- Hoja Nueva, Madre de Dios, Peru
This study provides novel insights into the ecology of the margay (Leopardus wiedii), focusing on its occupancy, density, and activity patterns in the Madre de Dios region, Peru, by utilizing both arboreal and terrestrial camera traps. Conducted across 10 km2, the research achieved 47 detections, utilizing semi-arboreal, lower canopy cameras for the first time to capture margay activities. Occupancy models revealed a mean occupancy probability of 53.82% and a detection probability of 6.57%. Among the environmental covariates, diameter at breast height (DBH) was identified as a significant predictor, negatively impacting occupancy, suggesting margays favor areas with smaller tree diameters. Contrary to expectations, the Normalized Difference Vegetation Index (NDVI) did not significantly influence occupancy. Tree density exhibited a positive, though non-significant, association with margay presence. Spatial capture-recapture (SECR) models estimated a margay density of 71.46 individuals per 100 km², with significant sex-based differences in spatial behavior. Males demonstrated larger home ranges (approximately 13.50 km²) compared to females (approximately 3.79 km²). Activity pattern analyses indicated primary nocturnal behavior with peaks at midnight to 3 am, 5 am, and 6 pm. Temporal overlap analysis revealed a low overlap coefficient with jaguarundis (Dhat1 = 0.21) and a higher overlap with ocelots (Dhat4 = 0.79), reflecting intricate interspecies dynamics. Our findings highlight important ecological aspects of margay behavior, including habitat preferences, nocturnal activity patterns, and interspecies interactions, which were effectively captured through the combined use of terrestrial and arboreal camera traps. The study emphasizes the importance of habitat preservation and the development of conservation strategies tailored to the ecological needs of margays, potentially influencing global practices for the management and protection of lesser-studied semi-arboreal wild cats.
Introduction
The margay (Leopardus wiedii, Schniz, 1821) stands out among Neotropical felids due to its semi-arboreal adaptations and nocturnal behavior, sharing morphological similarities with the more commonly recognized ocelot (Leopardus pardalis, Linnaeus, 1758) but exhibiting a more fragmented distribution (Sunquist and Sunquist, 2017). This felid occupies diverse habitats, from tropical lowlands to cloud forests, demonstrating a high degree of adaptability to both pristine and disturbed environments, often venturing close to human settlements (de Oliveira et al., 2014).
Margays excel in both arboreal and terrestrial locomotion, aided by unique physiological traits such as reversible ankles and long tails for balance. These adaptations highlight their ecological versatility but also expose them to significant risks such as deforestation, logging, and habitat fragmentation (Di Bitetti et al., 2010; de Oliveira et al., 2014). Despite their classification as “near threatened” by the IUCN Red List due to past fur exploitation and ongoing habitat loss, and a considerable density range of 9.6 ± 6.4 to 81 individuals per 100 km² noted across various studies (Pérez-Irineo and Santos-Moreno, 2016; Hernández-Sánchez et al., 2017; Horn et al., 2020), margays remain one of the least studied Neotropical felids, underscoring the urgency for focused research (MacDonald and Loveridge, 2010). Moreover, margays have been classified as “data deficient” on the Peruvian Red list (SERFOR, 2018) emphasizing the need for research efforts in the country.
Camera trapping has emerged as an invaluable, non-invasive tool for studying elusive species like the margay, capable of capturing detailed images that reveal unique spot patterns for individual identification. However, the effectiveness of this method is often compromised by the margay’s preference for dense, complex forests and forest canopies, challenging the collection of comprehensive data on their behavior and population dynamics (Vanderhoff et al., 2011; Bowler et al., 2017). To better capture the margay’s unique ecological behavior and habitat preferences, we placed camera traps at both arboreal and terrestrial heights, allowing for a more accurate assessment of margay occupancy, density, and activity patterns across different strata of the rainforest (Harmsen et al., 2021).
This research aims to provide new insights into margay ecology, focusing on their habitat use, spatial behavior, and interactions with other species in both arboreal and terrestrial environments. We hypothesize that incorporating semi-arboreal camera placements will offer a more comprehensive understanding of margay activity in the lower canopy and forest floor. We further hypothesize that specific arboreal structures, referred to as “margay trees,” act as critical access or choke points to the lower canopy, thereby increasing margay detections by facilitating their entry into this vertical stratum. By using Spatially Explicit Capture-Recapture (SECR) models, we estimate margay density and explore how environmental factors, such as the Normalized Difference Vegetation Index (NDVI) and prey availability, influence margay distribution and habitat use. Additionally, we will examine how habitat features such as NDVI, diameter at breast height (DBH), tree density, and the small prey index (SPI) influence margay occupancy patterns, with the hypothesis that higher NDVI and SPI values will positively correlate with higher occupancy rates. Furthermore, by analyzing activity patterns and exploring sex-based differences in spatial behavior, we seek to provide a detailed understanding of how margays navigate and utilize their environment, potentially avoiding competition with sympatric species like ocelots and jaguarundis (Herpailurus yagouaroundi). Through this multifaceted approach, this study aims to deepen our understanding of margay ecology and offer empirical data to inform and refine conservation strategies, thereby supporting the survival and conservation of this unique and understudied felid.
Methods
Study area
The study was conducted within a privately protected area in Las Piedras, Madre de Dios, Peru, centered around Hoja Nueva’s main research station (UTM 19L 442212E, 8667724N) (Figure 1). The study area of 10 km2 included both intact floodplain (seasonally flooded) and terra-firme (upland) forests, with a transitional zone in between. The land was primarily used for conservation and forest protection, with one incident of bushmeat hunting recorded during the study. Human activities were restricted to academic tourism, regular forest ranger patrols, and non-invasive wildlife research. The nearest mixed-use area with higher human impact, an agricultural association, was located more than 2 km south of the southernmost camera trap.
Camera trapping
Camera traps were deployed at 62 sites over 80 nights: 33 sites within grid A from July to September 2022, and 29 sites within grid B from September to November 2022. Grid placement ensured representation of both floodplain and terra firme forests, accessibility within hiking distance from Hoja Nueva’s centers, and geographic proximity to represent the same forest type. Surveys were conducted sequentially to allow temporal comparison, all within the dry-wet transition season. Camera station locations were randomly generated for each grid, with an inter-site distance of approximately 450 meters to promote recaptures of both male and female margays. To minimize bias, all camera stations were strategically located away from human trails. Camera traps were deployed at semi-arboreal (2-5 meters) and terrestrial (40 cm) heights to investigate margay activity and behavior across the forest understory and forest floor, providing a comprehensive view of the species’ habitat use and movement patterns.
Upon reaching the predetermined coordinates, suitable margay trees were identified based on criteria such as an angle of 20 - 60°, minimum trunk diameter of 20 centimeters, and canopy connectivity (Figure 2). Nearby locations for terrestrial cameras were then selected, ideally intersecting mammal trails where possible.
