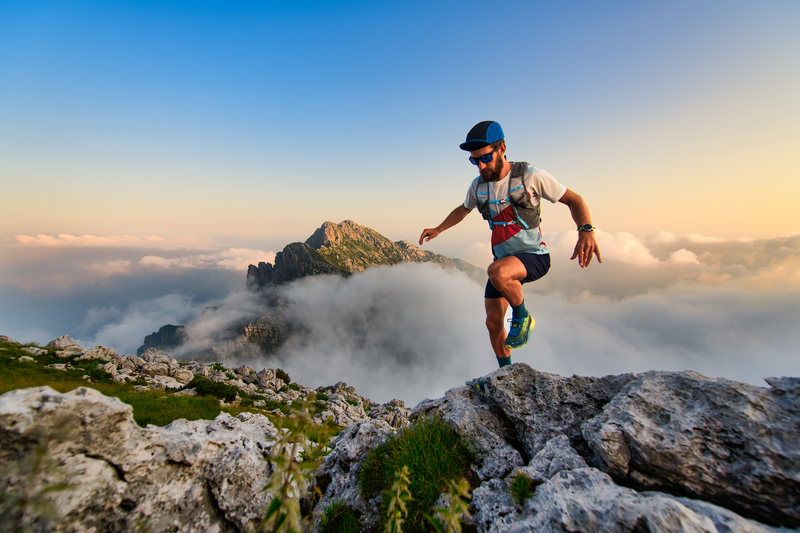
95% of researchers rate our articles as excellent or good
Learn more about the work of our research integrity team to safeguard the quality of each article we publish.
Find out more
ORIGINAL RESEARCH article
Front. Ecol. Evol. , 21 October 2024
Sec. Conservation and Restoration Ecology
Volume 12 - 2024 | https://doi.org/10.3389/fevo.2024.1431779
Introduction: The implementation of ecological agriculture practices in Chinese tea gardens plays a vital role in mitigating N2O emissions and addressing environmental degradation. Nevertheless, a dearth of discourse exists regarding the intricacies surrounding farmers' adoption of tea garden ecological agriculture practices (TGEAP), particularly the complex interplay between adoption factors and outcomes.
Methods: Using data of 310 farmers, this study employed complexity theories and Stimulus-Organism-Response theories, and integrated Partial Least Squares Structural Equation Modeling and fuzzy-set Qualitative Comparative Analysis to explore the complex relationships between farmer characteristics, internal and external factors, and adoption of farmer.
Results: The results show three influential paths in the Stimulus-Organism-Response model, and environmental protection attitude (EPA) and production expectation (PE) act as intermediaries. Notably, EPA exhibits a masking effect in one pathway. These paths linked closely to three farmer characteristics. Beyond the Stimulus-Organism-Response model, nine combinations lead to farmers' adoption, and four to non-adoption.
Discussion: We discover scenarios where opposing environmental states or age lead to adoption, explaining the masking effect. These combinations highlight how a favorable environment influences both adoption and non-adoption. We also discuss other combinations that lead to adoption or non-adoption. The study suggests that governments employ targeted incentives to facilitate tea farmers' transition in agriculture.
Globally, tea is a crucial natural resource, playing a pivotal role in the economic advancement of numerous developing nations through its production and circulation (Kalita et al., 2019). Moreover, it serves as a vital livelihood source for millions of smallholder tea growers globally (74th session of the United Nations General Assembly, 2022). Nevertheless, current research indicates that N2O emissions from tea garden soil surpass those from cereal farmland soil, establishing it as a focal point in global agricultural N2O emission hotspots (Wang et al., 2020). In the 21st century, the significant release of greenhouse gases has emerged as a global challenge impeding human progress (Balat, 2006; IPCC, 2022). Given the scale of tea cultivation and consumption, coupled with other greenhouse gas emissions from tea cultivation, tea has emerged as a central focus in global agricultural greenhouse gas reduction efforts (Liang et al., 2021). Simultaneously, the excessive utilization of agricultural inputs has contributed to the ecological degradation of tea gardens and the presence of pesticide residues in tea beverages, which hinder the sustainable livelihood of tea growers (Xu et al., 2021; Sun R. et al., 2021). These problems are evident in China. China is the world’s largest tea producer. In 2020, China’s tea garden area and tea production account for 62.1% and 47.6% of the world’s total, respectively (China Tea Marketing Association, 2022). Due to the government’s rural revitalization strategy, the scale of China’s tea industry has been expanding in recent years and has become an important industrial pillar of China’s agricultural sector (Liang et al., 2021). However, agricultural inputs are still heavily used, challenges of greenhouse gas emissions and ecological degradation remain, and there has been no substantial improvement in China’s tea gardens. Similar to China, the application of chemical fertilizers and pesticides has long been a tradition for tea growers in Vietnam. The overuse of agricultural inputs, such as fertilizers and pesticides, has also caused soil fertility loss and soil acidification in Vietnamese tea plantations, reducing tea production and quality, posing a threat to human health, and resulting in lower income and unsustainable production of tea plantations (Le et al., 2021). Kenya (Kagira et al., 2012), Sri Lanka (Piyathilake and Udayakumara, 2023) and India (Deka and Goswami, 2021), the world’s major tea-producing countries, are also facing this problem.
In order to solve this problem, the practice of ecological agriculture in tea gardens has been promoted worldwide. The Chinese government has begun to promote various ecological and low-carbon practices in tea garden management, and in 2021, the specifications for ecological and low-carbon tea garden practices were established. The tea garden ecological agriculture practice(TGEAP) covers many links such as soil management, grass damage prevention, and defines the application of organic fertilizer, grass control, and artificial weeding as ecological low-carbon tea garden management technologies (Tea Research Institute, Chinese Academy of Agricultural Sciences, 2021). This is a sustainable eco-agricultural practice (Nwosu and Oshunsanya, 2021). In Vietnam, the government has promoted Good Agricultural Practices in tea plantations since 2008 (Van Ho et al., 2019). In Kenya, international collaboration with FAO, China, and Germany has led to the adoption of shade trees, tea pruning, and organic fertilizers to reduce greenhouse gas emissions (Food and Agriculture Organization of the United Nations, 2024). India’s National Organic Production Program has encouraged the adoption of organic tea cultivation by promoting third-party certification (Deka and Goswami, 2022). In these major tea-producing countries, tea growers have been the primary managers of tea gardens and the key implementers of ecological agricultural practices.
Nevertheless, as a type of farmer, tea growers, like other farmers, have high heterogeneity in their behaviors and decisions. This poses a challenge to the popularization and application of tea garden eco-agriculture practices. Enhancing the understanding of the practices adopted by farmers, especially their heterogeneity, is of great significance for the promotion of agricultural practices (Karali et al., 2013).
The practice of eco-agriculture adopted by farmers has been the focus of the academic community for a long time. The initial study believed that farmers’ motivation to adopt practices was profit-driven, but subsequent studies showed that both environmental and profit-oriented factors play an important role in adoption behavior (Gedikoglu and McCann, 2012; Willock et al., 1999). In a review, economic and environmental attitudes are also the most frequently analyzed factors (Bartkowski and Bartke, 2018). In subsequent studies, many studies have incorporated production expectation drive and environmental attitude drive into the theory for analysis. Several notable examples include the Theory of Planned Behavior, the Technology Acceptance Model, and the Theory of Innovation Diffusion (Serebrennikov et al., 2020; Adnan et al., 2019). The researchers studied adoption of eco-agriculture practices from a wide range of perspectives, including personalities, beliefs, government, risk appetite, information sources, and broader farmer characteristics (Serebrennikov et al., 2020). Specifically in the field of tea growers, some studies have explored the influencing factors of tea growers’ adoption of TGEAP from three aspects: economy, psychology, and government (Iqbal et al., 2006; Lou et al., 2021; Li et al., 2022).
In many studies, traditional causal analysis methods such as logistic regression (Malila et al., 2023), structural equation modeling (Tapsoba et al., 2023), propensity score matching (Li et al., 2021), and experimental selection (Christensen et al., 2011) have been employed by researchers to investigate the impact of farmer characteristics on the adoption of sustainable ecological agriculture. For example, Iqbal et al. (2006) used the Logit model to examine the key factors affecting the adoption of rubber-tea intercropping by small tea growers in Sri Lanka. Lou et al. (2021) used SEM to analyze eco-friendly pest management practices adopted by green tea growers in China. Li et al. (2022) used Probit and Logit models to analyze the role of relational embedment in the government’s development of green tea production demonstration households.
However, these conventional causal analysis methods face challenges in capturing the complex interdependencies between input and output variables, especially when utilizing regression-based models. The intricate interdependence among these factors yields diverse or varying results, making the interpretation of such models complex and challenging (Hayat et al., 2022). Therefore, some scholars have turned to non-traditional causal asymmetry analysis methods such as fsQCA. Dong et al. (2023) utilized the fsQCA method to analyze the adoption of environmentally friendly land conservation techniques by farmers (Dong et al., 2023). Similarly, some scholars have used fsQCA to analyze causality in the adoption of conservation agriculture practices (Hayat et al., 2022; Zhang et al., 2022).
