoplankton, a hypothesis supported by further research (Pahlow, 2005; Loladze and Elser, 2011).
Although the Redfield ratio demonstrates a pronounced central tendency of 106:16:1, the existence of variation around these canonical values remains relevant and important to understand in order to distinguish stochastic variability in phytoplankton stoichiometry from more extreme changes that may indicate significant disturbances in global biogeochemical cycling. Shifts in cellular stoichiometry are frequently associated with specific growth rate, as at low growth rates, organisms tend to reflect the stoichiometry of their surrounding environment (“you are what you have available to eat”), whereas, at high growth rates, the stoichiometry of organisms deviate from the environment and converge towards a narrow range of values (“you eat what you need”) (Klausmeier et al., 2004a; Persson et al., 2010). Indeed, at maximum growth rate, cellular elemental composition reaches a species-specific ratio, often similar to the established Redfield Ratio of C:N:P 106:16:1 (Redfield, 1934; Rhee and Gotham, 1981; Sterner and Elser, 2003; Klausmeier et al., 2004a; Bi et al., 2012).
There is also a significant spatial component to the observed variation around the canonical Redfield Ratio in the oceans. Latitudinal variation in phytoplankton stoichiometry was identified in a model-data synthesis by Weber and Deutsch (2010) and subsequently supported by field sampling and further data synthesis from Martiny et al. (2013). The strongest deviations from Redfield were found in the warm, low-latitude, low-nutrient ocean gyres, which were found to have both higher C:N and higher N:P ratios (195:28:1), while ratios more closely resembling Redfield (78:13:1) were found in the colder, nutrient-rich, high-latitude waters. There is also spatial variation due to depth with most of the variation in stoichiometric ratios found in the surface while the deep ocean N:P was typically closer to the Redfield ratio, regulated by net export of production (Gruber and Deutsch, 2014).
Taxonomic differences are also relevant. For example, N-sensitive species (i.e., those with low C:N and high N:P ratios, which are therefore primarily N-limited) show higher C and lower N under N limitation, whereas insensitive species maintain stable C:N ratios (Goldman and Peavey, 1979; Garcia et al., 2016). Diurnal cycles (Olson et al., 1986) and light availability also affects stoichiometry, with C content increasing in some lineages (Leonardos and Geider, 2004) but less in others (MacIntyre et al., 2002), and N content decreasing with increasing light in certain lineages (Finkel et al., 2006). Much of what we see at the whole cellular level in C:N:P variation of planktonic microbes may be linked to metabolic and biochemical shifts at a subcellular level.Under high light and nutrient limitation, the N-rich light-harvesting apparatus is down-regulated, while the C-rich energy reserves increase (Kromkamp, 1987; Geider et al., 1996), leading to high C:N ratios. This is reversed under low light conditions (Bouman et al., 2006). Macromolecular allocation also varies diurnally, with photosynthetic proteins being expressed during the day, cell division proteins near sunset, and carbohydrate metabolism proteins at night, causing variations in nucleic acid, pigment, and protein contents (Vaulot et al., 1995; Matallana-Surget et al., 2014; Lopez et al., 2016) and resulting in diurnally variable C:N:P ratios. Under P limitation, phytoplankton upregulate proteins involved in phosphate regulation to increase P uptake or access organically bound P (Torriani-Gorini, 1987; Wanner, 1993), which can increase total cellular protein content (Liefer et al., 2019). Under N limitation, N uptake proteins are also upregulated (Herrero et al., 1985; Tolonen et al., 2006), but to a lesser extent than under P limitation, resulting in more modest changes in protein content (Liefer et al., 2019).
Despite their fundamental role at the base of the food web exerting bottom up controls on the ecosystem, planktonic microbes, including bacteria and phytoplankton, have historically been considered as “black boxes” (Riley, 1946), due to the inherent complexity and limited understanding of the underlying biochemistry at the microscopic scale. In fact, microbial communities encompass a wide range of metabolic pathways and can rapidly adapt to changing environmental conditions, with important implications for biogeochemical cycling. However, extensive work has been carried out to unlock this black box to better understand the role of planktonic microbes in global biogeochemical cycles and to incorporate this information into stoichiometric models of nutrient cycling. Thus, the microbial black box from the early days of marine biogeochemistry is now much more transparent but knowledge gaps do remain. We therefore argue that the microbial black box has become a semi-transparent “gray box” (Figure 1), involving a mixture of known and unknown aspects of cellular physiology. These improvements in understanding are due to the application of new methods, such as advanced elemental analysis techniques such as XRMA and Raman microscopy that have allowed researchers to quantify the C:N:P ratios of individual phytoplankton cells (Hall et al., 2011; Segura-Noguera et al., 2016). Mechanistic models of elemental allocation have also provided vital insights into how phytoplankton cells build biomass and allocate to macromolecular pools under varying resource supply (Inomura et al., 2020a; Armin and Inomura, 2021). Meanwhile, genome-scale metabolic modeling has been applied, to name but a few, to studying the evolution of phosphorus metabolism (Casey et al., 2016), the balance of nitrogen and sulfur in diatom-mediated redox equilibrium in the oceans (van Tol and Armbrust, 2021), the dynamics of carbon storage and nutrient release in marine cyanobacteria (Ofaim et al., 2021), and dynamic nitrogen metabolism in a model diatom (Smith et al., 2019).
Figure 1. Diagram showing fluxes of carbon, nitrogen, and phosphorus between the atmosphere, the oceans, and primary producers and between macromolecular pools within the phytoplankton ‘gray box’. The colors represent different elements involved in nutrient cycling: red indicates nitrogen, yellow represents carbon, and blue corresponds to phosphorus.
In addition to the clear roles of resource supply and taxonomic variation in determining the elemental balance of an ecosystem and its biota, other abiotic features of the environment can also have a significant effect on the balance and flow of nutrients through an ecosystem. Of particular concern are abiotic factors that are changing rapidly, radically, and on a global scale, as a result of human activities, and therefore have the potential to alter global biogeochemical cycles in unpredictable and non-additive ways (Velthuis et al., 2022).
Combinations of modeling, field sampling, and controlled experiments have generated important hypotheses about the role of the abiotic environment as a driver of stoichiometric variation. Ocean acidification for example, caused by increased aqueous pCO2 concentrations, has been mechanistically linked to stochastic changes in C:N ratios in particulate organic matter through in situ mesocosm experiments (Taucher et al., 2021) with modeling approaches also hypothesizing interactive effects of nutrient availability on responses to acidification (Verspagen et al., 2014). Meanwhile, both experimental and modeling approaches have demonstrated a highly interactive relationship between nutrient supply and temperature, with strongly temperature-dependent effects of nutrient supply on producer (Verspagen et al., 2014; DeVries, 2018; O'Donnell et al., 2021) and consumer (Starke et al., 2021) growth rates. A recent meta-analysis has also found strong interactions among temperature, biogeography and phenotype on determining the stoichiometry of marine phytoplankton (Yvon-Durocher et al., 2015).
Temperature can also influence macromolecular allocation, with higher temperatures leading to lower ribosome allocation (Hochachka and Somero, 1984; Toseland et al., 2013; Yvon-Durocher et al., 2015) and thus lower RNA content (Tempest and Hunter, 1965; Yun et al., 1996). Such decreases should increase cellular C:P and N:P. Toseland et al. (2013) also found that in low-latitude environments (<30°N/S), phytoplankton rates of protein synthesis were increased even though ribosomal production was decreased, indicating that phytoplankton require a lower density of ribosomes for protein synthesis at higher temperatures and a higher density at lower temperatures.
What many of the temperature-based studies (Yvon-Durocher et al., 2015; Moorthi et al., 2016; Starke et al., 2021) have in common is that they have identified and characterized the role of the temperature in determining organismal stoichiometry from a population, community, or whole-organism perspective. They have hypothesized that temperature-dependent processes occurring at a subcellular level, such as metabolism, play a key role in driving organismal stoichiometry at global scales, highlighting the benefit of incorporating omics data into ecological stoichiometry.
Omics data have been successfully integrated into lab- and field studies of ecological stoichiometry through the measurement of bulk pools of key metabolites in response to resource supply and other environmental drivers (Singh and Tiwari, 2000; Wagner and Frost, 2012; Zhang et al., 2022). These experiments have provided vital insights into the molecular processes determining organismal stoichiometry. For example, metabolomic analysis of a marine dinoflagellates demonstrated that the allocation of key macromolecular precursors, such as amino acids (i.e., N-rich) and soluble sugars (i.e., C-rich), are highly temperature-dependent, with increased allocation to C-pools (sugars) at high temperatures and increased allocation to N-pools (amino acids) at low temperatures (Singh and Tiwari, 2000; Wagner and Frost, 2012; Zhang et al., 2022). The enzyme alkaline phosphatase has been found to play a key role in cellular responses to varying phosphorus supply in both planktonic microbes (Singh and Tiwari, 2000) and metazoans (Wagner and Frost, 2012).
While invaluable, a drawback of quantifying bulk metabolite pools experimentally is the limited ability to study dynamic fluxes of metabolites. This can be achieved at a population level through sampling of the population at discrete time intervals, in a chemostat for example, but these measurements are complicated by potential effects of dilution on population stoichiometry (Klausmeier et al., 2004b) and still represent only snapshots in time rather a truly dynamic view of metabolite flux. Carrying out metabolomic analysis on individual cells meanwhile can only produce a single static measurement of metabolite concentration and composition under a single set of conditions.