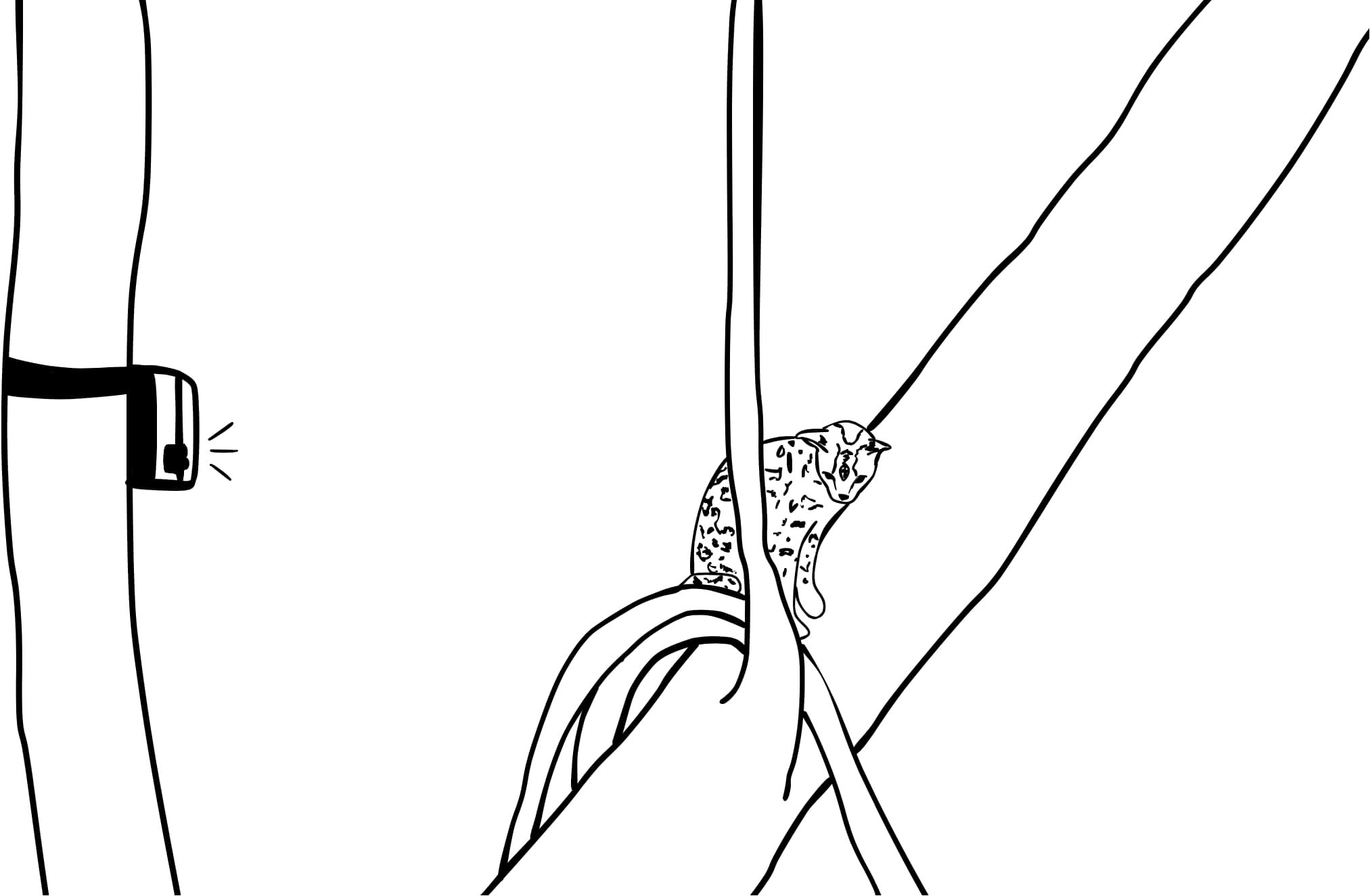
Figure 2. A drawing of a typical semi-arboreal camera placement, with the camera facing a margay tree within the understory layer.
Occupancy
To assess occupancy and detection probabilities of margays across various habitat characteristics, data from all 62 sites were pooled. Arboreal and terrestrial camera traps were treated as part of the same physical site due to their proximity, often within a few meters of each other, focusing either on the ground level (terrestrial) or the lower canopy (semi-arboreal). This approach prevented artificially inflating the perceived survey area and avoided spatial autocorrelation errors in occupancy modeling. Differentiation between semi-arboreal and terrestrial cameras was achieved through a categorical observation-level covariate. This allowed robust comparisons within the same spatial site but across different environmental viewpoints.
Occupancy models, fitted using this configuration, recorded the presence or absence of margays for each camera setup during observation periods. The analysis considered not only environmental and habitat variables but also the specific impact of camera placement on detection probability. NDVI data from a May 2022 Sentinel-22 raster obtained from NASA’s (2024) Earth Observing System Data and Information System using the Harmonized Landsat Sentinel-2 (HLS) S30 version 2.0 was used to estimate vegetation density at each site. We also incorporated finer scale habitat characteristics including the average DBH within ten meters of each site, the number of trees larger than 15cm DBH at each site (TREES), and the SPI, which were all standardized and analyzed to determine their influence on margay occupancy. The SPI was calculated as the number of captures of prey under 5 kilos divided by the total number of trap nights per site. Other covariates, such as proximity to rivers and elevation, did not significantly influence margay occupancy in a preliminary analysis (unpublished data) and were therefore excluded from the models. None of the continuous covariates showed high correlation, allowing for independent inclusion in occupancy analyses.
The analysis was conducted using the ‘unmarked’ package (Fiske and Chandler, 2011) in R (R Core Team, 2022). An ‘unmarkedFrame’ object, incorporating standardized site-specific ecological covariates (NDVI, DBH, TREES, SPI) and an observation-specific covariate for detection (camera placement), served as the input for fitting the occupancy models. Occupancy and detection models were fitted using the ‘occu’ function, with sub-models for detection influenced by camera placement, and occupancy influenced by environmental covariates. Model selection, based on ecological relevance and statistical parsimony, was optimized using the ‘dredge’ function from the ‘MuMIn’ package (Barton, 2009), exploring all plausible covariate combinations and ranking them by Akaike Information Criterion (AIC-Akaike, 1973).