The combination of PLS-SEM and fsQCA methods allows for the simultaneous integration of the strengths of both traditional and non-traditional causal analysis methods. This approach helps identify causal impact mechanisms and uncover other asymmetrical causal relationships. Therefore, Dong et al. utilized the PLS-SEM method to analyze the relationships between behavioral attitudes, subjective norms, perceived behavioral control, and farmers’ willingness to adopt rice prawn cropping technology. Subsequently, they employed the fsQCA method to identify four high intention configurations regarding the acceptance of high-tech agricultural practices (Dong et al., 2022a).
In these studies employing non-traditional methods, researchers have not sufficiently addressed individual factors, especially those related to farmer characteristics. This is true in other domains of research focused on various agricultural behaviors (Wu et al., 2023; Jin et al., 2022; Lee et al., 2022).Simultaneously, while researchers have categorized the influencing factors on farmers’ adoption practices into individual factors based on farmers and external factors originating outside farmers, there has been limited emphasis on certain external variables. Specifically, factors such as technology information acquisition (Liu et al., 2022; Xiuling et al., 2023), the agricultural production environment (Marenya and Barrett, 2009; Wang et al., 2016), and agricultural input supply and services (Cai et al., 2021; Shi et al., 2023) have received scant attention, despite evidence of their impact on farmers’ behavior. The mediation roles of the two most common influencing factors, profit-based production expectations and environmental conservation attitudes, in the process of how these external variables exert their impact have not been clearly elucidated. Additionally, there is limited research analyzing individual factors related to profit-driven production expectations and environmentally conscious attitudes (Hayat et al., 2022; Small et al., 2016; Khatri-Chhetri et al., 2017).
Although researchers have made a lot of efforts to explain the huge differences in farmer behavior, because farmers are a highly diverse group, the differences in physical and temporal aspects of agricultural practices, as well as the high variability of the determinants of practice, will complicate the study of farmer behavior (Reimer et al., 2014). Research on farmers’ use of agricultural practices often focuses on behavioral factors but fails to capture the complex relationship between farmer characteristics and farmer behavior.
According to Bente Castro Campos, farmers’ behaviors related to agricultural practices cannot be simply analyzed in isolation. Behavioral factors (cognition, will, emotion) and non-behavioral factors (sociodemographic characteristics) should be considered in a complex space. In the ignoring of farmer characteristics background, this often leads to a large number of unexplained variations (Campos, 2022).
Therefore, through a literature review, we can find that many studies have explored the influencing factors of tea growers adopting TGEAP from three aspects: economy, psychology, and government (Iqbal et al., 2006; Lou et al., 2021; Li et al., 2022). Nonetheless, there is a lack of research on the relationship between external variables and the dual drivers of profit-based production expectations and environmental protection attitudes. Similarly, there is a lack of discussion on the intricate relationships between adoption behavior and influencing factors. In the broader field of farmer adoption of eco-agriculture practices, it is still difficult to enhance the interpretation of the complexity of farmer behavior and determine the complex relationship between farmer characteristics and other factors. In this context, the main purpose of this study is to establish an effective research framework and delineate the pathways through which these external variables, influence tea growers’ adoption of TGEAP by the dual factors of production expectation and environmental protection attitude. Simultaneously, it investigates how the intricate relationships between farmer characteristics and other factors contribute to the tea growers’ adoption.
In view of this, the comprehensive methods of PLS-SEM and fsQCA were used in this study. Under the theoretical framework of the S-O-R theoretical model and complexity theory, we discuss how the farmer’s characteristic factors and other factors are complicated to lead to tea growers’ adoption of TGEAP. Finally, this study identifies multiple intermediate paths in which external variables influence the adoption. The study also reveals various configurations of influencing factors, demonstrating significant differences among these combinations, yet all leading to the adoption of TGEAP by tea growers. The factors configurations leading to tea growers not adopting eco-agricultural practices are also revealed. The research framework used in this study can be effectively used in the practice of eco-agriculture adopted by tea growers, and can also provide references for explaining the complex of other farmer behaviors. At the same time, this study also provides a scientific basis and policy recommendations for the promotion of TGEAP.
Complexity theory holds that the determinative factors are essentially causal conditions, and the combination of multiple antecedent conditions under different circumstances ultimately leads to the result (Chen et al., 2023). Especially in real life, outcomes are usually the result of a combination of various antecedents rather than a single combination (Kaya et al., 2020). Most empirical studies have employed traditional symmetry-based methods to study outcomes between variables. However, these methods emphasize the net impact of each influencing factor on each outcome, ignoring possible asymmetrical relationships between variables in complex environments. The introduction of complexity theory can determine the asymmetric relationship between variables and find different combinations of factors that lead to the same result (Chaparro-Peláez et al., 2016). Due to the strong heterogeneity of tea growers, and the existence of multiple complex causal relationships within the characteristics of tea growers and between the characteristics of tea growers and their behaviors, the behaviors of tea growers also show the characteristics of diversity and complexity. This situation lends itself to analysis using complexity theory.
Based on this, we take complexity theory as an important theoretical perspective and apply it to research. Age, family size, production number, and annual income are four farmer characteristic factors. Together with the five factors of no-farmer characteristic factors, they are the nine antecedent conditions for tea growers to adopt ecological tea garden management practices. And from the perspective of complexity theory, we discuss the combination of factors that lead to tea growers’ adoption of TGEAP.
S-O-R defines a stimulus (S) as a specific cue that affects an individual’s internal state, and an organism (O) as an individual’s cognitive process that begins to function once the individual receives a stimulus from a specific cue (Sultan et al., 2021). And the response (R) is the result that the individual gets from the stimulus and the organism (Robert and John, 1982). Because S-O-R theory can provide a flexible framework to explain the effects of various internal and external, tangible and intangible stimuli on individual psychology and individual behavior. Therefore, it has achieved wide success and application in many fields such as tourism (Yang et al., 2022), food consumption (Sultan et al., 2021) and education (Anwar et al., 2023).
Profit based production expectations and environmental protection attitudes have been regarded as important internal drivers for farmers to adopt eco-agricultural practices (Bartkowski and Bartke, 2018). Faced with new agricultural practices, farmers calculate the expected consequences of adopting them and are more willing to adopt them when production is expected to increase returns (Ding et al., 2023). Thus, external variables can enhance farmers’ adoption of new agricultural practices by increasing their production expectations. Concern for the environment is an important non-economic motivation for agricultural producers to decide to adopt green practices, farmers’ moral obligation and emotional resonance may affect their subjective views on ecological practices, and then affect adoption of practices (Xiong et al., 2016). The moral obligation and emotional resonance are all influenced by individual farmers and external factors. Tea garden production environment as an external physical factor, agricultural input supply and services, and technical information acquisition as two external social factors, will affect production expectations and environmental protection attitude. And they may further influence tea growers’ adoption of TGEAP. Therefore, we introduced the S-O-R model, taking tea garden production environment, technical information acquisition, agricultural input supply and services as external stimulus variables (S), and discussing how the external stimulus variable (S), through two internal driving factors (O), ultimately affects tea growers’ adoption of ecological agriculture practices (R).
Technical information acquisition can be divided into active information seeking and passive information receiving by tea growers. The sources include communication among communities, publicity of government policies by media (Boz and Ozcatalbas, 2010), and technical training (Liu et al., 2022). Interpersonal communication, policy advocacy, and technical training are typical forms of external environments. By introducing external technical knowledge and information, it is possible to effectively change endowment constraints, correct the information asymmetry between farmers and technology adoption, increase the correct perception of agricultural practices, and ultimately improve the production expectations of agroecological practices (Xiuling et al., 2023).
Environmental protection attitude includes the environmental emotion of tea growers and the price they are willing to pay for environmental protection. Previous studies have shown that value perception has direct and indirect positive effects on ecological behavior, and the indirect effects are mediated by environmental attitudes (Meng and Si, 2022). Environmental knowledge can enhance individual environmental emotion, and thus promote the implementation of individual ecological behavior (Carmi et al., 2015). In addition, technical information acquisition about eco-agricultural practices often increases an individual’s knowledge of environmental protection, and the more knowledge about environmental protection, the more willing they are to take on environmental improvement (Liu et al., 2023). Therefore, suppose:
H1: Technical information acquisition can have a positive impact on tea growers’ adoption of TGEAP by enhancing production expectations.
H2: Technical information acquisition can have a positive impact on tea growers’ adoption of TGEAP by enhancing environmental protection attitudes.
The tea garden production environment is mainly characterized by soil and water sources. As an external physical factor, the production environment will affect the technology adoption of tea growers. Especially in the context of promotion of organic agriculture production, a good ecological environment is often associated with high-quality agricultural products. Farmers may think that a good environment will bring high-quality tea products and a broad market and have higher ecological technology production expectations (Chi and Chine, 2022), thus promoting farmers to carry out ecological production. Farmers’ awareness of the local environmental situation will also have an impact on their environmental protection attitudes and behaviors (Gachango et al., 2015). In the case of poor environmental conditions, farmers choose to avoid the bad environment and show a negative attitude towards environmental protection because they cannot bear the financial cost of changing the environment (Liu et al., 2018). On the contrary, when farmers are in a good production environment, because the cost of changing the environment is relatively low, they show a positive attitude towards environmental protection, and promote farmers to adopt eco-agriculture practices. The research on environmental education also shows that the connection with nature as an internal motivation can enhance the acceptance of environmental education, and promote the ecological behavior of individuals (Otto and Pensini, 2017). Therefore, suppose:
H3: The tea garden production environment can have a positive impact on tea growers’ adoption of TGEAP by enhancing production expectations.
