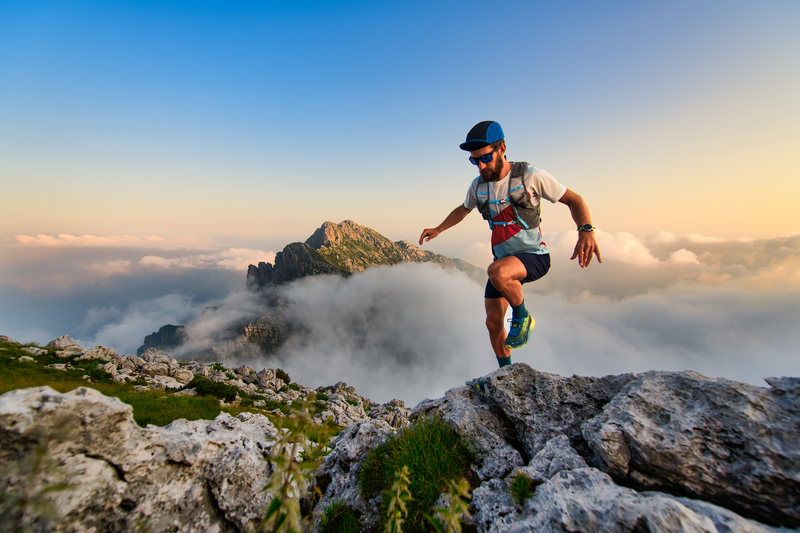
94% of researchers rate our articles as excellent or good
Learn more about the work of our research integrity team to safeguard the quality of each article we publish.
Find out more
METHODS article
Front. Ecol. Evol. , 09 December 2024
Sec. Behavioral and Evolutionary Ecology
Volume 12 - 2024 | https://doi.org/10.3389/fevo.2024.1465094
Most large carnivores feed on prey infrequently and may expend large amounts of energy to locate, capture and kill their prey. This makes them probabilistically vulnerable to fluctuating rates of energy acquisition over time, especially within the increasingly human-altered landscapes that dominate their remaining range. Consequently, quantifying their hunting behaviors and success rates is critical, yet direct observation of these events is rarely feasible. We theorized that we could determine prey pursuit and capture in African wild dogs (Lycaon pictus) using a mechanistic approach by constructing Boolean algorithms applied to accelerometer data derived from collar-mounted tags. Here, we used this method and then iteratively improved algorithms by testing them on observed hunts and kills of collared packs. Using this approach on 47 days of acceleration from three wild dogs in three packs, we identified 29 hunts with 10 kills, all of which were confirmed by direct observation except for a single kill. Our results demonstrate that hunting effort and success can largely be determined from acceleration data using a mechanistic approach. This is particularly valuable when such behaviors are rarely quantified and offers a template for research on foraging in canid species, while also contributing to the expanding body of literature that employs similar methods to quantify hunting in large carnivores.
The acquisition of energy from food is fundamental for animals, serving as the cornerstone of their survival and reproductive success (Perrin and Sibly, 1993). Within terrestrial endotherms, however, the details of food acquisition show extreme variability between taxa. For example, herbivores spend large portions of their day feeding on abundant, but energetically poor foodstuffs, while top predators feed relatively infrequently on high quality prey (Meunier et al., 2023) which requires considerable effort to obtain. Infrequent encounters with food, which can be couched as mathematically improbable (Wilson et al., 2018b), makes carnivores, especially top predators, susceptible to increased variability in the rate of energy intake over time compared to herbivores (Wilson et al., 2018b). Consequently, when prey become scarce, it can result in adults being unable to acquire enough energy to survive and meet reproductive requirements reliably (Scantlebury et al., 2014). African wild dogs (Lycaon pictus) have exceptionally high energetic costs of reproduction (Creel and Creel, 1991) and unusually high energy expenditure due to movement and hunting (Creel and Creel, 1995; Gorman et al., 1998), and recent declines in prey abundance are correlated with reduced survival and reproduction of African wild dogs (Creel et al., 2024; Reyes de Merkle et al., 2024). Predicting the energetic costs of hunting across different species, groups, or individuals presents inherent limitations due to variations in biotic and abiotic factors, such as habitat differences, prey availability, hunting strategies, and species-specific physiological adaptations. These factors can significantly affect both energy expenditure and hunting success, often in species- and environment-specific ways. However, a mechanistic approach provides a robust foundational framework that can be adapted in future studies to account for these variables, enhancing the accuracy and applicability of energetic predictions.