Given the presence of multiple competitive models within two delta AIC units, we opted to employ model averaging to robustly estimate the effects of environmental covariates on margay occupancy. This approach allowed for the integration of uncertainty across a suite of plausible models, providing weighted average estimates of each parameter based on their relative support by the data. Specifically, we reported conditional averages, which incorporate only the models where the parameter appears, reflecting a more nuanced interpretation of influence when a parameter is not universally relevant across all considered models. This method ensures that our results maintain robustness against the risk of model selection bias, particularly important given the ecological complexity and potential collinearity among covariates in our study. The average probability of both detection and occupancy for margays were then recorded using the top model based on AIC. Lastly, as the home range diameter of margays exceeds the distance between our camera traps, and therefore violates the assumption of independence between sites, resulting parameters were interpreted as proportion of area used rather than proportion of area occupied (MacKenzie and Nichols, 2004).
Spatial capture-recapture
Margay photo captures were analyzed to identify individual animals based on unique coat patterns (Figure 3). Initial identification involved one reviewer examining images from each paired camera setup, focusing on distinctive markings on both sides of the animals. HotSpotter software (Crall et al., 2013) facilitated the selection of specific rectangular regions of interest (ROIs) on the images to match against a labeled database of all captured margays. Images lacking clear coat patterns were excluded to maintain identification accuracy. A second reviewer cross-verified each identification using the original images and HotSpotter results.
To estimate margay density and investigate potential sexual dimorphism in spatial ecology, SECR models were employed using the ‘secr’ package (Efford, 2023) in R. The analysis focused on a single grid (Grid A) with 33 sites, which gathered over 26 occasions spanning 78 trap nights. Data were imported and formatted for compatibility with the SECR modeling framework. Capture times were standardized to the local time zone (America/Lima), and sampling occasions were defined based on the study period from July to September 2022. Effective trap operation per occasion at each site was calculated and integrated into the capture histories. We assessed the appropriate buffer size and mask resolution for the SECR models by testing four different mask configurations with buffer sizes of 1000m and 2000m, and spacings of 200m, 250m, and 300m. The models were compared using AIC values, and the mask with a 2000m buffer and 200m spacing was selected due to its lowest AIC, indicating the best fit.
NDVI was incorporated into the selected mask by extracting NDVI values derived from a May 2022 Sentinel-22 raster layer. We used the extract function to obtain the mean NDVI values within a 150m buffer around each trap location, scaling these values to standardize them. These NDVI values were added as covariates to the mask, enabling us to test their effect on margay density. We fitted six different SECR models to test the effect of NDVI on density, as well as the effect of sex on lambda0 (encounter rate) and sigma (scale parameter), all using a hazard half-normal detection function with a Poisson observation model. Model comparisons were based on AICc values to select the best-fitting model based on small sample sizes.
Activity patterns
To investigate margay activity patterns and compare them with ocelots and jaguarundis, camera trap data from 64 distinct margay captures were analyzed. Activity patterns were visualized using ‘activityDensity’ and ‘activityRadial’ functions from the ‘camtrapR’ package (Niedballa et al., 2016), centered around the diurnal midpoint (12 noon). The overlap package generated kernel density estimates and calculated coefficients of overlap (Dhat statistics) between species. Radial plots visually represented activity throughout the day and overlap analyses with bootstrapped confidence intervals assessed the significance of observed overlaps between margay, ocelot, and jaguarundi activity patterns.
An extensive dataset from eight grids encompassing 293 camera sites, collected over seven years (2015-2022), was then integrated to enhance robustness. This dataset included 147 margay captures, 1,249 ocelot captures, and 40 jaguarundi captures, enabling a more comprehensive activity analysis.
Results
Occupancy
The conditional model averaging approach provided a nuanced understanding of the influences on margay occupancy across our study area. Table 1 presents the conditional averages for key parameters, reflecting the combined influence of the top models. From the suite of environmental covariates considered, DBH emerged as a significant predictor, with a negative effect on occupancy probabilities (Estimate = -1.289, p = 0.0489).
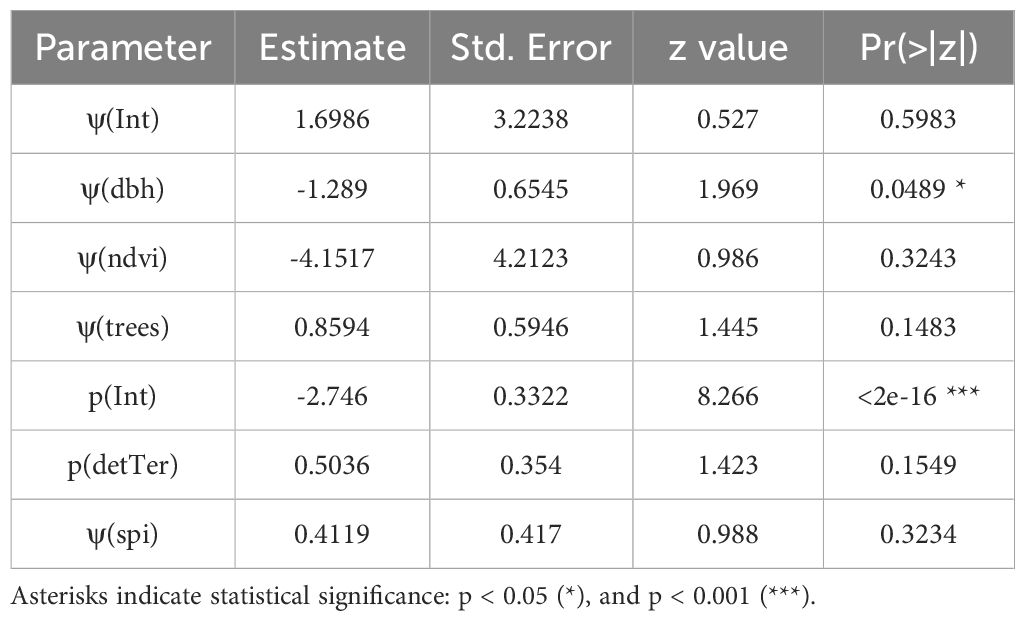
Table 1. Conditional average estimates from occupancy models showing the effects of environmental covariates on margay occupancy (ψ) and detection probability (p).
Contrarily, NDVI, a proxy for vegetation health and density, did not significantly influence margay occupancy (Estimate = -4.1517, p = 0.3243). Tree density, measured by the number of trees, showed a positive but non-significant association with margay occupancy (Estimate = 0.8594, p = 0.1483). SPI, while not included in the top model, appeared in several models close to the top model (Estimate = 0.4119, p = 0.3234).
Detection probability was significantly influenced by the intercept (Estimate = -2.7460, p < 2e-16), indicating a generally low detection probability across sites, which is typical for cryptic species like the margay. The effect of the detection covariate (detTer), representing terrestrial versus semi-arboreal camera trap placements, was positive but not significant (Estimate = 0.5036, p = 0.1549). 23 out of the total 47 detections were semi-arboreal, suggesting no substantial difference in detection probability between semi-arboreal and terrestrial camera setups (Figure 4).