H4: The tea garden production environment can have a positive impact on tea growers’ adoption of TGEAP by enhancing environmental protection attitudes.
Agricultural input supply and services refer to the difficulty of obtaining agricultural inputs such as fertilizers and pesticides and the guidance of their use after obtaining them. As a part of the input of agricultural production factors, agricultural inputs directly affect the products output and affect farmer’s income (Sibande et al., 2017). Existing studies have shown that the more convenient the supply of agricultural materials, the more likely farmers are to use ecological agricultural practices (Huang et al., 2019). As rational economic human tea growers, the decrease in the cost of acquiring and using materials means that the higher the expected production income, the more likely they are to adopt eco-agriculture practices. Therefore, suppose:
H5: Agricultural input supply and services can have a positive impact on tea growers’ adoption of TGEAP by enhancing production expectations.
There is a complex relationship between farmer characteristics and non-farmer characteristics, and these variables may affect tea growers’ adoption. Based on the above assumptions and reasoning, this study proposed a model as shown in Figure 1.
Anxi County is located in the southeast of Fujian Province. The region has a tea cultivation history for nearly a thousand years, with a yearly production of 75,600 tons of tea (Anxi County Statistics Bureau, National Bureau of Statistics Anxi survey team, 2021) and mountainous terrain suitable for the growth of tea trees (Anxi County People's Government, 2017). Anxi Tieguanyin tea culture system was recognized as a globally important agricultural cultural heritage by the Food and Agriculture Organization of the United Nations in 2022 and is a typical representative of China’s tea production (Food and Agriculture Organization of the United Nations, 2022). In this study, Anxi County was selected as the research site, and household interviews and questionnaires were conducted among tea growers in Anxi County from July 2022 to August 2023. 342 questionnaires were issued, and 310 were valid, with an effective rate of 90.64%.The map of the study area is shown in Figure 2.
We calculated the demographic characteristics of tea growers (Supplementary Table S1). In this survey, people between 40 and 55 years old account for 55.5%, which is consistent with the current situation of aging in rural China (Lou et al., 2021). In terms of household population size, tea growers with a population of 4–6 individuals accounted for more than half of the sample, this reflects that the current tea growers are mainly small-scale families. Tea growers with two tea production labor forces accounted for 49.2%. This is mainly because the joint production of husband and wife is the most common way of family labor input. The income than 120,000 yuan accounted for a relatively large proportion (41.3%), and the low tea income level (income less than 30,000 yuan and income of 30,000–60,000 yuan) also accounted for a certain proportion (7.1% and 17.7%). This reflects that the tea growers have a higher income, but a considerable proportion of tea growers are still relatively poor.
The tea growers’ adoption of TGEAP is the dependent variable, which is composed of three items: fertilizer type, fertilization method, and weeding method. The values of these items are based on a scale of 1–3. Tea growers who have never adopted ecological agriculture practices are set as 1, tea growers who have fully adopted ecological agriculture practices are set as 3, and those who are between the two types are actually set as 2.
On the basis of referring to existing studies and combining the actual situation, this study selected technical information acquisition, agricultural input supply and services and tea garden production environment in stimulus (S) as the core explanatory variables, and selected production expectation and environmental protection attitude in organism (O) as the mediating variables, and tested their mediating role in PLS-SEM analysis. After PLS-SEM analysis, this study added four farmer characteristics, such as age, family size, number of tea producers, and annual income, into the fsQCA analysis to determine the combination of farmer characteristics and other variables that lead tea growers to adopt TGEAP. The specific items and descriptive statistics of each variable are shown in Table 1. The specific contents of variable assignment and meaning are shown in the Supplementary Materials (Supplementary Table S2).
In this study, we used a composite method based on PLS-SEM and fsQCA (Rasoolimanesh et al., 2021). PLS-SEM is based on ordinary least squares (OLS) regression, which can model complex relationships between variables (Sukhov et al., 2023). Especially when the research goal is to test the theoretical framework from a predictive perspective and to understand complex relationships by advancing established theories, PLS-SEM has a good effect (Rasoolimanesh et al., 2021).The fsQCA method is based on asymmetric set theory and has good results in understanding connectivity causality, equivalence, and causal asymmetry. Therefore, it can deal with the problem that traditional research methods ignore asymmetric relationships between variables in complex environments, identify different combinations of independent variables that lead to the realization of results, and reveal patterns that are ignored by other methods due to their asymmetric logic (Sukhov et al., 2023; Chuah et al., 2021).
In this study, the PLS-SEM method was used to analyze the S-O-R model to determine the feasible influence path and the prediction ability of the model, and the latent variable factor scores of each factor under the S-O-R model were obtained. Then, this study takes age, number of tea producers, family size, and annual income as the characteristics of farmers, and includes them in the fsQCA analysis together with the latent variable factor scores of each factor under the S-O-R model. Under a unified research framework, we analyzed the necessity of each factor to encourage tea growers to adopt TGEAP and not to encourage tea growers to adopt TGEAP and identify the combination of factors that lead to the two different outcomes.
In order to ensure the stability of the research results, we refer to the practice guide of Rasoolimanesh et al. (2021). The analysis results are presented in Tables 2 and 3. An external load of 0.7 or higher is considered very satisfactory, while 0.5 is considered acceptable (Hulland, 1999). The estimation results of the PLS path model show that the majority of indicators have loadings greater than 0.7, with one indicator exceeding 0.65, and the reliability of the model is supported (Rasoolimanesh et al., 2021). Cronbach’s Alpha and Composite Reliability are both greater than 0.7, which has sufficient internal consistency and composite reliability (Dong et al., 2022b). AVE is greater than 0.5, meeting the convergence threshold of more than 0.5. VIF is less than 5, HTMT is less than 0.85, and both test results meet the requirements, indicating that the multicollinearity test and discriminant validity test of this study have passed, there is no multicollinearity between the measured variables, and the discriminant validity of the construction is supported (Rasoolimanesh et al., 2021; Dong et al., 2022b). In general, the robustness of the measurement model is appropriate, and the next SEM and fsQCA analysis can be carried out.
In this study, Bootstrapping based on 5000 samples was used to consider the path coefficients and their significance and R-square values. The analysis results are presented in Table 4.The path coefficients ranged from −0.22 to 0.382, and hypothesis H1, H2, and H4 passed the significance test (p<0.05). Some assumptions between access to technical information acquisition, agricultural input supply and services, tea garden production environment, production expectation, environmental protection attitude, and tea growers’ adoption of TGEAP are supported. The R-square values of environmental protection attitudes, production expectations, and TGEAP are 0.1707, 0.1429, and 0.2308, respectively. Considering the complexity of the model and similar situations in the field of farmer behavior (Bathaiy et al., 2021), the R-square values of the empirical modeling in the field of social science are reasonable when the values are within 0.1–0.5 and most explanatory variables are significant (Ozili, 2023). Therefore, we believe that the R-square values of environmental protection attitudes, production expectations, and TGEAP are acceptable.
A series of results of the hypothesis H4 all pass the significance test, and these results indicate that tea garden production environment plays a significant and negative role in influencing tea growers’ adoption. This suggests that adverse tea garden production environments promote tea growers’ adoption, while favorable environments hinder it, and environmental protection attitudes act as a mediator in this process. However, the mediation path has an opposite direction to the path coefficients and total effects about tea garden production environment → tea growers’ adoption of TGEAP, indicating that environmental protection attitudes have a masking effect in this influencing process. Good tea garden production environments negatively affect tea growers’ adoption but can increase adoption by enhancing tea growers’ environmental protection attitudes, thus offsetting the negative impact of the tea garden production environment on adoption.
H1 and H2 are both supported by the study results. Technical information acquisition has a positive and significant impact on tea growers’ adoption of TGEAP through both production expectations and environmental protection attitudes, with production expectations and environmental protection attitudes playing significant mediating roles in this process (p values all less than 0.05). Regarding H3, the results show that the hypothesis is not significantly supported in the PLS-SEM analysis (p>0.05). This suggests that a good tea garden production status cannot promote tea growers’ adoption practices by increasing their production expectations. Regarding H5, a series of results all did not pass the significance test. This indicates that agricultural input supply and services cannot significantly influence tea growers’ adoption.
The outcomes of our study reveal that within the S-O-R model, both technical information acquisition and tea garden production environment exert significant effects on tea growers’ adoption through 3 pathways. Environmental protection attitudes and production expectations act as mediators in the S-O-R model. However, agricultural input supply and services do not have a significant impact on tea growers’ adoption. The research findings support hypotheses H1, H2, and H4, while hypotheses H3 and H5 are not supported.
To assess and validate the predictive capability of the research model, we utilized the cross-validated predictive ability test (CVPAT) for model evaluation. In the prediction-based model assessment, average loss values were compared with the average values obtained using the Indicator Average (IA) as a naive benchmark. PLS-SEM average loss should be lower than the benchmark’s average loss, with a negative difference indicating a reduction in average loss, and if the negative difference is significantly less than 0, it suggests that the model has strong predictive capability (Cuevas et al., 2023). Results analysis indicates that the model’s predictive capability surpasses that of IA, demonstrating the model’s strong predictive performance. The analysis results are shown in Table 5.