In silico modeling approaches that integrate omics data into stoichiometric models allow for the dynamic quantification of metabolites and biomass composition, even at the level of an individual cell. These omic- and stoichiometric-explicit models have provided fascinating insights into the highly temporally dynamic nature of phytoplankton stoichiometry (Hagstrom et al., 2024; Omta et al., 2024). Moreover, they have suggested a potential hypothesis to account for discrepancies in field observations of phytoplankton, such as those observed in North Atlantic N:C stoichiometry datasets (Sauterey and Ward, 2022).
When developing models that integrate stoichiometry and omics, cellular physiology can be considered from (at least) two different perspectives: metabolic fluxes and macromolecular allocations. On one hand, a phytoplankton cell is a machine consisting of thousands of reactions that maintain the cell and eventually produce new biomass. Most reactions are mediated by enzymatic activities, and their presence can be informed by the presence of a gene, its transcript, or, more importantly, the protein itself. While this reaction-centric approach is a view highly focused on metabolic fluxes, the macromolecular viewpoint focuses on the net outcome of these fluxes: the amounts and relative allocations of different macromolecules. The need for a robust model integrating omics and stoichiometry for phytoplankton arises from the limitations of our current understanding of physiological responses and microbial contributions to nutrient dynamics in marine ecosystems. Models have been developed that embrace these two different perspectives but do so separately (Figure 2). Genome-scale metabolic modeling (GSM) reconstructs a detailed metabolic network based on `omics information, informing about the presence and absence of each metabolic step. The key in such models is the choice of the objective function. Typically this is the biomass objective function (Feist and Palsson, 2010). In GSMs, biomass stoichiometry must be predefined and is typically represented as a fixed composition of macromolecules in terms of their elemental components like C:N:P. This fixed representation of stoichiometry means that GSMs cannot inherently capture changes in the elemental composition of biomass that may occur under different environmental conditions or nutrient availability. Flux balance analysis (FBA) complements GSM by solving a linear function that is used to find the optimal distribution of metabolic fluxes that satisfy all the constraints, such as mass balance, and optimize a particular objective, such as maximizing biomass production. With the biomass stoichiometry given, FBA can predict fluxes for the precursors of macromolecules. The stoichiometric coefficients of each reaction constrain the flow of metabolites through the GSM in two ways. First, system boundaries are established to ensure that, at steady state, the total production of any given compound is equal to its total consumption. Second, each reaction is assigned upper and lower bounds that define the maximum and minimum allowable fluxes. These checks and balances determine the range of possible flux distributions within the system, specifying the rates at which metabolites are produced and consumed by each reaction. It is essential that these rates are physiologically relevant (Orth et al., 2010; Fang et al., 2020). FBA is highly standardized and is increasingly used as a tool in the analysis of `omics data (e.g. Nishimura and Yoshizawa, 2022; Paoli et al., 2022) and has proven useful in the bioengineering world, where models of phytoplankton and other microbes have been used extensively (e.g., Nambou et al., 2015; Banerjee et al., 2016; Vikromvarasiri et al., 2023) to help identify optimal growth parameters and biomass production for economically important species.
Figure 2. Schematic of MAGMA. Two complementary models for simulating phytoplankton physiology and C:N:P stoichiometry and how these may be integrated toward MAGMA. Left and right schematic describes the macromolecular model and detailed metabolic model respectively. The bullet points describe complementary features of each model. In the macromolecular model schematic, arrows describe metabolic fluxes and boxes represent pools of molecules and elements, except that the green box represents a cell. In the schematic of the detailed metabolic model, nodes represent metabolite and lines represent metabolic fluxes. The black arrows show information provided from one model to the other, demonstrating how these two models may complement each other toward MAGMA. Source: Right panel adapted from Inomura et al. (2020b) under CC BY 4.0 license.
FBA, however, treats the cell as a discrete unit, which limits the ability of the model to communicate with the external environment and reduces our ability to incorporate and explore ecologically relevant relationships and feedback between the cell and the ecosystem. To allow FBA to accommodate a range of environmental conditions, a conditional version of FBA (cFBA) was developed (Rügen et al., 2015). This introduces discrete time intervals into the model cycle, during which flux distributions can be captured without optimization. The approach was developed initially to study the effects of varying light availability in cyanobacteria, but has since been adapted to predict the impact of temperature on metabolic fluxes (Páez-Watson et al., 2023).
Macromolecular models resolve and simulate the actual mass and allocation of macromolecules in phytoplankton (Figure 2). There are a variety of methods and most of these are based on empirically informed equations (e.g., Inomura et al., 2020a, 2022; Omta et al., 2020; Sullivan et al., 2024). Given the fixed elemental stoichiometry of macromolecules, modeling of macromolecular allocation can readily lead to modeling of elemental stoichiometry of the entire cell. While macromolecular models typically do not resolve detailed metabolic fluxes, they do include key metabolic processes such as photosynthesis, respiration, and growth that are related to macromolecular allocation. This simplicity increases computational efficiency, allowing these models to be run at the scale of the global ocean (e.g., Inomura et al., 2022; Sullivan et al., 2024). Macromolecular models may interact with the external environment, as embedded in the ocean simulation (Inomura et al., 2022) and provide empirically supported physiological acclimation. However, macromolecular models alone do not typically represent the detailed metabolic fluxes that can be informed by the vast accumulation of ‘omics data.
To integrate these two approaches, we envision a combination that entails a stoichiometrically explicit model of macromolecular allocations with a genome-informed quantitative kinetic FBA: MAGMA (Macromolecular Allocation and Genome-scale Metabolic Analysis) (Figure 2).The first step in developing such an integrated model is to use a macromolecular model to predict elemental stoichiometry based on the typical elemental stoichiometry of each macromolecule based on environmental factors (light, nutrients) (e.g., Inomura et al., 2020a). In the second step, the predicted elemental stoichiometry is used to constrain the FBA. This will allow the FBA to predict metabolic fluxes with varying elemental stoichiometry under various environmental conditions. The FBA can provide the allocation of precursor molecules for various macromolecular allocations, which can then be compared with the output of a macromolecular allocation model (Figure 2). In Table 1, we demonstrate how integrating omics data into macromolecular allocation modeling may be used to test hypotheses about the role of temperature-dependent subcellular processes in determining phytoplankton stoichiometry ratios in the ocean (e.g.,Yvon-Durocher et al., 2015).
Table 1. Hypothesized effects of warming on C:N:P allocation to major macromolecular pools (lipids, carbohydrates, and proteins) and description of MAGMA-based hypothesis test.
It is important to note that FBA models, like other “omics-based models,” (e.g., Antoniewicz, 2020; Faure et al., 2021; McDaniel et al., 2022) are subject to substantial uncertainty. This is due to the fact that they are only as powerful as the available omics resources used to construct them. A significant number of phytoplankton genomes and metagenomes are incomplete, with a considerable number of metabolic reactions remaining unassigned. Additionally, there are species-specific variations and functions that have yet to be characterized (Lv et al., 2015; Faure et al., 2021; Faria et al., 2023). A number of resources are available for this purpose, including Prodigal (Hyatt et al., 2010), Prokka (Seemann, 2014), MetaGeneAnnotator (Noguchi et al., 2008), and GeneMark.hmm (Lukashin and Borodovsky, 1998), which can predict the functional annotation of uncharacterized genes by making comparisons to curated, characterized genomes. However, experimental work, such as knock-out studies (e.g., Schroer et al., 2023), is required to fully characterize these reactions and their variations. The MAGMA approach may prove beneficial in improving the accuracy of functional annotations and informing hypothesis formation in experimental annotation studies. It offers a biological context, in the form of mechanistic simulations, for understanding the behavior of these genes, their associated proteins, and the pathways in which they are involved, under varying resource supply and macromolecule production conditions. Because it includes extensive sets of equations for mechanistically simulating macromolecular allocation, the MAGMA approach can be differentiated from a recent integrative study (Casey et al., 2022), which predicts macromolecular allocation based on an optimization search bounded by empirical data.
Despite the exponential growth of genomic data, the availability of high-quality GSMs is limited for most microbial taxa. This is especially true for aquatic microorganisms. The intricate nature of microbial metabolism necessitates the development of de novo GSMs (Thiele and Palsson, 2010; Norsigian et al., 2020). The BiGG database (King et al., 2016), a prominent repository for GSMs, currently includes a model for the marine diatom Phaeodactylum tricornutum CCAP 1055/1 (Levering et al., 2016). In addition, GSMs have been developed for other microorganisms, including freshwater cyanobacteria such as Synechococcus elongatus PCC 7942 (Broddrick et al., 2016) and Synechocystis sp. PCC 6803 (Nogales et al., 2012), as well as the freshwater alga Chlamydomonas reinhardtii (Chang et al., 2011). In addition to the BiGG database, other marine GSMs include Synechococcus sp. PCC 7002 (Qian et al., 2017), Prochlorococcus MED4 (Ofaim et al., 2021), Synechococcus sp. PCC 11901 (Ravindran et al., 2024). and the marine diatom Thalassiosira pseudonana CCMP 1335 (van Tol and Armbrust, 2021). Other examples of freshwater cyanobacteria include Synechococcus sp. UTEX 2973 (Mueller et al., 2017), Arthrospira platensis NIES-39 (Yoshikawa et al., 2015), and Nostoc sp. PCC 7120 (Norena-Caro et al., 2021). Cyanobacteria-specific models are particularly challenging because of the vast number of genes that remain unidentified or hypothetical (Lv et al., 2015). Consequently, to function they require a comprehensive process of gap-filling reactions (Faria et al., 2023).