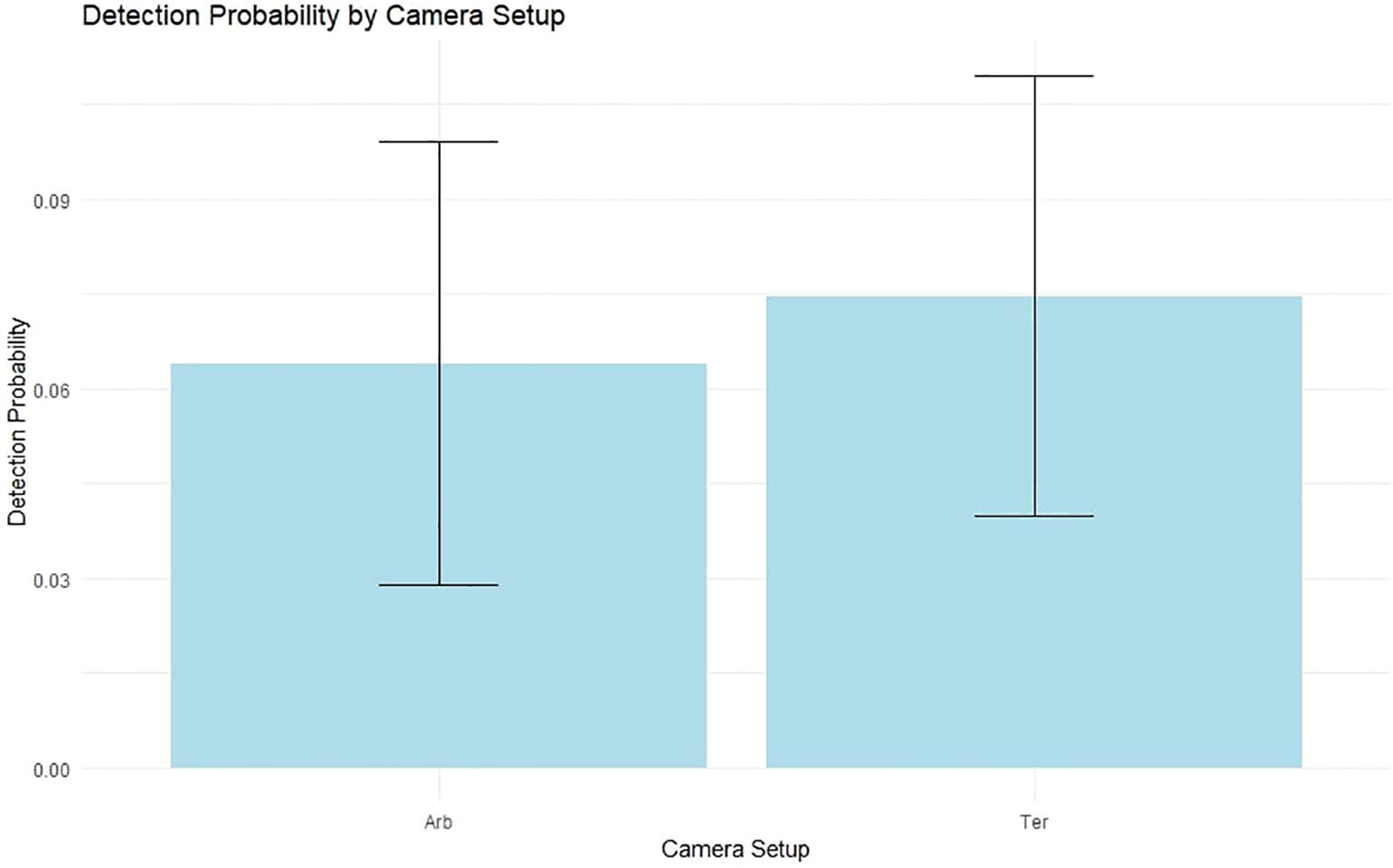
Figure 4. Detection probability by camera placement for margays. This boxplot shows the distribution of detection probabilities for margays using semi-arboreal and terrestrial camera setups. The probabilities on the y-axis reflect the likelihood of detecting margays with cameras placed in trees (Arb) and on the ground (Ter).
Predictions from the top-ranked model estimated the average occupancy probability across the study sites at approximately 53.82%, suggesting that nearly half of the sites likely support margay presence. However, the detection probability under this model remained low at about 6.57%, underscoring the inherent challenges in detecting these cryptic animals, even under presumably favorable conditions.
Spatial capture-recapture
The SECR analysis provided comprehensive insights into the population dynamics and spatial behavior of margays within the study area. Over the 78-day study period, a total of 34 capture events were recorded, involving 11 unique individuals. The selected model, based on AICc, incorporated sex as a covariate for sigma but not for detection probability (lambda0). Model results comparing AICc values can be found in Table 2.
Our results indicated that NDVI did not significantly affect margay density within the study area. The best-fitting model estimated a margay density of 71.46 individuals per 100 km2, with a standard error (SE) of 26.85. Details for the best-fitting model can be found in Table 3. The encounter rate (lambda0) was determined to be 0.025 (SE = 0.00748), indicating a low yet consistent detection probability across both sexes. Analysis showed that detection probability did not differ significantly between males and females, suggesting equal likelihood of capture when margays were present within the detection range of the traps.
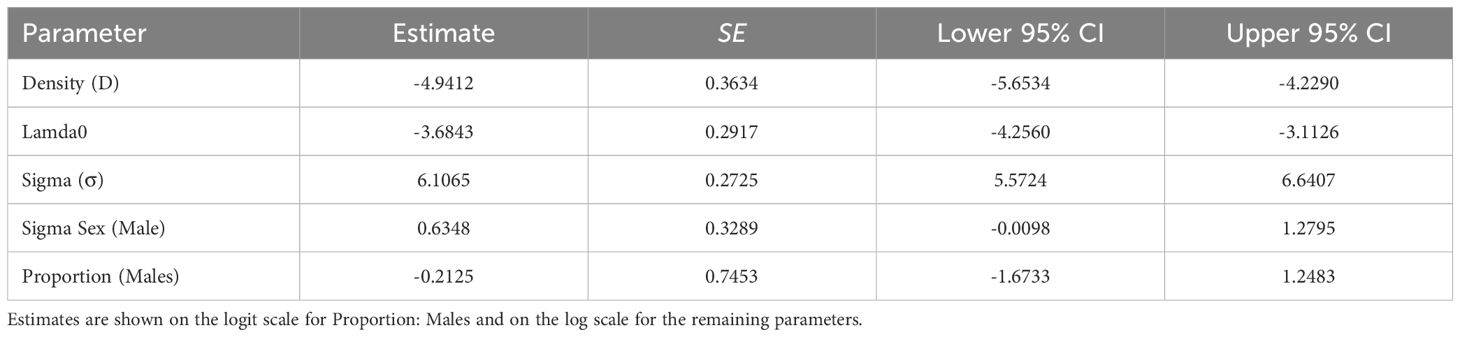
Table 3. Mean parameter estimates, standard errors (SE), and 95% confidence intervals (CI) from the resulting top model that included sex as a covariate for σ.