There are thus compelling reasons to examine the details of prey acquisition in wild dogs and other top predators. This is not trivial. Many species range widely, especially during hunts, and may operate at night in dense and topographic environments where observations are difficult (Hunter, 2018; Loarie et al., 2013). Animal-attached tags using videos and measuring acceleration have been used with some success to document feeding in animals as diverse as Caribou Rangifer tarandus (McNeill et al., 2020) ranging to more cryptic marine species including seals (Parrish et al., 2000) and penguins. However, videos can typically only record data for a few hours or days, which makes them unsuitable for animals that feed relatively rarely and sporadically (but see Yoshino et al., 2020). Additionally, mounting a video recorder on a carnivore poses challenges due to the species’ lifestyle and movement patterns, which expose the equipment to high impact forces and dirt accumulation, often obstructing the camera lens. In contrast, accelerometers on collars can operate for weeks or months (Fehlmann et al., 2017, Mortlock et al., 2024), although if the movement of the tag is uncoupled from that of the animal (Wilson et al., 2021), feeding can be problematic to determine.
This paper examines hunting in African wild dogs, an endangered species that is found at low densities under the best of conditions and is increasingly affected by anthropogenic prey depletion (Goodheart et al., 2021; Creel et al., 2023, 2024, Reyes de Merkle et al., 2024). We examined data from collar-mounted tri-axial accelerometers and, using first principles, derived rules for a Boolean-based algorithm (Wilson et al., 2018a) to blindly identify wild dog hunts and kills in long-term (>24 hours of data) acceleration data. We then used direct observation hunting data collected via intensive hunt follows of collared packs to compare to the predicted hunts that the algorithm generated from accelerometer data before iteratively changing the algorithm to improve its performance (Creel and Creel, 1995, 2002). The acceleration data features indicative of hunting are likely to be reflected in many large pursuit predators, depending on chase dynamics (e.g., African wild dogs dispersing while pursuing dik-diks, Antilope saltiana). Therefore, our general approach may hold broader applicability for studying other top predators.
Fieldwork was conducted in Greater Kafue, Luangwa Valley, and Greater Liuwa Ecosystems, Zambia during 2022 and 2023. Nineteen animals in 14 packs were equipped with Daily Diary tags (DDs) (Wilson et al., 2008), attached to Iridium-GPS radiocollars (TGW-4277-4, Telonics, Mesa, Arizona) to allow direct observation in continuous hunt follows (Creel and Creel, 1995). We radiocollared wild dogs after intramuscular injection of ~1.2 mg medetomidine and ~20 mg tiletamine–zolazepam, reversing the medetomidine by intramuscular injection of atipamezole after 45 min to 1 h. Anesthetics were injected by darting with an air-powered DanInject rifle, and all procedures were performed by an experienced and Zambian-registered veterinarian, in collaboration with the Zambia Department of National Parks and Wildlife (MSU IACUC 2020-123). We confirmed that radiocollaring did not detectably affect survival rates using a Bayesian Cormack–Jolly–Seber model that controlled for individual variation in the probability of detection (Goodheart et al., 2021; Reyes de Merkle et al., 2024). \ The DDs recorded orthogonal tri-axial acceleration (-16 – +16 g with 16 bit resolution) at 40 Hz, as well triaxial magnetic field intensity (7 Hz), temperature and pressure (both 1 Hz). The system used two 750 mAh batteries and data were written onto a micro-SD card. Collars were programmed to drop off (via remote release mechanisms on the collars) the animal after 31 days at which point the collar and tag was collected.