This study uses PLS-SEM latent variable scores as part of the dataset analyzed by fsQCA. Due to the limitation of the number of conditional variables in fsQCA analysis, we combined the results of previous studies on tea growers and took four variables as the characteristics of farmers (age, annual income, number of tea producers, and family size). Then, fsQCA analysis was performed using four farmer characteristic variables and five non-farmer characteristic variables (technical information acquisition, agricultural input supply and services, tea garden production environment, environmental protection attitudes, production expectations) as condition variables. In order to enhance the explanatory power, we not only analyze the tea growers’ adoption of TGEAP, but also analyze the tea growers’ non-adoption of TGEAP by using fsQCA method to deal with the characteristics of causal asymmetry (Chen et al., 2023).
Before fsQCA analysis can be performed, the data set needs to be transformed into a fuzzy set. The value of a fuzzy set ranges from 0 to 1, where 0 means that it does not belong to the set at all, 1 means that it belongs to all, and 0.5 means the intersection between the two. Since most of the study data did not show a normal distribution, we determined the calibration threshold based on the distribution of the variable values, the median, and the standard error (Supplementary Table S3).
Necessity analysis can check whether each condition variable is a necessary condition for adoption. If the result of necessity analysis exceeds the threshold value of 0.9, it indicates that this condition variable is a necessary condition for adopt (Jovanovic and Morschett, 2022). Our necessity analysis results show that there are no necessary conditions for both tea growers’ adoption and non-growers’ adoption, which indicates that we need to further analyze how different conditional variables are combined to form tea growers’ adoption (Supplementary Table S4).
The truth table algorithm can determine the relationship between different combinations of condition variables and the result (Nikou et al., 2022). After simplifying the truth table, a meaningful combination of conditional variables can be formed to explain the formation of tea growers’ adoption. When performing truth table operations, it is necessary to determine the raw consist threshold of the truth table. We adopt the mainstream practice of existing fsQCA analysis, set the threshold is 0.79. The raw consist of non-growers’ adoption is 0.8 (Supplementary Tables S5 and S6).
Based on the results of the truth table, we form the combined analysis results of tea growers’ adoption and non-growers’ adoption. The conditions present in the simple solution have an important effect on the result, the conditions under which only intermediate solutions occur are edge conditions, called auxiliary contribution conditions (Huang and Bu, 2023). We use the current broad practice of fsQCA to only report the intermediate solution and the simple solution, because the intermediate solution has the same configuration path as the complex solution. This study also reports the overall coverage and overall consistency of farmer adoption and non-farmer adoption. The overall coverage was 0.426814 and 0.450596, respectively, exceeding the threshold of 0.25, and the overall consistency was 0.76189 and 0.839613, respectively, exceeding the threshold of 0.75, indicating that these combinations all had a good explanation on the whole (Chen et al., 2023).
Our research findings suggest that there are numerous configurations of antecedent conditions leading to tea growers’ adoption and to tea growers not adopting. Additionally, we integrated the results from the PLS-SEM analysis and fsQCA analysis to categorize the configurations leading to tea growers’ adoption into 9 types, and configurations leading to non-adoption into 4 types, as illustrated in Figures 3 and 4.
Figure 3. The type of tea growers’adoption of TGEAP. Type 1, includes configuration combinations 2,3, 6and 9. Type 2, includes configuration combinations 4,5,7,8 and 18. Type 3, includes configuration combinations 1,2, 6and 17. Type 4 includes configuration combinations 1,3 and 14. Type 5 includes configuration combinations 9 and 18. Type 6 includes configuration combinations 11 and 12. Type 7 includes configuration combinations 15 and 16. Type 8 includes configuration combinations 10. Type 9 includes configuration combinations 14. A thick line indicates that all configuration combinations in the type satisfy this anthems, and thin line indicates that only some configuration combinations meet this antecedent condition. The solid line indicates that the antecedent condition is in a high degree, that is, sufficient state; The dashed line indicates that the antecedent condition is in a low degree, that is, the absence state.
Figure 4. The type of tea growers’non-adoption of TGEAP. Type 10, includes configuration combinations 1,3,4,7,9,16,21. Type 11, includes configuration combinations 10,12,13,24,25. Type 12, includes configuration combinations 2,5,6,8,11,14,15,18,19. Type 13, includes configuration combinations 17,20,22,23. A thick line indicates that all configuration combinations in the type satisfy this anthems, and thin line indicates that only some configuration combinations meet this antecedent condition. The solid line indicates that the antecedent condition is in high degree, that is, sufficient state; The dashed line indicates that the antecedent condition is in a low degree, that is, the absence state.
The PLS-SEM and fsQCA analysis results together show that there are many types of combinations that lead tea growers to adopt, which shows the complexity of tea grower behavior.
We used the method of Shahrokh Nikou et al. to test robustness by changing the consistency threshold, and if the results are very different due to a slight change in the threshold, it indicates that the combination scheme is not stable enough (Nikou et al., 2022). In this study, robustness tests were performed using raw consistency thresholds of 0.75 and 0.8 for tea growers’ adoption and 0.75 and 0.82 for tea growers’ non-adoption. We present the raw conformance adjusted results with the original results and compare them, and the results are acceptable (Supplementary Tables S7 and S8).
In this study, PLS-SEM and fsQCA were used to investigate the influence path and factor configuration combination of tea growers’ adoption of TGEAP. Based on PLS-SEM analysis, it was determined that the influence paths of technical information acquisition and tea garden production environment on tea growers’ adoption. Part of the results based on fsQCA analysis are consistent with the results of PLS-SEM, while the other part reveals the influence patterns that PLS-SEM fails to find, which have also been ignored by previous studies.
In general, types 1 and 5 support the establishment of the adoption path of the tea garden production environment and technical information acquisition influencing tea growers under the S-O-R model, and the PLS-SEM analysis results confirm each other with the fsQCA analysis results, further enhancing the authenticity of this theoretical model. The combination of farmers’ characteristics and the S-O-R model shows that under the conditions limited by the S-O-R model, low age, high annual income, and sufficient number of tea producers are effective factors to promote tea growers to adopt ecological agriculture. This is particularly evident in Type 1. This result is consistent with many studies (Ul Haq et al., 2021; Qiao et al., 2022; Xie and Huang, 2021).
One explanation for the high probability of younger tea growers adoption is at the level of personal attitudes. Younger tea growers may be more receptive to new technologies because they may be less dogmatic in their belief system of agricultural practices and have a welcoming attitude towards environmental protection (Liu Y. et al., 2019). Older tea growers will be more likely to rely on and prefer the experience of traditional farming (Pan et al., 2017). Our establishment of the mediating role of environmental protection attitudes and antecedent conditions combination supports this, however, in our study, we believe that it should be understood from a more complex and comprehensive perspective.
In types 1, 3, and 5, high incomes and sufficient producers have always been the influencing conditions. The combination of high income and low age appeared in types 1 and 3. This shows a very close relationship between low age and high income, and between high income and sufficient producers. Lower-age tea growers tend to have higher incomes and more labor to produce tea. This may be because younger tea growers have a variety of options in employment, and if agriculture income is low, they are likely to switch to non-farm employment (Zhang et al., 2017). Only the higher-earning tea growers are willing to stay for agricultural production and devote more labor to it. As a result, this group of lower-age tea growers has higher income, has enough capital and labor to try new practices, and is more likely to accept the concept of environmental protection.
This explanation is applicable to Type 1 and can also elucidate why low states of tea garden production environment become significant antecedent conditions in Type 3. In adverse tea garden production environments, tea growers, due to the financial costs associated with changing the environment, may opt to escape the adverse environments. This avoidance of adverse environments, coupled with a negative orientation toward environmental protection attitudes, hinders tea growers’ adoption (Liu et al., 2018). However, within the younger age group, where there is a higher proportion of professional farmers (Zhang et al., 2017), tea growers are more inclined to use TGEAP for long-term considerations. With the goal of improving the tea garden production environment, younger tea growers are more motivated to adopt TGEAP, leading to a higher adoption rate in this age group.
However, older tea growers also adopt TGEAP, as evidenced in Types 2 and 5. In these types, a high level of these factors – age, agricultural input supply and services, technical information acquisition, income, and environmental protection attitudes – are important components of their antecedent conditions. We posit that this situation is associated with technical information acquisition addressing cognitive limitations associated with older age, and agricultural input supply and services mitigating labor constraints associated with older age. Pan et al. (2017) showed that after receiving knowledge training, the intensity of fertilizer use of middle-aged and older wheat farmers decreased more than that of younger farmers. Our study showed that with a high annual income and sufficient number of tea producers, the higher the degree of tea growers’ technical information acquisition, the more likely they are to get rid of the cognitive limitations caused by age differences, which encourages tea growers of different ages to adopt eco-agriculture practices.
High agricultural input supply and services is one of the important sufficient conditions for the adoption of practices by older farmers (Liverpool-Tasie et al., 2017). Aging will reduce the labor capacity of farmers (Zhang et al., 2019), including the ability to obtain agricultural inputs. The difficulty of obtaining ecological agricultural inputs required is often higher than that of traditional agriculture (Janjhua et al., 2019). Therefore, for older tea growers, when it is difficult to supply agricultural resources and services, they will be more inclined to traditional agricultural practices with sufficient materials and easier access and utilization. However, when the supply of agricultural resources is convenient, the restrictions on the ability of older tea growers to obtain agricultural inputs are reduced, and older tea growers can adopt eco-agriculture. The academic community generally believes that older tea growers have more experience and knowledge, but they do not adopt new technologies due to their own declining labor capacity, risk aversion, and resistance to new technologies (Foguesatto et al., 2020; Lee and Gambiza, 2022; Branca and Perelli, 2020). And high agricultural input supply and services, coupled with high technical information acquisition, evidently helps mitigate negative influencing factors on tea growers’ adoption, thereby promoting adoption.