Sigma, the parameter representing the spatial scale of animal movements, demonstrated notable sex-specific differences. For female margays, sigma was estimated at 448.78 meters (SE = 124.61). In contrast, male margays exhibited a significantly larger sigma of 846.72 meters (SE = 196.36), indicating a broader spatial range for males. To elucidate home range sizes, 95% home range areas were calculated based on sigma values. The estimated home range for females was 3.79 km², whereas for males, the home range was substantially larger at 13.50 km². These values highlight the larger movement patterns and potentially greater territorial needs of male margays compared to females. The resulting sex ratio was 0.45 males to 0.55 females, indicating a slight female bias in the sampled population. This sex ratio, combined with the observed differences in spatial behavior, provides important context for understanding margay population dynamics in the study area.
Activity patterns
The activity analysis of margays based on 64 records indicated distinct nocturnal peaks at 12 am to 3 am, 5 am, and notably at 6 pm, as shown in the radial plot (Figure 5). This pattern establishes the margay as primarily nocturnal, contrasting sharply with the diurnal activity pattern of jaguarundis, which was predominantly between 7 am and 1 pm. The overlap coefficient (Dhat1) between margays and jaguarundis was notably low at 0.21, suggesting minimal temporal overlap.
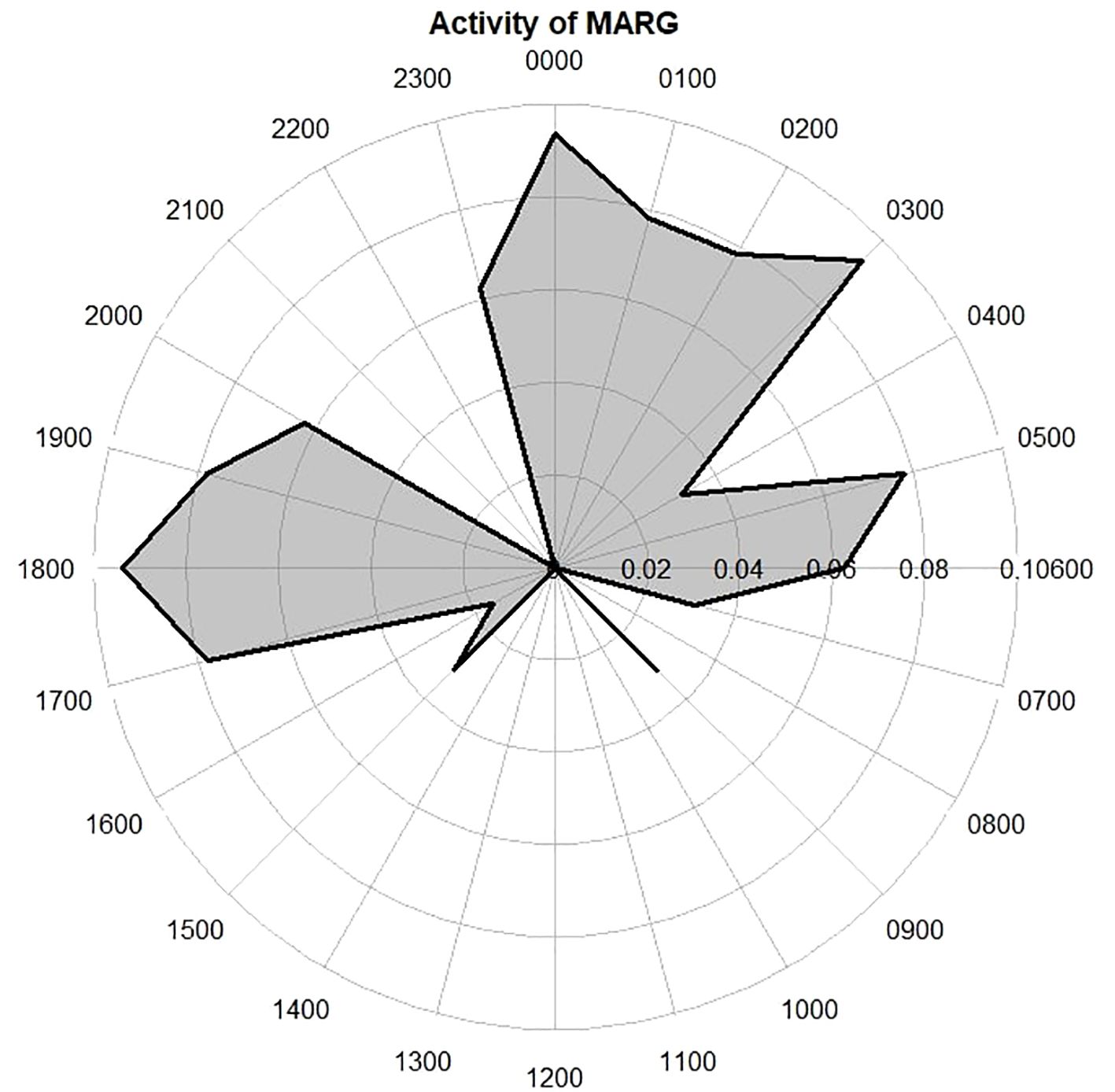
Figure 5. A radial plot of margay activity detailing primarily nocturnal activity with peaks at 5am, 6pm and from 12am-3am.
Conversely, the comparison between margays and ocelots revealed a higher degree of temporal overlap (Dhat4 = 0.79), with significant activity overlap during the early evening around 6 pm. Despite this, margays tended to avoid times when ocelots were most active, particularly during early morning (4 am-5 am) and late evening (11 pm-12 am) peaks. This pattern is visually represented in Figure 6, which also shows a moderate midday activity overlap between margays and ocelots, further contributing to the higher overall overlap coefficient.