Parallel to the DD tag deployments, we observed three collared packs during hunting periods on 47 days by following in a vehicle and using a commercially available software CyberTracker (for details see https://cybertracker.org/) to record all observed hunts, kills and feeding periods. In the observational data, we defined hunts using the criteria of Creel and Creel (1995). When following hunts, we maintained a distance at which the wild dogs did not detectably respond to our presence. At 730 wild dog kills observed since 2013, we never observed the dogs to stop feeding or abandon a kill in response to our presence. We also used data from Kafue in 2022 to test whether our presence affected the wild dogs’ energy expenditure, and found that VeDBA did not differ on days that packs were followed and days that they were not followed (means = 0.1201 vs 0.1216 respectively, b = 0.0015438, se = 0.0015210, t = 1.015, P = 0.31).The observed hunt and/or kill data were not compared to our algorithm-defined hunts (see below) until the Boolean algorithm for identifying them had been constructed and run on all data. In other words, the conditions we used to define hunts and kills in the acceleration data were constructed blind. However, comparison of the algorithm-defined hunts and kills with known hunts and kills subsequently allowed us to refine the algorithm to improve its performance.
Initially, data were inspected for periods of high vectorial dynamic body acceleration (VeDBA) according to;
where A refers to the acceleration in the x, y or z orthogonal axes and the ‘r’ and ‘s’ subscripts refer to the raw or smoothed acceleration, respectively (Qasem et al., 2012). The ‘s’ acceleration values were means from a smoothing window over 2 seconds (Shepard et al., 2008). In addition, a running mean across 3.5 seconds was applied to the VeDBA values to reduce noise caused by the collar movement. This metric was labelled VeDBA smoothed.
Since high VeDBA values are indicative of substantial body dynamism (see Wilson et al., 2020), and given that wild dogs are cursorial predators with chases that average 570 meters (840 meters in successful chases) and can exceed 60 km/h (Fanshawe and Fitzgibbon, 1993; Creel and Creel, 2002), we reasoned that most hunts would be indicated by high VeDBA values. In tandem, we expected runs indicative of prey chases to last at least 30 seconds (Hubel et al., 2016) and to be characterized by clear waveforms in the acceleration x, y or z-axes corresponding to the bounding gait of the dogs (Hayati et al., 2019) although the amplitude of such waveforms was expected to vary with axis somewhat according to collar rotation (Wilson et al., 2021). Examination of the period and amplitude of the waveforms indicated whether the dogs were most likely to be walking, trotting, running or sprinting. These behaviors were defined by simple VeDBA smoothed (using a running mean across 3.5 seconds or 140 events) thresholds and limits: Resting was VeDBA smth < 0.05 g, walking was VeDBA smth > 0.18 g AND VeDBA smth < 0.4 g, trotting was VeDBA smth > 0.4 g AND VeDBA smth < 0.9 g, running was VeDBA smth > 0.9 g AND VeDBA smth < 1.4 g, sprinting was VeDBA smth > 1.4 g. All behaviors apart from resting were filtered by time where all marked events less than 2 seconds were deleted. All other behaviors were marked as ‘other’ (Figure 1).
Figure 1. (A) Changes in the tri-axial acceleration and smoothed VeDBA recorded by a collar-mounted tag on a Wild Dog during behaviour defined as resting, walking, trotting, running, sprinting and ‘other behaviour’. Note the waveform in the signals that corresponds to the bounding gait of the dog. Running and sprinting were deemed to be behaviors associated with a prey pursuit. (B) The frequency distribution of smoothed VeDBA values for the first 100,000 seconds of each individual dog tagged from various packs show clear bimodality between low level movement and high level movement. The data from all individuals are summed in the left figure, and a break-down for each individual dog is provided on the right.
However, it was not necessary to differentiate between all running gaits to define chases (see chase definition below). This is important because wild dogs hunt cooperatively but individuals alter their speed (or even stop to listen) when they lose sight of the prey and packmates (for example in long grass or chasing a separated herd).
Frequency distributions for VeDBA smoothed showed a marked bimodality with most of the higher mode attributable to forms of running (trotting, running and sprinting) (Figure 1B).
To describe the conditions of a chase, we generated the following Boolean algorithm;
IF VeDBA smoothed (rolling mean across 3.5 s) is greater than 0.9 g, then mark as an event. We selected 0.9 g because it consistently fell within the nadir between the two modes of the frequency distribution. These markers were merged if they occurred within 10 s of each other. Smoothed VeDBA values > 0.9 g occurring for >38 seconds were considered as hunts/chases.