Notably, we found that the opposite tea garden production environment state can lead tea growers to adopt, that is, the opposite production environment state between type 3, 6 and type 5, 7. This provides an explanation for the masking effect of the production environment in influencing tea growers’ adoption. There are two scenarios in which a favorable production environment leads to adoption. In the first scenario, a good production environment encourages tea growers to reduce the use of chemical inputs and increase the input of other eco-agricultural materials. Simultaneously, as tea growers find it relatively cost-effective to change the environment in a good production setting, they exhibit a positive environmental protection attitude, promoting the adoption of eco-agricultural practices (Liu et al., 2018; Otto and Pensini, 2017). The motivation for adoption in these cases stems from production expectations and environmental protection attitudes, as evident in Type 5. In the second scenario, despite lacking production expectations for TGEAP and maintaining lower environmental protection attitudes, tea growers have sufficient agricultural inputs, a good production environment, and ample capital investment. In this situation, farmers have the potential to try new practices (Ul Haq et al., 2021; Xie and Huang, 2021), as observed in Type 7. However, the occurrence of this scenario is relatively low, with coverage rates for its configuration combinations being 0.037 and 0.0348.
The poor tea garden production environment leading to adoption manifests in two scenarios. There are two categories of adoption: active and passive. The first scenario is evident in Type 3, where the younger age group, with a higher proportion of professional farmers (Zhang et al., 2017), is more willing to use TGEAP to improve the production environment. This group is oriented towards improving the production environment, directly resulting in a higher willingness among younger tea growers to adopt TGEAP. High income and high producers provide sufficient capital to try new technologies (Ul Haq et al., 2021; Xie and Huang, 2021). High technical information acquisition increases tea growers’ environmental protection attitudes and production expectations, enhancing their adoption motivation. The second scenario is reflected in Type 6. Technical information acquisition, agricultural input supply and services, production environment, production expectations, and environmental protection attitudes all exist in Type 6 at low states, while producers and family size exist at high states. In this situation, tea growers find it challenging to access information about new agricultural technologies from the exterior, and they also face a harsh environment with higher production costs. The poor production environment is sometimes combined with the weak capital factors, which is consistent with previous studies (Reardon and Vosti, 1995). Economic constraints force tea growers to reduce production capital investment, leading them to adopt practices that require more labor but save economic capital, achieving low-cost input. Tea growers in this category adopt eco-agriculture more out of livelihood constraints than voluntary willingness.
The analysis results of PLS-SEM and fsQCA also indicate that different tea garden production environment states lead to adoption. Types with a favorable production environment and adoption have lower coverage rates, with coverage rates of 0.037 and 0.0348 for the involved configuration combinations. This aligns with the lower path coefficient in the PLS-SEM analysis for production environment → environmental protection attitudes → tea growers’ adoption of TGEAP (β=0.024). Types with a poor production environment leading to adoption have higher coverage rates. This corresponds to the strong negative impact of production environment on adoption of TGEAP in the PLS-SEM analysis (β=−0.22).
Combining the complex situations regarding tea growers’ adoption based on different environmental conditions, along with the analysis results from PLS-SEM and fsQCA, we argue that both favorable and poor environmental conditions can lead to adoption. Among them, the type where poor environmental conditions lead to tea growers’ adoption dominates. Although favorable environmental conditions can also result in tea growers’ adoption, with some influence mediated by environmental protection attitudes, the number of cases is relatively low. It is the presence of this type that weakens the significant negative impact of production environment on tea growers’ adoption of TGEAP from −0.22 (Path Coefficients) to −0.179 (Total Effects).
Additionally, in Type 8, even though other antecedent conditions are at low states, technical information acquisition and environmental protection attitudes are at high states and serve as core conditions. This implies that, even in the absence of other conditions, acquiring technical information about TGEAP can still promote tea growers’ adoption practices. Type 9 reveals that a high state of agricultural input supply and services and a high state of production expectations may still lead to tea growers’ adoption, although the path agricultural input supply and services → production expectations → tea growers’ adoption of TGEAP in the PLS-SEM analysis has a p-value of only 0.068.
Our results regarding tea growers’ non-adoption practices indicate 4 types of combinations of antecedent conditions. Overall, a good tea garden production environment often leads to non-adoption by tea growers.
The first type is the dual constraint type of information and capital, as manifested in Type 10. Knowler D. et al. argued that land fertility can negatively impact adoption because farmers with high-yielding soils may pay less attention to the conservation of their land (Knowler and Bradshaw, 2007), aligning with our findings. In situations with low states of technical information acquisition, tea growers lack positive production expectations for TGEAP, and due to their favorable tea garden production environment, their adoption motivation is further weakened. Additionally, these tea growers lack the corresponding investment capital. Therefore, this scenario means that in a favorable production environment, tea growers find more marginal benefits from using conventional agriculture, particularly in terms of fertilizer input. The second type is the lack of motivation type, as evidenced in Type 11. In a good tea garden production environment, tea growers, following the traditional production model, already have sufficient income. Additionally, due to inadequate agricultural inputs and low production expectations, tea growers lack the motivation to undergo practice transformation.
The third type is the absence of external variables leading to internal motivation deficiency, as observed in Type 12. Because technical information acquisition and the tea garden production environment are both at low states, they inhibit environmental protection attitudes and production expectations, thereby leading to tea growers’ non-adoption. This result confirms the mutual verification of the S-O-R model assumptions and supports some derivations of tea growers’ adoption. The fourth type is the dual constraint of motivation and capital, shown in Type 13. The producer number and income are at low levels, indicating that tea growers lack the capital to adopt new practices. Furthermore, tea growers’ environmental protection attitudes and production expectations are also not high. Therefore, even if tea growers have an adequate supply of agricultural inputs and a good production environment, they still do not adopt TGEAP.
This study still has some limitations. First of all, due to the limitation of data availability, this study lacks an in-depth analysis of the complexity of tea grower adoption from the time dimension. Secondly, this study only studied tea growers and did not analyze other types of farmers. As for the limitations of this study, future studies can work on these aspects, future studies can further carry out a more detailed investigation on the types of farmers, increase the sample size of data, and conduct a comprehensive and in-depth analysis on the adoption behavior of farmers from the time and space dimensions, so as to break through the limitations of this research and expand the research depth of existing studies.
Technical information acquisition can significantly influence tea growers’ adoption through production expectations and environmental protection attitudes. The tea garden production environment can positively affect tea growers’ adoption through environmental protection attitudes. However, adverse tea garden production environments also have a significant and positive impact on tea growers’ adoption. Environmental protection attitudes exhibit a masking effect in the impact of the tea garden production environment on tea growers’ adoption. Low age, high income, and an abundant number of producers, combined with the production environment and technical information acquisition, lead to tea growers’ adoption. Both low and high age can lead to tea growers’ adoption, each with different combinations of antecedent conditions. Low age is a core characteristic of farmers adopting eco-agriculture. However, high-age tea growers also adopt, often in conjunction with high states of agricultural inputs and services and high states of technical information acquisition variables. These combinations go beyond the theoretical assumptions of the S-O-R model. Both favorable and poor states of the tea garden production environment can lead to tea growers’ adoption, presenting a distinction from the results expected under the S-O-R theoretical model. In contrast to the S-O-R model’s assumptions, the favorable production environment not only promotes adoption but can also lead to non-adoption. The favorable production environment can induce tea growers’ adoption through environmental protection attitudes. The adoption resulting from poor production conditions can be classified into two types: one is adopted voluntarily, and the other is adopted due to livelihood constraints. There are 4 types of combinations of antecedent conditions leading to tea growers’ non-adoption. These include the double constraint of information and capital, the lack of motivation type, the dual constraint of motivation and capital, and the internal lack of motivation due to the absence of external variables.
Based on the research results, we put forward corresponding policies and suggestions: (1) Strengthen the dissemination of eco-agricultural technology information, increase the information sources and channels for tea growers to obtain information about eco-agriculture practices, improve the supply services of rural agricultural resources, and provide convenient conditions for tea growers to implement eco-agriculture practices. By improving the terms of technical information acquisition, agricultural input supply, and services, it can improve production expectations and environmental protection attitudes, and promote the practice adoption. (2) The government needs to conduct a more detailed investigation on the production environment of tea gardens and the capital of tea growers, so as to accurately identify and assess whether tea growers are suitable for switching to eco-agricultural practices, and adopt targeted encouragement policies. This is because there are many types of tea growers’ non-adoption, and different factors restrict them. For example, when tea growers do not adopt ecological agriculture because they lack financial capital support and production expectations but have sufficient agricultural labor force, and a good production environment, then the government can promote the adoption of eco-agriculture practices by giving preferential subsidy policies and credit support.
The original contributions presented in the study are included in the article/Supplementary Material, further inquiries can be directed to the corresponding author.
Written informed consent was obtained from the individual(s) for the publication of any potentially identifiable images or data included in this article.