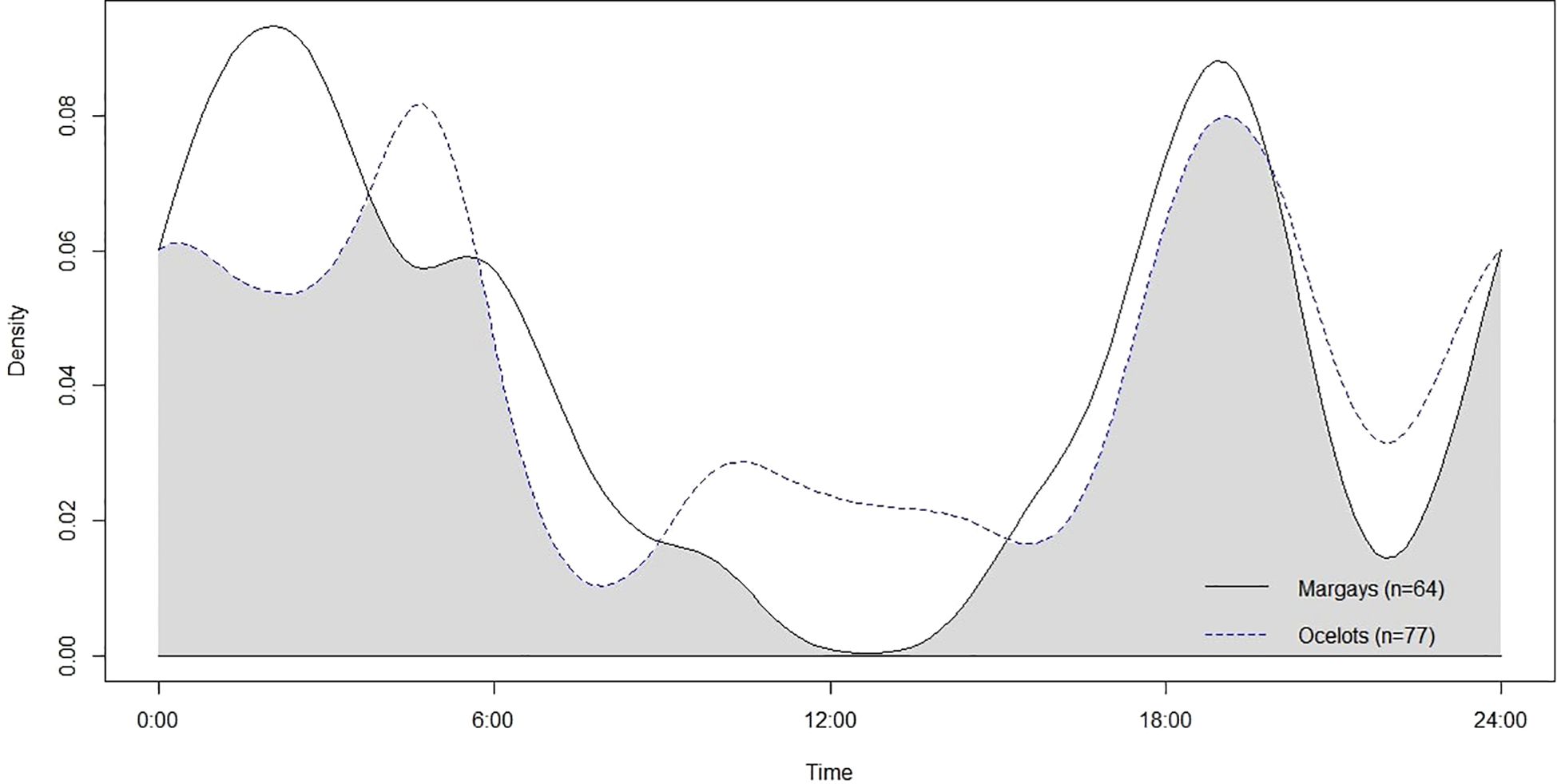
Figure 6. The temporal overlap of margays (n = 64) and ocelots (n = 77) based on kernel density functions (y axis) across a 24hr scale (x axis) with a center = noon. The shaded area represents the calculated coefficient of overlap (Dhat4) of 0.79.
The bootstrap analysis supported these findings, providing a confidence interval for the overlap coefficient between margays and ocelots (0.706 to 0.909), indicating a statistically significant overlap despite their distinct peak activity times. In contrast, the bootstrap results for the overlap with jaguarundis were inconclusive due to the small sample size (n=3 for jaguarundis), resulting in a wide confidence interval (0.013 to 0.413) and highlighting the need for more data for robust comparative analysis.
The analysis of the expanded dataset provided new insights and likely more accurate overlap estimates based on the higher number of captures per species. Based on mean bootstrap estimates, the overlap between ocelots and margays increased from 0.79 to 0.88 (CI 0.87-0.96), and the overlap between jaguarundis and margays increased from 0.21 to 0.35 (0.20-0.39). This broader dataset not only strengthens our current findings but also provides a more comprehensive view of interspecies interactions and activity patterns within this vital ecological region.
Discussion
The current study advances our ecological understanding of the margay, a lesser-studied Neotropical felid, by employing innovative camera trapping techniques alongside traditional methods. Our research highlights the value of capturing margay activity across both terrestrial and lower canopy environments, revealing previously under documented aspects of their habitat use and spatial behavior. This methodological innovation is crucial, given the species’ known tree-climbing capabilities and the challenges associated with documenting its arboreal behavior, which has been difficult to describe in previous studies (Vanderhoff et al., 2011; Bowler et al., 2017).
Our occupancy models emphasize the complex relationships between margay presence and environmental covariates. Contrary to previous studies that found NDVI to be a significant predictor of margay occupancy (Contreras-Díaz et al., 2022) and density (Horn et al., 2020), our results did not demonstrate a significant relationship between NDVI and margay presence. This discrepancy may suggest regional variations in habitat preferences or the species’ adaptability to different forest densities. However, while our study focused solely on a forest environment, the previously mentioned studies (Horn et al., 2020; Contreras-Díaz et al., 2022) incorporated data from a mosaic of habitats, including forests, wetlands, grasslands, and human-modified patches. The variability of habitats is likely influential, as NDVI values are expected to vary considerably more in a diverse landscape. This suggests that the strength of NDVI as a predictor for margay presence may be context-dependent, and likely exerts stronger effects at the landscape-level. In a further analysis (unpublished data), other factors like proximity to rivers and elevation, did not significantly influence margay occupancy in our study, indicating a possible unique ecological niche occupied by margays in our study area or the overriding importance of other unmeasured environmental or biotic factors.
The negative correlation with DBH indicates that margays prefer forests with smaller trees, likely due to their arboreal nature. Habitat preference has previously been described to be influenced by a species’ locomotion (Panciroli et al., 2017). Their unique climbing ability, which allows them to descend head-first by gripping tree trunks (Morales et al., 2018), may be better suited to smaller-diameter trees. This adaptation helps them to avoid competition with other felids like ocelots by using higher vertical strata (Di Bitetti et al., 2010). Consequently, forests with smaller trees might offer more escape routes, serving as “safe zones” for margays, especially in areas with a high ocelot presence, like in ours.
Further, while prey availability has shown to be a typical predictor for carnivores’ spatial ecology (Carbone et al., 2010; Santos et al., 2019), the SPI in our analysis was no important predictor for margay occupancy. However, previous authors have pointed out that using camera traps to quantify small mammals may under-represent their abundances (Santos et al., 2019). Thus, our results may reflect the limitations of currently available methodologies, rather than the spatial pattern of margays itself.