Following identification of chases within the data, we further inspected the data for evidence of feeding which typically occurs within about 5 minutes of making a kill, and usually immediately (Creel and Creel, 2002). Specifically, once a chase was defined and terminated, we searched the data stream over a further 375 seconds to identify feeding behavior. Feeding typically occurs by the (standing) dogs reaching down to secure pieces of flesh, either eating directly or, more often, pulling back to tear off tissue before swallowing it and repeating the process. The act of lowering the head to feed alters the pitch of the collar, apparent in the surge acceleration (the collar pitch of a stationary animal is given by the asin of the surge acceleration). In addition, the act of tearing off flesh, which typically involves a sudden backward acceleration, was apparent in the surge acceleration as a peak contributing to an increase in VeDBA values. This was followed by brief pauses during which the animals chewed and/or swallowed food. Such acceleration signatures (Figure 2) were apparent in 10 cases following 29 identified chases.
Figure 2. Tri-axial acceleration signal from a collar-mounted tag on a Wild Dog during hunting and the subsequent period for; (A) an unsuccessful hunt and (B) a successful hunt, followed by feeding (verified by observation). Note that running or sprinting occurred in both examples but following a successful kill, the pitch angle decreased to indicate the dog lowering its head. This pitch-down behavior typically occurred numerous times and for brief periods (although >3 seconds). The figure illustrates threshold values (dashed line) and shaded grey regions to indicate periods when a valid pitch angle event was detected. To be classified as a feeding event, the head movement had to persist for a specified duration (see feeding algorithm). Failure to secure prey is marked by a break in activity as the animal slows down and rests to recover from the pursuit with no change in pitch angle.
We constructed the following algorithm to identify feeding following an identified chase.
1. IF the pitch angle smoothed (running mean across 5 s) < -25°
2. AND VeDBA smoothed (running mean across 3.5 s) >0.02 g
3. AND VeDBA smoothed (running mean across 3.5 s) <0.15 g
4. AND acceleration in the Y axis (heave) smoothed (running mean across 0.5 s) > 0.7 g, then mark events.
These marked events were merged if they were adjacent and occurred within 1 second of each other. Any continuously marked data less than 18 s were deleted.
If feeding behavior, as defined, occurred within the 375 s search window following a chase, the pursuit was deemed to be a success. If no feeding behavior was identified, the chase was classified as a failure.
Our algorithm correctly identified all 29 observed chases where field observations were used for validation. Ten of these (34%) were followed by acceleration signatures indicative of feeding. In all cases of a field-observed chase and/or chase and kill, the Boolean algorithm had identified it too, except for one kill where only a chase was successfully identified, with feeding behavior quantified 40 minutes after that (and was therefore not within the algorithm’s search window). This exception is not surprising because individual wild dogs sometimes do not begin feeding until higher-ranking pack mates or pups have finished.
We recognize that, although our algorithms identified all cases where chase and feeding were observed, we cannot definitively say the extent to which predicted but unobserved chases and/or feeding were correctly identified. During follows of hunting packs in Kafue, continuous observation was not possible, and many intervals were recorded as ‘lost dogs’ if a hunt or kill could have occurred without being detected (for example if we could not maintain contact after a stream crossing). In our full study, which involved 19 Wild dogs from 14 packs, we identified a total of 1428 chases [47 wild dog days] of which 423 (30%) showed subsequent feeding. However, the almost perfect matching of chases where they could be observed lends confidence in the approach. The number of chases per day exceeds that reported (0-16 chases/day, mean = 4.2) from direct observation of wild dogs in the Selous Game Reserve (Creel and Creel, 1995). Prey density in the Selous was high, which might allow fewer chases per day, but our results suggest that some chases go undetected by direct observation. The 30% success rate we determined is lower than the success rate determined from direct observation in Selous (44%), consistent with the larger number of chases we detected and suggesting that kills are usually detected by both methods.