More recently, the use of GSMs for marine picocyanobacteria, such as Prochlorococcus, has facilitated a deeper understanding of their metabolic processes and ecological role within the global ocean. Marine picocyanobacteria Prochlorococcus and Synechococcus are estimated to constitute approximately 10% of the total marine picoplankton population in the upper 200m of the world’s oceans, contributing up to 25% of the ocean’s net primary productivity (Flombaum et al., 2013). Furthermore, their C:P and N:P ratios can span a range exceeding one order of magnitude larger than that observed in other phytoplankton taxa in the marine environment (Garcia et al., 2016).
Using an extended genome-scale metabolic model of Prochlorococcus strain MED4 coupled with FBA, Ofaim et al. (2021) investigated the relationships among key nutrients (C, N, P, and light), carbon storage, and excretion, in both static and dynamic settings. Under N-limited conditions, glycogen storage and organic acid exudation were favored, whereas amino acid exudation was the dominant process under P-limitation. Building upon this methodology, Casey et al. (2022) constructed a pan-GSM from 69 Prochlorococcus isolates to investigate strain-specific dynamics across an Atlantic Ocean transect (Casey et al., 2022). By optimizing the pan-GSM to align with local physical and chemical conditions, the authors were able to predict strain-specific growth rates, metabolic configurations, and niche adaptations. These predictions were then linked to the observed ecotype abundances and ecosystem organization. Most recently in a preprint by Régimbeau et al. (2023), the Prochlorococcus strain MED4 GSM (Ofaim et al., 2021) was integrated into Earth System Models (ESMs) to bridge the gap between current ESMs understanding of nutrient limitations, phenotypic, traits, and the available genome-centered information (Régimbeau et al., 2023). By incorporating the metabolic diversity of Prochlorococcus, the study explores the impact of nutritional constraints on phytoplankton physiology and biogeochemical functions, emphasizing the potential of genome-enabled ESMs to more accurately quantify contributions to dissolved organic carbon production.
The creation of a single-celled stoichiometric mass balance model of these major primary producers would assist in elucidating the influence of changes in nutrient availability on cellular processes, elemental composition, and growth, which in turn impact global carbon, nitrogen, and phosphorus budgets. Accordingly, this proof-of-concept MAGMA model could be applied to other ecologically important organisms and used in disparate ecosystems.
We next present a methodology for developing a joint GSM-macromolecular allocation model, MAGMA, for the cyanobacterium Parasynechococcus marenigrum (formerly known as Synechococcus sp. WH8102), to represent one of the most abundant phytoplankton genera in the ocean (Coutinho et al., 2016; Garcia et al., 2016). This organism constitutes a major and pervasive component of marine ecosystems (Figure 3A), inhabiting a range of habits from coastal to pelagic waters from subtropical to high latitudes (Bertilsson et al., 2003; Rost et al., 2003). Furthermore, a substantial body of research has evaluated the elemental stoichiometry of P. marenigrum’s under diverse nutrient conditions, making it an optimal model organism for assessing the feasibility of our MAGMA approach (Su et al., 2006; Tetu et al., 2009; Kretz et al., 2015; Mouginot et al., 2015; Garcia et al., 2016). The WH8102 strain has been established as a model organism due to the availability of its fully sequenced genome and its capacity for genetic manipulation (Palenik et al., 2003). As indicated by the Genome Taxonomy Database (GTDB), the species cluster for P. marenigrum WH8012 encompasses seven high-quality (Parks et al., 2022) representative marine genomes from disparate geographical regions (Figure 3A). The remaining representative genomes of this cluster include: WH8103, which was isolated from the Sargasso Sea; A18-40 and A18-46.1, which were isolated in the Atlantic Ocean; BOUM118, which was isolated from the Mediterranean Sea; RS9915, which was isolated from the Indian Ocean; and YX04-3, which was isolated in the South China Sea. The type strain of the species is WH8102, a strain isolated from the Sargasso Sea in the Atlantic Ocean. The creation of a P. marenigrum pan-GSM from these seven genomes would be advantageous for the purpose of capturing the diverse functions exhibited by the organisms across different marine environments. However, there is currently no GSM resource available for WH8102, nor for a closely related cyanobacteria GSM, as their average nucleotide identities (ANI) are less than 97% similar (Figure 3B).
Figure 3. Comparison of Parasynechococcus marenigrum WH8102 distribution and GSM. (A) Isolation location of P. marenigrum strains mapped onto global ocean N:P of total phytoplankton biomass. (B) Average nucleotide identity (ANI) between P. marenigrum strains and current existing GSMs of freshwater cyanobacteria S. elongatus PCC 7942 and Synechocystis sp. PCC6803, and marine Prochlorococcus MED4. (C) Unique and shared KEGG reactions between the WH8102 draft pan-GSM and S. elongatus PCC 7942 and Synechocystis sp. PCC6803. Source: (A) adapted from Inomura et al. (2022) under CC BY 4.0 license.
Seven high-quality genomes representing the P. marenigrum species cluster were obtained from the GTDB, release 220 (Parks et al., 2022). The ANI was determined in relation to strain WH8102 using fastANI (Jain et al., 2018). The genomes of the P. marenigrum strains exhibited ANI values exceeding 97% (with an average of 98.37% ± 0.46%), as indicated in Supplementary Table S1. These values were observed in comparison to the reference genome of P. marenigrum WH8102 (Supplementary Table S1). The genomes were annotated with Prokka (Seemann, 2014) using the default settings and with -genus Synechococcus. The average size of the seven genomes was 2.42 Mb ± 0.047 Mb, with an average of approximately 2,690 ± 46 proteins.
A pangenome-scale metabolic model was constructed using gapseq v. 1.3.1 (Zimmermann et al., 2021) with the ‘doall’ command using the protein fasta file derived from all genomes. Reaction identifiers and names that were absent from the data set were manually curated from the Kyoto Encyclopedia of Genes and Genomes (KEGG) (Kanehisa et al., 2021), BiGG (King et al., 2016), and ModelSeed (Seaver et al., 2020) databases.
To build on the basic pangenome-scale model and produce a model that can accurately carry flux through the network to produce realistic estimates of biomass production, macromolecular composition, and biomass stoichiometry, a multi-stage workflow that involves multiple rounds of literature review, gap filling, refinement and validation is necessary (Figure 4) (Thiele and Palsson, 2010; Knoop et al., 2013; Norsigian et al., 2020). The existence of other balanced and experimentally validated cyanobacterial GSMs, such as iJN678 for Synechocystis PCC6803 (Knoop et al., 2013) and iJB785 for Synechococcus PCC7942 (Broddrick et al., 2016), provides an excellent foundation for the construction of the WH8102 model, given that they are all within the Synechococcales order (Trautmann et al., 2012). However, the global distribution of the Synechococcales in marine and freshwater environments (Callieri et al., 2013; Dvořák et al., 2014) suggests that they likely differ significantly in their nutrient utilization and metabolic pathways. Indeed, considerable variation is evident not only in their genetic similarity (Figure 3B) but also metabolically (Figures 3C, 5A).
Figure 4. Flow chart showing the suggested workflow for building and tuning the pan-WH8102 GSM ready for use in cFBA. We approximate 6-8 months to complete the workflow, depending on the availability of necessary data and amount of QA/QC required.
Figure 5. (A) Comparison of biomass composition as macromolecular precursors between the P. marenigrum WH8102 pangenome, S. elongatus PCC 7942, Synechocystis sp. PCC6803, and Prochlorococcus sp. MED4 GSM. (B) Biomass precursor C:N:P stoichiometry and total biomass C:N:P stoichiometry estimated from GSM metabolite formulas and reaction stoichiometry for the three published models.
While our proposed integrated modeling approach will facilitate this process by establishing realistic bounds for macromolecular allocation and biomass composition, additional detailed information is required regarding the specific metabolites and reactions from which the macromolecules are produced.
A comparison of the Synechocystis PCC6803 (iJN678), Synechococcus elongatus PCC7942 (iJB785) and Prochlorococcus MED4 (iJC581) models’ metabolic pathways (using KEGG IDs) with our WH8102 pangenome model reveals that approximately 19% of the metabolic reactions present in the models are shared among all four of the strains/strain clusters (Figure 3C). Furthermore, additional pairwise overlaps of metabolic reactions were observed between the strains, representing approximately 10% to 20% of the reactions. Additionally, the Synechocystis PCC6803, Synechococcus PCC7942, and Prochlorococcus MED4 models exhibited approximately 2 to 20% of reactions that were exclusive to their respective models. In contrast, the WH8102 pan genome model displayed a higher proportion of unique metabolic reactions, with approximately 27% of its reactions not shared by either of the other strains. This reflects significant variations in habitat, as well as in the acquisition, use, and storage of carbon, nitrogen, and phosphorus (Figures 5A, B). These differences must be taken into account when developing the pan-WH8102 model and constructing the cluster-specific biomass objective function. For instance, Synechocystis PCC6803 is a facultative photoautotroph whereas Synechococcus PCC7942 and WH8102 are both obligate photoautotrophs (Palenik et al., 2003; Six et al., 2004; Billis et al., 2014). This distinction implies that the iJN678 biomass objective function is, in fact, composed of three discrete biomass reactions, representing three distinct trophic states and carbon metabolic pathways for autotrophy, heterotrophy, and mixotrophy. Accordingly, the iJB785 model would be the more appropriate model to inform the construction of the photosynthetically-dependent components of the biomass objective function for pan-WH8102, although key differences in photophysiology would also need to be considered.