ZY: Data curation, Formal analysis, Investigation, Methodology, Resources, Software, Validation, Visualization, Writing – original draft. WZ: Investigation, Supervision, Writing – review & editing, Formal analysis, Software, Validation, Visualization, Writing – original draft. XL: Investigation, Software, Validation, Visualization, Writing – review & editing. ZL: Conceptualization, Data curation, Formal analysis, Investigation, Supervision, Writing – review & editing. YZ: Formal analysis, Investigation, Writing – review & editing. MZ: Data curation, Investigation, Methodology, Resources, Writing – review & editing. SG: Conceptualization, Data curation, Funding acquisition, Investigation, Project administration, Resources, Supervision, Writing – review & editing.
The author(s) declare financial support was received for the research, authorship, and/or publication of this article. This research was funded by Innovation Fund Project of Special Talent Innovation Laboratory of Fujian Richun Industrial Co., grant number RCTL2024001, and Science and Technology Innovation Fund of Fujian Zhang Tianfu Tea Development Foundation, grant number FJZTF01.
ChatGPT3.5 was used to assist with the translation and check English grammar.
Authors ZY and SG was employed by the company Fujian Richun Industrial Company Limited.
The remaining authors declare that the research was conducted in the absence of any commercial or financial relationships that could be construed as a potential conflict of interest.
All claims expressed in this article are solely those of the authors and do not necessarily represent those of their affiliated organizations, or those of the publisher, the editors and the reviewers. Any product that may be evaluated in this article, or claim that may be made by its manufacturer, is not guaranteed or endorsed by the publisher.
The Supplementary Material for this article can be found online at: https://www.frontiersin.org/articles/10.3389/fevo.2024.1431779/full#supplementary-material
74th session of the United Nations General Assembly. (2022). Available online at: https://documents-dds-ny.un.org/doc/UNDOC/GEN/N19/437/86/pdf/N1943786.pdf (Accessed December 14, 2022). International Tea Day.
Adnan N., Nordin S. M., Bahruddin M. A., Tareq A. H. (2019). A state-of-the-art review on facilitating sustainable agriculture through green fertilizer technology adoption: Assessing farmers behavior. Trends Food Sci. Tech 86, 439–452. doi: 10.1016/j.tifs.2019.02.040
Anwar I., Ahmad A., Saleem I., Yasin N. (2023). Role of entrepreneurship education, passion and motivation in augmenting Omani students’ entrepreneurial intention: A stimulus-organism-response approach. Int. J. Manag Educ-Oxf 21, 100842. doi: 10.1016/j.ijme.2023.100842
Anxi County People's Government. (2017). Geography overview of Anxi. Available online at: http://fjax.gov.cn/zjax/axgl/201707/t20170717_789803.htm (Accessed June 1, 2022).
Anxi County Statistics Bureau, National Bureau of Statistics Anxi survey team. (2021). 2020 Anxi County National Economic and Social Development Statistical Bulletin. Available online at: http://www.fjax.gov.cn/zwgk/zfxxgkzl/zfxxgkml/tjxx/202104/t20210401_2537014.htm (Accessed June 1, 2022).
Balat M. (2006). Energy and greenhouse gas emissions: A global perspective. Energ Source Part B. 1, 157–170. doi: 10.1080/009083190881571
Bartkowski B., Bartke S. (2018). Leverage points for governing agricultural soils: A review of empirical studies of european farmers’ Decision-making. Sustainability 10, 3179. doi: 10.3390/su10093179
Bathaiy S. S., Chizari M., Sadighi H., Alambeigi A. (2021). Social media and farmer's resilience to drought as an environmental disaster: A moderation effect. Int. J. Disast Risk Re 59, 102209. doi: 10.1016/j.ijdrr.2021.102209
Boz I., Ozcatalbas O. (2010). Determining information sources used by crop producers: A case study of Gaziantep province in Turkey. Afr J. Age Res. 5, 980–987. doi: 10.5897/AJAR09.130
Branca G., Perelli C. (2020). [amp]]lsquo;Clearing the air’: common drivers of climate-smart smallholder food production in Eastern and Southern Africa. J. Clean Prod 270, 121900. doi: 10.1016/j.jclepro.2020.121900
Cai B., Shi F., Huang Y., Abatechanie M. (2021). The impact of agricultural socialized services to promote the farmland scale management behavior of smallholder farmers: empirical evidence from the rice-growing region of southern China. Sustainability 14, 316. doi: 10.3390/su14010316
Campos B. C. (2022). The Rules-Boundaries-Behaviours (RBB) framework for farmers' adoption decisions of sustainable agricultural practices. J. Rural Stud. 92, 164–179. doi: 10.1016/j.jrurstud.2022.03.012
Carmi N., Arnon S., Orion N. (2015). Transforming environmental knowledge into behavior: The mediating role of environmental emotions. J. Environ. Educ. 46, 183–201. doi: 10.1080/00958964.2015.1028517
Chaparro-Peláez J., Agudo-Peregrina Á.F., Pascual-Miguel F. J. (2016). Conjoint analysis of drivers and inhibitors of e-commerce adoption. J. Bus Res. 69, 1277–1282. doi: 10.1016/j.jbusres.2015.10.092
Chen M., Wang H., Liang Y., Zhang G. (2023). Net and configurational effects of determinants on cloud computing adoption by SMEs under cloud promotion policy using PLS-SEM and fsQCA. J. Innov. Knowl. 8, 100388. doi: 10.1016/j.jik.2023.100388
Chi S. Y., Chine L. H. (2022). Influence of ecologicaland quality concerns on the adoption intention of environment-smart agricultural systems. Appl. Ecol. Env. Res. 20, 363–381. doi: 10.15666/aeer/2001_363381
China Tea Marketing Association. (2022). World Tea Production and Marketing Situation Report. Available online at: https://www.ctma.com.cn/xiehuidongtai/72288.html (Accessed December 14, 2022).
Christensen T., Pedersen A. B., Nielsen H. O., Mørkbak M. R., Hasler B., Denver S. (2011). Determinants of farmers’ willingness to participate in subsidy schemes for pesticide-free buffer zones—A choice experiment study. Ecol. Econ. 70, 1558–1564. doi: 10.1016/j.ecolecon.2011.03.021
Chuah S. H. W., Tseng M. L., Wu K. J., Cheng C. F. (2021). Factors influencing the adoption of sharing economy in B2B context in China: Findings from PLS-SEM and fsQCA. Resour Conserv. Recy 175, 105892. doi: 10.1016/j.resconrec.2021.105892
Cuevas V. H., Parga M. N., Lozano G. J. J., Huerta M. E. (2023). Determinants of openness activities in innovation: The mediating effect of absorptive capacity. J. Innov. Knowl. 8, 100432. doi: 10.1016/j.jik.2023.100432
Deka N., Goswami K. (2021). What will drive the small tea growers towards environment-friendly cultivation? Implications from the tea sector in Assam, India. Climate And Dev. 14, 443–458. doi: 10.1080/17565529.2021.1930988
Deka N., Goswami K. (2022). Organic cultivation and sustainable value chain development for tea smallholders: Findings from Assam, India. Sustain. Production Consumption 32, 562–579. doi: 10.1016/j.spc.2022.05.004
Ding X., Lu Q., Li L., Sarkar A., Li H. (2023). Does labor transfer improve farmers’ Willingness to withdraw from farming?—A bivariate probit modeling approach. Land. 12, 1615. doi: 10.3390/land12081615
Dong H., Wang H., Han J. (2022a). Understanding ecological agricultural technology adoption in China using an integrated technology acceptance model—theory of planned behavior model. Front. Env. Sci-Switz. 10. doi: 10.3389/fenvs.2022.927668
Dong H., Wang B., Han J., Luo L., Wang H., Sun Z., et al. (2022b). Understanding farmers’ eco-friendly fertilization technology adoption behavior using an integrated SOR model: The case of soil testing and formulated fertilization technology in Shaanxi, China. Front. Env. Sci-Switz 10. doi: 10.3389/fenvs.2022.991255
Dong H., Zhou H., Wei J., Chen T., Wu X. (2023). How is it effective for farmers to adopt environmentally friendly cultivated land protection technology: a configurational perspective. Front. Env. Sci-Switz 11. doi: 10.3389/fenvs.2023.1130403
Foguesatto C. R., Borges J. A. R., MaChado J. A. D. (2020). A review and some reflections on farmers' adoption of sustainable agricultural practices worldwide. Sci. Total Environ. 729, 138831. doi: 10.1016/j.scitotenv.2020.138831
Food and Agriculture Organization of the United Nations. (2022). Anxi Tieguanyin Tea Culture System, China. Available online at: https://www.fao.org/giahs/giahsaroundtheworld/designated-sites/asia-and-the-pacific/anxi-tea-cultures-system/detailed-information/en (Accessed June 2, 2022).
Food and Agriculture Organization of the United Nations. (2024). Form Crop To Cup Working With Kenya To Make Tea Low Carbon. Available online at: https://www.fao.org/climate-change/news/news-detail/form-crop-to-cup–working-with-Kenya-to-make-tea-low-carbon/ (Accessed May 21, 2024).