Our findings demonstrate the extent to which at least some behaviors of wild animals can be derived by working from a basic understanding of acceleration data, even using collar-based data, without the need for e.g. machine learning approaches (e.g. Greener et al., 2022). This is particularly useful when behaviors are rare – or perhaps never observed, which would preclude any supervised machine learning approaches. In addition, our algorithms can run over appreciable lengths of time – e.g. first a period of high VeDBA lasting at least 30 s, followed by a pause of up to 375 s (or longer, though not examined here), after which feeding behavior may occur. Such serial, long periods are not always dealt with easily by machine learning systems such as simple regression models (Maulud and Abdulazeez, 2020) although both recurrent neural networks (RNNs) (Lipton et al., 2015) and Long Short-Term Memory (LSTM) networks (Lindemann et al., 2021) are specifically designed to handle sequential data.
The ability to predict animal activities that are energetically costly plays an important role in understanding the energetics of foraging efficiency [the energetic gain divided by the energy expended to get it (e.g. Nagy et al., 1984)]. This is particularly critical in top predators such as the larger felids and canids, which feed relatively infrequently on relatively scarce prey that are difficult to catch. The broad similarities between hunting techniques used by large carnivores suggests that the algorithms we used for wild dogs may be used for other top predators with minor modification, particularly for other coursing hunters. This approach could provide important information about the impact on prey populations of the study animal, and is likely to have implications for conservation efforts. Large herbivore populations are declining in many regions (Ripple et al., 2015), often leading to parallel decline of large carnivores (Estes et al., 2011).
Competitively subordinate species like African wild dogs were long thought to be buffered from the effects of prey depletion by the benefits of competitive release, but the density, survival and fecundity of wild dogs are all affected by current levels of anthropogenic prey depletion (Creel et al., 2024; Goodheart et al., 2021; Reyes de Merkle et al., 2024). With specific regard to wild dogs, combination of our Boolean approach with other technological advances, such as GPS-enabled dead-reckoning (Gunner et al., 2021) where the minutia of the movement paths can be resolved, could also provide important information on the pack dynamics of wild dogs which influences hunting success and plays a crucial role in feeding behavior, food distribution among pack members, and the social structure within the group (Creel and Creel, 2002).
Overall, our findings have broad and substantial implications for ecological and conservation research, offering a scalable and efficient tool to dissect the foraging behaviors of elusive or sparsely distributed top predators, circumventing the difficulties of more complex analytical techniques such as many forms of machine learning. The flexibility of our algorithm, with potential for adaptation across various taxa, paves the way for broader applications, enhancing our understanding of predator-prey interactions, and by extension, ecosystem dynamics.
The raw data supporting the conclusions of this article will be made available by the authors, without undue reservation.
The animal study was approved by Montana State University Institutional Animal Care and Use Committee. The study was conducted in accordance with the local legislation and institutional requirements.
JR: Visualization, Writing – original draft, Data curation, Formal analysis, Methodology. SC: Conceptualization, Data curation, Funding acquisition, Investigation, Project administration, Resources, Supervision, Writing – original draft, Writing – review & editing. BG: Data curation, Methodology, Writing – review & editing. JR: Data curation, Methodology, Writing – review & editing. SM: Data curation, Methodology, Writing – review & editing. MM: Data curation, Methodology, Writing – review & editing. RK: Data curation, Methodology, Writing – review & editing. EK: Data curation, Methodology, Writing – review & editing. WD: Writing – review & editing. AK: Writing – review & editing. CC: Writing – review & editing. MB: Data curation, Methodology, Writing – review & editing. RW: Conceptualization, Funding acquisition, Investigation, Supervision, Writing – original draft.
The author(s) declare financial support was received for the research, authorship, and/or publication of this article. The work was funded by NERC (NE/X015491/1) and by the National Science Foundation (IOS-1145749, DEB-2032131 and DEB-2221826), National Geographic Society Predator Research Grant, Dazzle Africa, World Wildlife Fund-Netherlands & Zambia, Tusk Trust, Painted Dog Conservation Inc., Gemfields Inc., Green Safaris.
We thank the Department of National Parks and Wildlife and the Zambia Department of Veterinary and Livestock Development for permission and collaboration with this research. This work was approved by Montana State University (IACUC approval number 2020-123).