Freshwater strains of cyanobacteria, such as Synechococcus PCC 7942, possess the ability to adjust the ratios of phycocyanin and phycoerythrin in their pigment composition in response to changes in light color. In contrast, the marine P. marenigrum WH8102 strain is unable to adapt its pigment composition in response to changes in light color, and instead exhibits a pigment composition similar to other marine strains, which possess unique phycobilisome components not found in freshwater species.
These components include R-phycocyanin II and two forms of phycoerythrin (PEI and PEII). Marine strains also exhibit a higher light tolerance, which allows them to adapt to the varying light conditions present in the deep, oligotrophic ocean (Six et al., 2004). This is in contrast to Prochlorococcus MED4, which is adapted to high light levels at the ocean surface (Steglich et al., 2006) and has a unique pigment composition (Partensky et al., 1999). It is therefore essential to exercise caution with regard to the light- and pigment-dependent aspects of the model, including the pigment composition specified in the final biomass objective function.
In addition to differences in photophysiology, key differences in nutrient metabolism will also need to be addressed because the strategies employed for the acquisition, processing, and allocating of nitrogen and phosphorus likely reflect the scarcity observed in the oligotrophic ocean in comparison to fresh and coastal waters. Indeed, the P. marenigrum strain cluster used in our pangenome model inhabits the nutrient-poor open ocean, a habitat also shared by Prochlorococcus MED4 while the freshwater (Synechocystis sp 6803, Synechococcus sp. 7942) and brackish/coastal (Synechococcus sp. 7002) species for which GSMs have been developed are adapted to shallower, nutrient-rich coastal and inland waters.
The open ocean strains (MED4 and WH8102) lack the ability to fix nitrogen and instead rely on urea as their primary N source, with occasional NOx/NH3 inputs (Palenik et al., 2003). They primarily scavenge for inorganic P with high-affinity phosphate-binding proteins (Ranjit et al., 2024). MED4 on the other hand is unable to utilize NOx and is entirely reliant on urea and NH4 as N sources (García-Fernández et al., 2004). The utilization of urea as a primary nitrogen source renders these marine strains susceptible to limitation by nickel, which is a vital component of Ni-containing urease (Dupont et al., 2008). Furthermore, compelling genomic evidence indicates that the WH8102 strain in particular has obligate requirements for Ni even when obtaining N as NOx or NH4 (Dupont et al., 2008). While there are notable similarities between MED4 and WH8102 as open ocean species adapted to oligotrophic environments (Partensky et al., 1999), WH8102 is more tolerant of varying nutrient conditions (Palenik et al., 2003) whereas MED4 is more specifically adapted to low nutrient conditions (Rocap et al., 2003). This has resulted in MED4 having a considerably smaller and more streamlined genome and metabolism with a paucity of regulatory genes (Dufresne et al., 2003; García-Fernández et al., 2004).
Finally, in order to study temperature-dependent effects using a constrained FBA, it is necessary to identify biologically and thermodynamically (or enzymatically and energetically) realistic temperature-dependent responses in the metabolic reactions and formulation of biomass. This then would allow the reaction bounds of the model to be constrained in a way that is consistent with the observed responses. The current temperature-dependent cFBA models for microbes were developed for polyphosphate- and glycogen-accumulating organisms (PAO and GAO) in wastewater (Lopez-Vazquez et al., 2009). The temperature coefficients may be of broad relevance in providing thermodynamically reasonable bounds. However, metabolism and allocation of resources of the organisms in question differ substantially. Integration with the macromolecular allocation model would be advantageous in this area. While it is not feasible to adjust temperature-coefficients for every reaction, temperature-based macromolecular data could serve as an independent dataset for validating the cFBA predictions and could be employed to develop broader level temperature constraints on biomass reaction formation, growth, photosynthetic reactions, uptake and assimilation rates, and cellular maintenance. In other words, the data can be combined to validate energetic constraints on select reactions/pathways using more general values, and species-specific macromolecular allocation data can be used to validate temperature-dependent biological constraints.
A comprehensive understanding of the effects of global change on the oceans and their biogeochemical cycles requires an examination of these effects at all levels of biological organization. A considerable proportion of the currently unknown or poorly characterized effects of temperature on nutrient flux (Figure 1) occur at the individual and molecular level. These instances underscore the knowledge gap that our MAGMA model would help to bridge, helping to understand how microbes evolve resource allocation strategies in response to shifting environmental pressures and providing insight into the metabolic adaptations that drive ecological and evolutionary dynamics.
The combined model in MAGMA has the potential to advance various fields, including, microbiology, ecology, biogeochemistry and global change sciences. This integration allows the metabolic network to operate within physiological and ecological constraints that are responsive to environmental conditions (such as nutrient availability and temperature), as opposed to utilizing fixed or arbitrary constraints. This integration thus bridges the gap between whole-cell stoichiometry (addressed by the macromolecular model) and detailed metabolic pathways (captured by FBA), allowing us to connect cellular-scale elemental allocation patterns to specific metabolic fluxes. This provides a more comprehensive understanding of cellular stoichiometry in response to environmental changes and leads to more reliable estimates of growth rates, nutrient uptake, and byproduct formation under different scenarios.
Furthermore, MAGMA would also allow the examination of how trade-offs at the macromolecular level (e.g., allocation to proteins vs. lipids) are reflected in specific metabolic trade-offs (e.g., energy allocation to different biosynthetic pathways) and elucidate the mechanisms by which environmental changes (such as warming or nutrient limitation) impact cellular composition. For example, one could trace how a change in P availability affects lipid allocation in the macromolecular model, and then see how this cascades through various metabolic pathways in the FBA. Alternatively, we could explore how changes in protein:lipid ratios under different nutrient regimes affect energy metabolism or nutrient acquisition capabilities. By predicting the impact of environmental conditions on cellular composition and metabolism in more detail, this integrated approach could provide more realistic inputs for ecosystem-level models of nutrient cycling and energy flow.
The MAGMA framework is sufficiently general to accommodate a range of ‘omics data. For instance, genomic and metagenomic data may offer a suite of community members to be analyzed with MAGMA to link inputs and outputs of resources shared across an ecosystem. Likewise, information from closely related species and/or strains can be used to see how genomic variations, across spatial scales, manifest into observable phenotypic traits, such as nutrient uptake rates or stress tolerance. Transcriptomic, metabolomic, lipidomic, and proteomic data will provide more precise measurements of expression regulation and actual metabolic fluxes. These are crucial components in understanding the “bio” in biogeochemical cycling, as well as in understanding how these processes respond to changing environments.
We note that MAGMA need not be applied only to steady-state systems but preferably to dynamic ones. For instance, if fluctuations of environmental parameters such as light and nutrients are known, these may be incorporated into the model to predict time-varying macromolecular allocation, elemental stoichiometry, growth rate, and metabolic fluxes. Both macromolecular (Omta et al., 2024) and FBA (Höffner et al., 2013; Gomez et al., 2014) models have dynamic versions, and these frameworks may be applied to MAGMA to accommodate the dynamic nature of the environment and cellular responses to it. Moreover, time-variant ‘omics data may enable MAGMA to progressively narrow down the potential fluxes for different time points. The resulting macromolecular allocation and elemental stoichiometry may be compared with time-variant data for these parameters.
Moreover, once implemented in comprehensive ocean simulations, MAGMA offers a distinctive instrument for examining a range of significant unresolved biogeochemical questions. For example, elucidating which factors contribute to the maintenance of deep ocean N:P:O2 ratios approaching the Redfield ratio (Gruber and Deutsch, 2014). Additionally, it would be beneficial to ascertain the resilience of this to anthropogenic perturbations, such as the application of fertilizers. The MAGMA model may facilitate an investigation into the potential regulation of the stoichiometry of export production at the ecophysiological level and the extent to which it may contribute to the resilience of deep ocean stoichiometry under anthropogenic influences.
To build MAGMA, biochemical and metabolic data are essential. The useful measurements include, elemental mass and ratios, macromolecular measurements, metabolic rates, especially major ones including carbon fixation, respiration, and nutrient uptake. At the same time, environmental data are highly informative, as they help the model to pin down the relationship between biochemistry and environment. These data include temperature, nutrient concentration, concentration of other inorganic molecules (e.g., O2) and light intensity. These environmental parameters may also inversely estimate elemental stoichiometry related values (e.g., DeVries and Deutsch, 2014). Ultimately, MAGMA will facilitate the linkage between theoretical, experimental, and observational studies to provide valuable insights into the impacts of global change on marine ecosystems, particularly in terms of species-specific responses, complex feedbacks, and the potential for nutrient imbalances.
CLCJ: Conceptualization, Data curation, Formal analysis, Investigation, Methodology, Project administration, Resources, Supervision, Validation, Visualization, Writing – original draft, Writing – review & editing. JC-C: Data curation, Investigation, Methodology, Project administration, Validation, Visualization, Writing – original draft, Writing – review & editing. MS: Data curation, Investigation, Methodology, Project administration, Validation, Visualization, Writing – original draft, Writing – review & editing. MSS: Data curation, Formal analysis, Investigation, Methodology, Project administration, Resources, Software, Validation, Visualization, Writing – original draft, Writing – review & editing. KI: Conceptualization, Data curation, Formal analysis, Investigation, Methodology, Project administration, Validation, Visualization, Writing – original draft, Writing – review & editing.