Gachango F. G., Andersen L. M., Pedersen S. M. (2015). Adoption of voluntary water-pollution reduction technologies and water quality perception among Danish farmers. Agr Water Manage 158, 235–244. doi: 10.1016/j.agwat.2015.04.014
Gedikoglu H., McCann L. M. (2012). Adoption of win-win, environment-oriented, and profit-oriented practices among livestock farmers. J. Soil Water Conserv. 67, 218–227. doi: 10.2489/jswc.67.3.218
Hayat N., Al Mamun A., Salameh A. A., Yang Q., Zainol N. R., Makhbul Z. K. M. (2022). A fuzzy-set analysis of conservative agriculture practice adoption: role of farmer orientations and attitude. Front. Psychol. 13. doi: 10.3389/fpsyg.2022.876912
Huang Y., Bu Y. (2023). Institutional environment and college students’ entrepreneurial willingness: A comparative study of Chinese provinces based on fsQCA. J. Innov. Knowl. 8, 100307. doi: 10.1016/j.jik.2023.100307
Huang Y., Luo X., Liu D., Yu W., Tang L. (2019). Factors influencing the adoption of organic fertilizer instead of chemical fertilizer by farmers: an explanation of the phenomenon of high willingness and low behavior. Resour. Environ. Yangtze Basin. 28, 632–641. doi: 10.11870/cjlyzyyhj201903014
Hulland J. (1999). Use of partial least squares (PLS) in strategic management research: A review of four recent studies. Strategic Manage J. 20, 195–204. doi: 10.1002/(SICI)1097-0266(199902)20:2<195::AID-SMJ13>3.0.CO;2-7
IPCC. (2022). Climate change 2022:Mitigation of Climate Change (Summary for Policymakers) (Cambridge: Cambridge University Press), 8.
Iqbal S. M., Ireland C., Rodrigo V. (2006). A logistic analysis of the factors determining the decision of smallholder farmers to intercrop: A case study involving rubber–tea intercropping in Sri Lanka. Agr Syst. 7, 296–312. doi: 10.1016/j.agsy.2005.02.002
Janjhua Y., Chaudhary R., Mehta P., Kumar K. (2019). Determinants of farmer’s attitude toward organic agriculture and barriers for converting to organic farming systems: Research insights. Int. J. Economic Plants 6, 97–103. doi: 10.23910/IJEP/2019.6.2.0303
Jin Y., Lin Q., Mao S. (2022). Tanzanian farmers’ Intention to adopt improved maize technology: analyzing influencing factors using SEM and fsQCA methods. Agriculture 12, 1991. doi: 10.3390/agriculture12121991
Jovanovic J., Morschett D. (2022). Under which conditions do manufacturing companies choose FDI for service provision in foreign markets? An investigation using fsQCA. Ind. Market Manage. 104, 38–50. doi: 10.1016/j.indmarman.2022.03.018
Kagira E. K., Kimani S. W., Githii K. S. (2012). Sustainable methods of addressing challenges facing small holder tea sector in Kenya: A supply chain management approach. J. Mgmt. Sustainability 2, 75. doi: 10.5539/jms.v2n2p75
Kalita R. M., Das A. K., Nath A. J. (2019). Role of smallholder tea growers in carbon sink management. Curr. Science. 9, 1560–1566. doi: 10.18520/cs/v116/i9/1560-1566
Karali E., Brunner B., Doherty R., Hersperger A. M., Rounsevell M. D. (2013). The effect of farmer attitudes and objectives on the heterogeneity of farm attributes and management in Switzerland. Hum. Ecol. 41, 915–926. doi: 10.1007/s10745-013-9612-x
Kaya B., Abubakar A. M., Behravesh E., Yildiz H., Mert I. S. (2020). Antecedents of innovative performance: Findings from PLS-SEM and fuzzy sets (fsQCA). J. Bus Res. 114, 278–289. doi: 10.1016/j.jbusres.2020.04.016
Khatri-Chhetri A., Aggarwal P. K., Joshi P. K., Vyas S. (2017). Farmers' prioritisation of climate-smart agriculture (CSA) technologies. Agric. Syst. 151, 184–191. doi: 10.1016/j.agsy.2016.10.005
Knowler D., Bradshaw B. (2007). Farmers’ adoption of conservation agriculture: A review and synthesis of recent research. Food Policy 32, 25–48. doi: 10.1016/j.foodpol.2006.01.003
Le V. S., Lesueur D., Herrmann L., Hudek L., Quyen L. N., Brau L. (2021). Sustainable tea production through agroecological management practices in Vietnam: a review. Environ. Sustainability 4, 589–604. doi: 10.1007/s42398-021-00182-w
Lee M., Gambiza J. (2022). The adoption of conservation agriculture by smallholder farmers in southern Africa: A scoping review of barriers and enablers. J. Rural Stud. 92, 214–225. doi: 10.1016/j.jrurstud.2022.03.031
Lee J. H., Joo D., Lee C. K., Parkt Y. N., Kwon Y. J. (2022). The role of residents' sustainable intelligence in agricultural heritage site management: Insights from PLS-SEM and Fs/QCA. J. Hosp Tour Manag 52, 65–74. doi: 10.1016/j.jhtm.2022.06.004
Li L., Sarkar A., Zhou X., Ding X., Li H. (2022). Influence and action mechanisms of governmental relations embeddedness for fostering green production demonstration household: evidence from Shaanxi, Sichuan, and Anhui Province, China. Int. J. Env. Res. Pub He 19, 11923. doi: 10.3390/ijerph191911923
Li C., Shi Y., Khan S. U., Zhao M. (2021). Research on the impact of agricultural green production on farmers’ technical efficiency: Evidence from China. Environ. Sci. pollut. R. 28, 38535–38551. doi: 10.1007/s11356-021-13417-4
Liang L., Ridoutt B. G., Wang L., Xie B., Li M., Li Z. (2021). China’s tea industry: Net greenhouse gas emissions and mitigation potential. Agriculture. 11, 363. doi: 10.3390/AGRICULTURE11040363
Liu J., Huang S., Wang Y. (2023). Study of farmers’ Willingness to participate in environmental governance based on recycling, reduction and resourcing. Sustainability 15, 10850. doi: 10.3390/su151410850
Liu K., Huisingh D., Zhu J., Ma Y., O'Connor D., Hou D. (2018). Farmers' perceptions and adaptation behaviours concerning land degradation: A theoretical framework and a case-study in the Qinghai–Tibetan Plateau of China. Land Degrad Devl. 29, 2460–2471. doi: 10.1002/ldr.3011
Liu Y., Ruiz-Menjivar J., Zhang L., Zhang J., Swisher M. E. (2019). Technical training and rice farmers’ adoption of low-carbon management practices: the case of soil testing and formulated fertilization technologies in Hubei, China. J. Clean Prod. 226, 454–462. doi: 10.1016/j.jclepro.2019.04.026
Liu Y., Shi R., Peng Y., Wang W., Fu X. (2022). Impacts of technology training provided by agricultural cooperatives on farmers’ adoption of biopesticides in China. Agriculture 12, 316. doi: 10.3390/agriculture12030316
Liverpool-Tasie L. S. O., Omonona B. T., Sanou A., Ogunleye W. O. (2017). Is increasing inorganic fertilizer use for maize production in SSA a profitable proposition? Evidence from Nigeria. Food Policy 67, 41–51. doi: 10.1016/j.foodpol.2016.09.011
Lou S., Zhang B., Zhang D. (2021). Foresight from the hometown of green tea in China: Tea farmers’ adoption of pro-green control technology for tea plant pests. J. Clean Prod 320, 128817. doi: 10.1016/j.jclepro.2021.128817
Malila B. P., Kaaya O. E., Lusambo L. P., Schaffner U., Kilawe C. J. (2023). Factors influencing smallholder Farmer's willingness to adopt sustainable land management practices to control invasive plants in northern Tanzania. Environ. Sustain Ind. 19, 100284. doi: 10.1016/j.indic.2023.100284
Marenya P. P., Barrett C. B. (2009). Soil quality and fertilizer use rates among smallholder farmers in Western Kenya. Agr Econ-Blackwell 40, 561–572. doi: 10.1111/j.1574-0862.2009.00398.x
Meng L., Si W. (2022). Pro-environmental behavior: examining the role of ecological value cognition, environmental attitude, and place attachment among rural farmers in China. Int. J. Env. Res. Pub He 19, 17011. doi: 10.3390/ijerph192417011
Nikou S., Mezei J., Liguori E. W., El Tarabishy A. (2022). FsQCA in entrepreneurship research: Opportunities and best practices. J. Small Bus Manage. 62, 1–18. doi: 10.1080/00472778.2022.2147190
Nwosu N. J., Oshunsanya S. O. (2021). “Management of agricultural lands for sustainable crop cultivation and greenhouse gas reduction,” in Handbook of Climate Change Management. Eds. Leal Filho W., Luetz J., Ayal D. (Springer Press, Singapore), 1–28. doi: 10.1007/978-3-030-22759-3_104-1
Otto S., Pensini P. (2017). Nature-based environmental education of children: Environmental knowledge and connectedness to nature, together, are related to ecological behaviour. Global Environ. Chang 47, 88–94. doi: 10.1016/j.gloenvcha.2017.09.009
Ozili P. K. (2023). “The acceptable R-square in empirical modelling for social science research,” in Social Research Methodology and Publishing Results: A Guide to Non-Native English Speakers. Ed. Saliya C. A. (IGI Global, Hershey, Pennsylvania, USA), 134–143. doi: 10.2139/ssrn.4128165
Pan D., Kong F., Zhang N., Ying R. (2017). Knowledge training and the change of fertilizer use intensity: Evidence from wheat farmers in China. J. Environ. Manage 197, 130–139. doi: 10.1016/j.jenvman.2017.03.069
Piyathilake I. D. U. H., Udayakumara E. P. N. (2023). “Effects of herbicides application on the sustainability of tea production in Sri Lanka,” in Proceedings of the 2nd International Symposium on Disaster Resilience and Sustainable Development, vol. 283 . Eds. Pal I., Shaw R., Ichinose T., Yonariza, Oda T. (Springer, Singapore).