The authors declare that this study received funding from y NERC (NE/X015491/1) and by the National Science Foundation (IOS-1145749, DEB-2032131 and DEB-2221826), National Geographic Society Predator Research Grant, Dazzle Africa, World Wildlife Fund-Netherlands & Zambia, Tusk Trust, Painted Dog Conservation Inc., Gemfields Inc., Green Safaris. The funders were not involved in the study design, collection, analysis, interpretation of data, the writing of this article or the decision to submit it for publication.
All claims expressed in this article are solely those of the authors and do not necessarily represent those of their affiliated organizations, or those of the publisher, the editors and the reviewers. Any product that may be evaluated in this article, or claim that may be made by its manufacturer, is not guaranteed or endorsed by the publisher.
The Supplementary Material for this article can be found online at: https://www.frontiersin.org/articles/10.3389/fevo.2024.1465094/full#supplementary-material
Creel S., Becker M. S., de Merkle J. R., Goodheart B. (2023). Hot or hungry? A tipping point in the effect of prey depletion on African wild dogs. Biol. Conserv. 282, 110043. doi: 10.1016/j.biocon.2023.110043
Creel S. R., Creel N. M. (1991). Energetics, reproductive suppression and obligate communal breeding in carnivores. Behav. Ecol. Sociobiol. 28, 263–270. doi: 10.1007/BF00175099
Creel S., Creel N. M. (1995). Communal hunting and pack size in African wild dogs, Lycaon pictus. Anim. Behav. 50, 1325–1339. doi: 10.1016/0003-3472(95)80048-4
Creel S., Creel N. M. (2002). The African wild dog: behavior, ecology, and conservation Vol. 25 (Princeton, NJ: Princeton University Press). Available at: https://books.google.co.uk/books?hl=en&lr=&id=ys4ZSof2BnMC&oi=fnd&pg=PR11&dq=Creel+and+Creel+2002&ots=QwIv1bxjX9&sig=isNnH_QANLHFgRPlH-ErXRjaVps&redir_esc=y#v=onepage&q=Creel%20and%20Creel%202002&f=false
Creel S., Reyes de Merkle J., Goodheart B., Mweetwa T., Mwape H., Simpamba T., et al. (2024). An integrated population model reveals source-sink dynamics for competitively subordinate African wild dogs linked to anthropogenic prey depletion. J. Anim. Ecol. 93, 417–427. doi: 10.1111/1365-2656.14052
Estes J. A., Terborgh J., Brashares J. S., Power M. E., Berger J., Bond W. J., et al. (2011). Trophic downgrading of planet Earth. Science 333, 301–306. doi: 10.1126/science.1205106
Fanshawe J. H., Fitzgibbon C. D. (1993). Factors influencing the hunting success of an African wild dog pack. Anim. Behav. 45, 479–490. doi: 10.1006/anbe.1993.1059
Fehlmann G., O’Riain M. J., Hopkins P. W., O’Sullivan J., Holton M. D., Shepard E. L., et al. (2017). Identification of behaviours from accelerometer data in a wild social primate. Animal Biotelemetry. 5, 1–11.