The author(s) declare financial support was received for the research, authorship, and/or publication of this article. This work was supported by the Simons Foundation (LS-ECIAMEE-00001549 to KI) and the U.S. National Science Foundation (OCE-2048373, subaward SUB0000525 from Princeton University to KI). MSS is supported by the NASA Astrobiology Postdoctoral Program, administered by Oak Ridge Associated Universities under contract with NASA. CLCJ is supported by the EPA’s Great Lakes Restoration Initiative (GLRI). MS is supported by the German Research Foundation (DFG, 379417748; research unit, FOR 2716). We thank these foundations for their support.
We thank the AWI for providing access to the guest research facilities of the Biologische Anstalt Helgoland during the Woodstoich Workshop, grant number AWI_BAH_34. We thank everyone who supported and enabled Woodstoich 5 workshop, especially Cédric Meunier and Maarten Boersma for organizing a productive and groovy event for early career researchers. We thank Jim Elser for his constructive feedback on the manuscript and mentorship through the process. We thank Eric Pelletier for useful discussions and Dieter Wolf-Gladrow for insightful comments on the manuscript drafts. We thank the reviewers, whose comments and suggestions led to substantial improvements on this manuscript.
The authors declare that the research was conducted in the absence of any commercial or financial relationships that could be construed as a potential conflict of interest.
All claims expressed in this article are solely those of the authors and do not necessarily represent those of their affiliated organizations, or those of the publisher, the editors and the reviewers. Any product that may be evaluated in this article, or claim that may be made by its manufacturer, is not guaranteed or endorsed by the publisher.
The Supplementary Material for this article can be found online at: https://www.frontiersin.org/articles/10.3389/fevo.2024.1505025/full#supplementary-material
Antoniewicz M. R. (2020). A guide to deciphering microbial interactions and metabolic fluxes in microbiome communities. Curr. Opin. Biotechnol. 64, 230–237. doi: 10.1016/j.copbio.2020.07.001
Armin G., Inomura K. (2021). Modeled temperature dependencies of macromolecular allocation and elemental stoichiometry in phytoplankton. Comput. Struct. Biotechnol. J. 19, 5421–5427. doi: 10.1016/j.csbj.2021.09.028
Banerjee C., Singh P. K., Shukla P. (2016). Microalgal bioengineering for sustainable energy development: Recent transgenesis and metabolic engineering strategies. Biotechnol. J. 11, 303–314. doi: 10.1002/biot.201500284
Bertilsson S., Berglund O., Karl D. M., Chisholm S. W. (2003). Elemental composition of marine Prochlorococcus and Synechococcus: Implications for the ecological stoichiometry of the sea. Limnol. Oceanogr. 48, 1721–1731. doi: 10.4319/lo.2003.48.5.1721
Bi R., Arndt C., Sommer U. (2012). Stoichiometric responses of phytoplankton species to the interactive effect of nutrient supply ratios and growth rates(1). J. Phycol. 48, 539–549. doi: 10.1111/j.1529-8817.2012.01163.x
Billis K., Billini M., Tripp H. J., Kyrpides N. C., Mavromatis K. (2014). Comparative transcriptomics between Synechococcus PCC 7942 and Synechocystis PCC 6803 provide insights into mechanisms of stress acclimation. PloS One 9, e109738. doi: 10.1371/journal.pone.0109738
Bouman H. A., Ulloa O., Scanlan D. J., Zwirglmaier K., Li W. K. W., Platt T., et al. (2006). Oceanographic basis of the global surface distribution of Prochlorococcus ecotypes. Science 312, 918–921. doi: 10.1126/science.1122692
Broddrick J. T., Rubin B. E., Welkie D. G., Du N., Mih N., Diamond S., et al. (2016). Unique attributes of cyanobacterial metabolism revealed by improved genome-scale metabolic modeling and essential gene analysis. Proc. Natl. Acad. Sci. U. S. A. 113, E8344–E8353. doi: 10.1073/pnas.1613446113
Callieri C., Coci M., Corno G., Macek M., Modenutti B., Balseiro E., et al. (2013). Phylogenetic diversity of nonmarine picocyanobacteria. FEMS Microbiol. Ecol. 85, 293–301. doi: 10.1111/femsec.2013.85.issue-2
Casey J. R., Boiteau R. M., Engqvist M. K. M., Finkel Z. V., Li G., Liefer J., et al. (2022). Basin-scale biogeography of marine phytoplankton reflects cellular-scale optimization of metabolism and physiology. Sci. Adv. 8, eabl4930. doi: 10.1126/sciadv.abl4930
Casey J. R., Mardinoglu A., Nielsen J., Karl D. M. (2016). Adaptive evolution of phosphorus metabolism in Prochlorococcus. mSystems 1, 10.1128/msystems.00065-16. doi: 10.1128/mSystems.00065-16
Chang R. L., Ghamsari L., Manichaikul A., Hom E. F. Y., Balaji S., Fu W., et al. (2011). Metabolic network reconstruction of Chlamydomonas offers insight into light-driven algal metabolism. Mol. Syst. Biol. 7, 518. doi: 10.1038/msb.2011.52
Coutinho F. H., Dutilh B. E., Thompson C. C., Thompson F. L. (2016). Proposal of fifteen new species of Parasynechococcus based on genomic, physiological and ecological features. Arch. Microbiol. 198, 973–986. doi: 10.1007/s00203-016-1256-y
DeVries T. (2018). New directions for ocean nutrients. Nature Geosci. 11, 15–16. doi: 10.1038/s41561-017-0042-z
DeVries T., Deutsch C. (2014). Large-scale variations in the stoichiometry of marine organic matter respiration. Nat. Geosci. 7, 890–894. doi: 10.1038/ngeo2300
Dufresne A., Salanoubat M., Partensky F., Artiguenave F., Axmann I. M., Barbe V., et al. (2003). Genome sequence of the cyanobacterium Prochlorococcus marinus SS120, a nearly minimal oxyphototrophic genome. Proc. Natl. Acad. Sci. U. S. A. 100 (17), 10020–10025. doi: 10.1073/pnas.1733211100
Dupont C. L., Barbeau K., Palenik B. (2008). Ni uptake and limitation in marine Synechococcus strains. Appl. Environ. Microbiol. 74, 23–31. doi: 10.1128/AEM.01007-07
Dvořák P., Casamatta D. A., Poulíčková A., Hašler P., Ondřej V., Sanges R. (2014). Synechococcus: 3 billion years of global dominance. Mol. Ecol. 23, 5538–5551. doi: 10.1111/mec.12948"10.1111/mec.12948
Fang X., Lloyd C. J., Palsson B. O. (2020). Reconstructing organisms in silico: genome-scale models and their emerging applications. Nat. Rev. Microbiol. 18, 731–743. doi: 10.1038/s41579-020-00440-4
Faria J. P., Liu F., Edirisinghe J. N., Gupta N., Seaver S. M. D., Freiburger A. P., et al. (2023). ModelSEED v2: High-throughput genome-scale metabolic model reconstruction with enhanced energy biosynthesis pathway prediction. bioRxiv 10.04.556561. doi: 10.1101/2023.10.04.556561
Faure E., Ayata S.-D., Bittner L. (2021). Towards omics-based predictions of planktonic functional composition from environmental data. Nat. Commun. 12, 4361. doi: 10.1038/s41467-021-24547-1
Feist A. M., Palsson B. O. (2010). The biomass objective function. Curr. Opin. Microbiol. 13, 344–349. doi: 10.1016/j.mib.2010.03.003
Finkel Z. V., Quigg A., Raven J. A., Reinfelder J. R., Schofield O. E., Falkowski P. G. (2006). Irradiance and the elemental stoichiometry of marine phytoplankton. Limnol. Oceanogr. 51, 2690–2701. doi: 10.4319/lo.2006.51.6.2690
Flombaum P., Gallegos J. L., Gordillo R. A., Rincón J., Zabala L. L., Jiao N., et al. (2013). Present and future global distributions of the marine Cyanobacteria Prochlorococcus and Synechococcus. Proc. Natl. Acad. Sci. U. S. A. 110, 9824–9829. doi: 10.1073/pnas.1307701110
Garcia N. S., Bonachela J. A., Martiny A. C. (2016). Interactions between growth-dependent changes in cell size, nutrient supply and cellular elemental stoichiometry of marine Synechococcus. ISME J. 10, 2715–2724. doi: 10.1038/ismej.2016.50
García-Fernández J. M., de Marsac N. T., Diez J. (2004). Streamlined regulation and gene loss as adaptive mechanisms in Prochlorococcus for optimized nitrogen utilization in oligotrophic environments. Microbiol. Mol. Biol. Rev. 68, 630–638. doi: 10.1128/MMBR.68.4.630-638.2004
Geider R. J., MacIntyre H. L., Kana T. M. (1996). A dynamic model of photoadaptation in phytoplankton. Limnol. Oceanogr. 41, 1–15. doi: 10.4319/lo.1996.41.1.0001
Goldman J. C., Peavey D (1979). Steady-state growth and chemical composition of the marine chlorophyte Dunaliella tertiolecta in nitrogen-limited continuous cultures. G. Appl. Environ. Microbiol. 38, 894–901. doi: 10.1128/aem.38.5.894-901.1979
Gomez J. A., Höffner K., Barton P. I. (2014). DFBAlab: a fast and reliable MATLAB code for dynamic flux balance analysis. BMC Bioinf. 15, 409. doi: 10.1186/s12859-014-0409-8
Grettenberger C. L., Abou-Shanab R., Hamilton T. L. (2024). Limiting factors in the operation of photosystems I and II in cyanobacteria. Microb. Biotechnol. 17, e14519. doi: 10.1111/1751-7915.14519
Gruber N., Deutsch C. A. (2014). Redfield’s evolving legacy. Nat. Geosci. 7, 853–855. doi: 10.1038/ngeo2308
Hagstrom G. I., Stock C. A., Luo J. Y., Levin S. A. (2024). Impact of dynamic phytoplankton stoichiometry on global scale patterns of nutrient limitation, nitrogen fixation, and carbon export. Global Biogeochem. Cycles 38. doi: 10.1029/2023gb007991
Hall E. K., Maixner F., Franklin O., Daims H., Richter A., Battin T. (2011). Linking microbial and ecosystem ecology using ecological stoichiometry: A synthesis of conceptual and empirical approaches. Ecosystems 14, 261–273. doi: 10.1007/s10021-010-9408-4
Herrero A., Flores E., Guerrero M. G. (1985). Regulation of nitrate reductase cellular levels in the cyanobacteria Anabaena variabilis and. Synechocystis FEMS Microbiol. Lett. 26, 21–25. doi: 10.1111/j.1574-6968.1985.tb01559.x
Hochachka P. W., Somero G. N. (1984). Biochemical adaptation (Princeton, New Jersey: Princeton University Press).