Our results reveal key insights into margay behavior, showing that both semi-arboreal and terrestrial cameras are essential for capturing the full range of their activities across different forest layers. Margays were frequently detected at both semi-arboreal and terrestrial levels, underscoring the species’ dynamic use of different vertical strata in the forest and highlighting the importance of multi-layered monitoring for understanding their ecological behavior. This finding supports the notion that margays, despite their arboreal tendencies, are frequently detected at both levels, which is essential for accurately assessing their population dynamics and behavioral ecology (de Oliveira et al., 2014). These findings suggest that margays utilize both terrestrial and arboreal environments, and future studies should continue to monitor multiple forest layers to gain a complete understanding of their behavior, habitat preferences, and interactions with sympatric species (O'Connell et al., 2011).
Furthermore, the “ocelot effect” suggests that in areas with high ocelot presence, densities of margays and other small cats are kept to a minimum, and can only reach high numbers when ocelots are absent or rare (de Oliveira et al., 2014). This general pattern has since been reported across different study areas (Kasper et al., 2016; Horn et al., 2020). However, our estimates substantially contrast this trend as our estimated margay density is 71.46 individuals per 100 km2, while the local ocelot population has been estimated to be 31.46 individuals per 100 km2 (Zwicker and Gardner, 2024). Coexistence between competitors can be maintained by partitioning through space, time and resources (Rodriguez Curras et al., 2022), indicating that one of these factors contrasts considerably between ours and other study areas. However, to be achieve robust explanations for this unique pattern of co-occurrence, more research incorporating fine-scale data on shared resources and habitat use (horizontal and vertical) is required.
Sexual dimorphism in spatial behavior was evident, with male margays exhibiting larger home ranges than females. This finding aligns with the known behaviors of other felids, where males typically require larger territories to maximize their reproductive success (Carvajal-Villarreal et al., 2012; Horn et al., 2020). Such sex-based differences in spatial behavior emphasize the need for conservation strategies to consider the distinct habitat requirements and pressures faced by each sex.
Our analysis delineated margay activity patterns and their temporal overlap with sympatric species such as ocelots and jaguarundis. The predominantly nocturnal activity of margays, with significant overlaps during specific periods, suggests intricate interspecies dynamics that may involve both competition and opportunistic coexistence (Kiltie, 1984). This observed temporal partitioning likely plays a crucial role in mitigating direct competition for resources, a strategy that has been observed in various sympatric species across ecosystems (Monterroso et al., 2013).
Our findings highlight the critical importance of preserving intact habitats and maintaining ecological corridors to ensure the survival of margay populations. By providing detailed insights into their spatial behavior, habitat preferences, and interactions with sympatric species, this study contributes valuable knowledge for developing conservation strategies tailored to the ecological needs of this elusive felid. Future research should include longitudinal studies across multiple regions to track changes in margay populations and refine our understanding of their ecological requirements. The use of semi-arboreal and terrestrial camera traps offers a more comprehensive view of their habitat use, aiding in the creation of targeted conservation efforts that address both arboreal and terrestrial habitats. Future research could also consider two-species occupancy models to explore potential spatial interactions between margays and sympatric species like ocelots and jaguarundis. This could complement the temporal overlap analysis by providing further insight into how these species partition space. Such analyses might enhance our understanding of competition or coexistence in shared habitats, though this remains a secondary consideration compared to the primary focus on margay ecology. In summary, this study makes a significant contribution to margay ecology, providing essential data on their spatial and activity patterns, which will help guide conservation actions and ensure the protection of critical habitats for this species.
Data availability statement
The raw data supporting the conclusions of this article will be made available by the authors, without undue reservation.
Ethics statement
The animal study was approved by Servicio Nacional Forestal - SERFOR. The study was conducted in accordance with the local legislation and institutional requirements.
Author contributions
SZ: Conceptualization, Data curation, Formal Analysis, Funding acquisition, Investigation, Methodology, Project administration, Resources, Software, Supervision, Validation, Visualization, Writing – original draft, Writing – review & editing. CS-L: Data curation, Formal Analysis, Investigation, Writing – original draft, Writing – review & editing, Methodology. CL: Conceptualization, Data curation, Investigation, Methodology, Project administration, Validation, Writing – original draft, Writing – review & editing.
Funding
The author(s) declare that financial support was received for the research, authorship, and/or publication of this article. Funding and resources were provided by Friends of Hoja Nueva.
Acknowledgments
We thank Dr. Beth Gardner and members of the University of Washington’s Quantitative Ecology Lab for their comments on earlier drafts of this manuscript. We also thank the members of nonprofit Hoja Nueva and the community Puerto Lucerna for their local support, resources, and collaboration. Finally, we are grateful for the insights gained from two of our rewilded margays, Loki and Guapuru, whose journeys have deepened our understanding of this remarkable species.
Conflict of interest
The authors declare that the research was conducted in the absence of any commercial or financial relationships that could be construed as a potential conflict of interest.
Publisher’s note
All claims expressed in this article are solely those of the authors and do not necessarily represent those of their affiliated organizations, or those of the publisher, the editors and the reviewers. Any product that may be evaluated in this article, or claim that may be made by its manufacturer, is not guaranteed or endorsed by the publisher.