Goodheart B., Creel S., Becker M. S., Vinks M., Schuette P., Banda K., et al. (2021). Low apex carnivore density does not release a subordinate competitor when driven by prey depletion. Biol. Conserv. 261, 109273. doi: 10.1016/j.biocon.2021.109273
Gorman M. L., Mills M. G., Raath J. P., Speakman J. R. (1998). High hunting costs make African wild dogs vulnerable to kleptoparasitism by hyaenas. Nature 391, 479–481. doi: 10.1038/35131
Greener J. G., Kandathil S. M., Moffat L., Jones D. T. (2022). A guide to machine learning for biologists. Nat. Rev. Mol. Cell Biol. 23, 40–55. doi: 10.1038/s41580-021-00407-0
Gunner R. M., Holton M. D., Scantlebury M. D., van Schalkwyk L., English H. M., Williams H. J., et al. (2021). Dead-reckoning animal movements in R–A reappraisal using Gundog.Tracks. Animal Biotelemetry, 9, pp.1-37. doi: 10.1186/s40317-021-00245-z
Hayati H., Mahdavi F., Eager D. (2019). Analysis of agile canine gait characteristics using accelerometry. Sensors 19, 4379. doi: 10.3390/s19204379
Hubel T. Y., Myatt J. P., Jordan N. R., Dewhirst O. P., McNutt J. W., Wilson A. M. (2016). Energy cost and return for hunting in African wild dogs and cheetahs. Nat. Commun. 7, 11034. doi: 10.1038/ncomms11034
Hunter L. (2018). Carnivores of the world Vol. 117 (Priceton, NJ: Princeton University Press). Available at: https://books.google.co.uk/books?hl=en&lr=&id=gz9xDwAAQBAJ&oi=fnd&pg=PA1&dq=Hunter,+L.+(2018).+Carnivores+of+the+world+Vol.+117+(Princeton+University+Press).&ots=BUJCOXBDvN&sig=ABSnOZJ8axm439BH4MILcwpKkJQ&redir_esc=y#v=onepage&q&f=false
Lindemann B., Müller T., Vietz H., Jazdi N., Weyrich M. (2021). A survey on long short-term memory networks for time series prediction. Proc. Cirp 99, 650–655. doi: 10.1016/j.procir.2021.03.088
Lipton Z. C., Berkowitz J., Elkan C. (2015). A critical review of recurrent neural networks for sequence learning. arXiv preprint arXiv:150600019. doi: 10.48550/arXiv.1506.00019
Loarie S. R., Tambling C. J., Asner G. P. (2013). Lion hunting behaviour and vegetation structure in an African savanna. Anim. Behav. 85, 899–906. doi: 10.1016/j.anbehav.2013.01.018
Maulud D., Abdulazeez A. M. (2020). A review on linear regression comprehensive in machine learning. J. Appl. Sci. Technol. Trends 1, 140–147. doi: 10.38094/jastt1457
McNeill E. P., Thompson I., Wiebe P. A., Street G. M., Shuter J., Rodgers A. R., et al. (2020). Multi-scale foraging decisions made by woodland caribou (Rangifer tarandus caribou) in summer. Can. J. zool. 98, 331–341. doi: 10.1139/cjz-2019-0197
Meunier C. L., Boersma M., Declerck S. A., Laspoumaderes C. (2023). How sharp is the knife? Herbivore and carnivore sensitivity to resource stoichiometric quality. Oikos 2023, e09898. doi: 10.1111/oik.v2023.i9
Mortlock E., English H., Fitzsimmons J., Börger L., Jennings D. J., Capellini I. (2024). Early life sleep in free-living fallow deer, Dama dama: the role of ontogeny, environment and individual differences. Anim. Behav. 211, pp.163-180. doi: 10.1016/j.anbehav.2024.03.006
Nagy K. A., Siegfried W. R., Wilson R. P. (1984). Energy utilization by free-ranging jackass penguins, Spheniscus demersus. Ecology 65, 1648–1655. doi: 10.2307/1939143
Parrish F. A., Craig M. P., Ragen T. J., Marshall G. J., Buhleier B. M. (2000). Identifying diurnal foraging habitat of endangered Hawaiian monk seals using a seal-mounted video camera. Mar. Mammal Sci. 16, 392–412. doi: 10.1111/j.1748-7692.2000.tb00932.x
Perrin N., Sibly R. M. (1993). Dynamic models of energy allocation and investment. Annu. Rev. Ecol. Systematics 24, 379–410. doi: 10.1146/annurev.es.24.110193.002115
Qasem L., Cardew A., Wilson A., Griffiths I., Halsey L. G., Shepard E. L., et al. (2012). Tri-axial dynamic acceleration as a proxy for animal energy expenditure; should we be summing values or calculating the vector? PloS One 7, e31187. doi: 10.1371/journal.pone.0031187