Höffner K., Harwood S. M., Barton P. I. (2013). A reliable simulator for dynamic flux balance analysis. Biotechnol. Bioeng. 110, 792–802. doi: 10.1002/bit.v110.3
Hyatt D., Chen G.-L., Locascio P. F., Land M. L., Larimer F. W., Hauser L (2010). Prodigal: prokaryotic gene recognition and translation initiation site identification. J. BMC Bioinf. 11, 119. doi: 10.1186/1471-2105-11-119
Inomura K., Deutsch C., Jahn O., Dutkiewicz S., Follows M. J. (2022). Global patterns in marine organic matter stoichiometry driven by phytoplankton ecophysiology. Nat. Geosci. 15, 1034–1040. doi: 10.1038/s41561-022-01066-2
Inomura K., Deutsch C., Masuda T., Prášil O., Follows M. J. (2020b). Quantitative models of nitrogen-fixing organisms. Comput. Struct. Biotechnol. J 18, 3905–3924.
Inomura K., Omta A. W., Talmy D., Bragg J., Deutsch C., Follows M. J. (2020a). A mechanistic model of macromolecular allocation, elemental stoichiometry, and growth rate in phytoplankton. Front. Microbiol. 11, 86. doi: 10.3389/fmicb.2020.00086
Jain C., Rodriguez-R L. M., Phillippy A. M., Konstantinidis K. T., Aluru S. (2018). High throughput ANI analysis of 90K prokaryotic genomes reveals clear species boundaries. Nat. Commun. 9, 5114. doi: 10.1038/s41467-018-07641-9
Kanehisa M., Furumichi M., Sato Y., Ishiguro-Watanabe M., Tanabe M. (2021). KEGG: Integrating viruses and cellular organisms. Nucleic Acids Res. 49, D545–D551. doi: 10.1093/nar/gkaa970
King Z. A., Lu J., Dräger A., Miller P., Federowicz S., Lerman J. A., et al. (2016). BiGG Models: A platform for integrating, standardizing and sharing genome-scale models. Nucleic Acids Res. 44, D515–D522. doi: 10.1093/nar/gkv1049
Klausmeier C. A., Litchman E., Daufresne T., Levin S. A. (2004a). Optimal nitrogen-to-phosphorus stoichiometry of phytoplankton. Nature 429, 171–174. doi: 10.1038/nature02454
Klausmeier C. A., Litchman E., Levin S. A. (2004b). Phytoplankton growth and stoichiometry under multiple nutrient limitation. Limnol. Oceanogr. 49, 1463–1470. doi: 10.4319/lo.2004.49.4_part_2.1463
Knoop H., Gründel M., Zilliges Y., Lehmann R., Hoffmann S., Lockau W., et al. (2013). Flux balance analysis of cyanobacterial metabolism: the metabolic network of Synechocystis sp. PCC 6803. PloS Comput. Biol. 9(6), e1003081. doi: 10.1371/journal.pcbi.1003081
Kretz C. B., Bell D. W., Lomas D. A., Lomas M. W., Martiny A. C. (2015). Influence of growth rate on the physiological response of marine Synechococcus to phosphate limitation. Front. Microbiol. 6, 85. doi: 10.3389/fmicb.2015.00085
Kromkamp J. (1987). Formation and functional significance of storage products in cyanobacteria. N. Z. J. Mar. Freshw. Res. 21, 457–465. doi: 10.1080/00288330.1987.9516241
Leonardos N., Geider R. J. (2004). Responses of elemental and biochemical composition of Chaetoceros muelleri to growth under varying light and nitrate: phosphate supply ratios and their influence on critical N: P. Limnol. Oceanogr. 49, 2105–2114. doi: 10.4319/lo.2004.49.6.2105
Levering J., Broddrick J., Dupont C. L., Peers G., Beeri K., Mayers J., et al. (2016). Genome-scale model reveals metabolic basis of biomass partitioning in a model diatom. PloS One 11, e0155038. doi: 10.1371/journal.pone.0155038
Liefer J. D., Garg A., Fyfe M. H., Irwin A. J., Benner I., Brown C. M., et al. (2019). The macromolecular basis of phytoplankton C:N:P under nitrogen starvation. Front. Microbiol. 10, 763. doi: 10.3389/fmicb.2019.00763
Loladze I., Elser J. J. (2011). The origins of the Redfield nitrogen-to-phosphorus ratio are in a homoeostatic protein-to-rRNA ratio. Ecol. Lett. 14, 244–250. doi: 10.1111/j.1461-0248.2010.01577.x
Lopez J. S., Garcia N. S., Talmy D., Martiny A. C. (2016). Diel variability in the elemental composition of the marine cyanobacterium Synechococcus. J. Plankton Res. 38, 1052–1061. doi: 10.1093/plankt/fbv120
Lopez-Vazquez C. M., Oehmen A., Hooijmans C. M., Brdjanovic D., Gijzen H. J., Yuan Z., et al. (2009). Modeling the PAO-GAO competition: effects of carbon source, pH and temperature. Water Res. 43, 450–462. doi: 10.1016/j.watres.2008.10.032
Lukashin A. V., Borodovsky M. (1998). GeneMark.hmm: new solutions for gene finding. Nucleic Acids Res. 26, 1107–1115. doi: 10.1093/nar/26.4.1107
Lv Q., Ma W., Liu H., Li J., Wang H., Lu F., et al. (2015). Genome-wide protein-protein interactions and protein function exploration in cyanobacteria. Sci. Rep. 5, 15519. doi: 10.1038/srep15519
MacIntyre H. L., Kana T. M., Anning T., Geider R. J. (2002). Photoacclimation of photosynthesis irradiance response curves and photosynthetic pigments in microalgae and cyanobacteria. J. Phycol. 38, 17–38. doi: 10.1046/j.1529-8817.2002.00094.x
Mai G., Liu J., Xia X., Pang X., Li B., Yu L., et al. (2021). Acutely rising temperature reduces photosynthetic capacity of phytoplankton assemblages in tropical oceans: A large-scale investigation. Front. Mar. Sci. 8, 710697. doi: 10.3389/fmars.2021.710697
Martiny A. C., Pham C. T. A., Primeau F. W., Vrugt J. A., Moore J. K., Levin S. A., et al. (2013). Strong latitudinal patterns in the elemental ratios of marine plankton and organic matter. Nature Geosci 6, 279–283. doi: 10.1038/ngeo1757
Matallana-Surget S., Derock J., Leroy B., Badri H., Deschoenmaeker F., Wattiez R. (2014). Proteome-wide analysis and diel proteomic profiling of the cyanobacterium Arthrospira platensis PCC 8005. PloS One 9, e99076. doi: 10.1371/journal.pone.0099076
McDaniel E. A., van Steenbrugge J. J. M., Noguera D. R., McMahon K. D., Raaijmakers J. M., Medema M. H., et al. (2022). TbasCO: trait-based comparative ’omics identifies ecosystem-level and niche-differentiating adaptations of an engineered microbiome. ISME Commun. 2, 111. doi: 10.1038/s43705-022-00189-2
Moorthi S. D., Schmitt J. A., Ryabov A., Tsakalakis I., Blasius B., Prelle L., et al. (2016). Unifying ecological stoichiometry and metabolic theory to predict production and trophic transfer in a marine planktonic food web. Philos. Trans. R. Soc Lond. B Biol. Sci. 371, 20150270. doi: 10.1098/rstb.2015.0270
Mouginot C., Zimmerman A. E., Bonachela J. A., Fredricks H., Allison S. D., Van Mooy B. A. S., et al. (2015). Resource allocation by the marine cyanobacterium Synechococcus WH8102 in response to different nutrient supply ratios. Limnol. Oceanogr. 60, 1634–1641. doi: 10.1002/lno.10123