References
Akaike H. (1973). Maximum likelihood identification of Gaussian autoregressive moving average models. Biometrika 60, 255–265. doi: 10.1093/biomet/60.2.255
Barton K. (2009). MuMIn: multi-model inference. R package version 1. 0. 0. Available online at: http://r-forge.r-project.org/projects/mumin/. (accessed November 21, 2023)
Bowler M. T., Tobler M. W., Endress B. A., Gilmore M. P., Anderson M. J. (2017). Estimating mammalian species richness and occupancy in tropical forest canopies with arboreal camera traps. Remote Sens. Ecol. Conserv. 3, 146–157. doi: 10.1002/rse2.35
Carbone C., Pettorelli N., Stephens P. A. (2010). The bigger they come, the harder they fall: body size and prey abundance influence predator–prey ratios. Biol. Lett. 7, 312–315. doi: 10.1098/rsbl.2010.0996
Carvajal-Villarreal S., Caso A., Downey P., Moreno A., Tewes M. E., Grassman L. I. (2012). Spatial patterns of the margay (Leopardus wiedii; Felidae, Carnivora) at “El Cielo” Biosphere Reserve, Tamaulipas, Mexico. Mammalia 76, 237–244. doi: 10.1515/mammalia-2011-0100
Contreras-Díaz R. G., Falconi M., Osorio-Olvera L., Cobos M. E., Soberón J., Townsend Peterson A., et al. (2022). On the relationship between environmental suitability and habitat use for three neotropical mammals. J. Mammalogy 103, 425–439. doi: 10.1093/jmammal/gyab152
Crall J. P., Stewart C. V., Berger-Wolf T. Y., Rubenstein D. I., Sundaresan S. R. (2013). “HotSpotter — Patterned species instance recognition," 2013 IEEE Workshop on Applications of Computer Vision (WACV), Clearwater Beach, FL, USA, 2013, pp. 230–237. doi: 10.1109/WACV.2013.6475023
de Oliveira T., Paviolo A., Schipper J., Bianchi R., Payan E., Carvajal S. V. (2014). Leopardus wiedii: the IUCN Red List of Threatened Species 2015. Gland, Switzerland: International Union for Conservation of Nature and Natural Resources. doi: 10.2305/IUCN.UK.2015-4.RLTS.T11511A50654216.en
Di Bitetti M. S., De Angelo C. D., Di Blanco Y. E., Paviolo A. (2010). Niche partitioning and species coexistence in a Neotropical felid assemblage. Acta Oecologica 36, 403–412. doi: 10.1016/j.actao.2010.04.001
Efford M. G. (2023). ipsecr: An R package for awkward spatial capture–recapture data. Methods Ecol. Evol. 14, 1182–1189. doi: 10.1111/2041-210X.14088
Fiske I., Chandler R. (2011). unmarked: an R package for fitting hierarchical models of wildlife occurrence and abundance. J. Stat. Software 43, 1–23. doi: 10.18637/jss.v043.i10
Harmsen B. J., Saville N., Foster R. J. (2021). Long-term monitoring of margays (Leopardus wiedii): Implications for understanding low detection rates. PLoS One 16, e0247536. doi: 10.1371/journal.pone.0247536
Hernández-Sánchez A., Santos-Moreno A., Pérez-Irineo G. (2017). Abundance of mesocarnivores in two vegetation types in the southeastern region of Mexico. Southwestern Nat. 62, 101–108. doi: 10.1894/0038-4909-62.2.101
Horn P. E., Pereira M. J. R., Trigo T. C., Eizirik E., Tirelli F. P. (2020). Margay (Leopardus wiedii) in the southernmost Atlantic Forest: Density and activity patterns under different levels of anthropogenic disturbance. PLoS One 15, e0232013. doi: 10.1371/journal.pone.0232013
Kasper C. B., Schneider A., Oliveira T. G. (2016). Home range and density of three sympatric felids in the Southern Atlantic Forest, Brazil. Braz. J. Biol. 76, 228–232. doi: 10.1590/1519-6984.19414
Kiltie R. A. (1984). Size ratios among sympatric neotropical cats. Oecologia 61, 411–416. doi: 10.1007/BF00379644
MacDonald D. W., Loveridge A. J. (2010). The Biology and Conservation of Wild Felids (Oxford: Oxford University Press).
MacKenzie D. I., Nichols J. D. (2004). Occupancy as a surrogate for abundance estimation. Anim. biodiversity Conserv. 27, 461–467. doi: 10.32800/abc.2004.27.0461
Monterroso P., Alves P. C., Ferreras P. (2013). Catch me if you can: diel activity patterns of mammalian prey and predators. Ethology 119, 1044–1056. doi: 10.1111/eth.12156
Morales M. M., Moyano S. R., Ortiz A. M., Ercoli M. D., Aguado L. I., Cardozo S. A., et al. (2018). Comparative myology of the ankle of Leopardus wiedii and L. geoffroyi (Carnivora: Felidae): functional consistency with osteology, locomotor habits and hunting in captivity. Zoology 126, 46–57. doi: 10.1016/j.zool.2017.12.004
NASA (2024). Earth Observing System Data and Information System (EOSDIS). Available online at: https://www.earthdata.nasa.gov (Accessed March 21, 2024).
Niedballa J., Sollmann R., Courtiol A., Wilting A. (2016). camtrapR: An R package for efficient camera trap data management. Methods Ecol. Evol. 7, 1457–1462. doi: 10.1111/2041-210X.12600
O'Connell A. F., Nichols J. D., Karanth K. U. (2011). Camera traps in animal ecology: methods and analyses Vol. 271 (New York: Springer).
Panciroli E., Janis C., Stockdale M., Martín-Serra A. (2017). Correlates between calcaneal morphology and locomotion in extant and extinct carnivorous mammals. J. Morphology 278, 1333–1353. doi: 10.1002/jmor.20716
Pérez-Irineo G., Santos-Moreno A. (2016). Abundance and activity patterns of medium-sized felids (Felidae, Carnivora) In Southeastern Mexico. Southwestern Nat. 61, 33–39. doi: 10.1894/0038-4909-61.1.33
R Core Team (2022). R: A language and environment for statistical computing (Vienna, Austria:R Foundation for Statistical Computing). Available at: https://www.R-project.org/.
Rodriguez Curras M., Donadio E., Middleton A. D., Pauli J. N. (2022). Carnivore niche partitioning in a human landscape. Am. Nat. 199, 496–509. doi: 10.5061/dryad.00000004p
Santos F., Carbone C., Wearn O. R., Rowcliffe J. M., Espinosa S., Lima M. G. M., et al. (2019). Prey availability and temporal partitioning modulate felid coexistence in Neotropical forests. PloS One 14, e0213671. doi: 10.1371/journal.pone.0213671
SERFOR (2018). Libro Rojo de la Fauna Silvestre Amenazada del Perú, 1st edn SERFOR. Lima, Peru: Servicio Nacional Forestal y de Fauna Silvestre.
Vanderhoff E., Hodge A.-M., Arbogast B., Nilsson J., Knowles T. (2011). Abundance and activity patterns of the margay (Leopardus wiedii) at a mid-elevation site in the eastern Andes of Ecuador. Mastozoologia Neotropical 18, 271–279.
Keywords: behavioral ecology, home ranges, Leopardus wiedii, occupancy modeling, semiarboreal camera, spatial capture-recapture
Citation: Zwicker S, Sánchez-Latorre C and Lukasser C (2024) Increasing detections of the margay: occupancy, density, and activity patterns in Madre de Dios, Peru. Front. Ecol. Evol. 12:1500202. doi: 10.3389/fevo.2024.1500202
Received: 22 September 2024; Accepted: 17 October 2024;
Published: 12 November 2024.
Edited by:
Mathew Samuel Crowther, The University of Sydney, AustraliaReviewed by:
J. Weldon McNutt, Botswana Predator Conservation Trust, BotswanaJames Hines, USGS, United States
Copyright © 2024 Zwicker, Sánchez-Latorre and Lukasser. This is an open-access article distributed under the terms of the Creative Commons Attribution License (CC BY). The use, distribution or reproduction in other forums is permitted, provided the original author(s) and the copyright owner(s) are credited and that the original publication in this journal is cited, in accordance with accepted academic practice. No use, distribution or reproduction is permitted which does not comply with these terms.
*Correspondence: Samantha Zwicker, c2p6d2lja2VyQGhvamFudWV2YS5vcmc=