Reyes de Merkle J., Creel S., Becker M. S., Goodheart B., Mweetwa T., Mwape H., et al. (2024). Long-term data reveal fitness costs of anthropogenic prey depletion for a subordinate competitor, the African wild dog (Lycaon pictus). Ecol. Evol. 14, e11402. doi: 10.1002/ece3.11402
Ripple W. J., Newsome T. M., Wolf C., Dirzo R., Everatt K. T., Galetti M., et al. (2015). Collapse of the world’s largest herbivores. Sci. Adv. 1, e1400103. doi: 10.1126/sciadv.1400103
Scantlebury D. M., Mills M. G., Wilson R. P., Wilson J. W., Mills M. E., Durant S. M., et al. (2014). Flexible energetics of cheetah hunting strategies provide resistance against kleptoparasitism. Science 346, 79–81. doi: 10.1126/science.1256424
Shepard E. L., Wilson R. P., Halsey L. G., Quintana F., Laich A. G., Gleiss A. C., et al. (2008). Derivation of body motion via appropriate smoothing of acceleration data. Aquat. Biol. 4, 235–241. doi: 10.3354/ab00104
Wilson R. P., Börger L., Holton M. D., Scantlebury D. M., Gómez-Laich A., Quintana F., et al. (2020). Estimates for energy expenditure in free-living animals using acceleration proxies: A reappraisal. J. Anim. Ecol. 89, 161–172. doi: 10.1111/1365-2656.13040
Wilson R., Holton M., d. Virgilio A., Williams H., Shepard E., Lambertucci S., et al. (2018a). Give the machine a hand: A Boolean time-based decision-tree template for rapidly finding animal behaviors in multisensor data. Methods Ecol. Evol. 9, 2206. doi: 10.1111/mee3.2018.9.issue-11
Wilson R. P., Neate A., Holton M. D., Shepard E. L., Scantlebury D. M., Lambertucci S. A., et al. (2018b). Luck in food finding affects individual performance and population trajectories. Curr. Biol. 28, 3871–3877. e5. doi: 10.1016/j.cub.2018.10.034
Wilson R. P., Rose K. A., Gunner R., Holton M. D., Marks N. J., Bennett N. C., et al. (2021). Animal lifestyle affects acceptable mass limits for attached tags. Proc. R. Soc. B 288, 20212005. doi: 10.1098/rspb.2021.2005
Wilson R. P., Shepard E., Liebsch N. (2008). Prying into the intimate details of animal lives: use of a daily diary on animals. Endangered species Res. 4, 123–137. doi: 10.3354/esr00064
Keywords: African wild dogs, accelerometry, hunts, kills, VeDBA, movement, pitch angle
Citation: Redcliffe J, Creel S, Goodheart B, Reyes de Merkle J, Matsushima SS, Mungolo M, Kabwe R, Kaseketi E, Donald W, Kaluka A, Chifunte C, Becker MS and Wilson R (2024) Using triaxial accelerometry to detect hunts and kills by African wild dogs. Front. Ecol. Evol. 12:1465094. doi: 10.3389/fevo.2024.1465094
Received: 15 July 2024; Accepted: 06 September 2024;
Published: 09 December 2024.
Edited by:
Pascual López-López, University of Valencia, SpainReviewed by:
Greg Breed, University of Alaska Fairbanks, United StatesCopyright © 2024 Redcliffe, Creel, Goodheart, Reyes de Merkle, Matsushima, Mungolo, Kabwe, Kaseketi, Donald, Kaluka, Chifunte, Becker and Wilson. This is an open-access article distributed under the terms of the Creative Commons Attribution License (CC BY). The use, distribution or reproduction in other forums is permitted, provided the original author(s) and the copyright owner(s) are credited and that the original publication in this journal is cited, in accordance with accepted academic practice. No use, distribution or reproduction is permitted which does not comply with these terms.
*Correspondence: James Redcliffe, amFtZXMucmVkY2xpZmZlQHN3YW5zZWEuYWMudWs=
Disclaimer: All claims expressed in this article are solely those of the authors and do not necessarily represent those of their affiliated organizations, or those of the publisher, the editors and the reviewers. Any product that may be evaluated in this article or claim that may be made by its manufacturer is not guaranteed or endorsed by the publisher.
Research integrity at Frontiers
Learn more about the work of our research integrity team to safeguard the quality of each article we publish.