Mueller T. J., Ungerer J. L., Pakrasi H. B., Maranas C. D. (2017). Identifying the metabolic differences of a fast-growth phenotype in Synechococcus UTEX 2973. Sci. Rep. 7, 41569. doi: 10.1038/srep41569
Nambou K., Jian X., Zhang X., Wei L., Lou J., Madzak C., et al. (2015). Flux balance analysis inspired bioprocess upgrading for lycopene production by a metabolically engineered strain of Yarrowia lipolytica. Metabolites 5, 794–813. doi: 10.3390/metabo5040794
Nishimura Y., Yoshizawa S. (2022). The OceanDNA MAG catalog contains over 50,000 prokaryotic genomes originated from various marine environments. Sci. Data 9, 305. doi: 10.1038/s41597-022-01392-5
Nogales J., Gudmundsson S., Knight E. M., Palsson B. O., Thiele I. (2012). Detailing the optimality of photosynthesis in cyanobacteria through systems biology analysis. Proc. Natl. Acad. Sci. U. S. A. 109, 2678–2683. doi: 10.1073/pnas.1117907109
Noguchi H., Taniguchi T., Itoh T. (2008). MetaGeneAnnotator: detecting species-specific patterns of ribosomal binding site for precise gene prediction in anonymous prokaryotic and phage genomes. DNA Res. 15, 387–396. doi: 10.1093/dnares/dsn027
Norena-Caro D. A., Zuniga C., Pete A. J., Saemundsson S. A., Donaldson M. R., Adams A. J., et al. (2021). Analysis of the cyanobacterial amino acid metabolism with a precise genome-scale metabolic reconstruction of Anabaena sp. UTEX 2576. Biochem. Eng. J. 171, 108008. doi: 10.1016/j.bej.2021.108008
Norsigian C. J., Fang X., Seif Y., Monk J. M., Palsson B. O. (2020). A workflow for generating multi-strain genome-scale metabolic models of prokaryotes. Nat. Protoc. 15, 1–14. doi: 10.1038/s41596-019-0254-3
O’Donnell D. R., Beery S. M., Litchman E. (2021). Temperature-dependent evolution of cell morphology and carbon and nutrient content in a marine diatom. Limnol. Oceanogr. 66, 4334–4346. doi: 10.1002/lno.11964"10.1002/lno.11964
Ofaim S., Sulheim S., Almaas E., Sher D., Segrè D. (2021). Dynamic allocation of carbon storage and nutrient-dependent exudation in a revised genome-scale model of Prochlorococcus. Front. Genet. 12, 586293. doi: 10.3389/fgene.2021.586293
Olson R. J., Vaulot D., Chisholm S. W. (1986). Effects of environmental stresses on the cell cycle of two marine phytoplankton species. Plant Physiol. 80, 918–925. doi: 10.1104/pp.80.4.918
Omta A. W., Liefer J. D., Finkel Z. V., Irwin A. J., Sher D., Follows M. J. (2024). A model of time-dependent macromolecular and elemental composition of phytoplankton. J. Theor. Biol. 592, 111883. doi: 10.1016/j.jtbi.2024.111883
Omta A. W., Talmy D., Inomura K., Irwin A. J., Finkel Z. V., Sher D., et al. (2020). Quantifying nutrient throughput and DOM production by algae in continuous culture. J. Theor. Biol. 494, 110214. doi: 10.1016/j.jtbi.2020.110214
Orth J. D., Thiele I., Palsson B.Ø. (2010). What is flux balance analysis? Nat. Biotechnol. 28, 245–248. doi: 10.1038/nbt.1614
Páez-Watson T., van Loosdrecht M. C. M., Wahl S. A. (2023). Predicting the impact of temperature on metabolic fluxes using resource allocation modelling: Application to polyphosphate accumulating organisms. Water Res. 228, 119365. doi: 10.1016/j.watres.2022.119365
Pahlow M. (2005). Linking chlorophyll-nutrient dynamics to the RedÞeld N:C ratio with a model of optimal phytoplankton growth. Mar. Ecol. Prog. Ser. 287, 33–43. doi: 10.3354/meps287033
Palenik B., Brahamsha B., Larimer F. W., Land M., Hauser L., Chain P., et al. (2003). The genome of a motile marine Synechococcus. Nature 424, 1037–1042. doi: 10.1038/nature01943
Paoli L., Ruscheweyh H.-J., Forneris C. C., Hubrich F., Kautsar S., Bhushan A., et al. (2022). Biosynthetic potential of the global ocean microbiome. Nature 607, 111–118. doi: 10.1038/s41586-022-04862-3
Parks D. H., ChuvoChina M., Rinke C., Mussig A. J., Chaumeil P. A., Hugenholtz P. (2022). GTDB: An ongoing census of bacterial and archaeal diversity through a phylogenetically consistent, rank normalized and complete genome-based taxonomy. Nucleic Acids Res. 50, D785–D794. doi: 10.1093/nar/gkab776
Partensky F., Hess W. R., Vaulot D. (1999). Prochlorococcus, a marine photosynthetic prokaryote of global significance. Microbiol. Mol. Biol. Rev. 63, 106–127. doi: 10.1128/MMBR.63.1.106-127.1999
Persson J., Fink P., Goto A., Hood J. M., Jonas J., Kato S. (2010). To be or not to be what you eat: regulation of stoichiometric homeostasis among autotrophs and heterotrophs. Oikos 119, 741–751. doi: 10.1111/j.1600-0706.2009.18545.x
Qian X., Kim M. K., Kumaraswamy G. K., Agarwal A., Lun D. S., Dismukes G. C. (2017). Flux balance analysis of photoautotrophic metabolism: Uncovering new biological details of subsystems involved in cyanobacterial photosynthesis. Biochim. Biophys. Acta Bioenerg. 1858, 276–287. doi: 10.1016/j.bbabio.2016.12.007
Ranjit P., Varkey D., Shah B. S., Paulsen I. T. (2024). Substrate specificity and ecological significance of PstS homologs in phosphorus uptake in marine Synechococcus sp. WH8102. Microbiol. Spectr. 12, e02786-23. doi: 10.1128/spectrum.02786-23
Ravindran S., Hajinajaf N., Kundu P., Comes J., Nielsen D. R., Varman A. M., et al. (2024). Genome-scale metabolic model reconstruction and investigation into the fluxome of the fast-growing Cyanobacterium Synechococcus sp. PCC 11901. ACS Synth. Biol. 13(10), 3281-3294. doi: 10.1021/acssynbio.4c00379
Redfield A. C. (1934). On the proportions of organic derivatives in sea water and their relation to the composition of plankton. In James Johnstone Memorial Daniel R. J. (Univ. Press of Liverpool) Vol. 1, 177–192.
Régimbeau A., Aumont O., Bowler C., Guidi L., Jackson G. A., Karsenti E., et al. (2023). Towards modeling genome-scale knowledge in the global ocean. bioRxiv 11.23, 568447. doi: 10.1101/2023.11.23.568447
Rhee G.–U., Gotham I. J. (1981). The effect of environmental factors on phytoplankton growth: Light and the interactions of light with nitrate limitation1. Limnol. Oceanogr. 26, 649–659. doi: 10.4319/lo.1981.26.4.0649
Riley G. A. (1946). Factors controlling phytoplankton populations on Georges Bank 1. J. Mar. Res. 6.