Qiao D., Li N., Cao L., Zhang D., Zheng Y., Xu T. (2022). How agricultural extension services improve farmers’ Organic fertilizer use in China? The perspective of neighborhood effect and ecological cognition. Sustainability 14, 7166. doi: 10.3390/su14127166
Rasoolimanesh S. M., Ringle C. M., Sarstedt M., Olya H. (2021). The combined use of symmetric and asymmetric approaches: Partial least squares-structural equation modeling and fuzzy-set qualitative comparative analysis. Int. J. Contemp Hosp M 33, 1571–1592. doi: 10.1108/IJCHM-10-2020-1164
Reardon T., Vosti S. A. (1995). Links between rural poverty and the environment in developing countries: asset categories and investment poverty. World Dev. 23, 1495–1506. doi: 10.1016/0305-750X(95)00061-G
Reimer A., Thompson A., Prokopy L. S., Arbuckle J. G., Genskow K., Jackson-Smith D., et al. (2014). People, place, behavior, and context: A research agenda for expanding our understanding of what motivates farmers' conservation behaviors. J. Soil Water Conserv. 69, 57A–61A. doi: 10.2489/jswc.69.2.57A
Robert D., John R. (1982). Store atmosphere: an environmental psychology approach. J. Retailing 58, 34–57. doi: 10.2307/1991569
Serebrennikov D., Thorne F., Kallas Z., McCarthy S. N. (2020). Factors influencing adoption of sustainable farming practices in europe: A systemic review of empirical literature. Sustainability 12, 9719. doi: 10.3390/su12229719
Shi F., Cai B., Meseretchanie A., Geremew B., Huang Y. (2023). Agricultural socialized services to stimulate the green production behavior of smallholder farmers: the case of fertilization of rice production in south China. Front. Env. Sci-Switz 11. doi: 10.3389/fenvs.2023.1169753
Sibande L., Bailey A., Davidova S. (2017). The impact of farm input subsidies on maize marketing in Malawi. Food Policy 69, 190–206. doi: 10.1016/j.foodpol.2017.04.001
Small B., Brown P., Munguia O. M. D. (2016). Values, trust, and management in New Zealand agriculture. Int. J. Agric. Sustain 14, 282–306. doi: 10.1080/14735903.2015.1111571
Sukhov A., Friman M., Olsson L. E. (2023). Unlocking potential: An integrated approach using PLS-SEM, NCA, and fsQCA for informed decision making. J. Retail Consum Serv. 74, 103424. doi: 10.1016/j.jretconser.2023.103424
Sultan P., Wong H. Y., Azam M. S. (2021). How perceived communication source and food value stimulate purchase intention of organic food: An examination of the stimulus-organism-response (SOR) model. J. Clean Prod 12, 127807. doi: 10.1016/j.jclepro.2021.127807
Sun R., Yang W., Li Y., Sun C. (2021). Multi-residue analytical methods for pesticides in teas: a review. Eur. Food Res. Technol. 247, 1839–1858. doi: 10.1007/s00217-021-03765-3
Tapsoba P. K., Aoudji A. K., Ouédraogo F., Dassekpo I. S., Kestemont M. P., Konkobo M. K., et al. (2023). Under-standing the behavioral drivers of smallholder agro-ecological practice adoption in Benin and Burkina Faso. Farming System. 1, 100023. doi: 10.1016/j.farsys.2023.100023
Tea Research Institute, Chinese Academy of Agricultural Sciences. (2021). Guidelines for the Evaluation of Ecological Low-Carbon Tea. Available online at: https://tri.caas.cn/docs/2022-09/f0cac0419405425c88a5cc446d726c58.pdf (Accessed December 14, 2022).
Ul Haq S., Boz I., Shahbaz P. (2021). Sustainability assessment of different land tenure farming systems in tea farming: The effect of decisional and structural variables. Integr. Environ. Asses 17, 814–834. doi: 10.1002/ieam.4379
Van Ho B., Nanseki T., Chomei Y. (2019). Profit efficiency of tea farmers: case study of safe and conventional farms in Northern Vietnam. Environment Dev. Sustainability 21, 1695–1713. doi: 10.1007/s10668-017-0073-z
Wang N., Gao Y., Wang Y., Li X. (2016). Adoption of eco-friendly soil-management practices by smallholder farmers in Shandong Province of China. Soil Sci. Plant Nutr. 62, 185–193. doi: 10.1080/00380768.2016.1149779
Wang Y., Yao Z., Pan Z., Wang R., Yan G., Liu C., et al. (2020). Tea-planted soils as global hotspots for N2O emissions from croplands. Environ. Res. Lett. 15, 104018. doi: 10.1088/1748-9326/aba5b2
Willock J., Deary I. J., Edwards-Jones G., Gibson G. J., McGregor M. J., Sutherland A., et al. (1999). The role of attitudes and objectives in farmer decision making: business and environmentally-oriented behavior in Scotland. J. Agr Econ 50, 286–303. doi: 10.1111/j.1477-9552.1999.tb00814.x
Wu X., Nie X., Chen Z., Wang H., Kang Q., Lei W. (2023). How to improve the adaptive capacity of coastal zone farm house-holds?–explanation based on complementary and alternative relationships of livelihood capital in a sustainable livelihood framework. Ocean Coast. Manage 245, 106853. doi: 10.1016/j.ocecoaman.2023.106853
Xie H., Huang Y. (2021). Influencing factors of farmers' adoption of pro-environmental agricultural technologies in China: Meta-analysis. Land Use Policy 109, 105622. doi: 10.1016/j.landusepol.2021.105622
Xiong Y., Li X., He P. (2016). Farmers’ adoption of pollution-free vegetable farming in China: Economic, informational, or moral motivation? Cogent Food Agr 2, 1240022. doi: 10.1080/23311932.2016.1240022
Xiuling D., Qian L., Lipeng L., Sarkar A. (2023). The impact of technical training on farmers adopting water-saving irrigation technology: an empirical evidence from China. Agriculture 13, 956. doi: 10.3390/agriculture13050956
Xu Q., Yang Y., Hu K., Chen J., Djomo S. N., Yang X., et al. (2021). Economic, environmental, and emergy analysis of China's green tea production. Sustain Prod Consump. 28, 269–280. doi: 10.1016/j.spc.2021.04.019
Yang J., Zhang D., Liu X., Li Z., Liang Y. (2022). Reflecting the convergence or divergence of Chinese outbound solo travellers based on the stimulus-organism-response model: A gender comparison perspective. Tour Manag Perspect. 43, 100982. doi: 10.1016/j.tmp.2022.100982
Zhang Y., Lu X., Zhang M., Ren B., Zou Y., Lv T. (2022). Understanding farmers’ willingness in arable land protection cooperation by using fsQCA: Roles of perceived benefits and policy incentives. J. Nat. Conserv. 68, 126234. doi: 10.1016/j.jnc.2022.126234
Zhang J., Manske G., Zhou P. Q., Tischbein B., Becker M., Li Z. H. (2017). Factors influencing farmers’ decisions on nitrogen fertilizer application in the Liangzihu Lake basin, Central China. Environ. Dev. Sustain 19, 791–805. doi: 10.1007/s10668-016-9765-z
Keywords: ecological agricultural practice, farmers behavior, stimulus-organism-response theories, complexity theory, tea garden
Citation: Yan Z, Zhang W, Liu X, Li Z, Zhao Y, Zeng M and Gao S (2024) Complex factors combinations driving tea growers to adopt ecological agricultural practices in tea gardens. Front. Ecol. Evol. 12:1431779. doi: 10.3389/fevo.2024.1431779
Received: 12 May 2024; Accepted: 16 September 2024;
Published: 21 October 2024.
Edited by:
Abhay K. Pandey, North Bengal Regional R & D Center, IndiaReviewed by:
Sudipta Sankar Bora, Assam Agricultural University, IndiaCopyright © 2024 Yan, Zhang, Liu, Li, Zhao, Zeng and Gao. This is an open-access article distributed under the terms of the Creative Commons Attribution License (CC BY). The use, distribution or reproduction in other forums is permitted, provided the original author(s) and the copyright owner(s) are credited and that the original publication in this journal is cited, in accordance with accepted academic practice. No use, distribution or reproduction is permitted which does not comply with these terms.
*Correspondence: Shuilian Gao, Z2Fvc2h1aWxpYW5AMTI2LmNvbQ==
Disclaimer: All claims expressed in this article are solely those of the authors and do not necessarily represent those of their affiliated organizations, or those of the publisher, the editors and the reviewers. Any product that may be evaluated in this article or claim that may be made by its manufacturer is not guaranteed or endorsed by the publisher.
Research integrity at Frontiers
Learn more about the work of our research integrity team to safeguard the quality of each article we publish.