Rocap G., Larimer F. W., Lamerdin J., Malfatti S., Chain P., Ahlgren N. A., et al. (2003). Genome divergence in two Prochlorococcus ecotypes reflects oceanic niche differentiation. Nature 424, 1042–1047. doi: 10.1038/nature01947
Rost B., Liu J., Nair R., Wrzeszczynski K. O., Ofran Y. (2003). Automatic prediction of protein function. Cell. Mol. Life Sci. 60, 2637–2650. doi: 10.1007/s00018-003-3114-8
Rügen M., Bockmayr A., Steuer R. (2015). Elucidating temporal resource allocation and diurnal dynamics in phototrophic metabolism using conditional FBA. Sci. Rep. 5, 15247. doi: 10.1038/srep15247
Sauterey B., Ward B. A. (2022). Environmental control of marine phytoplankton stoichiometry in the North Atlantic Ocean. Proc. Natl. Acad. Sci. U. S. A. 119, e2114602118. doi: 10.1073/pnas.2114602118
Schroer W. F., Kepner H. E., Uchimiya M., Mejia C., Rodriguez L. T., Reisch C. R., et al. (2023). Functional annotation and importance of marine bacterial transporters of plankton exometabolites. ISME Commun. 3, 37. doi: 10.1038/s43705-023-00244-6
Seaver S., Liu F., Zhang Q., Jeffryes J. G., Faria J. P., Edirisinghe J. N., et al. (2020). The ModelSEED Biochemistry Database for the integration of metabolic annotations and the reconstruction, comparison and analysis of metabolic models for plants, fungi and microbes. Nucleic Acids Res. 49, D575–D588. doi: 10.1093/nar/gkaa746"10.1093/nar/gkaa746
Seemann T. (2014). Prokka: rapid prokaryotic genome annotation. Bioinformatics 30, 2068–2069. doi: 10.1093/bioinformatics/btu153
Segura-Noguera M., Blasco D., Fortuño J.-M. (2016). Taxonomic and environmental variability in the elemental composition and stoichiometry of individual dinoflagellate and diatom cells from the NW Mediterranean Sea. PloS One 11, e0154050. doi: 10.1371/journal.pone.0154050
Singh S. K., Tiwari D. N. (2000). Control of alkaline phosphatase activity in Anabaena oryzae Fritsch. J. Plant Physiol. 157, 467–472. doi: 10.1016/S0176-1617(00)80100-5
Six C., Thomas J. C., Brahamsha B., Lemoine Y., Partensky F. (2004). Photophysiology of the marine cyanobacterium Synechococcus sp. WH8102, a new model organism. Aquat. Microb. Ecol. 35, 17–29. doi: 10.3354/ame035017
Smith S. R., Dupont C. L., McCarthy J. K., Broddrick J. T., Oborník M., Horák A., et al. (2019). Evolution and regulation of nitrogen flux through compartmentalized metabolic networks in a marine diatom. Nat. Commun. 10 (4552). doi: 10.1038/s41467-019-12407-y
Starke C. W. E., Jones C. L. C., Burr W. S., Frost P. C. (2021). Interactive effects of water temperature and stoichiometric food quality on Daphnia pulicaria. Freshw. Biol. 66, 256–265. doi: 10.1111/fwb.13633
Steglich C., Futschik M., Rector T., Steen R., Chisholm S. W. (2006). Genome-wide analysis of light sensing in Prochlorococcus. J. Bacteriol. 188, 7796–7806. doi: 10.1128/JB.01097-06
Sterner R. W., Elser J. J. (2003). Ecological stoichiometry (Princeton, NJ: Princeton University Press). doi: 10.1515/9781400885695
Su Z., Mao F., Dam P., Wu H., Olman V., Paulsen I. T., et al. (2006). Computational inference and experimental validation of the nitrogen assimilation regulatory network in cyanobacterium Synechococcus sp. WH 8102. Nucleic Acids Res. 34, 1050–1065. doi: 10.1093/nar/gkj496
Sullivan M. R., Primeau F. W., Hagstrom G. I., Wang W.-L., Martiny A. C. (2024). Integrating trait-based stoichiometry in a biogeochemical inverse model reveals links between phytoplankton physiology and global carbon export. Global Biogeochem. Cycles 38. doi: 10.1029/2023gb007986
Taucher J., Boxhammer T., Bach L. T., Paul A. J., Schartau M., Stange P., et al. (2021). Changing carbon-to-nitrogen ratios of organic-matter export under ocean acidification. Nat. Clim. Change 11, 52–57. doi: 10.1038/s41558-020-00915-5
Tempest D. W., Hunter J. R. (1965). The influence of temperature and pH value on the macro-molecular composition of magnesium-limited and glycerol-limited Aerobacter aerogenes growing in a chemostat. J. Gen. Microbiol. 41, 267–273. doi: 10.1099/00221287-41-2-267
Tetu S. G., Brahamsha B., Johnson D. A., Tai V., Phillippy K., Palenik B., et al. (2009). Microarray analysis of phosphate regulation in the marine cyanobacterium Synechococcus sp. WH8102. ISME J. 3, 835–849. doi: 10.1038/ismej.2009.31
Thiele I., Palsson B.Ø. (2010). A protocol for generating a high-quality genome-scale metabolic reconstruction. Nat. Protoc. 5, 93–121. doi: 10.1038/nprot.2009.203
Tolonen A. C., Aach J., Lindell D., Johnson Z. I., Rector T., Steen R., et al. (2006). Global gene expression of Prochlorococcus ecotypes in response to changes in nitrogen availability. Mol. Syst. Biol. 2, 53. doi: 10.1038/msb4100087
Torriani-Gorini A. (1987). “The birth and growth of the Pho regulon,” in Phosphate Metabolism and Cellular Regulation in Microorganisms, ed. Torriani-Gorini A., Rothman F. G., Silver S., Wright A., Yagil E (Washington, DC: ASM Press), 3–11.
Toseland A., Daines S. J., Clark J. R., Kirkham A., Strauss J., Uhlig C., et al. (2013). The impact of temperature on marine phytoplankton resource allocation and metabolism. Nat. Clim. Change 3, 979–984. doi: 10.1038/nclimate1989
Trautmann D., Voss B., Wilde A., Al-Babili S., Hess W. R. (2012). Microevolution in cyanobacteria: re-sequencing a motile substrain of Synechocystis sp. PCC 6803. DNA Res. 19, 435–448. doi: 10.1093/dnares/dss024
van Tol H. M., Armbrust E. V. (2021). Genome-scale metabolic model of the diatom Thalassiosira pseudonana highlights the importance of nitrogen and sulfur metabolism in redox balance. PloS One 16, e0241960. doi: 10.1371/journal.pone.0241960
Vaulot D., Marie D., Olson R. J., Chisholm S. W. (1995). Growth of prochlorococcus, a photosynthetic prokaryote, in the equatorial pacific ocean. Science 268, 1480–1482. doi: 10.1126/science.268.5216.1480
Velthuis M., Keuskamp J. A., Bakker E. S., Boersma M., Sommer U., Donk E., et al. (2022). Differential effects of elevated p CO 2 and warming on marine phytoplankton stoichiometry. Limnol. Oceanogr. 67, 598–607. doi: 10.1002/lno.12020
Verspagen J. M. H., Van de Waal D. B., Finke J. F., Visser P. M., Huisman J. (2014). Contrasting effects of rising CO2 on primary production and ecological stoichiometry at different nutrient levels. Ecol. Lett. 17, 951–960. doi: 10.1111/ele.2014.17.issue-8
Vikromvarasiri N., Noda S., Shirai T., Kondo A. (2023). Investigation of two metabolic engineering approaches for (R,R)-2,3-butanediol production from glycerol in Bacillus subtilis. J. Biol. Eng. 17, 3. doi: 10.1186/s13036-022-00320-w
Wagner N. D., Frost P. C. (2012). Responses of alkaline phosphatase activity in Daphnia to poor nutrition. Oecologia 170, 1–10. doi: 10.1007/s00442-012-2277-0
Wanner B. L. (1993). Gene regulation by phosphate in enteric bacteria. J. Cell. Biochem. 51, 47–54. doi: 10.1002/jcb.240510110
Weber T. S., Deutsch C. (2010). Ocean nutrient ratios governed by plankton biogeography. Nature 467, 550–554.
Yoshikawa K., Aikawa S., Kojima Y., Toya Y., Furusawa C., Kondo A., et al. (2015). Construction of a genome-scale metabolic model of Arthrospira platensis NIES-39 and metabolic design for cyanobacterial bioproduction. PloS One 10, e0144430. doi: 10.1371/journal.pone.0144430
Young J. N., Kranz S. A., Goldman J. A. L., Tortell P. D., Morel F. M. M. (2015). Antarctic phytoplankton down-regulate their carbon-concentrating mechanisms under high CO2 with no change in growth rates. Mar. Ecol. Prog. Ser. 532, 13–28. doi: 10.3354/meps11336
Yun H. S., Hong J., Lim H. C. (1996). Regulation of ribosome synthesis in Escherichia coli: effects of temperature and dilution rate changes. Biotechnol. Bioeng. 52, 615–624. doi: 10.1002/(SICI)1097-0290(19961205)52:5<615::AID-BIT9>3.0.CO;2-M
Yvon-Durocher G., Dossena M., Trimmer M., Woodward G., Allen A. P. (2015). Temperature and the biogeography of algal stoichiometry. Glob. Ecol. Biogeogr. 24, 562–570. doi: 10.1111/geb.2015.24.issue-5
Zhang H., Gu B., Zhou Y., Ma X., Liu T., Xu H., et al. (2022). Multi-omics profiling reveals resource allocation and acclimation strategies to temperature changes in a marine dinoflagellate. Appl. Environ. Microbiol. 88, e0121322. doi: 10.1128/aem.01213-22
Keywords: cyanobacteria, flux balance analysis, MAGMA, macromolecular model, macromolecules, microbes, pangenome, stoichiometry
Citation: Jones CLC, Camps-Castella J, Smykala M, Sobol MS and Inomura K (2024) Seeing through the gray box: an integrated approach to physiological modeling of phytoplankton stoichiometry. Front. Ecol. Evol. 12:1505025. doi: 10.3389/fevo.2024.1505025
Received: 01 October 2024; Accepted: 11 November 2024;
Published: 09 December 2024.
Edited by:
Giovanna Battipaglia, University of Campania Luigi Vanvitelli, ItalyReviewed by:
Timothy Ferdelman, Max Planck Society, GermanyCopyright © 2024 Jones, Camps-Castella, Smykala, Sobol and Inomura. This is an open-access article distributed under the terms of the Creative Commons Attribution License (CC BY). The use, distribution or reproduction in other forums is permitted, provided the original author(s) and the copyright owner(s) are credited and that the original publication in this journal is cited, in accordance with accepted academic practice. No use, distribution or reproduction is permitted which does not comply with these terms.
*Correspondence: Catriona L. C. Jones, am9uZTI0MjVAcHVyZHVlLmVkdQ==
†These authors have contributed equally to this work
‡ORCID: Catriona L. C. Jones, orcid.org/0000-0002-0225-6371
Camps-Castella J., orcid.org/0000-0001-7360-4102
Smykala M., orcid.org/0009-0004-7439-8514
Sobol M. S., orcid.org/0000-0002-9990-8507
Inomura K., orcid.org/0000-0001-9232-7032
Disclaimer: All claims expressed in this article are solely those of the authors and do not necessarily represent those of their affiliated organizations, or those of the publisher, the editors and the reviewers. Any product that may be evaluated in this article or claim that may be made by its manufacturer is not guaranteed or endorsed by the publisher.
Research integrity at Frontiers
Learn more about the work of our research integrity team to safeguard the quality of each article we publish.